7 AI Features That Save Time in QA Monitoring
-
Bella Williams
- 10 min read
In today's fast-paced digital environment, maintaining quality assurance (QA) standards is more crucial than ever. With increasing demands for efficiency, organizations are turning to innovative technology to streamline monitoring processes. AI QA efficiency allows teams to rapidly analyze data and gain insights that were previously time-consuming.
This section delves into how artificial intelligence enhances QA monitoring by automating tasks, detecting anomalies, and providing real-time feedback. By harnessing these capabilities, companies can improve the accuracy of their assessments and significantly reduce the time spent on manual reviews. Understanding AI QA efficiency will empower organizations to make informed decisions and optimize their operations.
Analyze & Evaluate Calls. At Scale.
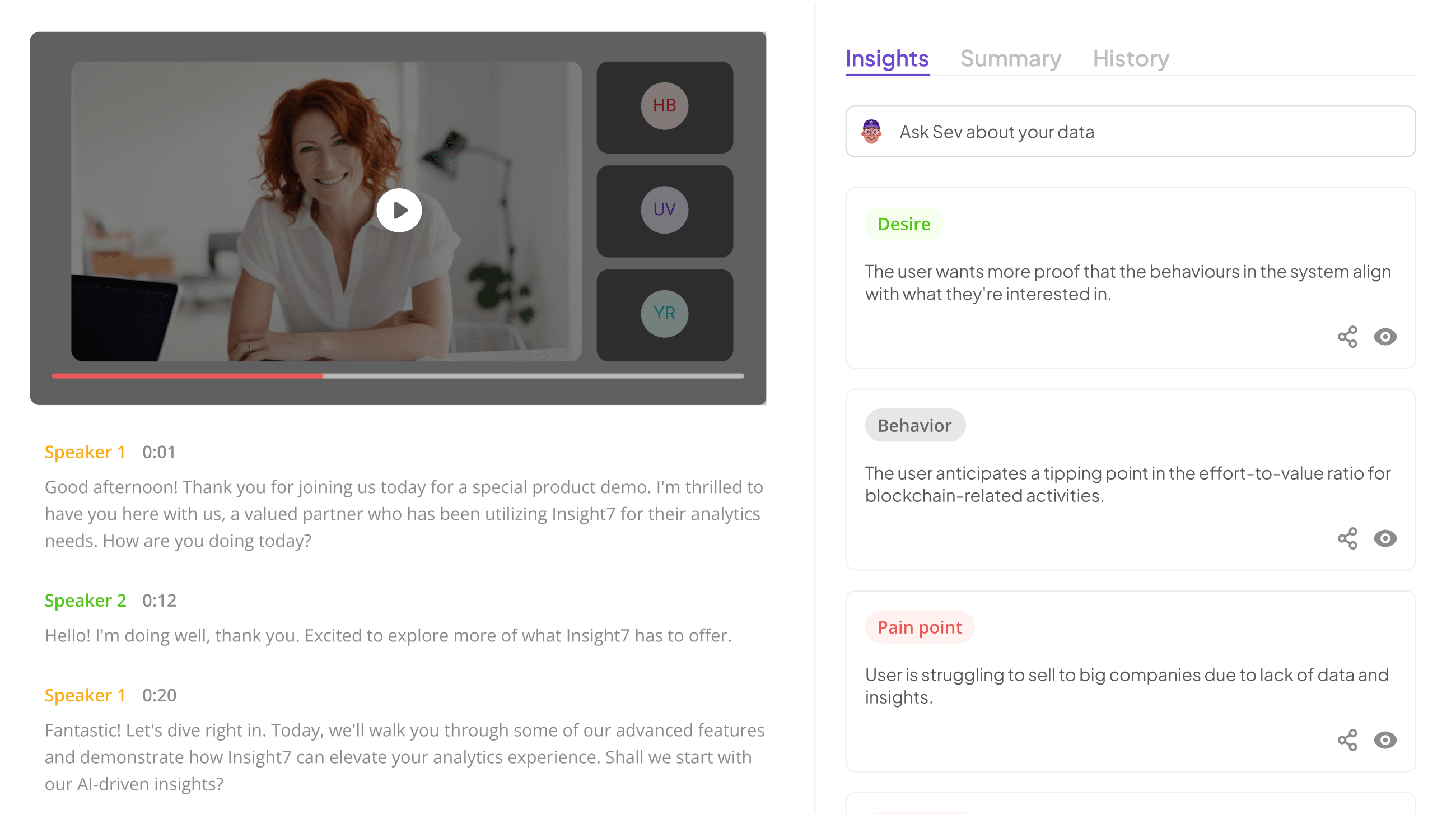
Key AI Features for Enhanced AI QA Efficiency
Key AI features are essential for enhancing AI QA efficiency within quality assurance monitoring. These features streamline processes, reduce manual effort, and provide valuable insights, fundamentally improving overall performance. Automated reporting tools can compile data from various sources promptly, offering real-time insights to QA teams. This capability allows for quicker decision-making and more accurate evaluations.
