How to Analyze Text Responses in Voice of Customer Programs
-
Bella Williams
- 10 min read
Text Response Analysis plays a vital role in understanding customer sentiments and improving Voice of Customer programs. By diving into textual feedback, businesses can uncover valuable insights that drive decision-making and enhance customer experiences. This approach helps to clarify customer expectations, pinpoint emerging trends, and identify areas needing attention.
However, analyzing text responses can be a daunting task due to its complexity and the potential for bias in interpretations. As teams may differ in their analysis methodologies, achieving consistency becomes challenging. Streamlining this process is essential for timely and accurate insights, ensuring that the voice of the customer is authentically represented in business strategies.
Analyze & Evaluate Calls. At Scale.
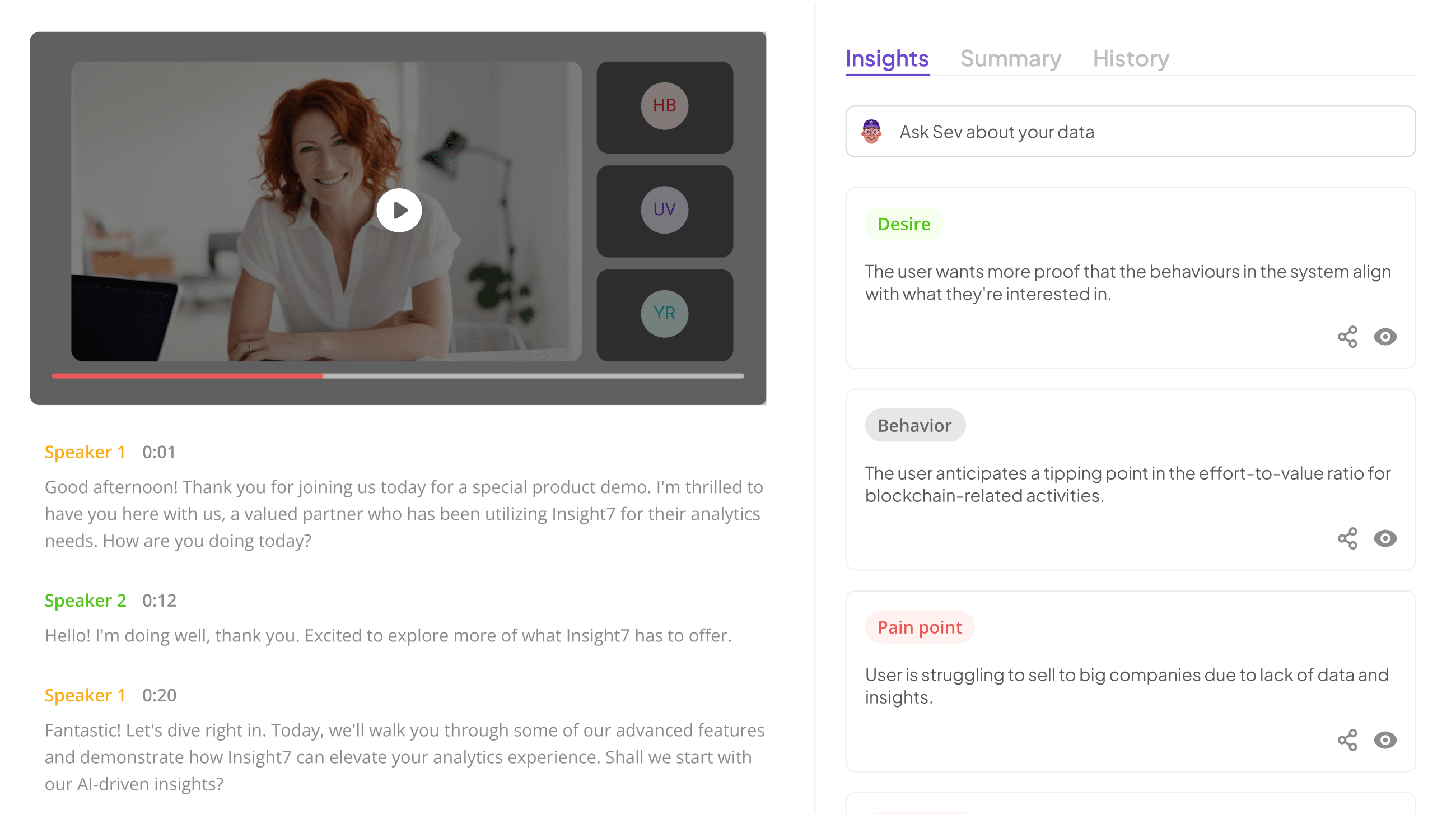
Understanding the Basics of Text Response Analysis
Text Response Analysis serves as a crucial tool for understanding customer feedback in Voice of Customer programs. To effectively analyze text responses, one must first grasp its fundamentals. This process involves interpreting qualitative data from various sources, such as surveys or social media comments. By understanding sentiment and identifying key themes, organizations can derive valuable insights into customer experiences and preferences.
