Sentiment-Adaptive Coaching opens new avenues for effective communication between agents and users, making interactions more intuitive and personalized. As technology evolves, understanding emotional context becomes crucial. Large Language Models (LLMs) are at the forefront of this shift, enabling adaptive coaching that responds to an agent's sentiment.
This innovative approach not only enhances engagement but also fosters deeper understanding. By analyzing emotions, LLMs can tailor responses and learning experiences to meet individual needs. The integration of sentiment analysis in coaching allows for more dynamic and responsive interactions, ensuring that users feel heard and supported throughout their journey.
Analyze qualitative data. At Scale.
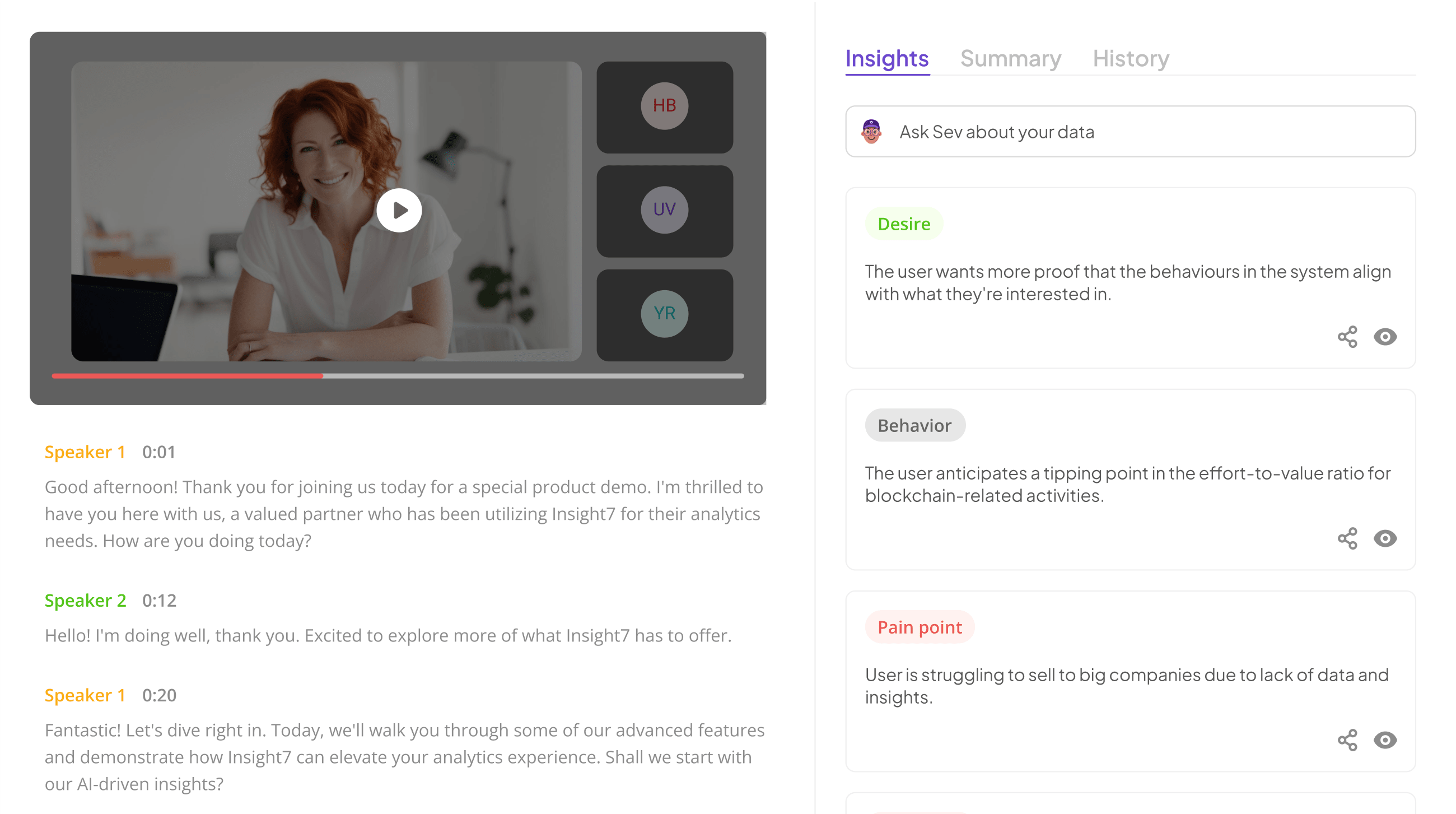
The Mechanics of Sentiment-Adaptive Coaching in LLMs
Sentiment-Adaptive Coaching in LLMs operates on a layered mechanism that enhances user interaction and overall effectiveness. By analyzing emotional cues within communication, LLMs can tailor their coaching strategies to meet specific user needs. This adaptive approach ensures that coaching is not only relevant but also empathetic, addressing the emotional state of the user to foster a more supportive learning environment.
