AI Tools for Tagging Focus Group Responses by Product Feature
-
Bella Williams
- 10 min read
Feature-based Response Tagging serves as a pivotal approach in the realm of AI tools, particularly in the analysis of focus group feedback. This technique enhances the accuracy and efficiency of understanding customer insights by categorizing responses based on specific product features. As businesses strive to glean actionable insights from qualitative data, the need for precise tagging mechanisms becomes increasingly vital.
Moreover, traditional methods of analyzing focus group responses can often lead to inconsistent findings and slow report generation. By implementing advanced AI solutions that specialize in Feature-based Response Tagging, teams can streamline their analysis processes. This not only reduces the time needed for generating insights but also fosters a more structured and unbiased understanding of customer opinions. With these tools, organizations can effectively respond to client demands for timely and reliable insights.
Transcribe & extract insights from interviews. At Scale.
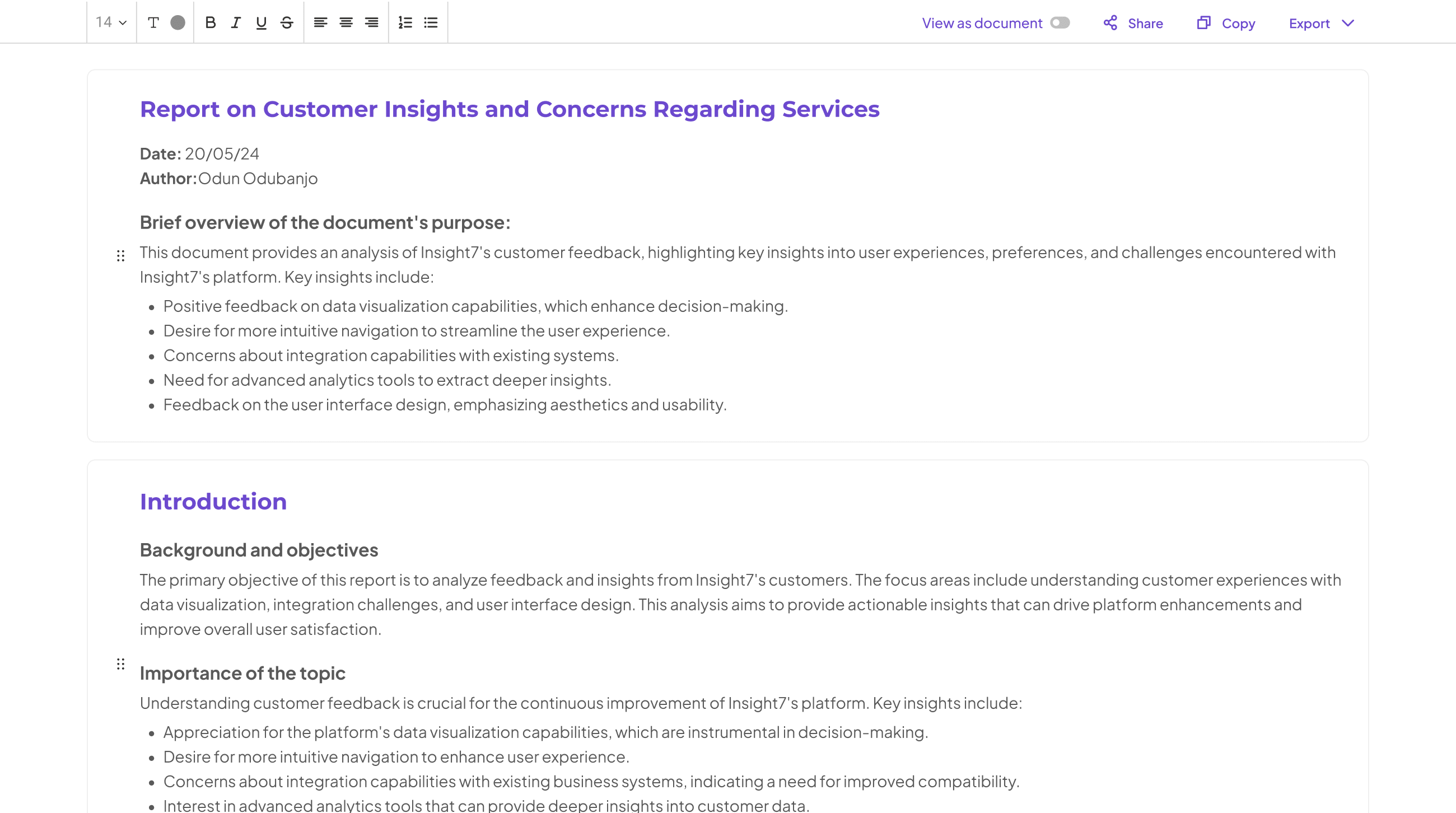
Understanding the Importance of Feature-based Response Tagging
Feature-based Response Tagging plays a crucial role in analyzing focus group data. This method allows researchers to categorize insights based on specific product features, making it easier to identify trends and patterns. For instance, when responses are tagged according to the features they discuss, it becomes simpler to evaluate which aspects resonate with participants the most. This targeted approach not only enhances the depth of analysis but also streamlines the extraction of actionable insights.