The essence of Text Response Analysis lies in translating raw feedback into actionable strategies. Begin by collecting and organizing the text data, ensuring a comprehensive overview of customer sentiments. Next, employing techniques such as sentiment analysis helps in categorizing responses into positive, negative, or neutral sentiments. The final step is synthesizing these insights into clear recommendations for improving products or services. By mastering the basics of Text Response Analysis, organizations can enhance their decision-making processes and strengthen customer relationships.
The Role of Text Response Analysis in Customer Insight
Text response analysis plays a crucial role in extracting valuable insights from customer feedback. By carefully reviewing qualitative responses, businesses can unearth sentiments, opinions, and preferences that surveys and structured data might overlook. This analysis enables companies to personalize their services, improving customer satisfaction and loyalty over time.
To effectively harness the power of text response analysis, it is important to focus on several key aspects. First, analyzing trends and themes helps identify common customer concerns and desires. Second, comparing insights across different demographics can reveal distinct behavior patterns. Finally, integrating findings from text response analysis with broader market research enhances decision-making and strategy development. By adopting these approaches, organizations can turn unstructured customer feedback into actionable strategies that drive growth and enhance overall customer experience.
Key Challenges in Text Response Analysis
Text response analysis is essential in understanding customer sentiment and feedback, yet it presents some key challenges. One significant hurdle is the manual analysis of open-ended responses, which can be incredibly time-consuming. In many teams, differing perspectives among researchers can lead to inconsistent interpretations, ultimately affecting the quality of insights generated.
Additionally, the increasing demand for quicker results adds pressure on teams to deliver faster reports. Without effective systems in place, this can lead to rushed analysis, hurting the quality of derived insights. Many organizations struggle with bandwidth, relying on simple AI tools to streamline the process but often finding these solutions inadequate. Tackling these challenges effectively requires a thoughtful approach to text response analysis that emphasizes consistency, speed, and accuracy. Adopting better tools and methodologies can help mitigate these issues and enhance the quality of customer insights derived from text analysis.
Tools and Techniques for Text Response Analysis
To effectively analyze text responses, selecting the right tools and techniques is crucial. A variety of software platforms, such as insight7, MonkeyLearn, Lexalytics, Textblob, and NVivo, offer features tailored to streamline text response analysis. These tools can efficiently process qualitative data from interviews and surveys, transforming raw text into actionable insights. By automating certain tasks, they significantly reduce bias and accelerate the reporting process, which is essential for maintaining the quality of insights produced.
Implementing a structured approach enhances the effectiveness of text response analysis. First, collecting and aggregating text responses from various sources allows researchers to create a comprehensive dataset. Next, preprocessing the text data ensures that it is clean and ready for analysis. Finally, applying analytical techniques, whether through AI or traditional methods, will yield meaningful results that inform decision-making strategies. Each of these steps contributes to a robust analysis framework, ultimately leading to better customer insights and improved responsiveness in voice of customer programs.
Extract insights from interviews, calls, surveys and reviews for insights in minutes
Top Tools for Effective Text Response Analysis
When engaging in effective Text Response Analysis, utilizing the right tools is essential. Each tool offers unique capabilities to streamline the process and derive actionable insights. For instance, Insight7 is renowned for its user-friendly interface and comprehensive analytical functions, making it ideal for qualitative projects. MonkeyLearn excels in automating text classification, which can significantly reduce manual processing time for handling open-ended survey responses.