The mechanics involve several key components essential for successful implementation. First, real-time sentiment analysis processes emotional data from user interactions. Second, feedback loops are integrated into the coaching workflow, enabling LLMs to adjust responses dynamically. Third, thorough training using sentiment-rich datasets enhances the model's ability to recognize diverse emotional states. Together, these elements create a robust system that empowers LLMs to deliver personalized coaching aligned with individual emotional contexts. This depth of engagement could significantly improve the overall user experience and learning outcomes in various applications.
Understanding LLMs: Basics and Potential
Large Language Models (LLMs) present remarkable opportunities in enhancing interpersonal interactions through advanced adaptive learning. At their core, LLMs are powerful algorithms designed to understand and generate human-like text based on vast datasets. They possess the ability to analyze language patterns, making them ideal for applications such as Sentiment-Adaptive Coaching, where the focus is on modifying coaching techniques based on real-time emotional responses.
The potential of LLMs goes beyond simple text generation. They can enrich the coaching experience by responding dynamically to the sentiments expressed during conversations. This adaptive approach not only improves the quality of the coaching provided but also fosters better engagement and understanding. By integrating sentiment analysis, coaches can receive valuable insights, enabling them to adjust their methods to effectively meet the emotional needs of their clients. This evolving landscape of coaching powered by LLMs promises to revolutionize how personal development is approached, ensuring it is both responsive and effective.
- Brief overview of Large Language Models (LLMs)
Large Language Models (LLMs) are advanced AI systems designed to understand and generate human-like text. They are built on large datasets, allowing them to comprehend context, semantics, and nuances in language. As a result, LLMs can produce coherent and relevant responses across a variety of domains, making them powerful tools for adaptive coaching scenarios.
In the realm of Sentiment-Adaptive Coaching, LLMs analyze user sentiment through natural language processing techniques. This enables them to adjust their feedback and guidance based on emotional cues. For instance, if a customer service representative is perceived to be frustrated, the LLM can alter its responses to better support a positive interaction. By integrating sentiment analysis, LLMs not only enhance communication but also contribute to more personalized coaching experiences, ultimately improving performance outcomes for users.
- The potential of LLMs in adaptive learning environments
In adaptive learning environments, the potential of Large Language Models (LLMs) cannot be overstated. These advanced models can analyze emotional cues from user interactions, allowing them to tailor educational content accordingly. By understanding learner sentiment, LLMs can adapt coaching strategies in real time, ensuring that educational experiences are personalized and relevant.
The ability to provide Sentiment-Adaptive Coaching is a game changer for educators and trainers. It enables LLMs to identify when learners are struggling or disengaged, allowing for timely interventions. This adaptive capability fosters an engaging learning atmosphere where feedback is immediate and attuned to individual emotional states. As a result, not only can learners receive content that aligns with their feelings, but they can also benefit from a support system that encourages deeper understanding and progress. Thus, LLMs represent a transformative approach to enhancing education through emotional intelligence and adaptability.
Sentiment Analysis: The Heartbeat of Adaptive Coaching
Sentiment analysis serves as the foundation of Sentiment-Adaptive Coaching, playing a critical role in the adaptability of coaching models. Through careful examination of emotional cues, coaches can tailor their responses to better meet the needs of learners. This heightened awareness allows for a more engaging and personalized learning experience, ultimately leading to improved outcomes.
By integrating sentiment analysis into the coaching process, training programs can become more dynamic. Coaches can identify emotional fluctuations and provide support that aligns with the learner's current state. Understanding these sentiments paves the way for not just reactive but proactive coaching. Thus, the ability to adapt based on emotional insights fosters a stronger relationship between the coach and learner, facilitating a sustainable growth environment. The heartbeat of successful coaching resides in the understanding of sentiment, ensuring that responses are not only timely but also empathetic.
