Integrated Data Synthesis is a pivotal process in qualitative reporting workflows, where diverse data sources converge to create a cohesive narrative. As researchers confront the intricacies of multi-source data, understanding how to effectively synthesize information becomes essential. This practice not only enhances the reliability of insights but also provides a framework for triangulating data, ensuring a richer understanding of complex subjects.
Incorporating Integrated Data Synthesis into qualitative reporting empowers researchers to present well-rounded findings. By carefully merging and analyzing varying data streams, practitioners can address specific themes and generate impactful reports. As we delve deeper into this methodology, we will explore effective strategies, common challenges, and tools that elevate the synthesis of multi-source data, driving better decision-making and insightful outcomes.
Analyze qualitative data. At Scale.
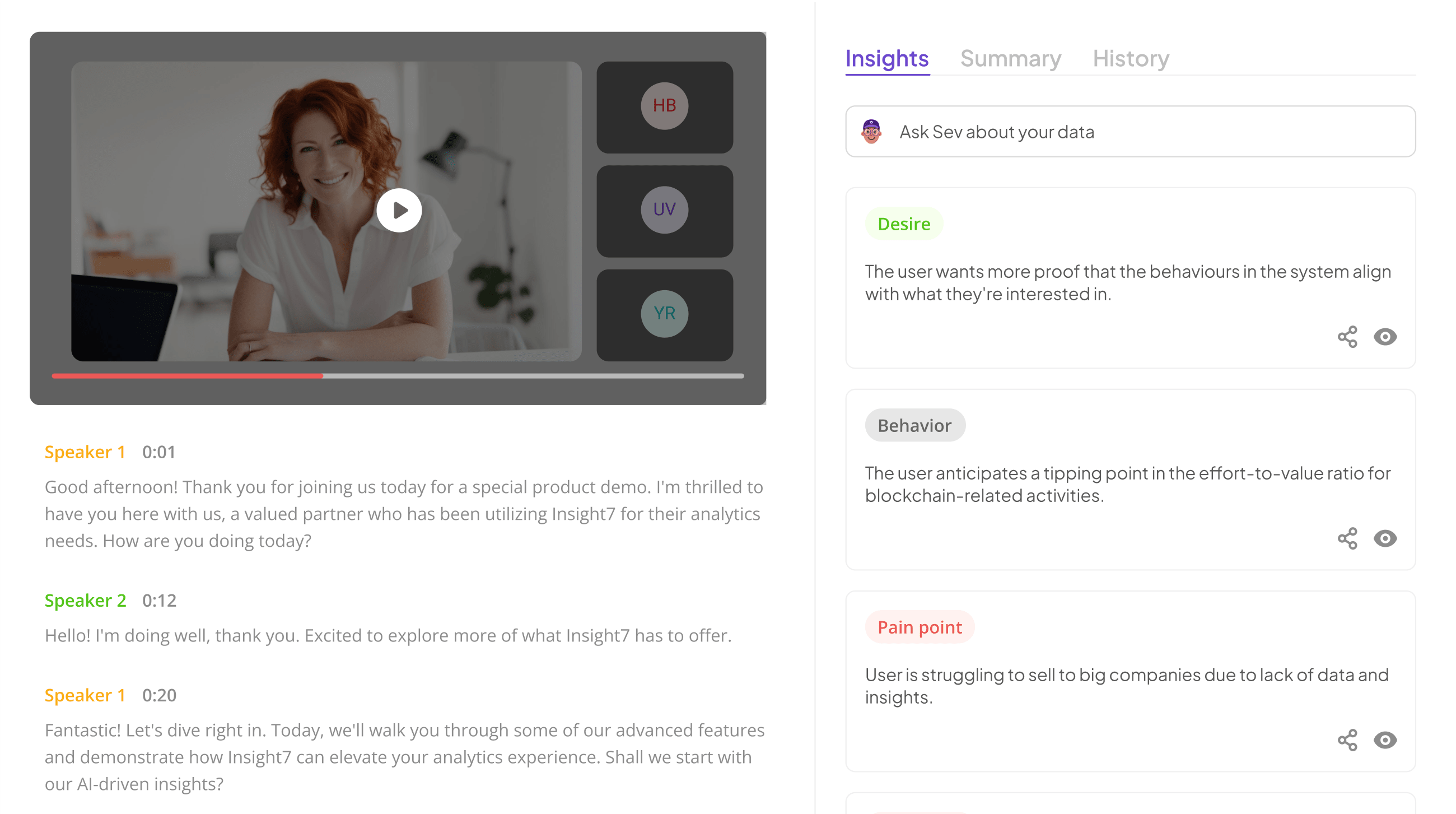
Foundations of Integrated Data Synthesis
Integrated Data Synthesis forms the backbone of effectively managing and interpreting qualitative data from various sources. This approach enables researchers to aggregate diverse data points, creating a holistic understanding of complex phenomena. However, the journey toward successful synthesis begins with recognizing the various sources of data, which may range from interviews to surveys and observations. Understanding these data types lays the groundwork for meaningful analysis.
