Predictive Call Analytics lays the foundation for effective call management strategies by harnessing the power of historical data. This approach allows organizations to analyze past call volumes and quality assurance metrics, which reveal trends and potential future demand. By examining patterns from previous customer interactions, teams can enhance operational efficiency and improve customer satisfaction.
Understanding how to use historical QA data is key in this context. It involves carefully collecting and analyzing relevant data points to identify trends and abnormalities. This enables proactive decision-making and enhances resource allocation, ultimately leading to a more efficient call center operation that meets customers' needs effectively.
Analyze & Evaluate Calls. At Scale.
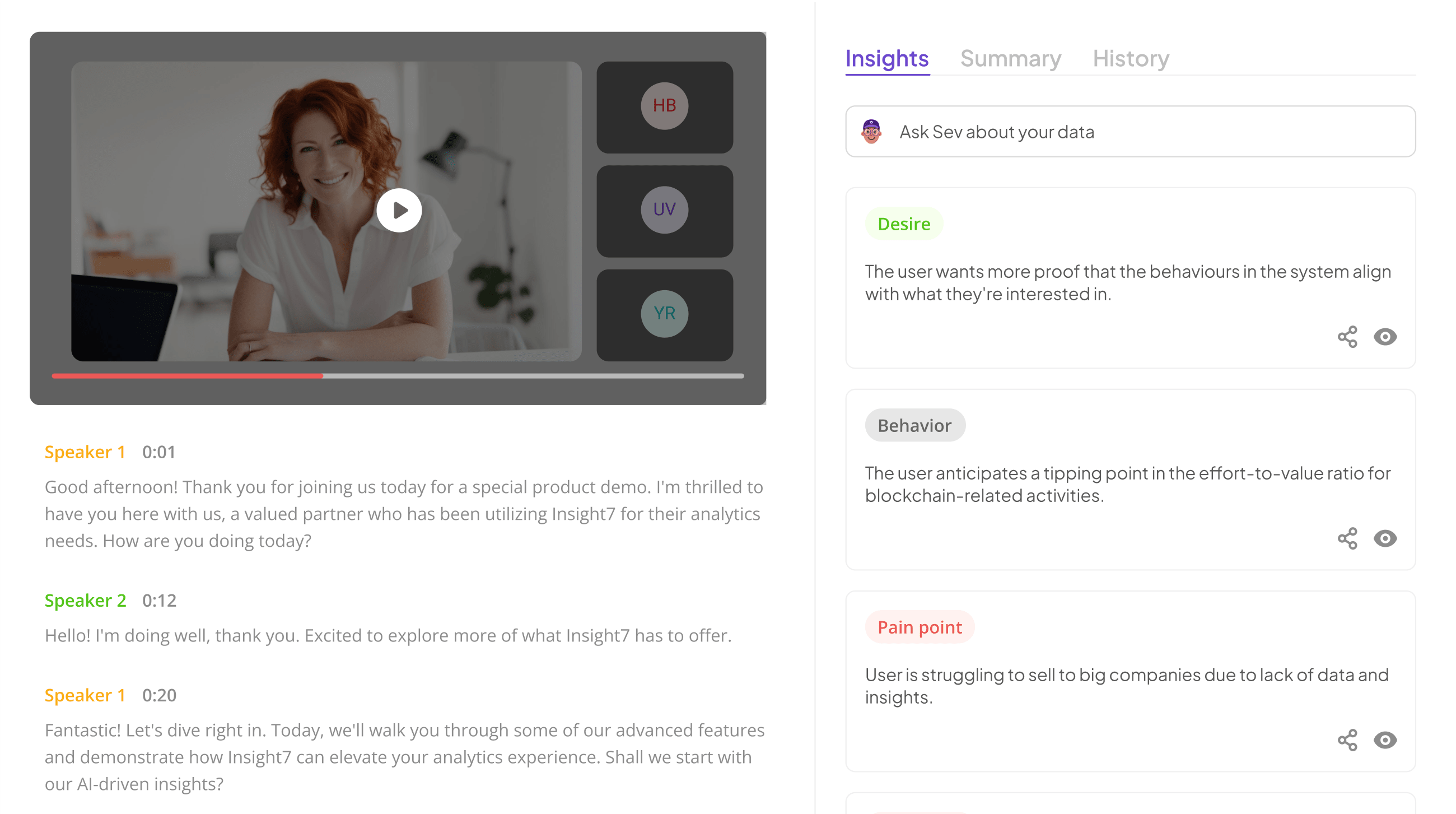
Understanding Predictive Call Analytics in Customer Service
Predictive Call Analytics plays a pivotal role in enhancing customer service operations. By analyzing historical quality assurance (QA) data, businesses can identify trends that help forecast future call volumes. This predictive capability enables organizations to allocate resources effectively, ensuring that customer service representatives are available during peak times. Understanding these patterns is essential for optimizing team efficiency and improving customer satisfaction.