- Definition and importance of sentiment analysis
Sentiment analysis involves the systematic identification and categorization of emotional states expressed in textual data. This process plays a pivotal role in understanding user emotions, preferences, and behaviors, enabling more personalized experiences. In the context of Sentiment-Adaptive Coaching, sentiment analysis becomes essential, as it allows coaches to tailor their approaches based on the emotional cues of learners. By analyzing feedback and sentiments, coaches can discern pain points, motivations, and areas needing improvement.
The importance of sentiment analysis cannot be overstated. It provides valuable insights into learner engagement, helping coaches adapt their strategies in real-time. When Agent Sentiments are accurately assessed, LLMs can adjust their coaching methods to better resonate with users. This adaptive capability not only enhances learning outcomes but also fosters a supportive environment that encourages growth and development. Ultimately, embracing sentiment analysis in coaching practices leads to richer and more effective interactions.
- How sentiment analysis fuels coaching adaptability in LLMs
Effective sentiment analysis plays a crucial role in enhancing the adaptability of coaching provided by large language models (LLMs). By examining the emotional tone of user interactions, LLMs can tailor their responses and coaching strategies to match the sentiments expressed by agents. This approach, known as Sentiment-Adaptive Coaching, enables a more personalized and responsive learning environment, which can lead to improved engagement and retention.
The adaptability offered by sentiment analysis fosters dynamic coaching interactions. When LLMs can detect when an agent feels uncertain, frustrated, or confident, they can adjust their feedback accordingly. For instance, if an agent displays signs of anxiety during a training exercise, the LLM can offer reassurance and encouragement. Conversely, if an agent shows confidence, the LLM can challenge them with more advanced content. This capability ensures that coaching is not only reactive but also proactive, allowing agents to grow within their roles and enhance performance.
Tools and Technologies Enabling Sentiment-Adaptive Coaching
Sentiment-Adaptive Coaching relies heavily on innovative tools and technologies that enhance the coaching experience. By leveraging advanced capabilities of Large Language Models (LLMs), sentiment analysis can be seamlessly integrated into coaching frameworks. This process begins with the collection of varied data sources, such as transcripts, audio, and video files. Effective transcription engines convert these inputs into actionable insights, helping coaches understand emotional nuances in real-time.
Key technologies play a vital role in facilitating this adaptive coaching. Platforms included in this discourse, like IBM Watson, OpenAIโs ChatGPT, and Microsoft Azure AI, utilize sentiment analysis to tailor feedback based on individual needs. These tools offer features that not only enhance the learning experience but also help mitigate biases in insights. By transforming raw data into visual journey maps and thematic reports, coaching becomes more personalized and effective, ultimately allowing coaches to act on insights quickly and confidently.
Extract insights from interviews, calls, surveys and reviews for insights in minutes
Top LLM Tools for Sentiment-Adaptive Coaching
In the realm of sentiment-adaptive coaching, various LLM tools stand out for their ability to analyze emotions and tailor coaching strategies accordingly. These tools utilize advanced algorithms to decode agent sentiments, ensuring a more personalized coaching experience. This adaptability not only enhances learning outcomes but also fosters an environment where agents feel understood and supported.
The leading tools in this space include IBM Watson, which elegantly combines AI with sentiment analysis for dynamic coaching solutions. OpenAI's ChatGPT excels at adapting responses by recognizing emotional cues, while Microsoft Azure AI offers valuable sentiment insights for customized feedback. Google Cloud NLP is transforming coaching practices through its emotional intelligence capabilities, allowing for a nuanced approach to agent development. These platforms collectively embody the progress of sentiment-adaptive coaching and their potential to reshape coaching landscapes for various organizations.
- insight7: Leading the way in sentiment-driven coaching
Sentiment-driven coaching represents a transformative approach to training and engagement, especially in leveraging large language models (LLMs). Leading the way in this evolution, a new paradigm focuses on understanding and responding to agent sentiment during interactions. By prioritizing emotional intelligence in coaching techniques, organizations can foster more personalized and effective training experiences.
In this narrative, sentiment-adaptive coaching emerges as a key differentiator. It allows coaches to interpret the emotional undertones of conversations, adjusting their methodologies to suit the emotional state of clients. This responsiveness enhances engagement, making learning opportunities more effective and relevant. As LLMs evolve, the integration of sentiment analysis will further refine coaching practices, ensuring that every interaction is not only informative but also empathetic. Ultimately, this pioneering approach to coaching emphasizes both the cognitive and emotional dimensions of learning, setting the stage for more meaningful connections in professional development.