Next, overcoming common challenges in multi-source integration is crucial. These include discrepancies in data formats, contextual relevance, and varying quality levels across sources. To tackle these issues, one must adopt strategies for data harmonization, ensuring compatibility and enhancing overall validity. Once integrated, synthesized data yields comprehensive insights, fortifying qualitative reports with robust evidence and fostering informed decision-making. Thus, mastering Integrated Data Synthesis is key to unlocking the full potential of multi-source qualitative data.
Understanding Multi-Source Data Integration
Multi-source data integration involves combining information from various origins to cultivate a comprehensive understanding of a subject. This method allows researchers to draw insights from diverse types of data, such as interviews, surveys, and observational notes. However, synthesizing data from different sources presents challenges. These may include discrepancies in data formats, varying quality levels, and differing contexts, making it hard to weave together a holistic picture.
To effectively achieve integrated data synthesis, it's essential to establish clear protocols for data compatibility and standardization. By addressing these challenges, researchers can enhance the validity of their findings. Moreover, integrating multi-source data allows for a richer analysis and deeper insights, ultimately leading to more informed qualitative reporting workflows. Adopting structured approaches in integrating data aids not only in clarity but also bolsters the reliability of conclusions drawn from comprehensive analyses.
- Explanation of multi-source data
Integrating multi-source data is crucial for effective qualitative analysis. Multi-source data refers to the collection of information from various origins, such as interviews, surveys, and existing documents. Each source contributes unique perspectives, enriching the understanding of the research topic. When combined, these data sources provide a more comprehensive view, allowing researchers to identify patterns and themes that may not emerge from a single data point alone.
However, organizing and analyzing multi-source data can present challenges. Data compatibility is often an issue, as varying formats and contexts can complicate integration. Additionally, ensuring the reliability of insights is paramount. To achieve successful Integrated Data Synthesis, researchers must carefully curate their sources, ensuring that each piece complements the others. This groundwork lays the foundation for richer qualitative reporting workflows, ultimately enhancing the quality and depth of the insights generated from the analysis.
- Common challenges in combining data from diverse sources
Combining data from diverse sources presents a range of challenges that can complicate Integrated Data Synthesis. One significant hurdle is varying data formats, which can lead to inconsistencies in analysis. When data originates from different platforms or methodologies, harmonizing it becomes essential for reliability. In addition, discrepancies in data quality can impact the overall validity of insights drawn from the synthesis. Diverse perspectives must be reconciled, requiring careful consideration of biases and context to present a coherent narrative.
Another challenge lies in establishing a common framework for analysis. Different sources may employ unique coding schemes or terminologies, complicating the synthesis process. This necessitates a clear strategy to unify the data under consistent categories. Moreover, managing the volume of data can be overwhelming, often leading to difficulties in retaining focus on key insights. By acknowledging these challenges, organizations can enhance their workflows and ensure a more integrated approach to data synthesis and reporting.
Benefits of Integrated Data Synthesis for Reporting
Integrated Data Synthesis offers numerous advantages for reporting by enhancing the overall reliability and validity of findings. By incorporating insights from multiple sources, this approach fosters a more nuanced understanding of complex issues. The combination allows for triangulation of data, which strengthens the conclusions drawn and minimizes biases inherent in single-source studies.
Moreover, Integrated Data Synthesis promotes comprehensive insights that can inform decision-making processes effectively. A well-structured synthesis brings clarity to diverse themes and facilitates the identification of patterns and trends across multiple datasets. This method allows stakeholders to pinpoint specific issues, leading to targeted recommendations. Ultimately, Integrated Data Synthesis not only streamlines reporting workflows but also enriches the quality of insights generated, providing a robust foundation for informed strategies and actions.
- Enhanced data reliability and validity
Data reliability and validity are critical components when organizing and analyzing multi-source data for qualitative workflows. Enhanced data reliability ensures that findings consistently reflect the underlying phenomena being studied. It allows researchers to trust their insights and facilitates informed decision-making based on sound evidence.
