AI-Driven Coding for interview data offers a transformative approach to qualitative research, enabling researchers to uncover insights more efficiently. Traditional methods of manual coding can be arduous, leading to inconsistencies that hinder the quality of results. By integrating AI tools, teams can streamline their analysis, ensuring that insights are derived swiftly and objectively, regardless of the number of researchers involved.
With AI technologies, the process of descriptive coding becomes not only quicker but also more systematic. Researchers can harness advanced algorithms to analyze large datasets, reducing time spent on repetitive tasks while enhancing the accuracy of findings. As the demand for timely insights grows, embracing AI-Driven Coding opens up new avenues for delivering high-quality research outputs without compromising on rigor.
Analyze qualitative data. At Scale.
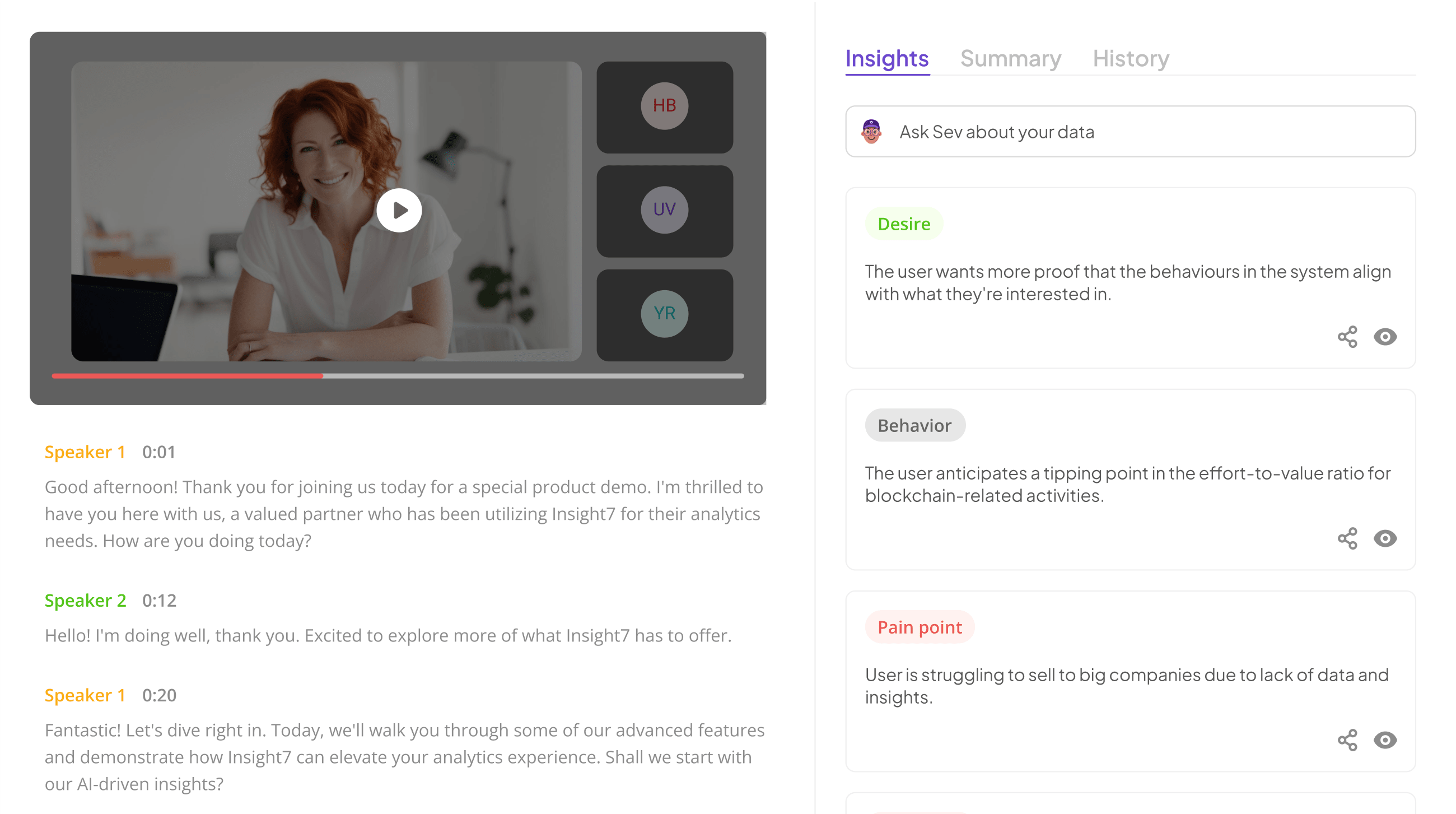
Understanding the Basics of AI-Driven Coding
AI-Driven Coding begins with understanding its core principles and applications. At its essence, this approach utilizes artificial intelligence to streamline and enhance the coding process in qualitative research, particularly when analyzing interview data. By automating the coding of responses, researchers can save valuable time and focus more on interpreting the results rather than laboriously organizing data manually.