- IBM Watson: Integrating AI and sentiment for dynamic coaching
The integration of AI into dynamic coaching systems has opened new avenues for enhancing agent performance. By employing advanced sentiment analysis techniques, AI can adapt coaching strategies in real-time, ensuring that guidance resonates with each agent's emotional state. This methodology, known as Sentiment-Adaptive Coaching, trains agents in a more personalized manner by recognizing their needs and motivations through sentiment data.
Through continuous monitoring of emotional responses, AI-driven systems can identify when agents may become disengaged or overwhelmed. This concept allows for timely interventions, ensuring agents receive support tailored to their specific situational context. Consequently, the coaching process becomes more fluid and responsive, ultimately promoting a deeper connection between agents and the system. As AI capabilities continue to advance, incorporating sentiment analysis will remain critical for achieving effective and meaningful coaching experiences in evolving professional environments.
- OpenAIs ChatGPT: Adapting responses with emotion recognition
OpenAIs ChatGPT plays a crucial role in sentiment-adaptive coaching by effectively recognizing and responding to users' emotions. This capability allows the model to tailor its interactions based on the emotional state of the user, fostering a more personalized coaching experience. By analyzing sentiment, it can identify when a user is feeling stressed, confused, or enthusiastic and adjust its responses accordingly.
The integration of emotion recognition enhances the coaching dialogues, making them more relevant and timely. For instance, if a user expresses frustration, the model can pivot to a more supportive and reassuring tone, providing guidance that aligns with the userโs current emotional needs. This dynamic interaction not only improves the coaching relationship but also aids in achieving learning objectives more effectively. Essentially, sentiment-adaptive coaching through emotional awareness turns generic exchanges into meaningful conversations that resonate with users and support their growth.
- Microsoft Azure AI: Utilizing sentiment insights for tailored feedback
Understanding sentiment insights is pivotal in creating a more personalized coaching experience for agents. In the context of sentiment-adaptive coaching, it allows LLMs to effectively interpret emotional cues from customer interactions. By harnessing these insights, organizations can tailor feedback to better meet individual agent needs and enhance their performance.
The incorporation of advanced AI technologies enables these models to gauge emotional states during interactions. This responsive mechanism allows for immediate adjustments based on agent sentiment, providing a platform for constructive feedback tailored to specific challenges faced by the agents. Continuous monitoring of emotional metrics ensures that the coaching is dynamic and aligns with the agents' real-time experiences. Consequently, utilizing sentiment insights leads to more engaged agents and improved customer interactions, marking a significant leap forward in personalized development strategies.
- Google Cloud NLP: Transforming coaching with emotional intelligence
Using advanced technology, it is now possible to enhance coaching through emotional intelligence. By focusing on sentiment analysis, coaching methodologies can evolve to be more responsive and personalized. Sentiment-Adaptive Coaching utilizes insights derived from language models to understand individual emotions. This understanding allows coaches to tailor their approach to each client's emotional state, significantly improving engagement and effectiveness.
Emotional intelligence in coaching can transform interactions. The analysis of verbal and non-verbal cues enables coaches to respond appropriately to their clients' needs. For example, adapting communication styles based on real-time sentiment can help foster a supportive environment. This innovation not only streamlines the coaching process but also empowers clients. When clients feel understood, they are more likely to open up, leading to deeper insights and improved outcomes. Ultimately, integrating this technology into coaching practices can significantly enhance the overall experience for both coaches and clients alike.
Implementing Sentiment-Adaptive Coaching: Steps for Success
Implementing Sentiment-Adaptive Coaching involves several key steps that set the foundation for successful integration. First, you must identify the right large language model (LLM) that aligns with your specific coaching needs. This requires assessing the features and capabilities of various models to determine which best supports your objectives. Next, training LLMs with sentiment-based data becomes critical. This step involves collecting and tagging datasets rich in emotional context, ensuring the models are fine-tuned to recognize and respond to sentiment effectively.
Integrating real-time feedback loops is the third vital step in this process. Establishing continuous sentiment monitoring allows for immediate adjustments to coaching strategies based on agent emotions. By implementing these structured steps, organizations can enhance coaching effectiveness and foster a deeper, more intuitive connection with agents, ultimately driving performance and satisfaction in the workplace.