To leverage predictive call analytics effectively, organizations should focus on two key aspects. First, they must employ data collection methods that capture relevant metrics, such as call volume, duration, and customer feedback. Second, analyzing this data for significant trends can uncover seasonal fluctuations and specific events that trigger increased call activity. By synthesizing these insights, businesses can better anticipate customer needs and respond proactively, ultimately enhancing the overall service experience.
The Importance of Historical QA Data
Historical QA data stands as a pillar for understanding past interactions and their influence on future call volumes. This data provides essential insights that can guide businesses in shaping their strategies. By analyzing what has occurred in the past, organizations can harness predictive call analytics to forecast future customer behavior more accurately.
The importance of this historical data can be further explored through two critical functions: identifying trends and enhancing customer experience. First, by examining previous calls, businesses can identify recurring patterns concerning peak call times, types of inquiries, and customer satisfaction levels. This information is invaluable for staffing and resource allocation. Second, understanding past customer interactions allows organizations to tailor their services based on what has historically resonated with their audience. Therefore, recognizing the importance of historical QA data not only aids in developing accurate projections but also fosters an environment for improved customer satisfaction and loyalty.
- Data Collection Methods
To effectively use historical QA data in predictive call analytics, data collection methods play a crucial role. Various techniques must be employed to gather relevant information, ensuring it is reliable and comprehensive. The primary methods usually include surveys, call recordings, and customer feedback forms. These tools allow organizations to collect quantitative and qualitative data, which reveals insights into customer behavior and preferences.
Once the data is collected, the next step is to organize and analyze it appropriately. Data cleaning is essential to remove any inaccuracies that may skew the results. By categorizing the data based on factors such as call resolution time and customer satisfaction rates, analysts can identify patterns and trends. This foundational work is vital for developing predictive models that forecast future call volumes effectively. By understanding the types of data collection methods available, organizations can enhance their predictive analytics processes and ultimately improve customer service outcomes.
- Key Metrics to Analyze
Understanding which key metrics to analyze is essential for effectively using historical QA data. These metrics provide insights into call patterns, representative performance, and customer interactions. By closely examining data points such as average handle time, first contact resolution rate, and call volume trends, organizations can gain a clearer picture of their operational efficiency.
Additionally, it is crucial to evaluate qualitative metrics like customer satisfaction scores and representative feedback. These qualitative insights can reveal areas where training may be beneficial or highlight changing customer needs. By focusing on both quantitative and qualitative data, predictive call analytics can drive improvements. Tracking these key metrics over time enables organizations to better anticipate future call volumes and optimize their resources accordingly.
By implementing a structured approach to data analysis, organizations can refine their strategies and prepare for evolving customer demands. Continuous monitoring of these key metrics ensures that teams remain proactive and responsive to trends.
Extract insights from interviews, calls, surveys
and reviews for insights in minutes
Steps to Forecast Call Volumes Using Historical Data
Extract insights from interviews, calls, surveys and reviews for insights in minutes
Extract insights from interviews, calls, surveys and reviews for insights in minutes
Step 1: Preparing Your Data
In Step 1 of utilizing historical QA data for predictive call analytics, the primary focus is on preparing your data effectively. Begin by cleaning and organizing your dataset, which is crucial for accurate analysis. Removing irrelevant information and correcting inconsistencies ensures that your data reflects true patterns and trends. This step can significantly enhance the quality of insights derived during later analysis.
Next, it's important to identify relevant patterns within the cleaned data. Look for recurring themes in call volume, customer issues, and agent performance metrics. Understanding these patterns will not only guide your predictive model but will also illustrate how different factors impact overall call volumes. As you prepare your data, keep in mind that a well-structured data set positions you to make confident predictions about future call volumes, ultimately improving your call center's efficiency.