To achieve enhanced data reliability and validity, it is essential to implement several key strategies. First, triangulation involves cross-checking data from various sources to confirm findings, reducing the impact of bias. Second, maintaining rigorous data collection and analysis standards can prevent discrepancies that undermine validity. Finally, fostering a collaborative analysis process encourages diverse perspectives, which strengthens the integrity of the insights generated. By focusing on integrated data synthesis, researchers can produce richer and more reliable qualitative reports, ultimately leading to more informed conclusions and actionable strategies.
- Comprehensive insights through data triangulation
Data triangulation involves combining different data sources to create a fuller, more nuanced understanding of your research topic. This approach enhances the integrity and depth of insights derived from qualitative reporting workflows. By integrating multiple perspectives, researchers can effectively cross-verify findings, leading to more robust conclusions that stand up to scrutiny.
The process benefits significantly from integrated data synthesis. It not only aids in identifying patterns or discrepancies across various sources but also fosters a comprehensive view of the subject matter. For instance, data drawn from interviews can be complemented with survey results and contextual information, allowing for richer interpretations. Ultimately, this multi-faceted perspective empowers researchers to draw actionable insights, making the analysis more relevant and useful to stakeholders.
Steps in Organizing and Analyzing Multi-Source Data
Organizing and analyzing multi-source data begins with a structured approach to ensure comprehensive insights. The first step is collecting and preparing data from various sources, which involves identifying relevant datasets and verifying their quality. This foundational work sets the stage for effective analysis, as clean, compatible data is crucial for drawing meaningful conclusions.
The next step focuses on integrating the data sources for synthesis. Techniques such as thematic analysis and data merging ensure coherence in the insights derived from distinct datasets. Utilizing specific tools designed for effective integration can streamline this process, allowing researchers to visualize data and uncover patterns more easily. Integrated data synthesis not only enhances reporting workflows but also enriches understanding by providing a holistic view of the data landscape. This structured outline helps guide analysts in transforming multi-source data into actionable insights, ultimately leading to better-informed decisions and strategies.
Extract insights from interviews, calls, surveys and reviews for insights in minutes
Step 1: Collecting and Preparing Data
In the process of integrated data synthesis, the first crucial step involves collecting and preparing data effectively. Begin by identifying relevant data sources, which may include interviews, survey results, and existing literature. It is essential to verify the compatibility of these diverse data types to ensure seamless integration later in the analysis. Quality is paramount; thus, do thorough checks for consistency, accuracy, and relevance.
Furthermore, organizing your data helps in avoiding potential pitfalls and enhances the reliability of the insights derived. After gathering your data, it's important to categorize and format it properly. This preparation phase lays a strong foundation for synthesis, guiding you to draw meaningful insights from the multi-source data effectively. Ultimately, this meticulous approach to data collection and preparation will facilitate a smoother workflow in qualitative reporting narratives.
- Identify relevant data sources
Identifying relevant data sources is a critical first step in the process of integrated data synthesis. Begin by considering various types of qualitative data, such as interviews, surveys, and focus group discussions. Each source provides unique insights that enhance the depth and breadth of your analysis. Consider not only the data itself but also the context, including the audience and objectives of your research. This ensures that the data you select aligns with your reporting goals.
Next, evaluate the quality and compatibility of these data sources. High-quality data is crucial for reliable insights; therefore, investigating the credibility of each source is essential. Additionally, assess how well these sources can be integrated. This involves recognizing patterns, themes, and discrepancies across sources, which can be pivotal in your reporting workflows. By carefully choosing and validating your data sources, you can effectively lay the groundwork for successful integrated data synthesis, ultimately leading to actionable insights.
- Ensure data compatibility and quality
Data compatibility and quality are crucial for an effective integrated data synthesis process. When combining multiple sources, it’s essential first to ensure that the data types and formats align. This prevents discrepancies that can skew analysis and insights. Start by standardizing data formats across your sources, which helps facilitate a smoother integration process. Additionally, establish quality benchmarks to assess the accuracy and reliability of the data, which directly affects the output of your analysis.
To ensure ongoing compatibility, engage in consistent audits of your data sources and integration processes. Regularly check for updates, changes, or inconsistencies that could emerge from external sources. This proactive approach helps maintain data integrity over time and allows for more informed decision-making. In summary, prioritizing data compatibility and quality not only enhances the clarity of your insights but also strengthens the overall reliability of your qualitative reporting workflows.