Additionally, machine learning algorithms can process historical data to predict potential issues, enhancing preventive measures before they become critical. Effective keyword monitoring and data categorization are also crucial as they help to identify trends in performance metrics effortlessly. By employing advanced technologies, teams can efficiently address compliance concerns while ensuring service quality. Altogether, these features contribute significantly to a more effective QA process, ultimately saving time and increasing organizational efficiency.
Real-time Data Processing for Improved AI QA Efficiency
Real-time data processing plays a vital role in enhancing AI QA efficiency. By continuously analyzing data as it comes in, AI systems can swiftly identify trends and anomalies. This capability allows for immediate adjustments and improvements, ensuring quality assurance processes remain robust and responsive. For instance, when a system detects unusual patterns in customer interactions, it can alert operators to potential issues. This proactive approach minimizes delays and enhances overall monitoring effectiveness.
Moreover, real-time processing leads to faster decision-making. Teams no longer need to wait for periodic reports to understand performance metrics. Instead, they can access insights instantly, optimizing workflows and increasing productivity. The seamless integration of real-time data into AI QA processes means that monitoring becomes a dynamic rather than static endeavor. As a result, businesses can enhance their operational efficiency, streamline compliance, and ultimately improve customer satisfaction.
Automated Anomaly Detection for Increased AI QA Efficiency
Automated anomaly detection significantly enhances AI QA efficiency by swiftly identifying deviations from expected behaviors or patterns. This technology analyzes vast datasets in real-time, enabling quality assurance teams to pinpoint issues that may otherwise go unnoticed. By using advanced algorithms, it learns from historical data, continuously improving its understanding of what constitutes normal operations.
The benefits of integrating automated anomaly detection include faster problem identification, reduced manual effort, and improved accuracy in quality monitoring. Teams can focus on resolving critical issues rather than spending time sifting through data. This level of efficiency not only saves time but also assures higher quality outcomes, as detecting anomalies early helps maintain overall system integrity. With AI-driven solutions, organizations can realize transformative improvements in their QA processes, ensuring that products consistently meet quality standards while adapting to changing industry demands.
Extract insights from interviews, calls, surveys and reviews for insights in minutes
Tools Enhancing AI QA Efficiency
Tools play a pivotal role in enhancing AI QA efficiency, allowing organizations to perform quality assurance tasks with greater speed and accuracy. These innovative solutions not only automate mundane processes but also provide insightful analytics that drive improvements. For instance, tools like automated transcription can quickly convert calls into text, making it easier to analyze conversations comprehensively.
Integrating these tools enables teams to shift their focus from manual monitoring to strategic analysis. By using predefined templates, evaluating calls based on established criteria, and generating reports, QA professionals can ensure compliance and quality without excessive time commitment. This automation fosters a continuous feedback loop, encouraging quicker adjustments and ultimately leading to a more efficient quality assurance process. Embracing such tools can profoundly enhance overall QA efficiency, streamlining workflows and improving outcomes.
Insight7: Leading the Way in AI QA Efficiency
In the realm of AI QA efficiency, adopting innovative methodologies can significantly transform how quality assurance processes unfold. The current demands for speed and accuracy necessitate solutions that not only streamline operations but also provide actionable insights. With advanced technologies, organizations can enhance their QA processes, ensuring that the increasing volume of data generated by customer interactions is effectively managed and analyzed.
AI-driven tools offer remarkable capabilities, such as real-time data analysis and automated anomaly detection. These features minimize human error and speed up decision-making processes significantly. By implementing an AI-centric approach, teams can effortlessly translate valuable insights into strategic actions. This efficiency is crucial in staying competitive, as timely responses to customer needs and concerns can make all the difference in the business landscape. Ultimately, enhancing AI QA efficiency is not just about keeping pace; it's about establishing a proactive stance in addressing quality assurance challenges.