Understanding the significance of Feature-based Response Tagging empowers organizations to refine their products and services. By properly tagging feedback, businesses can address specific needs and pain points expressed by focus group participants. Moreover, it encourages a data-driven decision-making process. As groups share their thoughts, tagging responses leads to clearer pathways for handling recommendations and addressing areas needing improvement, ensuring that user experiences are always prioritized.
The Role of Tagging in Focus Group Analysis
In focus group analysis, tagging plays a crucial role in organizing and interpreting participant feedback. By effectively implementing feature-based response tagging, you can distill complex discussions into manageable themes. This process enables researchers to identify prevalent sentiments, challenges, and desires related to specific product features. With AI tools, you can create default tags or customize them according to your analytical needs, ensuring you capture the most relevant insights.
Additionally, tagging allows for a structured approach to sentiment analysis, where comments can be categorized based on user experiences. This means you can assess how participants feel about collaboration tools or pinpoint potential risks tied to a product feature. The real power of tagging lies in its ability to streamline data analysis, making it more efficient and impactful. Overall, utilizing feature-based response tagging transforms focus group responses into strategic insights that drive meaningful product decisions.
Benefits of Feature-based Response Tagging
Feature-based response tagging plays a crucial role in streamlining the analysis of focus group discussions. By categorizing comments according to specific product features, this method enables researchers to quickly identify patterns and sentiments linked to each feature. This approach not only enhances the clarity of insights but also ensures that feedback is organized efficiently. Consequently, decision-makers can prioritize product improvements more effectively based on direct consumer feedback.
Implementing feature-based response tagging can significantly save time. Instead of manually sifting through numerous responses, teams can rely on AI tools to automate tagging, allowing them to focus on interpreting the data. Furthermore, this method fosters a deeper understanding of customer needs, as it aligns insights with the features that matter most to users. As a result, organizations can better tailor their products and marketing strategies, ultimately driving user satisfaction and engagement.
Generate Detailed Reports from Your Qualitative Data in Minutes.
Exploring AI Tools for Feature-based Response Tagging
AI technologies have made significant strides in improving the efficiency of feature-based response tagging. Today, advanced AI tools enable researchers to automate the analysis of focus group insights, reducing manual effort and minimizing biases. These tools not only enhance the accuracy of tagging responses based on product features but also expedite the overall research process. Utilizing AI for feature-based response tagging empowers teams to deliver quicker reports and actionable insights, addressing the growing demand for timely data analysis.
Several AI tools stand out in this realm. Insight7 provides comprehensive features tailored specifically for qualitative analysis, allowing teams to quickly identify key themes related to product features. MonkeyLearn offers versatile applications that can be custom-fit to various tagging requirements, making it ideal for diverse industries. Tagtog excels in facilitating user-friendly tagging experiences, while Thematic provides robust support specifically for linking responses to product attributes. Lastly, integration with tools like ChatGPT can further streamline the tagging process. Such tools not only enhance efficiency but also ensure that insights are both reliable and comprehensive.
Top AI Tools for Tagging
When considering the best AI tools for tagging focus group responses, several options stand out. These tools are designed to streamline the process of identifying trends and insights within participant feedback. Each tool offers unique features that cater to various tagging needs while enhancing efficiency and accuracy.
First, insight7 provides a comprehensive suite for analyzing customer feedback, focusing on feature-based response tagging. It allows researchers to categorize responses effortlessly, making it easier to pinpoint relevant themes. Similarly, MonkeyLearn excels in machine learning capabilities, offering seamless integration of tagging functions to improve analysis outcomes. Tagtog stands out for its user-friendly interface, allowing non-technical users to quickly adapt and utilize tagging features effectively. Thematic specializes in organizing insights around product features, enabling teams to derive actionable conclusions. Finally, ChatGPT can assist in tagging processes by synthesizing responses intelligently, fostering deeper understanding and analysis of focus group data.
Selecting the right AI tool requires consideration of several key criteria. These include the tool’s ease of use, the ability to customize tagging frameworks, integration capabilities with existing systems, and the accuracy of its machine learning algorithms. Ensuring these factors align with your project requirements can significantly enhance your analysis of focus group responses.
- insight7: Overview and Features
The section delves into the functionalities and advantages of a leading AI tool dedicated to tagging focus group responses effectively. The insight7 platform streamlines the process of Feature-based Response Tagging, allowing users to gain actionable insights with ease. It features a user-friendly interface, making it accessible for individuals without technical expertise. Simply put, anyone can utilize the app's capabilities with minimal guidance.
