AI-Powered Pattern Detection offers a transformative approach to analyzing qualitative research data, allowing researchers to uncover insights efficiently. Traditional methods of manual data analysis can be time-consuming and prone to bias, often leading to inconsistencies in the findings. By integrating AI tools, researchers can streamline the analysis process, ensuring a more objective and faster generation of insights.
In this section, we explore how AI-Powered Pattern Detection enhances qualitative research. Utilizing advanced algorithms, these tools can identify recurring themes and trends within interview transcripts and survey responses. As a result, researchers can focus on interpreting the results rather than being bogged down by lengthy data processing. This shift not only expedites project timelines but also elevates the quality of insights derived from qualitative research efforts.
Analyze & Evaluate Calls. At Scale.
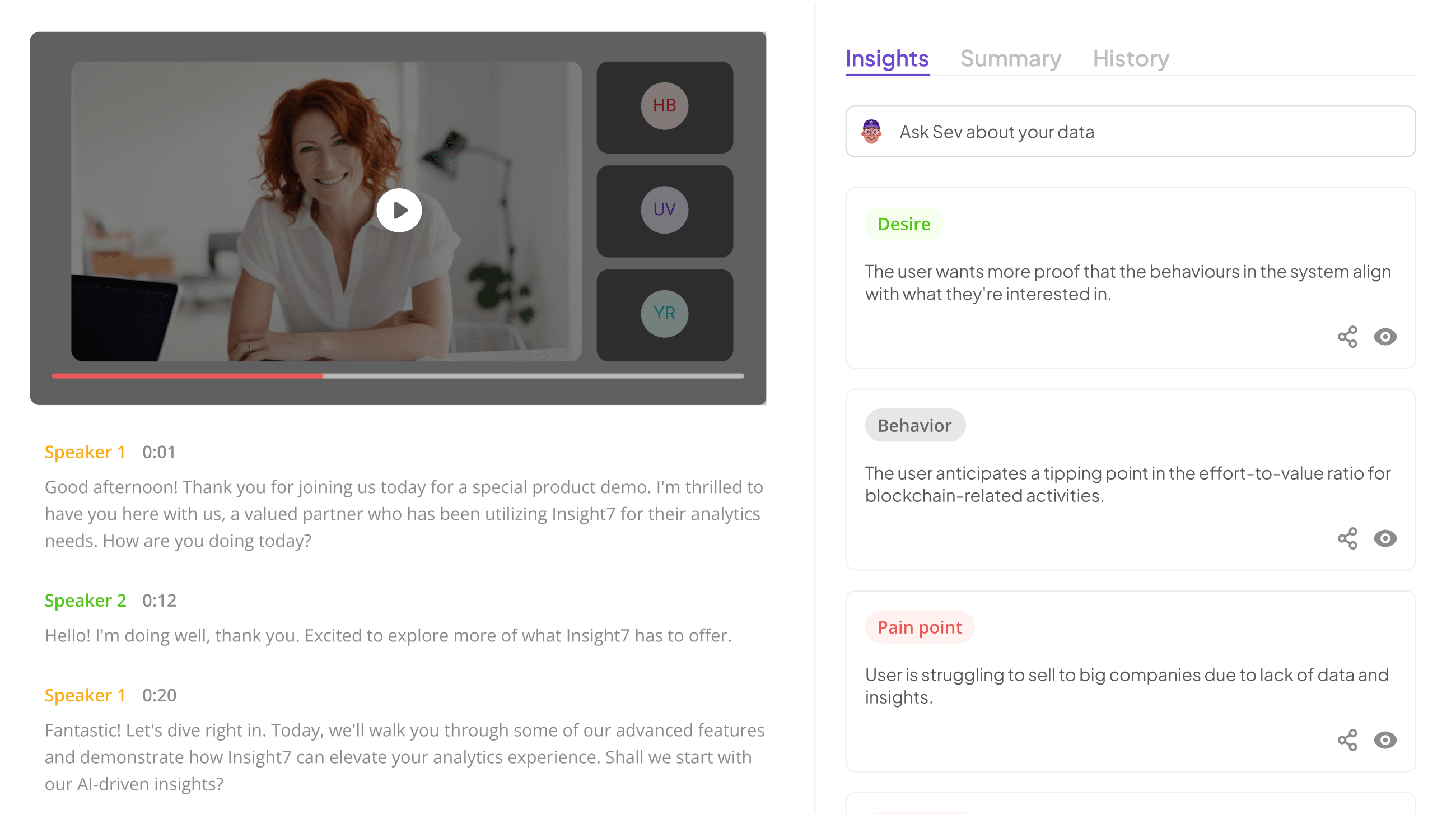
Understanding the Basics of AI-Powered Pattern Detection
AI-Powered Pattern Detection begins with a foundational understanding of how artificial intelligence processes qualitative research data. This technology analyzes large volumes of unstructured data, such as interview transcripts or open-ended survey responses, to discover underlying patterns and themes. By leveraging algorithms, AI tools can identify correlations and trends that might not be easily visible through manual analysis. Ultimately, this approach enhances the reliability and depth of research insights.
