How to Prepare Call Recordings for AI Analysis
-
Bella Williams
- 10 min read
Call data preparation begins with a vital understanding of your objectives. When dealing with call recordings intended for AI analysis, it’s essential to establish a systematic approach. This process involves gathering and organizing your recordings efficiently to maximize the insights that artificial intelligence can uncover.
Proper call data preparation enhances the ability to analyze conversations effectively. By transcribing recordings and ensuring quality control, you pave the way for actionable insights. Developing a structured framework not only streamlines your analysis efforts but also enriches the quality of data you work with, ultimately leading to better decision-making based on customer interactions.
Analyze & Evaluate Calls. At Scale.
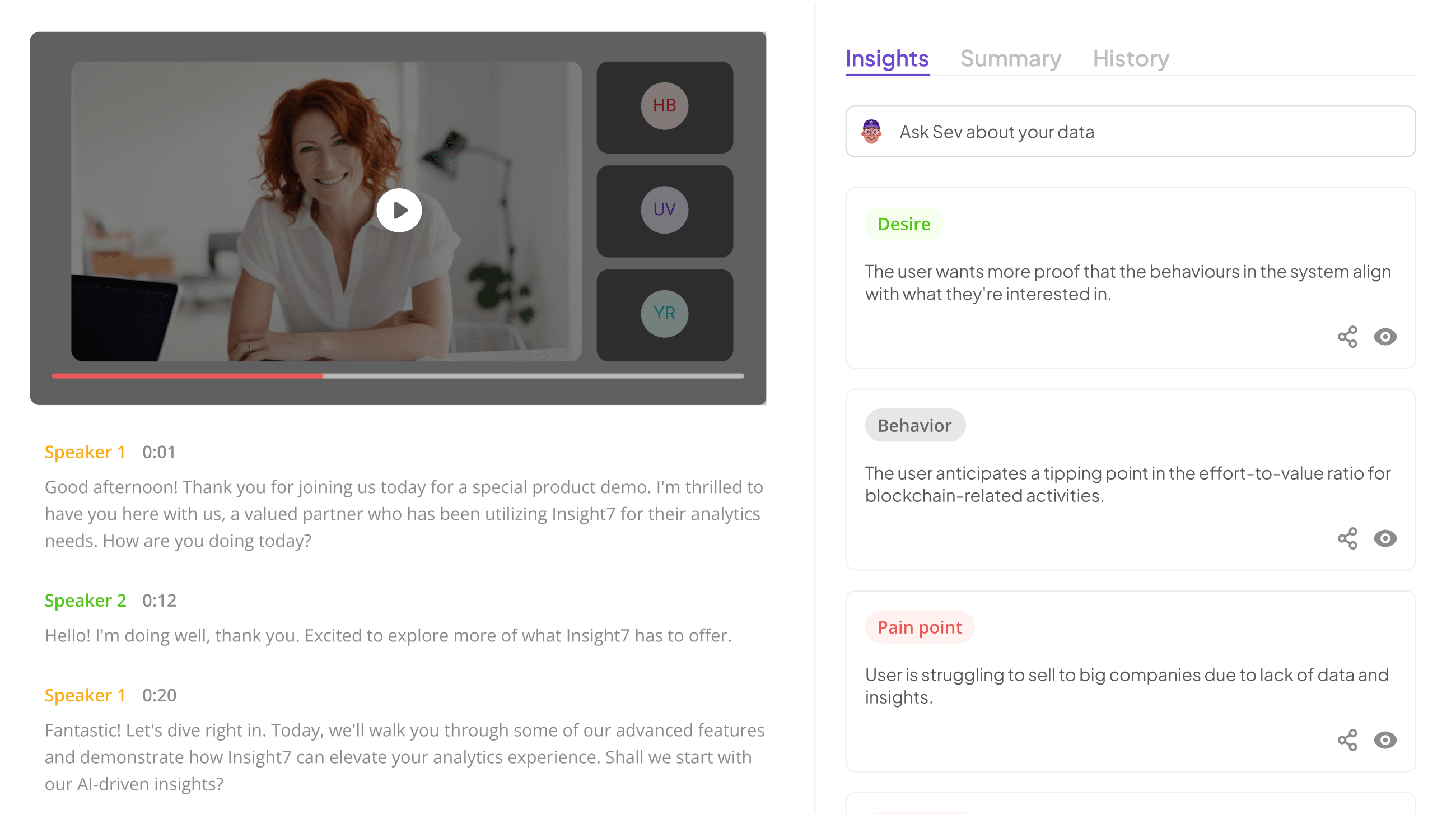
Key Steps in Call Data Preparation
Call Data Preparation is an essential process for harnessing the full potential of call recordings in AI analysis. The first key step involves data collection and storage. Gathering high-quality recordings is critical; poor audio quality can hinder analysis efficacy. Use secure storage solutions to safeguard your data, ensuring compliance with privacy regulations and maintaining organizational integrity.