This method relies heavily on algorithms that can identify patterns, themes, and insights from vast amounts of qualitative data. When effectively implemented, AI-Driven Coding not only increases efficiency but also improves the accuracy and reliability of the insights generated. As you dive deeper into this approach, you'll encounter various effective AI tools designed for coding, each offering unique features to meet your research needs. Understanding these tools can empower you to leverage AI capabilities for transforming qualitative analyses into actionable insights.
What is AI-Driven Coding?
AI-Driven Coding is the intersection of artificial intelligence and qualitative research, specifically in coding interview data. This innovative approach utilizes algorithms to identify patterns and themes in textual information, making sense of complex qualitative data. By automating portions of the coding process, researchers can save time and reduce human error, leading to more reliable insights.
The process begins with the collection of interview data, which AI then analyzes to identify key themes. Users can customize the AI model to align with specific research goals, enhancing the relevance of the findings. In this way, AI-Driven Coding not only streamlines the analysis but also empowers researchers to focus more on interpreting the insights rather than getting bogged down by manual coding tasks. Overall, this cutting-edge methodology transforms how researchers approach qualitative data, promoting efficiency and depth of understanding.
Benefits of Using AI for Descriptive Coding in Qualitative Research
AI-Driven Coding offers numerous advantages for qualitative research, particularly in the realm of descriptive coding. One primary benefit is the speed at which data can be processed. Manual coding can be labor-intensive and time-consuming, causing delays in delivering insights. AI-driven solutions can analyze large volumes of interview data almost instantaneously, ensuring quicker reporting and faster decision-making.
Another significant advantage is the reduction of human bias and inconsistency. Different researchers might interpret the same data in varied ways, leading to discrepancies in findings. By employing AI for descriptive coding, researchers can achieve a more standardized approach that minimizes these inconsistencies. This not only enhances the reliability of the insights but also instills greater confidence in the conclusions drawn from the research. Overall, the integration of AI in the coding process streamlines workflows, resulting in more efficient and accurate qualitative research outcomes.
Extract insights from interviews, calls, surveys and reviews for insights in minutes
Implementing AI-Driven Coding: A Step-by-Step Guide
To implement AI-Driven Coding for analyzing interview data, start by preparing your dataset thoroughly. This includes organizing your transcripts or recordings and ensuring all necessary data is clean and accessible. A well-structured dataset will enhance the AI's ability to identify patterns and generate useful codes effectively. Pay careful attention to clarity in transcripts, as any ambiguity can hinder the outcome.
Next, select the best AI tools suited for your coding needs. Platforms like NVivo and ATLAS.ti offer powerful features for qualitative analysis, while others like MonkeyLearn and MAXQDA provide unique advantages. Once you've chosen the right tool, focus on training your AI model for descriptive coding. This involves feeding it relevant data and adjusting parameters to refine its analysis capabilities. Lastly, validate the results by cross-checking the AI-generated codes with your own insights, allowing for better interpretation and ensuring that human judgment complements AI. Engaging in these systematic steps will help harness the full potential of AI in coding your interview data efficiently.