Similarly, Lexalytics provides advanced sentiment analysis features, enabling deeper understanding of customer feelings. Textblob is a lightweight option that offers basic natural language processing, making it suitable for small teams. Lastly, NVivo is widely used for more complex qualitative analysis, aiding researchers in organizing and analyzing large volumes of text data efficiently. Selecting the right tool depends on the specific needs of your analysis, but these options can profoundly enhance the effectiveness of your text response analysis efforts.
- insight7
Effective Text Response Analysis serves as a cornerstone in Voice of Customer programs, transforming customer feedback into actionable insights. By examining text responses, businesses can identify common themes and sentiments, shedding light on customer preferences and suggestions. This deep dive into qualitative data fosters a profound understanding of customer needs and enhances engagement strategies.
The process involves several critical steps, beginning with data collection and aggregation of textual responses. Next, preprocessing ensures that the data is clean and manageable, paving the way for effective analytical techniques. Finally, applying these techniques, such as sentiment analysis and thematic coding, allows organizations to extract meaning from the data. Ultimately, robust Text Response Analysis enables companies to anticipate customer trends and make informed decisions that drive satisfaction and loyalty.
- MonkeyLearn
In the realm of text response analysis, utilizing advanced tools can significantly enhance your insights from Voice of Customer programs. When selecting a suitable solution, itโs essential to consider features such as sentiment analysis, entity recognition, and keyword extraction. These functionalities enable users to derive deeper meanings from customer feedback, driving informed decisions.
To effectively harness text response analysis, focus on several key aspects. First, data preprocessing is critical; it ensures the text is clean and representative of genuine feedback. Second, the application of analytics techniques can unveil trends and patterns that are otherwise hidden in raw text. Finally, adopting user-friendly technology enhances collaboration and encourages your team to engage more deeply with customer insights. By following these steps, organizations can transform extensive text data into actionable strategies that resonate with their customers.
- Lexalytics
Text Response Analysis is critical in understanding customer sentiments and feedback effectively. Utilizing advanced text analysis solutions enables organizations to transcribe and analyze responses efficiently. By ingesting data across various channels, businesses gain valuable insights, facilitating better decision-making and strategies.
The use of intuitive platforms simplifies the process, allowing users to upload audio or video files for transcription directly into projects. Once transcribed, teams can analyze these responses individually or collectively, extracting themes, generating reports, and uncovering impactful quotes. This organized approach helps prioritize actionable insights, thus enhancing the overall Voice of Customer programs.
Key aspects of a robust text response analysis solution include ease of use, data privacy compliance, and advanced analytical capabilities. By focusing on text, organizations can capture customer feedback clearly. On the roadmap, future enhancements may include tone analysis and sentiment signals to further refine understanding, ensuring a comprehensive grasp of customer perspectives for improved service and engagement.
- Textblob
Textblob is an innovative library designed for processing textual data. It simplifies the tasks of text response analysis by providing intuitive tools for sentiment analysis, classification, and translation. By harnessing Textblobโs capabilities, organizations can extract meaningful insights from customer feedback, enhancing their understanding of voice of customer programs.
Using Textblob, users can convert raw text into actionable insights efficiently. The library allows for quick sentiment assessments, helping to classify responses as positive, neutral, or negative. This feature is crucial for identifying areas where customers feel satisfied or dissatisfied. Furthermore, Textblob supports natural language processing tasks, such as noun phrase extraction, enabling users to uncover prevalent themes and topics in customer responses. Thus, incorporating Textblob into text response analysis can significantly streamline the processing and evaluation of customer feedback, ultimately contributing to improved customer interactions and experiences.
- NVivo
NVivo serves as a powerful tool for text response analysis, particularly in the context of Voice of Customer programs. This qualitative data analysis software allows you to manage, analyze, and visualize text data effectively. By leveraging its capabilities, organizations can extract meaningful insights from customer feedback, revealing themes and patterns that may not be immediately apparent.