The next step is data preprocessing for AI analysis. Implement noise reduction techniques to enhance audio clarity, as this improvement allows AI models to function more effectively. Consistency is also crucial, so format the recordings uniformly before processing them. By carefully preparing your call data, you enable AI tools to extract valuable insights more reliably, leading to enhanced customer service training and improved decision-making based on detailed analyses.
Step 1: Data Collection and Storage
In preparing call recordings for AI analysis, the first crucial step involves data collection and storage. Start by gathering high-quality recordings from your sources. Ensure that these recordings are clear and free from substantial background noise, as sound clarity is paramount for accurate analysis. Collect a diverse range of calls to represent various scenarios, topics, and sentiments effectively. This diversity will enhance the AI's ability to understand context and nuances in communication.
Once collected, secure storage solutions are essential for managing this data effectively. Consider using cloud-based systems that offer both security and easy access for your team. Organize the recordings systematically, perhaps by date, type, or other relevant categories. This organization will facilitate quicker retrieval and analysis. By following these steps in data collection and storage, you lay the groundwork for effective call data preparation, helping the AI deliver actionable insights efficiently.
- How to Gather High-Quality Recordings
Gathering high-quality recordings is essential for effective call data preparation, particularly for AI analysis. Start by ensuring that your recording environment is quiet and free from background noise; this will drastically improve audio quality. Using a reliable recording device with good sensitivity is crucial. Whether you choose software or hardware, select options known for their clarity and ease of use.
Next, establish clear guidelines on the types of calls to record. Focus on calls that include significant interactions, whether customer service inquiries or sales conversations. This targeted approach helps capture the most relevant data. Lastly, verify that you have the appropriate permissions to record calls, as compliance with legal standards is paramount. By implementing these strategies, you can gather recordings that provide valuable insights for AI analysis and enhance overall call evaluation.
- Secure Storage Solutions
Secure storage solutions are critical for the safe management of call recordings. These recordings contain sensitive information and must be stored securely to ensure data protection and compliance with regulations. Utilizing cloud-based storage platforms like AWS or Google Cloud allows for high-level security protocols, which protect data from unauthorized access.
Establishing a secure storage system involves encrypting the data both during transmission and while at rest. This protects against potential data breaches. Additionally, implementing access controls ensures that only authorized personnel can retrieve or analyze the call data. Creating regular backups further safeguards your recordings against accidental loss or corruption. Adopting these secure storage solutions not only enhances compliance but also fosters trust among customers by demonstrating a commitment to protecting their information.
Step 2: Data Preprocessing for AI
Data preprocessing for AI involves several crucial steps to ensure that call recordings are ready for analysis. First, it's essential to focus on noise reduction techniques. Background noise can significantly interfere with transcription accuracy and the detection of key insights within the recordings. Utilizing noise-canceling software helps in isolating important audio, thereby enhancing data quality.
Next, standardizing call formats is vital. Different recordings may come in various audio formats, which could lead to inconsistencies during analysis. Transforming all recordings to a uniform format, such as WAV or MP3, ensures seamless integration during processing.
Lastly, organizing and categorizing records based on themes, such as customer pain points or feedback, adds another layer to call data preparation. By implementing these strategies, the data becomes more usable and insightful, enabling the AI model to extract valuable patterns and trends efficiently.
- Noise Reduction Techniques
To effectively prepare call recordings for AI analysis, implementing noise reduction techniques is crucial. First, it's important to understand the common sources of audio noise, including background chatter, static, and echo. These unwanted sounds can obscure key dialogues, making it difficult for AI models to accurately analyze the content. By addressing noise levels, you'll ensure a clearer, more focused dataset that enhances the quality of insights derived from the recordings.