Key aspects of insight7 include its dual components: Projects and Library. The Library stores all data seamlessly while Projects enable users to cluster and analyze relevant information efficiently. With tools that facilitate quick data importation and intuitive analysis options, the platform enhances the user experience dramatically. Additionally, pre-built analysis kits offer a head start, allowing users to extract essential insights in a fraction of the time. Thus, insight7 exemplifies how AI tools can transform the tagging process, making it simpler and more effective for identifying key product features in focus group discussions.
- MonkeyLearn: Capabilities and Applications
In the realm of feature-based response tagging, a popular AI tool excels by offering a versatile platform designed for analyzing focus group data. This tool allows users to effortlessly upload data from various sources and extract critical insights, making the analysis process significantly more efficient. With capabilities such as native transcription that supports over 60 languages and an accuracy rate of 95%, users can quickly convert raw interviews into actionable insights.
Additionally, the platform facilitates the organization of data into projects, allowing teams to collaboratively analyze patterns and themes. Users can generate reports and visualizations tailored to specific needs, enhancing their ability to communicate findings effectively. By prioritizing data privacy and compliance, this tool ensures that sensitive information remains secure throughout the analysis process. Overall, its robust capabilities cater to diverse industries, empowering organizations to translate focus group responses into strategic actions successfully.
- Tagtog: Features and User Experience
Tagtog offers an array of features designed to enhance user experience in feature-based response tagging. Users can configure customizable theme boards according to specific use cases, allowing for a tailored analysis of focus group responses. This flexibility enables users to define what insights they wish to extract, such as pain points, desires, and behaviors, which are then systematically clustered and organized for deeper exploration.
With an intuitive interface, Tagtog empowers users to navigate and analyze data effectively. Users can filter insights by speaker or type, providing a granular view of feedback. Additionally, the platform supports the creation of detailed reports by summarizing key findings and generating outlines based on selected insights. This enhances the overall experience, making response analysis not only efficient but also productive, allowing for informed decision-making based on customer feedback.
- Thematic: How it Supports Feature Tagging
Thematic analysis plays a pivotal role in feature-based response tagging, particularly in organizing qualitative data from focus group responses. By identifying recurring themes and patterns within responses, this approach allows researchers to categorize insights more effectively. Each theme serves as a lens through which product features can be scrutinized, breaking down user sentiments and preferences regarding specific functionalities.
Implementing thematic analysis yields several benefits for feature-based response tagging. First, it allows for a structured understanding of user feedback, making it easier to pinpoint areas needing improvement. Second, themes drawn from responses highlight varying perspectives on product features, giving stakeholders a comprehensive view of user experience. Lastly, the streamlined process of categorizing responses ensures that essential insights are not overlooked, facilitating clearer communication within teams. By using these techniques, organizations can transform focus group data into actionable insights, ultimately aligning product offerings with user needs and expectations.
- ChatGPT: Integration with Tagging Processes
Integrating ChatGPT into tagging processes can significantly enhance feature-based response tagging. This AI tool enables users to analyze focus group responses more efficiently and accurately. By processing large volumes of text, ChatGPT identifies keywords and themes that align with product features. Consequently, it saves time previously spent on manual tagging while ensuring consistency across data sets.
One of the key advantages of utilizing ChatGPT is its ability to provide nuanced insights by recognizing patterns in participant feedback. It can automate the extraction of significant quotes, themes, and relevant product features, transforming qualitative data into actionable insights. As a result, teams equipped with ChatGPT can focus on strategic decision-making rather than getting bogged down in manual analysis. This integration not only streamlines the tagging process but also enriches the overall analysis of focus group discussions.
Key Criteria for Selecting AI Tools
Selecting the right AI tools for feature-based response tagging is crucial for accurate data analysis. Start with the technology's ability to handle diverse data sources and analyze responses effectively. Consider tools that offer robust transcription capabilities, allowing for the quick conversion of discussions into text. Speed is also vital, so prioritize solutions that can provide insights promptly, which helps to meet client expectations for timely results.
Another key criterion is ease of use. The interface should be intuitive, enabling users to tag efficiently without extensive training. Data security is paramount as well; ensure the tool complies with GDPR and other regulations. Finally, look for solutions that offer customization options for tagging based on specific needs. These factors combined will lead to better outcomes in feature-based response tagging, enhancing your analysis and reporting processes.
Conclusion: Leveraging AI for Efficient Feature-based Response Tagging
In conclusion, utilizing AI for efficient feature-based response tagging transforms the analysis of focus group responses. By adopting cutting-edge tools, teams can streamline their process, minimizing manual labor while enhancing accuracy and consistency. These tools categorize responses based on product features, allowing for quicker insights and timely reporting, which are critical for effective decision-making.
Furthermore, the integration of AI tools alleviates common challenges faced during qualitative research. Teams can generate comprehensive reports with reduced bias and improved clarity, enabling a more informed understanding of customer feedback. As a result, organizations can react swiftly to market needs, fostering innovation and competitive advantage in their respective industries.
💬 Need Help? Chat with Support
Our team typically responds within minutes