Key components of AI-Powered Pattern Detection include natural language processing (NLP) and machine learning. NLP enables the AI to grasp the context, sentiment, and nuances of language, resulting in more accurate interpretations of qualitative data. Machine learning further enhances pattern detection by allowing AI systems to learn from historical data and improve over time. Together, these technologies enable researchers to obtain actionable insights more efficiently, thereby increasing the overall value of qualitative research efforts.
The Role of AI Tools in Qualitative Research
AI-powered pattern detection offers innovative solutions for qualitative research, transforming how researchers analyze data. Traditionally, qualitative insights came from time-consuming manual analyses often resulting in inconsistencies. By utilizing AI tools, researchers can now quickly identify and synthesize themes, improving accuracy and speed in delivering insights.
The integration of AI enables a more systematic approach to data evaluation. First, these tools can process large volumes of qualitative data rapidly, allowing researchers to focus on interpreting findings rather than getting bogged down by analysis. Second, AI algorithms highlight patterns that may go unnoticed in manual reviews, thus enhancing the depth of insights. Finally, the reproducibility of AI-powered methods minimizes researcher bias and promotes consistency across teams, making it an essential resource in modern qualitative research strategies. Through AI-powered pattern detection, researchers can unlock deeper understanding and actionable insights from their qualitative data.
How Pattern Detection Works in Qualitative Data
AI-Powered Pattern Detection works by identifying recurring themes or trends within qualitative data, streamlining the research process significantly. This method relies on algorithms that analyze large volumes of text, such as interview transcripts and open-ended survey responses. First, the AI tool processes the data, extracting key phrases and categorizing them into themes. This accelerates the analysis phase, reducing human bias and increasing consistency across interpretations.
The effectiveness of AI-powered pattern detection stems from its capability to learn from the data it processes. It continuously improves its accuracy through feedback loops, adapting to the nuances of language and context. By employing such tools, researchers can efficiently uncover insights and generate reports much faster than traditional methods. Ultimately, this approach not only enhances the quality of analysis but also enables researchers to focus on strategic decision-making, unlocking deeper understanding within their qualitative findings.
Extract insights from interviews, calls, surveys and reviews for insights in minutes
Steps to Implementing AI-Powered Pattern Detection
Implementing AI-Powered Pattern Detection in qualitative research begins with the preparation of your data. First, ensure your data is clean and organized, which facilitates effective analysis. This step is crucial, as high-quality input data leads to more accurate insights. Next, select the appropriate AI tools that align with your research goals. Various tools offer different features, so choose one that best fits the nature of your qualitative data.
Once your data is prepared and the right tools selected, proceed to interpret the results. Analyzing AI-generated patterns involves refining your understanding of these insights, ensuring they align with your research questions. By following these steps, researchers can effectively utilize AI-Powered Pattern Detection, uncovering nuanced patterns that traditional methods might overlook. Adopting this technology not only enhances data analysis but also provides deeper insights into qualitative research, ultimately contributing to more informed decision-making.