Other Remarkable Tools Improving AI QA Efficiency
The realm of AI QA efficiency benefits significantly from various innovative tools designed to streamline the quality assurance process. Notably, platforms such as Qualitest and Applitools stand out for their ability to automate testing and enhance visual assurance. These tools utilize advanced algorithms to identify discrepancies and ensure consistency across applications, drastically reducing manual workloads and time spent on quality checks.
Another commendable option is Testim, which employs AI-driven test creation to build intelligent tests that adapt to changing application environments. Coupled with Mabl, which offers an end-to-end testing solution that learns from application behavior, these tools collectively improve AI QA efficiency by allowing teams to focus on critical tasks rather than repetitive testing. Together, these remarkable tools redefine how quality assurance is approached, ultimately fostering a more efficient and effective QA ecosystem.
- Qualitest
The integration of AI in quality assurance profoundly enhances QA monitoring. One of the standout features is its ability to automate the evaluation process, significantly reducing time spent on manual assessments. Leveraging AI technologies allows organizations to quickly analyze large volumes of data, ensuring that quality metrics are consistently met.
Moreover, AI-driven tools enable real-time monitoring of QA metrics, which facilitates immediate feedback and quick adjustments. This proactive approach leads to more efficient identification of potential issues before they escalate. By employing features like automated anomaly detection, teams can target specific areas that require improvement, thus optimizing QA processes. As a result, AI QA efficiency is not merely about speed, but also about elevating the overall quality assurance strategy. Embracing these AI features ultimately leads to a more effective and reliable QA monitoring workflow.
- Applitools
In the realm of quality assurance, Applitools stands out as a powerful tool enhancing AI QA efficiency. Its visual testing capabilities leverage artificial intelligence to automate the validation of user interfaces, which significantly reduces the time QA teams spend on manual testing. By comparing screenshots to expected outcomes, it identifies visual discrepancies instantly, ensuring the applicationโs appearance remains consistent across various devices and browsers.
Moreover, this tool enables quicker issue identification, allowing teams to address bugs before they escalate. With features such as smart maintenance and robust reporting, it streamlines the testing process, freeing developers to focus on high-priority tasks. Ultimately, integrating Applitools into your QA processes can transform your approach, ensuring speed and accuracy in maintaining quality standards while optimizing resources. In doing so, organizations can achieve higher efficiency in their quality assurance efforts.
- Testim
Testim stands out as a valuable tool in the realm of AI QA efficiency, particularly in enhancing quality assurance monitoring. Its unique features streamline the testing process while reducing manual oversight. With sophisticated automation capabilities, teams can execute tests rapidly, saving both time and resources.
One of the key functionalities is its ability to create and manage test scripts effortlessly. This feature allows users to generate scripts without extensive coding knowledge, enabling more team members to contribute to QA efforts. Additionally, Testim's powerful analytics provide insights into testing outcomes, helping teams make informed decisions quickly. The combination of automated testing and detailed feedback significantly enhances QA efficiency, allowing teams to focus on higher-level tasks that drive product innovation. By incorporating such tools into their workflows, organizations can effectively elevate their quality assurance practices.
- Mabl
Mabl is a powerful tool that enhances AI QA efficiency through its automation capabilities. It focuses on eliminating repetitive tasks often associated with quality assurance. By automating test case generation and execution, Mabl enables teams to free up valuable time while increasing test coverage and consistency.
Moreover, its integration with various platforms improves collaboration among team members. This seamless connectivity allows for real-time feedback and faster iterations, ensuring that issues are resolved without significant downtime. With the ability to run tests across multiple environments simultaneously, Mablโs efficiency in QA monitoring becomes even more pronounced.
Additionally, Mabl utilizes AI to continuously learn from previous test results, ensuring that your QA efforts evolve and improve over time. By adopting this tool, organizations can streamline their QA processes, allowing for quicker releases while maintaining high-quality standards. This not only minimizes risks but also promotes a culture of continuous improvement within the team.
Conclusion: Embracing AI QA Efficiency in Monitoring
Embracing AI QA efficiency in monitoring offers companies an innovative frontier for optimizing their quality assurance processes. By utilizing advanced AI features, businesses can significantly reduce the time needed for comprehensive monitoring and analysis. This shift enables teams to focus on higher-value tasks, fostering a proactive culture centered on continuous improvement.
Moreover, integrating AI technologies leads to more accurate data interpretations, ultimately enhancing decision-making quality. As organizations invest in AI QA efficiency, they pave the way for streamlined operations and better compliance outcomes. Adopting these AI capabilities not only improves efficiency but also elevates the overall quality of services provided.
๐ฌ Need Help? Chat with Support
Our team typically responds within minutes