Step 2: Integrating Data Sources for Synthesis
Integrating various data sources is a crucial step in achieving integrated data synthesis for qualitative reporting workflows. When approaching this integration, it is essential to identify the techniques that allow for effective merging of data. One can start by combining transcripts, recordings, and other data assets into a unified structure. This step ensures that diverse data types complement each other, fostering richer insights leading to more robust findings.
Using tools designed for data integration can significantly streamline this process. For instance, specialized software can facilitate the organization and visualization of combined data sets, helping to identify patterns and themes more efficiently. Furthermore, applying techniques like thematic analysis can distill information into manageable insights, guiding the overall synthesis. When effectively integrated, the data not only reveals deeper narratives but also enhances the overall quality and validity of the qualitative analysis, empowering informed decision-making and insightful reporting.
- Techniques for data merging and synthesis
Data merging and synthesis require strategic techniques to effectively integrate diverse datasets. A primary method involves thematic analysis, where key themes are identified across distinct data sources. This process allows researchers to extract valuable insights, facilitating a comprehensive understanding of trends and patterns. When merging data, ensure that data formats are compatible and that any discrepancies are addressed early on to maintain integrity.
Utilizing software tools can further streamline this integration. Popular platforms offer functions for coding data, which help categorize and visualize information. This makes it easier to synthesize findings from various sources into cohesive reports. Lastly, employing triangulation enhances the reliability of insights drawn from the data by corroborating findings from multiple sources. By systematically applying these techniques, researchers can achieve integrated data synthesis that supports nuanced qualitative reporting workflows.
- Tools for effective data integration
Effective data integration is crucial in organizing and analyzing multi-source data for qualitative reporting workflows. To achieve integrated data synthesis, utilizing the right tools is essential, as they help streamline processes and improve accuracy. Key platforms like NVivo, Dedoose, and MAXQDA are popular choices in the field. Each tool offers unique features tailored to different data integration needs, making it vital to evaluate which aligns best with your project requirements.
NVivo, for example, excels at managing large datasets and facilitating in-depth qualitative analysis. Dedoose is notable for its user-friendly interface, ideal for teams that prioritize collaboration. On the other hand, MAXQDA supports both qualitative and quantitative data, allowing for diverse analytical approaches. Selecting the right tool ultimately enhances the efficiency of integrated data synthesis, helps minimize biases, and maximizes insightful outcomes in qualitative research.
Tools for Effective Integrated Data Synthesis
Effective integrated data synthesis relies on the right tools and methodologies to harmonize insights from diverse sources. Various platforms can assist researchers in transforming fragmented data into coherent narratives, thereby elevating the quality of qualitative reporting. These tools facilitate the organization, analysis, and visualization of information, enabling researchers to identify patterns, themes, and trends quickly.
Key tools for integrated data synthesis include platforms such as NVivo and Dedoose, which offer features like thematic coding and sentiment analysis. MAXQDA is another robust option, especially for handling large datasets, while Quirkos excels in its user-friendly interface, making it accessible for those new to data analysis. Selecting the appropriate tool not only streamlines the workflow but also enhances the overall integrity and depth of the analysis. By utilizing these tools thoughtfully, researchers can significantly improve their ability to synthesize integrated data effectively, leading to actionable and insightful conclusions.
Key Tools and Platforms
In today's data-driven environment, employing the right tools and platforms is crucial for effective integrated data synthesis. A range of powerful applications can streamline the process of organizing and analyzing multi-source data for qualitative reporting workflows. These tools provide the necessary functionalities to address common challenges like data compatibility and integration complexities.
Key tools include NVivo, known for its robust qualitative data analysis features, and Dedoose, which excels in mixed-methods research. MAXQDA offers versatile capabilities for document analysis, while Quirkos is celebrated for its intuitive interface. Each of these platforms can facilitate the synthesis of insights, ultimately allowing teams to generate comprehensive reports that reflect various data points. By leveraging these key tools, organizations can transform disparate information into actionable insights, significantly enhancing the quality and reliability of their qualitative reporting efforts.
- insight7: Overview and advantages
insight7: Overview and Advantages
insight7 is a powerful tool designed to facilitate integrated data synthesis by seamlessly organizing multi-source data. This platform allows users to import various data types, such as transcripts and video files, enabling a comprehensive analysis. By centralizing data in one location, researchers can easily access insights across multiple projects. The user-friendly interface encourages efficiency, minimizing the learning curve associated with more complex software.