- Cleaning and Organizing
When it comes to predictive call analytics, cleaning and organizing your data is crucial for meaningful insights. Start by collecting historical QA data from all relevant sources, ensuring consistency across various formats. This could involve standardizing call categories, agent performance metrics, and customer feedback ratings. Proper organization will help you easily identify trends and patterns, enabling a more accurate forecast of future call volumes.
Next, segment your data based on different locations or markets. This granularity allows you to tailor your analysis according to specific dynamics influencing each area. For example, training programs may need to be adjusted based on regional product preferences. By creating a structured approach to your data collection and organization, you can more effectively utilize predictive analytics. This foundational step sets the stage for advanced analysis, where you can unveil deeper insights and make data-driven decisions that optimize call center performance.
- Identifying Relevant Patterns
Identifying relevant patterns in historical QA data is crucial for predicting future call volumes. First, understanding the data you have enables you to spotlight significant trends. By organizing calls chronologically, you can pinpoint which periods experienced high volume and what factors contributed to those spikes.
Next, consider specific metrics such as customer pain points or common inquiries. Analyzing these can reveal recurring themes that often coincide with higher call volumes. For example, if many calls during a particular month discuss product issues, it may indicate a problem that needs immediate attention. These insights can guide future resource allocation and training, ultimately leading to improved customer service.
Lastly, developing visual representations of your findings, like charts or dashboards, can foster clearer communication across teams. By implementing a systematic approach to identifying patterns, organizations can enhance their predictive call analytics, ensuring they remain responsive to customer needs.
Step 2: Analyzing Trends and Patterns
Analyzing trends and patterns is crucial for harnessing the power of historical data in predicting future call volumes. Begin with the collection of relevant data points, which may include call frequency, duration, and customer feedback. By dissecting this data, you can identify recurring themes, such as seasonal fluctuations or spikes related to specific events. This approach allows you to establish a clearer understanding of the factors that influence call volumes.
To dive deeper, apply statistical methods to your data set. For instance, consider using moving averages or regression analysis to spot trends over time. Additionally, pay attention to seasonality, as it often plays a significant role in determining call volume. Remember, the insights gained from these analyses are not merely numbers; they present opportunities for improved customer service and resource allocation. Embracing these findings will enhance your predictive call analytics strategy and enable more accurate forecasting.
- Utilizing Statistical Methods
Understanding statistical methods is essential for effectively utilizing historical QA data to predict future call volumes. By leveraging these techniques, teams can identify patterns that inform decision-making processes. Statistical methods help in discerning correlations and developing predictive models that can forecast call trends.
One effective approach is regression analysis, which evaluates the relationship between various variables correlated with call volumes, such as time of day or seasonal factors. Additionally, time-series analysis is beneficial for recognizing trends over specific periods, allowing for better anticipation of call spikes. It is crucial to ensure that the data is cleaned and organized before applying these methods to enhance accuracy. Employing these statistical techniques lays a strong foundation for predictive call analytics, ultimately driving better operational strategies in customer service settings.
- The Role of Seasonality
Seasonality plays a significant role in Predictive Call Analytics by influencing call volumes throughout the year. Understanding seasonal trends can help organizations anticipate fluctuations in demand, thereby optimizing resource allocation and staffing. For example, businesses often experience spikes in call volume during particular seasons, such as tax time or holiday shopping periods. These variations can be traced through historical QA data, allowing for informed decision-making in scheduling and training.
Moreover, analyzing seasonal patterns alongside historical data can reveal unique insights into customer behavior. Identifying these trends not only improves operational efficiency but also enhances customer satisfaction by ensuring that agents are readily available during peak times. By integrating seasonality into predictive analytics, organizations can develop a robust framework to forecast call volumes accurately and adjust their strategies accordingly. Understanding these seasonal dynamics empowers businesses to respond proactively to changing customer needs.
Step 3: Building a Predictive Model
Building a predictive model is a crucial step in harnessing historical QA data to forecast future call volumes accurately. First, it's essential to identify the right predictive analytics model that suits your specific needs and objectives. Various models, from linear regression to machine learning algorithms, can be used to achieve better insights into future call trends. Selecting the most appropriate one will depend on the complexity of your data and your forecasting goals.