Step 1: Preparing Your Interview Data
To begin with AI-Driven Coding, preparing your interview data is a crucial first step. Collect all your interview transcripts and any related audio or video files in a centralized location. Organizing this data will make it easier to analyze later. Consider using consistent formatting for your transcripts to enhance clarity and readability. Each transcript should contain important details such as participant identifiers, dates, and key themes discussed. This initial groundwork lays the foundation for effective analysis.
Next, review your transcripts for accuracy. If necessary, transcribe audio files using reliable transcription software to ensure high fidelity to spoken words. This quality check is essential as it directly influences the coding process. Once your data is polished and organized, you can confidently move ahead to using AI tools for descriptive coding. This method not only streamlines analysis but also helps unearth valuable insights within your data, enhancing the depth of your research.
Step 2: Selecting the Right AI Tools for Coding
Choosing the right AI tools for coding is essential in streamlining your qualitative research process. These tools facilitate AI-driven coding, allowing researchers to efficiently manage and analyze interview data. Start by assessing your specific needs, such as the scale of your project or the complexity of the data. Consider tools like NVivo or ATLAS.ti that offer robust support for qualitative analysis.
Next, evaluate features like usability, integration capabilities, and support for multimedia data. You want a tool that not only automates coding but also enhances your ability to derive meaningful insights from the data. Additionally, check for compliance features that ensure data privacy and security, which are crucial in today's research environment. By aligning your selection process with these criteria, you can effectively harness AI-driven coding to turn your interview data into actionable insights.
insight7
To effectively utilize AI-Driven Coding, practitioners should first identify the characteristics of their interview data. Understanding the nuances of this data informs the AI model's training process. Prioritize the coding categories based on the key themes emerging from initial analyses. This foundational work guides the AI in generating relevant, meaningful codes.
Next, exploring suitable AI tools is crucial. Various platforms like NVivo and ATLAS.ti offer robust capabilities for descriptive coding, helping researchers automate the identification of patterns within qualitative data. Selecting the right tool enhances efficiency, enabling deeper insights from interview data. With careful preparation and the right technological support, your research can yield more actionable findings. Remember, the aim of AI-Driven Coding is to streamline the process from data collection to insight generation, ensuring that researchers can focus more on interpretation and strategy.
NVivo
NVivo provides an innovative platform for performing AI-driven coding on qualitative data, especially interview transcripts. Utilizing advanced algorithms, NVivo streamlines the coding process, helping researchers identify patterns and themes with greater efficiency. When working with AI, users can expect a tool that enhances their analytical capabilities rather than replacing their intuition and expertise.
One of the key features of NVivo is its ability to manage large volumes of data effectively. Users can import interview transcripts and let the AI system highlight potential themes, making it easier to assign relevant codes. The program's interactive visualization tools also allow researchers to explore connections between coded data, adding depth to their analysis. In summary, NVivo serves as an essential asset for those looking to harness AI-driven coding in their qualitative research endeavors, facilitating informed decision-making and rich insights.
ATLAS.ti
ATLAS.ti is a powerful software tool designed for qualitative data analysis, particularly effective for managing and coding interview data. This platform simplifies the process of AI-driven coding by allowing users to organize, code, and analyze large amounts of qualitative data efficiently. Users can input their interview transcripts, making it easier to apply descriptive coding and identify themes or patterns that emerge from the data.
The software features advanced coding tools, making it beneficial for researchers looking to gain actionable insights from qualitative research. By harnessing AI capabilities, ATLAS.ti helps streamline the analytic process, reducing the time required to sift through unstructured data. This allows researchers to focus on interpreting the results and drawing meaningful conclusions that can drive decision-making. Ultimately, using ATLAS.ti in conjunction with AI-driven coding can enhance the effectiveness of qualitative research efforts.