There are several methods to reduce noise. One effective technique involves using digital audio processing software that includes noise-cancellation features. This software can intelligently filter out background noises while preserving essential audio clarity. Additionally, implementing proper microphone techniques, such as positioning the mic closer to the speaker, can drastically minimize ambient noise. Lastly, consider conducting calls in quieter environments whenever possible. By employing these noise reduction techniques, you significantly improve the overall quality of your call data preparation, ultimately aiding AI in delivering more reliable analyses.
- Formatting for Consistency
To achieve effective call data preparation for AI analysis, formatting for consistency is crucial. Consistent formatting helps ensure that the data is organized and easily interpretable during analysis. Start by standardizing file types, ensuring all recordings are in a compatible format for your analysis tools. Likewise, utilize uniform naming conventions to label files clearly, which facilitates easy identification and retrieval.
Additionally, maintaining a structured organization system for your recordings enhances accessibility and efficiency. Group recordings by categories, such as date or participant, allowing for streamlined analysis later. Ensure that metadata for each recording is consistently applied, including timestamps and speaker identification where necessary. This meticulous approach to formatting not only aids AI systems in processing call recordings but also improves the overall reliability of insights gleaned from the data. By investing time in these organizational practices, you'll be better prepared for meaningful AI-driven analyses.
Extract insights from interviews, calls, surveys and reviews for insights in minutes
Essential Tools for Call Data Preparation
To effectively prepare call recordings for AI analysis, selecting the right tools is essential. These tools streamline the process, ensuring that you'll efficiently handle your call data preparation. Start with transcription services like Otter.ai, which convert your recordings into text format, allowing for easier analysis and extraction of insights. High-quality transcription is fundamental to ensuring that the nuances of conversations are captured accurately.
Next, consider using Descript for editing and annotation. This tool allows users to make necessary adjustments to the transcripts, adding contextual notes that can enrich the data’s value. Quality control is another crucial aspect of call data preparation, and platforms like Rev offer services to verify and refine transcripts for maximum reliability. By incorporating these tools, you create a solid foundation for analyzing call data, ultimately enhancing the outcomes of your AI analysis initiatives.
💬 Ask Questions about How to Prepare Call Recordings for AI Analysis?
Our team typically responds within minutes
insight7
To effectively prepare call recordings for AI analysis, understanding the nuances of call data preparation is crucial. First, start by ensuring that your audio recordings are of high quality. Background noise and disruptions can hinder the accuracy of AI insights. Employ noise reduction techniques to enhance audio clarity, enabling smoother analysis.
Next, maintain consistency in formatting across your recordings. This includes standardizing file types and ensuring a uniform structure in metadata. Consistency aids AI in identifying patterns and drawing reliable insights. Additionally, consider implementing a secure storage solution to manage your audio files optimally. Protecting this data is essential, especially when dealing with sensitive customer information. Following these guidelines ensures that your recordings are primed for analysis, ultimately leading to actionable business strategies derived from valuable customer insights.
- Features and Benefits
Call data preparation plays a crucial role in ensuring optimal AI analysis. By transforming raw call recordings into structured data, businesses can unlock valuable insights that drive strategic decisions. This process involves identifying key attributes, categorizing call topics, and ensuring data accuracy. Accurate preparation helps to create a solid foundation for effective analysis, which in turn enhances the understanding of customer interactions.
The benefits of meticulously preparing call data extend beyond immediate insights. It improves training and mentoring opportunities for teams, fostering a culture of continuous improvement. Well-prepared data also enhances data privacy compliance, ensuring adherence to regulations while promoting trust with customers. Ultimately, a thorough approach to call data preparation empowers businesses to shift from mere observation to meaningful engagement, enabling them to adapt swiftly to market demands and customer needs.
Otter.ai
Otter.ai serves as a robust tool for streamlining the process of preparing call data for AI analysis. Through its transcription services, users can easily convert audio recordings into text, making the data more accessible for insights extraction. This step is crucial, as well-structured transcripts enhance the effectiveness of AI algorithms. The tool accommodates various recording formats, ensuring that quality is maintained across diverse applications.
Moreover, the platform integrates features that allow for quick edits and keyword highlighting, which further aids in identifying essential themes within conversations. By organizing the transcripts efficiently, teams can collaborate better and expedite their workflow. Thus, maintaining a systematic approach to call data preparation with supportive tools like this can lead to more insightful analysis and informed business strategies. Effective call data preparation ultimately empowers organizations to harness customer insights and drive actionable results.