Next, testing and validation play pivotal roles in ensuring the reliability of your model. It is vital to run simulations with historical data to evaluate how well the model performs in predicting actual call volumes. Regularly updating the model with new data and insights enhances its precision over time. By building a robust predictive model, organizations can streamline operations, manage resources more effectively, and ultimately improve customer service outcomes through predictive call analytics.
- Choosing the Right Model
To choose the right model in predictive call analytics, you must consider the nature of your data and what you aim to achieve. Start by assessing your historical QA data, which offers valuable insights into call volume patterns. Understanding your goals is crucial because different models cater to varying objectives, such as short-term predictions or long-term trends.
Next, identify the algorithms best suited for your dataset. Commonly used models include regression analysis, time series forecasting, and machine learning techniques. Each of these methods has strengths and weaknesses, making it essential to test several options. Ultimately, finding the right model involves evaluating its performance against your historical data and validating its predictions through ongoing tests. This structured approach allows you to enhance your predictive capabilities over time, ensuring your call center is well-prepared for the future.
- Testing and Validation
Testing and validation are crucial steps in the application of predictive call analytics. This process ensures that the models built using historical QA data are accurate and reliable for forecasting future call volumes. Initially, it involves comparing the predictions generated by your models against actual call data to assess their performance. Ensuring accuracy in your analytics can significantly enhance decision-making, leading to more effective resource allocation and improved customer experiences.
Once the model is developed, thorough testing is necessary to evaluate its effectiveness. This includes running simulations using historical call data, adjusting parameters, and monitoring outcomes. Validation checks whether the model maintains accuracy over time, particularly as call patterns evolve. By continually fine-tuning your predictive call analytics approach and validating results, you position your organization to anticipate customer demand more effectively and prepare accordingly.
Top Predictive Call Analytics Tools
Predictive call analytics tools play a vital role in refining customer service strategies by utilizing historical data. These tools can analyze past interactions in order to anticipate future call volumes and trends effectively. By understanding patterns in call data, organizations can ensure optimal staffing and resource allocation, improving overall customer experience.
Several key tools stand out in the space of predictive call analytics. First, Insight7 provides an intuitive platform that allows users to transcribe and analyze calls at scale, extracting actionable insights with ease. It simplifies bulk analysis and offers templates for specific objectives like customer discovery.
Additionally, platforms like ChilliePiper facilitate efficient scheduling while Aircall boasts seamless integrations for better communication. Talkdesk enhances support with AI-driven insights, while Freshdesk offers robust ticketing solutions. Utilizing these predictive call analytics tools helps organizations stay ahead in managing call volumes and improving service delivery.
insight7
Effective use of historical QA data enables organizations to forecast future call volumes more accurately. Insight7 highlights the importance of understanding customer interactions and feedback trends. By analyzing this data, businesses can uncover actionable insights that inform staffing decisions and operational strategies. Customers' needs and preferences change over time, and utilizing predictive call analytics allows companies to remain agile in their responses.
To implement these insights effectively, consider the following key aspects:
- Identify Patterns: Look for recurring themes in customer inquiries and complaints. Understanding these trends helps in anticipating future call fluctuations.
- Data-Driven Decisions: Use historical data to make informed predictions rather than relying on gut feeling. This approach fosters a proactive customer service strategy.
- Monitor Results: Continuously assess the accuracy of your predictions against actual call volumes. Adjust your model based on this feedback to enhance future forecasting.
In summary, integrating historical QA data with predictive call analytics can dramatically improve how businesses forecast call volumes and optimize customer service operations.
- Features and Benefits
Leveraging historical QA data brings several features and benefits that can significantly enhance predictive call analytics. First, the ability to analyze past performance enables accurate forecasting of future call volumes, helping organizations prepare better for demand surges. By identifying trends in customer interactions, companies can allocate resources effectively, ensuring sufficient staffing during peak periods.
Furthermore, historical QA data allows for more personalized customer service. By understanding the common questions and concerns raised in past calls, agents can be equipped with insights that lead to improved customer interactions. This proactive approach not only enhances customer satisfaction but also contributes to a more engaged workforce.
In summary, utilizing historical QA data for predictive call analytics provides organizations with tools to optimize operations and elevate customer experiences. It enables informed decision-making, creating a win-win scenario for both businesses and their clients.