One of the primary advantages of using insight7 is its collaborative features. Teams can share resources, ensuring that all members contribute to the qualitative reporting process. Furthermore, the platform offers predefined analysis kits tailored to specific research needs, making it easier to extract meaningful insights from vast data sets. This combination of intuitive design and collaborative functionality enhances the entire qualitative research experience, helping users achieve precise and actionable results.
- NVivo: Features and use cases
NVivo serves as a powerful tool for organizing and analyzing multi-source data in qualitative research. It offers various features designed to streamline the coding process and visualize complex information, helping researchers uncover actionable insights. Users can easily import data from various sources, allowing for seamless integration and comprehensive analysis tailored to specific research needs.
Among its unique capabilities, NVivo allows for collaborative projects, enabling multiple users to work together while maintaining data integrity. It supports diverse media types, ensuring that users can capture a full spectrum of qualitative data—from interviews to focus group discussions. By utilizing coding frameworks and queries within NVivo, researchers can implement integrated data synthesis strategies, resulting in deeper understandings and richer narratives. Thus, NVivo plays a crucial role in contemporary qualitative reporting workflows, enhancing the reliability of synthesized data findings.
- Dedoose: Comparative analysis
In the realm of qualitative reporting, Dedoose serves as a powerful tool for comparative analysis, making integrated data synthesis more accessible. This platform facilitates the organization of multi-source data, allowing researchers to examine various insights systematically. Through its features, users can efficiently tag and code data, which simplifies the process of finding thematic connections among different data sources.
By employing Dedoose, analysts can categorize insights based on predefined themes, enhancing clarity. Each theme pulls relevant data, streamlining the journey from raw information to insightful conclusions. This process not only supports the identification of trends but also fosters a deeper understanding of complex relationships within the data. Ultimately, utilizing Dedoose empowers researchers to generate comprehensive, data-driven narratives that bolster the integrity of qualitative reporting workflows.
- MAXQDA: Strengths and limitations
MAXQDA presents a mixed bag of strengths and limitations when it comes to integrated data synthesis in qualitative reporting workflows. One of its primary strengths lies in its intuitive interface, enabling users to organize and analyze various data types, such as interviews and survey results. This versatility facilitates seamless integration of data, which enhances the overall quality of analysis. Additionally, MAXQDA offers comprehensive coding capabilities that help identify patterns across diverse data sources, ultimately leading to richer insights.
However, there are notable limitations to consider. The software may not fully accommodate users who require automated data processing features for large datasets. Users often find themselves spending excessive time on preliminary coding, which can detract from deeper analysis, especially in high-volume scenarios. Moreover, while MAXQDA excels in qualitative data management, it may require supplemental tools for comprehensive quantitative analysis. By understanding these strengths and limitations, users can better determine if MAXQDA aligns with their specific qualitative reporting needs.
- Quirkos: User-friendly aspects
The user-friendly aspects of an effective data integration tool significantly enhance the experience of qualitative researchers. Central to its appeal is the intuitive interface that allows users to navigate seamlessly through various functions. Simplifying complex tasks is paramount, as researchers often juggle multiple data sources during analysis. User-centric design empowers researchers by reducing cognitive load, making it easier to focus on extracting meaningful insights from diverse datasets.
In this context, clarity and simplicity are essential for fostering engagement. Features like drag-and-drop functionality facilitate efficient organization, while visual data representation aids in comprehension. Moreover, the tool’s easy onboarding process minimizes the time investment required for training. By prioritizing user experience and accessibility, researchers can synthesize their data effectively, ensuring a more robust foundation for insightful qualitative reporting. Ultimately, integrating user-friendly elements contributes to the overall efficiency of qualitative workflows, transforming data synthesis into an intuitive process that meets the demands of modern research.
Conclusion: Achieving Excellence in Integrated Data Synthesis
Achieving excellence in integrated data synthesis is essential for effective qualitative reporting. By synthesizing data from multiple sources, researchers can uncover deeper insights and enhance the reliability of their findings. This process involves not only gathering relevant information but also ensuring coherence and alignment between different data sets, which can ultimately lead to more nuanced conclusions.
To reach this level of excellence, it is crucial to utilize appropriate tools and techniques that facilitate seamless data integration. Additionally, fostering a clear understanding of the themes and insights allows for a more targeted analysis. By prioritizing these elements, organizations can significantly improve their reporting workflows and deliver data-driven insights that support informed decision-making.