- Transcription Services
To effectively utilize call data for AI analysis, transcription services play a crucial role. Accurate transcription of call recordings transforms spoken language into written text, enabling deeper analysis. Transcriptions facilitate the identification of trends, patterns, and key insights from conversations that might otherwise be overlooked in audio format.
When preparing call recordings for AI analysis, the transcription process can be streamlined and simplified. Ideally, a reliable service will allow users to handle multiple files in bulk, ensuring efficiency. Users can easily upload their recordings, receive transcripts, and move on to data analysis without delay. This process not only saves time but also enhances the accuracy of insights derived from the analysis, thus maximizing the potential of call data preparation. By ensuring high-quality transcripts, businesses can better understand customer sentiment and improve decision-making.
Descript
Descript is an innovative tool that streamlines the process of call data preparation, enhancing how businesses analyze audio recordings. With its user-friendly interface, everyone in an organization can easily access its features, making it particularly appealing for teams looking to democratize insights derived from call data. Users can efficiently upload recording files, transcribe conversations, and visually navigate through the insights with minimal training.
The platform enables users to extract critical information such as pain points, customer desires, and overall sentiments from calls. By organizing these findings into insightful cards, Descript simplifies the analysis process, ensuring vital information is readily available for review and action. This capability fosters a robust understanding of the customer experience and reveals friction areas that can be addressed. Overall, Descript is instrumental for organizations aiming for effective call data preparation and actionable insights.
- Editing and Annotation
Editing and annotation play a vital role in the call data preparation process for AI analysis. First, it's essential to eliminate any irrelevant portions of the recording to ensure the dataset's quality and focus. By removing unwanted segments, you can streamline the data, making it easier for AI algorithms to analyze crucial content effectively.
Next, adding annotations enhances comprehension and context. Annotations may include labeling key phrases, identifying speakers, or summarizing sections of dialogue. This step not only enriches the dataset but also allows AI models to learn patterns and gain insights more accurately. Moreover, consistent formatting in text annotations guarantees readability and eases data processing later in the analysis phase. Implementing these strategies enhances the overall efficiency and effectiveness of your call recordings for AI analysis.
Rev
Rev plays a crucial role in ensuring high-quality call data preparation for AI analysis. In this context, quality control processes serve as the backbone for transforming raw recordings into reliable datasets. By implementing consistent quality checks, organizations can eliminate inaccuracies and enhance the overall reliability of their call data.
To optimize the quality control processes, several key practices can be adopted. First, establish a review system where transcriptions and recordings are regularly evaluated against predetermined accuracy benchmarks. Second, invest in advanced audio enhancement tools to improve sound quality, thereby reducing background noise and ensuring clear dialogue. Lastly, solicit feedback from agents who engage with the recordings for coaching. This input is invaluable for identifying patterns that may need addressing. These steps not only streamline the call data preparation process but also significantly improve the effectiveness of AI analysis, ultimately leading to better insights and decision-making.
- Quality Control Processes
Quality control processes are crucial for ensuring that call recordings are reliable and suitable for AI analysis. To begin with, establishing a rigorous framework for evaluating call data is essential. This involves identifying the key metrics and benchmarks, such as compliance accuracy and customer satisfaction scores, that the AI will analyze. By setting these criteria, organizations can facilitate effective assessment and uphold data integrity throughout the preparation phase.
The next step is implementing frequent reviews and audits of recorded calls. These reviews help in detecting anomalies and allow for timely adjustments to the quality assurance procedures. Additionally, using a structured template during evaluations ensures thoroughness and consistency. Each recorded call should be compared against predetermined standards, such as response completeness and issue resolution effectiveness. Consistently applying these quality control processes will enhance the overall accuracy of call data preparation for AI analysis, ultimately leading to more accurate insights and decision-making.
Conclusion: Finalizing Your Call Data Preparation for AI Analysis
Finalizing your call data preparation sets the foundation for effective AI analysis. Begin by reviewing the organization of your call recordings, ensuring that they are categorized and accessible based on your analysis goals. Create meaningful projects that reflect timeframes or themes for a clearer examination of trends and insights, as discussed earlier.
Next, implement systematic preprocessing methods to enhance data quality. Techniques such as noise reduction and consistent formatting are vital for optimizing AI performance. The insights gained from this structured approach will be invaluable, guiding decision-making and revealing patterns that drive business growth.
💬 Ask Questions about How to Prepare Call Recordings for AI Analysis?
Our team typically responds within minutes