Competitive Tools
In todayโs competitive landscape, organizations must utilize effective tools to anticipate future call volumes. Competitive tools like ChilliePiper, Aircall, Talkdesk, and Freshdesk stand out in the realm of Predictive Call Analytics. Each tool offers distinct features that help businesses harness historical QA data, transforming it into actionable insights for future planning.
ChilliePiper specializes in optimizing scheduling and automated responses, ensuring that customer inquiries are met quickly. Aircall integrates seamlessly with various workflows, providing real-time analytics and reporting. Talkdesk excels in its robust AI capabilities, offering advanced features for data analysis, while Freshdesk focuses on enhancing customer experience through its intuitive interface and support capabilities. By employing these resources, organizations can not only respond rapidly to market changes but also fulfill customer needs more effectively. Ultimately, selecting the right combination of tools is crucial for leveraging historical data to shape future strategies.
- ChilliePiper
ChilliePiper plays a significant role in the evolving landscape of predictive call analytics. By utilizing historical QA data, it enables businesses to understand better how past call patterns may influence future expectations. Analyzing this data allows organizations to identify trends and develop efficient strategies for resource allocation. The integration of advanced analytics helps streamline processes, ultimately enhancing customer satisfaction and operational efficiency.
For companies aiming to optimize their call volumes, leveraging predictive call analytics is crucial. Through careful examination of historical metrics, organizations can anticipate call surges during peak periods or anticipate downturns during quieter times. Understanding these fluctuations empowers teams to adjust staffing levels accordingly, ensuring that customer service remains responsive while minimizing costs. By applying these techniques, businesses can transform insights into actionable steps that pave the way for effective call center management.
- Aircall
Aircall stands out as a powerful tool for managing call volume and enhancing customer service through predictive call analytics. With its robust features, users can seamlessly capture and analyze call data, drawing meaningful insights from past performance. By understanding historical trends, teams can tailor their strategies to anticipate future call volumes effectively.
First, it allows organizations to clean and organize their data systematically, highlighting patterns that facilitate informed decision-making. Next, by employing advanced statistical methods, teams can identify significant trends and seasonal fluctuations. This predictive capability aids in optimizing staffing levels and ensuring that customer demands are met without overwhelming agents. Ultimately, embracing such analytics empowers teams to respond efficiently, creating a proactive approach to customer engagement and support.
- Talkdesk
Using advanced technology, an efficient approach to call center management can be realized. One effective tool focuses on predictive call analytics, which taps into historical QA data to foresee call volumes. By examining previous patterns in call data, businesses can anticipate peak periods, ensuring they are prepared to meet customer demands. Understanding these trends can lead to optimized staffing and improved customer service experiences.
Adopting predictive analytics enhances decision-making processes. Organizations can analyze key metrics to forecast trends, identify high-demand periods, and make strategic staffing choices. This proactive strategy mitigates waiting times for customers and ultimately boosts satisfaction rates. Moreover, analyzing historical data enables centers to adapt their operations based on real-time insights, creating a more agile and responsive service environment. In a rapidly evolving market, the integration of these insights positions companies to outperform their competition effectively.
- Freshdesk
Freshdesk is a vital tool in the realm of Predictive Call Analytics. It provides comprehensive features that enable businesses to harness historical QA data effectively. By analyzing trends in call volumes, agents can identify patterns and predict future demand, ensuring optimal staffing and resource allocation. This platform simplifies data management, allowing users to easily retrieve and examine past call data.
Furthermore, Freshdeskโs intuitive interface facilitates quick insights generation. Users can access conversation transcripts and derive valuable customer feedback for future interaction strategies. Over time, this analysis can highlight recurring issues and opportunities for improvement, ultimately enhancing customer service quality. As organizations move toward predictive analytics, leveraging tools like Freshdesk becomes essential, allowing them to be proactive rather than reactive in managing call volumes. The insights gained can significantly shape future planning and operational efficiency.
Conclusion: The Future of Predictive Call Analytics in Optimizing Call Centers
Predictive Call Analytics stands at the forefront of transforming call center operations. By intelligently analyzing historical QA data, businesses can forecast call volumes, allowing for better resource allocation and training. This proactive approach not only enhances customer experience but also streamlines operational efficiency.
As we look ahead, the integration of advanced analytics will refine training processes, ensuring that representatives are equipped to address customer needs effectively. By harnessing insights from customer interactions, call centers can adapt their strategies, ultimately elevating service and performance across the board. Embracing this technology will define the future of customer service excellence.