Step 1: Preparing Your Data for Analysis
Before diving into AI-Powered Pattern Detection, itโs crucial to prepare your data for analysis effectively. Begin by gathering all relevant materials, such as transcripts, audio recordings, or interview notes. Organizing this data allows you to access key insights more easily. Also, consider using folders to categorize your data based on themes or research questions, making it simpler to analyze later.
Next, ensure your data is clean and formatted correctly. Remove any unnecessary details, such as background noise in audio records or irrelevant comments in transcripts. This step is vital, as clean data will lead to more accurate findings. By thoughtfully preparing your data, you are laying the groundwork for effective pattern detection through AI tools. Proper data preparation not only streamlines your analysis but enhances the overall quality of insights you gain from your qualitative research.
Step 2: Choosing the Right AI Tools for Pattern Detection
Choosing the right AI tools for pattern detection is essential for maximizing the effectiveness of your qualitative research. Begin by exploring the specific features that various AI-powered pattern detection tools offer. Look for capabilities such as advanced filtering options, natural language processing, and customizable reporting. These functionalities can greatly enhance your ability to capture nuanced insights from your data.
Next, consider the user experience of these tools. A user-friendly interface can streamline the research process, making it easier to navigate and analyze qualitative data. Tools that offer robust support and training resources can also prove invaluable, helping you harness the full potential of the software. Moreover, ensure that your chosen tools integrate seamlessly with existing data management systems. This integration enhances efficiency and promotes a smooth workflow, ultimately leading to richer insights from your research data.
Step 3: Interpreting Results and Refining Patterns
Interpreting results in qualitative research is foundational for drawing meaningful conclusions. AI-Powered Pattern Detection plays a central role during this phase, enabling researchers to sift through large volumes of data. The process begins by examining the themes or codes that have emerged, allowing for deeper insights to be gleaned. For instance, analyzing sentiment around specific themes can reveal participant attitudes effectively, giving context to quantitative metrics.
๐ฌ Ask About This Article
Have questions? Get instant answers about this article.
Once you have identified patterns, refining them is essential. This can be achieved by clustering insights into specific goals like improving user experience or highlighting risks. Utilizing AI tools to visualize these clusters aids in recognizing gaps and avenues for further exploration. By returning to the original data, researchers can trace the reasoning behind each theme, ensuring that the conclusions drawn are evidence-based. This iterative process not only saves time but also enhances the validity of the findings, allowing for impactful decision-making.
Top AI Tools for Pattern Detection in Qualitative Research
In qualitative research, harnessing the potential of AI-powered pattern detection is essential for efficient data analysis. Several tools are designed to streamline this process, allowing researchers to uncover meaningful insights from complex datasets. Noteworthy among them are NVivo, ATLAS.ti, MAXQDA, Dedoose, and others, which offer unique features that cater to different research needs.
NVivo is renowned for its powerful text analysis capabilities, making it ideal for managing large volumes of qualitative data. ATLAS.ti excels in visualizing connections through networks, which aids in identifying themes. MAXQDA combines qualitative and quantitative analysis, allowing researchers to integrate various data types effectively. Dedoose is particularly beneficial for mixed-method studies, offering an intuitive interface for collaboration. By integrating these AI tools into research workflows, teams can enhance their efficiency and achieve more reliable insights, ultimately delivering quicker results to clients and stakeholders.
insight7
AI-Powered Pattern Detection offers groundbreaking tools to extract meaningful insights from qualitative research data. To maximize its potential, researchers must first prepare their data, ensuring it is clean and organized for analysis. This foundational step enhances the accuracy of pattern detection. Next, selecting the appropriate AI tools tailored to specific research goals is crucial. By choosing tools that fit the qualitative context, researchers can uncover nuanced themes that might otherwise remain hidden.
After obtaining results, interpreting them accurately is key. Patterns identified should be examined in detail, allowing for deeper understanding and refinement of insights. Additionally, discussing the findings with stakeholders can foster collaborative interpretations and informed decision-making. Overall, AI-Powered Pattern Detection revolutionizes how we approach qualitative data, transforming raw information into actionable strategies. This innovative process bridges the gap between data collection and real-world application, making qualitative research more dynamic than ever.