Step 1: Identifying the Right LLM for Your Needs
Selecting the right LLM for your needs is pivotal in implementing effective sentiment-adaptive coaching. Begin by evaluating the specific features that you require. Consider the capabilities of the LLM, such as its ability to analyze sentiment in real time, its adaptability to different coaching methodologies, and how well it integrates with existing tools. Knowing your coaching objectives will guide you in narrowing down your options.
Next, align the LLM's capabilities with your intended outcomes. If the goal is to enhance engagement during client interactions, prioritize LLMs that provide tailored feedback based on emotional cues. Additionally, consider the support services offered, such as technical assistance and implementation guidance. This ensures a smoother transition and maximizes the effectiveness of sentiment-adaptive coaching in your organization. With thorough evaluation and alignment, you will be well on your way to harnessing the full potential of sentiment-adaptive coaching solutions.
- Assessing feature requirements and capabilities
When exploring the feature requirements and capabilities for Sentiment-Adaptive Coaching, it's crucial to recognize the importance of aligning technology with coaching objectives. This involves clearly defining what the coaching process should achieve and what features will support those goals. Key capabilities may include advanced sentiment analysis tools, performance metrics for coaching effectiveness, and integration options with existing systems.
A thorough assessment should consider the technical requirements needed for proper functionality. This includes API integration capabilities for seamless data exchange, along with robust data management systems for secure storage and accessibility of coaching records. Additionally, evaluating user experience and communication styles ensures that the LLM is equipped to foster empathetic interactions, enhancing the overall coaching experience. By focusing on these features, organizations can successfully implement a coaching model that adapts to agent sentiment dynamically.
- Aligning LLM choice with coaching goals
Choosing the right Large Language Model (LLM) is essential for effective sentiment-adaptive coaching. This decision should align closely with your coaching goals to enhance overall impact. For instance, if your focus is on promoting empathy and understanding during coaching sessions, an LLM equipped with robust sentiment analysis capabilities will better serve that purpose.
When selecting an LLM, consider key aspects such as its ability to process emotional nuances and adapt responses accordingly. Additionally, look for features that allow seamless integration with your existing tools, such as project management and analysis mechanisms. By ensuring that the chosen LLM complements your coaching methodology, you create a more engaging and responsive environment for both coaches and clients. Ultimately, this strategic alignment will facilitate richer interactions and better outcomes, steering your sentiment-adaptive coaching efforts toward greater success.
Step 2: Training LLMs with Sentiment-Based Data
Training LLMs with sentiment-based data is essential for developing effective Sentiment-Adaptive Coaching systems. This process involves collecting sentiment-rich datasets that reflect varied emotional responses from users. By tagging this data accurately, we enable LLMs to recognize and interpret nuanced sentiments, paving the way for more adaptive coaching interactions.
The next step is fine-tuning LLMs to enhance their sensitivity to these sentiments. This involves adjusting model parameters and training techniques to ensure that the model not only understands the emotional context but also responds in a way that aligns with the userโs emotional state. Through this meticulous process, LLMs can provide guidance that resonates with users, ensuring a more personalized coaching experience. Such an approach ultimately transforms how agents receive feedback and support, making Sentiment-Adaptive Coaching a powerful tool for growth and engagement.
- Collecting and tagging sentiment-rich datasets
Collecting and tagging sentiment-rich datasets is a crucial step in developing Sentiment-Adaptive Coaching systems. Data collection begins with identifying relevant sources, such as customer feedback, chat logs, and social media interactions. Gathering diverse content ensures a comprehensive view of emotional expressions and behavior patterns. This process involves scrutinizing existing datasets and, when necessary, creating custom datasets tailored to specific coaching scenarios.
Once data is collected, tagging emerges as a significant task. Each dataset must undergo sentiment labeling, where emotions are categorized, from joy to frustration. This tagging process enables LLMs to recognize emotional cues accurately, fostering effective coaching responses. Quality control during tagging is critical, as misclassified sentiments can lead to incorrect coaching strategies. Overall, meticulous collecting and tagging paves the way for LLMs to adapt their coaching based on real-time sentiment analytics, enhancing user interaction and outcomes.