One key aspect of using NVivo is its ability to facilitate coding, which helps in organizing responses for deeper analysis. You can create nodes to represent different themes, making it easier to categorize responses. Additionally, NVivo offers various visualization techniques, such as word clouds and charts, that allow researchers to represent their findings in an engaging and informative manner. By integrating NVivo into your text response analysis process, you can enhance the reliability of your insights and make more informed decisions that reflect customer sentiments.
Steps to Implement Text Response Analysis
To implement Text Response Analysis effectively, start with the collection and aggregation of text responses. Gather data from various customer touchpoints like surveys, reviews, and social media. This comprehensive collection ensures that your analysis will capture the full spectrum of customer feedback. Once you have collected the feedback, organize it into a manageable format, such as a database or spreadsheet, to streamline the analysis process.
The next step involves preprocessing the text data. This includes cleaning the data by removing unnecessary information, correcting spelling errors, and standardizing terminology. These actions are crucial for accurate analysis. Finally, apply analytical techniques such as theme extraction or sentiment analysis to uncover insights from the data. Techniques like word frequency analysis or text classification can also be useful depending on your goals. By following these steps, you will be positioned to extract meaningful insights to enhance your Voice of Customer programs effectively.
Step 1: Collect and Aggregate Text Responses
When embarking on Text Response Analysis, the first crucial step is to collect and aggregate text responses. Gathering qualitative data from customers helps uncover their thoughts, feelings, and motivations. Ensure that you create a well-structured process to collect feedback through surveys or interviews, focusing on open-ended questions that invite detailed responses. This richness of data is essential as it forms the basis for deeper analysis.
After collection, aggregation allows for organizing the responses into manageable formats. Utilize tools that can combine multiple text inputs, making the data easier to analyze collectively. This step may involve categorizing responses by themes or key topics, providing a clearer overview of customer sentiments. Adopting a comprehensive approach at this stage sets the foundation for effective analysis, enabling you to extract meaningful insights that drive improvements in your voice of the customer programs.
Step 2: Preprocess the Text Data
Preprocessing text data is a crucial step in the analysis of text responses in Voice of Customer programs. This phase transforms raw text into a structured format, facilitating a better understanding of customer sentiments and insights. Initially, you need to clean the data by removing irrelevant information, such as punctuation and special characters. This ensures that the analysis focuses solely on the meaningful content.
Next, consider standardizing the text by converting all words to a common format, typically lowercase. This helps to maintain consistency and aids in accurate interpretation during the analysis phase. Finally, tokenization is essential, wherein you break down the text into individual components, or tokens, like words or phrases, for easier processing. By diligently preprocessing the text data, you lay a strong foundation for effective text response analysis, enabling deeper insights into customer feedback and preferences.
Step 3: Apply Analytical Techniques
Analyzing text responses is an essential phase in enhancing Voice of Customer programs. In this step, various analytical techniques are applied to identify themes, sentiments, and trends within the data. The goal is to extract meaningful insights that can guide decision-making and strategy improvements. By utilizing analytical tools, you can efficiently categorize responses into distinct themes, such as risks, challenges, and customer needs, allowing for a clearer understanding of customer sentiments.
Once you have established your themes, focus on sentiment analysis to gauge how customers feel about particular aspects of your products or services. This analysis can reveal critical areas for improvement while pinpointing strengths to amplify in future initiatives. Ultimately, applying robust analytical techniques ensures that your understanding of customer feedback leads to meaningful action, reinforcing a customer-centric approach in your organization.
Conclusion: The Impact of Improved Text Response Analysis on Voice of Customer Programs
Improved text response analysis significantly enhances the effectiveness of Voice of Customer programs. By streamlining the process of extracting insights from customer feedback, organizations can respond more swiftly to customer needs and preferences. This ongoing analysis allows for the identification of key themes and patterns, ultimately leading to actionable strategies that improve customer satisfaction and loyalty.
Moreover, refining text response analysis helps reduce bias and inconsistency in interpreting data. When teams utilize a structured approach to analyze feedback, they can generate insights that are not only insightful but also reliable. Consequently, organizations can better tailor their products and services to align with customer expectations, fostering a customer-centric culture that values continuous improvement.
