Introduction to AI QA Automation is reshaping how businesses handle feedback processes. In an era where swift and accurate evaluations are vital, AI QA Automation streamlines quality assurance by offering real-time insights that significantly enhance decision-making. This technology not only minimizes manual effort but also delivers precise scoring, allowing teams to identify areas for improvement swiftly.
By automating QA feedback, organizations can foster a culture of continuous improvement. With AI monitoring and scoring performance, quality assurance becomes proactive rather than reactive. This transformation enables teams to focus on strategic initiatives while AI effortlessly maintains high standards in their feedback processes. As industries embrace this innovation, the potential for improved product quality and customer satisfaction continues to rise.
Analyze & Evaluate Calls. At Scale.
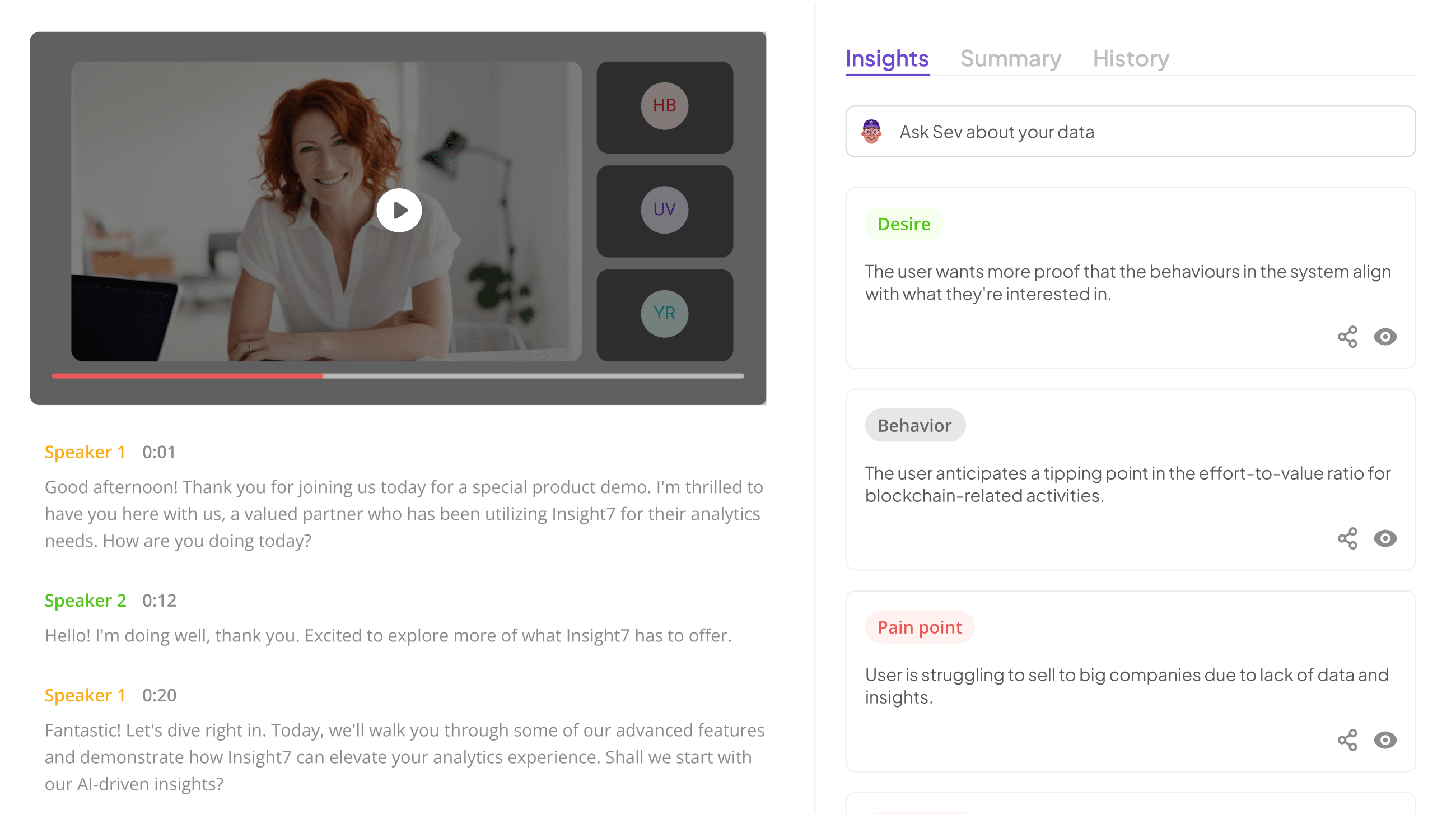
Understanding AI-Based Scoring in QA Automation
AI-based scoring in QA automation introduces a transformative approach to evaluating and enhancing the quality assurance process. Central to this concept is the use of advanced algorithms that analyze various performance metrics to identify strengths and weaknesses in teams or systems. By leveraging these capabilities, organizations can automate the scoring of agents or products and generate insightful reports quickly and accurately.