- Fine-tuning LLMs for sentiment sensitivity
Fine-tuning LLMs for sentiment sensitivity plays a pivotal role in creating Sentiment-Adaptive Coaching systems. This process begins by gathering extensive sentiment-rich datasets. Training LLMs on this data allows the models to understand the nuances of human emotions in conversations. By incorporating varied emotional contexts, LLMs become more responsive and adaptable to user sentiments.
Next, the fine-tuning stage involves adjusting model parameters to enhance sensitivity towards emotional cues. This entails both supervised learning, where the model learns from labeled data, and reinforcement learning, which optimizes its performance through continuous interaction. Once these models are fine-tuned, they can generate more empathetic and contextually appropriate responses, improving the overall coaching experience. In essence, this meticulous process ensures that LLMs not only comprehend text but also navigate the intricate landscape of human emotions effectively.
Step 3: Integrating Real-Time Feedback Loops
Integrating real-time feedback loops is crucial for making Sentiment-Adaptive Coaching effective. This approach enables LLMs to continuously monitor agent sentiment, allowing for immediate adjustments in coaching strategies. By collecting user insights as they interact with the system, organizations can better cater their responses to meet the emotional needs of agents. This not only enhances engagement but also promotes a sense of understanding and support between the LLM and users.
To implement effective feedback loops, focus on three key components. First, establish a responsive monitoring system to detect sentiment fluctuations during interactions. Next, ensure that LLMs can analyze this data in real-time, allowing for prompt adjustments in the coaching narrative. Lastly, regularly review and refine these feedback mechanisms to enhance responsiveness. This iterative process leads to more personalized and effective coaching experiences. Through such integration, Sentiment-Adaptive Coaching becomes a transformative tool for developing engaged and resilient agents.
- Setting up continuous sentiment monitoring
Continuous sentiment monitoring is essential for effective Sentiment-Adaptive Coaching. This process involves systematically gathering and analyzing sentiments expressed in various interactions, enabling real-time adjustments to coaching methods based on agent needs. By implementing tools that can analyze verbal and non-verbal cues, organizations can gain profound insights into agent sentiment, fostering a more supportive coaching environment.
To set up continuous sentiment monitoring effectively, consider these steps:
Select Appropriate Tools: Choose platforms equipped with sentiment analysis capabilities that align with your coaching objectives.
Incorporate Feedback Mechanisms: Establish processes for agents to provide ongoing feedback, ensuring their sentiments are consistently captured.
Analyze and Act on Data: Regularly assess the gathered sentiment data and adjust coaching strategies accordingly, creating a dynamic feedback loop that enriches the coaching experience for agents.
These steps facilitate a proactive approach, ensuring that coaching remains responsive to individual emotions and overall team sentiment dynamics.
- Adjusting coaching strategies in real-time based on feedback
Real-time feedback plays a pivotal role in shaping coaching strategies, particularly within the realm of Sentiment-Adaptive Coaching. By continuously monitoring an agent's emotional state, coaches can fine-tune their approaches to cater to specific needs and enhance learning outcomes. This adaptive model allows for a responsive coaching experience, where strategies are adjusted on-the-fly based on the sentiments expressed by agents.
To effectively adjust coaching strategies, consider the following steps:
Monitor Sentiment Indicators: Utilize tools that track agent sentiment continuously. These indicators will inform whether an agent is experiencing frustration, confusion, or motivation.
Evaluate Feedback Loops Regularly: Establish clear channels for receiving real-time feedback from agents. This feedback can highlight areas needing immediate adjustment.
Customize Coaching Approaches: Tailor coaching tactics dynamically, utilizing insights from sentiment analysis. This personalization ensures that agents receive support relevant to their emotional states at any given moment.
By implementing these steps, coaches can foster an environment that prioritizes adaptability, creating a more supportive and effective learning experience.
Conclusion: Embracing the Future of Sentiment-Adaptive Coaching in LLMs
The future of sentiment-adaptive coaching in LLMs holds immense promise for enhancing personalized learning experiences. By harnessing the power of real-time sentiment analysis, we can tailor coaching interventions to meet individual needs effectively. This innovative approach ensures that feedback and guidance are responsive, making interactions more engaging and effective for users.
As we embrace this future, the collaboration between technology and emotional intelligence will reshape the coaching landscape. Organizations that prioritize sentiment-adaptive coaching will not only improve learner outcomes but also foster deeper connections. Ultimately, the integration of sentiment analysis into LLMs represents a transformative leap towards more intelligent and empathetic coaching frameworks.