MonkeyLearn
In the realm of qualitative research, employing AI-driven coding tools can significantly streamline the analysis process. One such tool enhances your ability to extract insights from interview data effortlessly. The intuitive platform allows users to import transcripts directly and analyze them without requiring extensive training or prior knowledge. This simplicity enables researchers to focus on deriving meaningful themes and insights from their data rather than getting bogged down by the technical aspects of the analysis.
Users can cluster related interviews and utilize pre-designed kits tailored for specific types of analysis. This functionality enables quick, efficient data processing and offers insights that may otherwise be overlooked. Consequently, this tool not only enhances productivity but also ensures that critical insights are readily available for interpretation. By leveraging AI-driven coding, researchers can maximize the value of their qualitative research, resulting in richer, data-driven conclusions that inform their findings.
MAXQDA
MAXQDA is a powerful tool that supports the process of AI-driven coding for qualitative research. As researchers increasingly face the challenge of analyzing large volumes of interview data, this software provides an efficient way to categorize and interpret that data. By facilitating intuitive coding practices, MAXQDA allows researchers to focus on meaningful themes and insights without becoming overwhelmed by the sheer quantity of information.
When utilizing MAXQDA for descriptive coding, users benefit from a range of features. First, it offers user-friendly interfaces that help streamline the coding process. Second, it supports automatic coding, making it easier to organize and analyze unstructured data. Lastly, its integration with AI capabilities presents a significant advantage, allowing researchers to uncover deeper insights while saving time. Incorporating MAXQDA into your research workflow can enhance the quality and speed of your data analysis, making it an invaluable resource for those seeking to harness the power of AI-driven coding effectively.
Step 3: Training Your AI Model for Descriptive Coding
Once you have selected the appropriate AI tools, the next step is training your AI model for descriptive coding. Training the model involves feeding it with relevant data, specifically interview transcripts, to ensure it learns to identify and categorize themes effectively. This process is crucial because an accurately trained model can streamline your analysis and improve data insights significantly.
To train your model effectively, consider the following steps: First, curate a diverse and representative dataset to enhance the model's learning capability. Second, define specific coding schemes aligned with your research objectives. This helps in guiding the AI to recognize nuanced patterns within the qualitative data. Lastly, regularly evaluate the performance of your AI model. By iterating on your training data and tuning parameters, you can gradually refine the model's ability to offer valuable insights, ultimately fostering a robust AI-driven coding approach for your interview data analysis.
Step 4: Validating and Interpreting the Results
After employing AI-Driven Coding to analyze your interview data, the next crucial step involves validating and interpreting the results. This phase ensures that the insights gleaned from the data are both accurate and meaningful. Effective validation can include cross-referencing coded themes with the original transcripts to confirm their relevance and ensure they align with your research objectives. It's crucial to involve multiple reviewers in this process to reduce bias and enhance the robustness of the findings.
Interpreting results requires careful consideration of the context surrounding the data. Look for patterns that may indicate underlying sentiments or trends recognized through AI analysis. Documentation of notable quotes alongside themes can help establish credibility and provide context. By comprehensively validating your findings, you can ensure they are actionable and aligned with your research goals, ultimately enriching your qualitative insights.
Conclusion: Enhancing Qualitative Research with AI-Driven Coding
AI-driven coding offers a transformative approach to qualitative research, streamlining the analysis of interview data. This innovative technology not only reduces the time spent on manual coding but also minimizes inconsistencies and biases that can occur when multiple researchers interpret data differently. By employing AI-driven coding, researchers can efficiently uncover themes and insights that may otherwise remain hidden in extensive datasets.
Additionally, the integration of AI in the research process enhances the overall quality of findings and allows for quicker delivery of insights to clients. As qualitative research continues to evolve, leveraging AI-driven coding will become essential for professionals seeking to improve their methodologies. By embracing this technology, researchers can expect to gain deeper, more reliable understandings of their interview data, ultimately paving the way for informed decision-making.