NVivo
NVivo serves as a vital tool for qualitative researchers aiming to apply AI-powered pattern detection techniques effectively. At its core, NVivo helps in organizing, analyzing, and visualizing qualitative data, making it easier to identify emerging themes and connections. By using sophisticated algorithms, it enables researchers to process large datasets quickly, revealing insights that may not be immediately apparent through manual analysis.
As you engage with NVivo, consider how its features, such as coding and text search capabilities, facilitate a deeper understanding of your data. Users can benefit from visualizations that illustrate relationships and trends, enhancing the storytelling aspect of research findings. The combination of human intuition and AI efficiencies positions NVivo as an essential asset in modern qualitative research, promoting a collaborative approach between researchers and technology. By harnessing these tools, researchers can elevate their analysis to uncover nuanced patterns within their qualitative data.
ATLAS.ti
In the evolving landscape of qualitative research, ATLAS.ti provides a robust platform for AI-powered pattern detection. This tool allows researchers to systematically analyze complex data sets, making sense of varied interviews, open-ended survey responses, and focus group discussions. By employing advanced algorithms, it identifies recurring themes and concepts that may not be immediately apparent, helping researchers draw insightful conclusions from dense qualitative data.
Utilizing ATLAS.ti empowers researchers to visualize relationships between different data categories, thus enhancing the depth of analysis. The software allows users to code data effectively, facilitating an organized approach to pattern detection. With features like network views and data visualization tools, researchers can illustrate patterns and connections dynamically. Overall, ATLAS.ti streamlines the qualitative analysis process, ensuring that the journey from raw data to meaningful insights is not only efficient but also insightful. Through a systematic approach, it supports researchers in navigating the complexities of qualitative research data with ease and confidence.
MAXQDA
MAXQDA is a powerful tool designed to facilitate qualitative research through AI-powered pattern detection. It assists researchers in efficiently organizing and analyzing qualitative data, such as interviews and open-ended survey responses. With an intuitive interface, MAXQDA enables users to code data segments, helping to identify recurring themes and insights quickly. This ease of use is crucial for researchers managing large datasets, as it streamlines the often tedious process of manual coding.
By integrating advanced AI capabilities, MAXQDA enhances traditional qualitative research methods. AI algorithms can automate initial data readings, significantly reducing the time spent on preliminary analysis. In turn, this allows researchers to focus on more profound interpretations and connections within their data. The balance between human insight and AI efficiency makes MAXQDA an invaluable asset for those wanting to uncover patterns and actionable insights in qualitative research.
Dedoose
Dedoose is an effective platform that aids in analyzing qualitative research data through AI-powered pattern detection. Researchers can upload data from various sources, including interviews and surveys, creating projects that facilitate collaborative analysis. The application's ability to transcribe and analyze text simplifies the research process, allowing teams to focus on extracting meaningful insights rather than getting bogged down in manual data handling.
With Dedoose, users can discover themes, generate visualizations, and create reports from their data. Its native transcription service supports over 60 languages, boasting impressive accuracy, making it an essential tool for global research initiatives. Additionally, the platform prioritizes data privacy, complying with regulations such as GDPR. As researchers seek to navigate complex data, Dedoose provides a streamlined method for harnessing qualitative insights, ultimately improving the effectiveness of AI-powered pattern detection methodologies.
Conclusion: Maximizing Insights with AI-Powered Pattern Detection
In conclusion, harnessing AI-Powered Pattern Detection can significantly enhance your understanding of qualitative research data. By effectively identifying and analyzing patterns, researchers can unveil insights that might otherwise remain hidden. The ability to filter data by specific themes or keywords adds a layer of depth, providing clarity and coherence to complex information.
Furthermore, the rapid reporting capabilities of AI tools allow for swift dissemination of findings. This means that actionable insights can be integrated into decision-making processes more efficiently. By embracing AI-Powered Pattern Detection, professionals can elevate their research quality and drive meaningful outcomes in their respective fields.
๐ฌ Ask About This Article
Have questions? Get instant answers about this article.