Analyze & Evaluate Calls. At Scale.
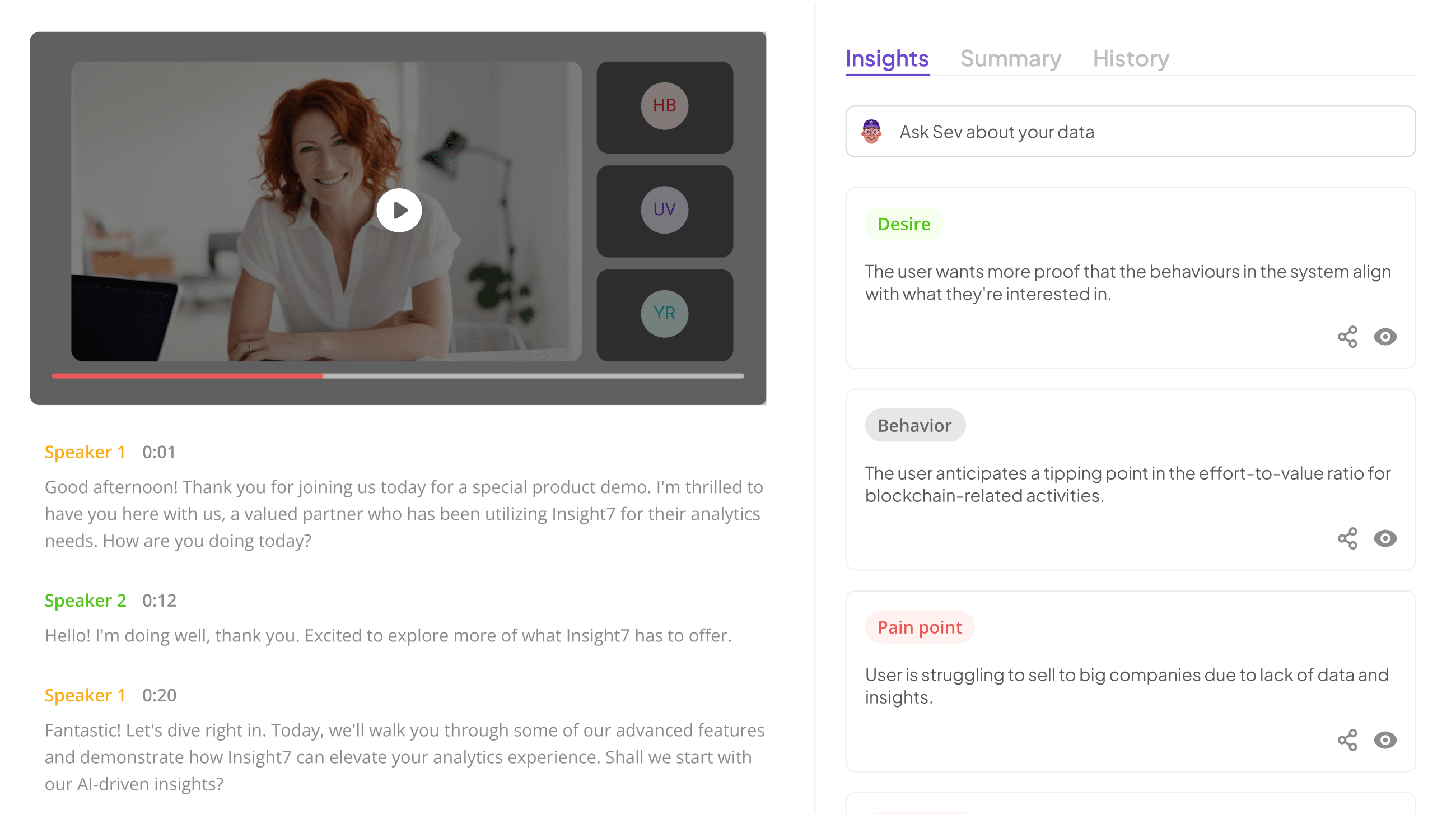
๐ฌ Need Help? Chat with Support
Our team typically responds within minutes