This scoring mechanism taps into vast amounts of data, allowing it to provide real-time feedback. Users can access evaluations that gauge performance against established benchmarks, facilitating swift improvements. Additionally, AI scoring fosters a more objective assessment environment, mitigating human biases and contributing to more consistent evaluations. Overall, understanding AI-based scoring is crucial for leveraging its full potential in AI QA automation, streamlining feedback processes, and fostering continuous improvement.
The Role of AI in Modern QA
Artificial Intelligence is transforming Quality Assurance (QA) processes in profound ways. Emphasizing AI QA Automation allows organizations to improve efficiencies and accuracy in evaluating feedback. By utilizing sophisticated algorithms, companies can automate the scoring of QA interactions, ensuring that assessments are consistent and objective. This shift not only reduces manual workloads but also enhances the reliability of insights gathered.
Moreover, AI enables real-time analysis of data, facilitating quicker feedback loops and agile responses to quality concerns. The system can monitor various metrics, identifying trends and patterns that might be overlooked by human evaluators. As AI continues to evolve, its role in modern QA will only expand, positioning it as a crucial element in maintaining high standards and customer satisfaction. By embracing AI QA Automation, organizations can anticipate challenges and streamline processes for improved outcomes.
How AI QA Automation Enhances Feedback Quality
AI QA Automation plays a vital role in enhancing the quality of feedback generated during quality assurance processes. With traditional methods often leading to inconsistencies, the automation provided by AI introduces a level of accuracy and efficiency that significantly improves feedback quality. This technological advancement allows organizations to streamline feedback collection by assessing agents based on standardized scoring metrics. Consequently, specific strengths and weaknesses can be identified more clearly.
Moreover, the insights generated through AI QA Automation can be tailored to meet the needs of different team members. Organizations can quickly analyze performance and engagement metrics, resulting in immediate action points for improvement. By utilizing AI-driven tools, feedback becomes more actionable and reliable, ultimately fostering a culture of continuous improvement. This capability not only optimizes performance but also enhances accountability within teams, leading to higher customer satisfaction and better overall service quality.
Extract insights from interviews, calls, surveys and reviews for insights in minutes
Implementing AI QA Automation: Steps to Success
Implementing AI QA Automation can significantly enhance the efficiency and reliability of your quality assurance processes. To embark on this journey successfully, it's essential to follow a structured approach. The first step is identifying key performance metrics relevant to your QA goals. Knowing what to measure helps specify what success looks like and informs your overall strategy.
Next, selecting the right AI tools for scoring is crucial. Popular tools like TensorFlow, IBM Watson, and Selenium can streamline your automation efforts. Each of these tools brings unique capabilities to address various QA requirements. After selecting the tools, ensure they integrate smoothly with your current QA systems. A seamless integration facilitates better data flow and enhances the scoring accuracy, leading to more reliable outcomes. By adhering to these steps, you can effectively implement AI QA Automation and achieve lasting success in your feedback processes.
Step 1: Identifying Key Performance Metrics
To effectively automate QA feedback using AI, the first step is identifying key performance metrics. These metrics act as the foundation for evaluating performance and ensuring that feedback remains actionable. Itโs vital to establish both quantitative and qualitative metrics, as they together provide a comprehensive view of quality assurance effectiveness.
Quantitative metrics might include the number of calls evaluated, response accuracy rates, and time taken for feedback cycles. Qualitative metrics, on the other hand, could encompass customer satisfaction ratings and insights derived from user interactions. Regularly reviewing these metrics will also allow teams to attune their approaches based on historical trends and performance data, enhancing the overall impact of the AI QA automation process. By precisely tracking these indicators, organizations can refine their feedback mechanisms, ensuring AI-based scoring continues to drive improved outcomes in quality assurance.
Step 2: Selecting the Right AI Tools for Scoring
Choosing the right AI tools for scoring is a crucial step in the process of automating QA feedback. To ensure successful implementation, it's essential to evaluate various AI tools based on specific needs and goals. Begin by assessing the unique requirements of your QA processes. Consider factors such as the types of scripts used, compliance standards, and scoring methods tailored to your clients. Each choice should align closely with your objectives for quality assurance.
Next, investigate features of potential AI solutions, such as their ability to handle customized scorecards and adaptability to varying compliance needs. Look for tools that not only provide accurate scoring but also offer insightful analytics to enhance decision-making. Additionally, consider ease of integration with existing systems, as this can greatly affect the efficiency of your automation processes. Selecting the right AI tools for scoring will ultimately streamline your QA efforts and improve overall feedback quality.
insight7: The Leading AI Tool for QA Automation
In the realm of QA automation, AI tools have emerged as game-changers, enhancing the efficiency and accuracy of feedback processes. Insight7 distinguishes itself as a pioneering platform designed to streamline the analysis of customer interactions and feedback. By automating the scoring mechanism, this tool enables organizations to interpret vast amounts of data rapidly, ensuring quicker insights that drive strategic decision-making.
The intuitiveness of Insight7 makes it accessible for users across diverse industries. It simplifies the complexity of analyzing customer conversations, making it easy to extract meaningful insights. As organizations increasingly rely on customer feedback to improve their products and services, having a leading AI tool for QA automation is not just beneficialโit's essential. With Insight7, companies can ensure that they remain competitive while fostering a culture of continuous improvement based on real customer data.
TensorFlow: Leveraging Machine Learning for Quality Assurance
TensorFlow stands out as a powerful tool for automating quality assurance through machine learning. By utilizing TensorFlow, organizations can analyze vast amounts of data efficiently, leading to improved quality and consistency in their products. This framework allows for the development of models that evaluate and score various aspects of quality assurance, ultimately streamlining the feedback process.
To successfully implement AI QA automation using TensorFlow, it's essential to follow a structured approach. First, identify the key performance indicators that matter most to your QA objectives. Next, leverage TensorFlow's capabilities to create and train models that assess these indicators effectively. Finally, integrate these models with your existing QA systems to ensure seamless feedback loops. This holistic approach transforms traditional QA processes, making them more data-driven and responsive to quality issues, thus enhancing overall product reliability and customer satisfaction.
IBM Watson: AI-Powered Insights for QA Processes
AI-powered insights serve as a cornerstone for enhancing QA processes in any organization. Through intelligent data analysis and machine learning, businesses gain the ability to identify patterns and issues that might be overlooked. Real-time feedback enables teams to act swiftly, allowing for continuous improvement in product quality and customer satisfaction. With this technological advancement, any member of the team can engage with data easily, facilitating a culture of insight-driven decision-making.
Moreover, the platform provides access to a diverse library of interactions, allowing users to revisit valuable conversations for future analysis. This feature enhances the understanding of customer pain points and preferences, enabling personnel to address issues proactively. By automating the insights generation, organizations can focus more on strategic initiatives rather than manual data collection, ultimately streamlining QA processes and improving overall performance.
Selenium: Automating Feedback Loop in QA
Selenium plays a critical role in automating the feedback loop in QA processes, significantly boosting efficiency. By harnessing Selenium's capabilities, teams can streamline automated testing while collecting actionable data. This method fundamentally transforms how quality assurance operates, allowing for faster response times to identified issues.
To effectively implement Selenium in your AI QA Automation workflow, focus on the following steps:
Setup Selenium WebDriver: Installing the WebDriver is essential for initiating automated tests on web applications. This step ensures an effective interaction between your tests and the browser.
Create Test Scripts: Develop reusable scripts that validate various functionalities of your application. Well-structured test cases lead to consistent performance evaluations.
Integrate AI Scoring Mechanisms: Incorporate AI-based scoring to assess the quality of test outcomes. This enables the identification of patterns and insights that might otherwise go unnoticed.
Schedule Regular Test Executions: Automate the running of these tests at defined intervals, facilitating continuous monitoring of software quality and performance.
By following these steps, Selenium can significantly enhance your feedback loop, making your QA process more effective and reliable.
Appium: Integrating AI Scoring in Mobile Testing
Appium serves as a pivotal framework for integrating AI scoring in mobile testing, enhancing the efficacy of QA processes. By leveraging Appium's capabilities, teams can automate the testing of mobile applications while simultaneously employing AI to provide nuanced scoring based on performance metrics. This integration allows testers to assess user experiences, identifying areas of improvement through automated, intelligent feedback mechanisms.
Implementing AI scoring with Appium involves a few essential steps. First, teams must establish key performance indicators that reflect user expectations. Next, they integrate AI algorithms that evaluate the application against these benchmarks in real-time. Finally, the AI-driven insights generated by Appium facilitate targeted enhancements and streamlined workflows, improving the overall quality of mobile applications. By considering these steps, organizations can achieve significant advancements in mobile testing through AI QA Automation.
Step 3: Integrating AI Tools with Existing QA Systems
Integrating AI tools with existing quality assurance systems is crucial for improving the efficiency of QA processes. Companies often struggle to implement AI QA automation seamlessly within their established frameworks. First, assess your current QA infrastructure to understand the compatibility with prospective AI tools. Identify areas where AI can enhance workflow without disrupting ongoing operations.
Next, focus on integrating AI scoring systems that complement existing templates and evaluation criteria. This may involve customizing these systems to ensure that they align with your specific quality metrics and feedback processes. Providing proper training for your QA team is also essential, as it will enable them to utilize AI tools effectively and interpret the results accurately. With a thoughtful approach, integrating AI tools can result in improved accuracy and faster feedback loops, ultimately leading to a more robust QA process.
Conclusion: Transforming QA Feedback with AI QA Automation
The implementation of AI QA Automation represents a significant shift in how quality assurance feedback is processed and utilized. By harnessing artificial intelligence, businesses can revolutionize feedback mechanisms, leading to more accurate evaluations and insightful reports. This transformation not only streamlines the assessment process but also enhances the quality and reliability of feedback delivered to stakeholders.
Moreover, AI QA Automation grants organizations the ability to quickly analyze performance metrics across various interactions. By automating the feedback loop, teams can focus more on strategic improvements rather than manual data entry. As a result, the agility and responsiveness of quality assurance efforts are greatly enhanced, driving continuous improvement in overall performance.