How to Compare Performance Between Human and AI QA Review
-
Bella Williams
- 10 min read
Human-AI QA Benchmarking plays a vital role in understanding the comparative effectiveness of human reviewers and AI systems in quality assurance. As businesses increasingly integrate AI into their workflows, establishing reliable benchmarks becomes essential. This section will guide you through the intricacies of comparing human and AI performance in QA processes, highlighting key aspects that influence overall quality outcomes.
Understanding the strengths and weaknesses of both human and AI reviewers allows organizations to optimize their quality assurance efforts. By identifying specific metrics and evaluation criteria, we can develop a framework that supports informed decision-making. This introduction sets the stage for a deeper exploration of strategies and methods that can enhance Human-AI QA Benchmarking, ensuring that both human expertise and AI capabilities are effectively utilized.
Analyze & Evaluate Calls. At Scale.
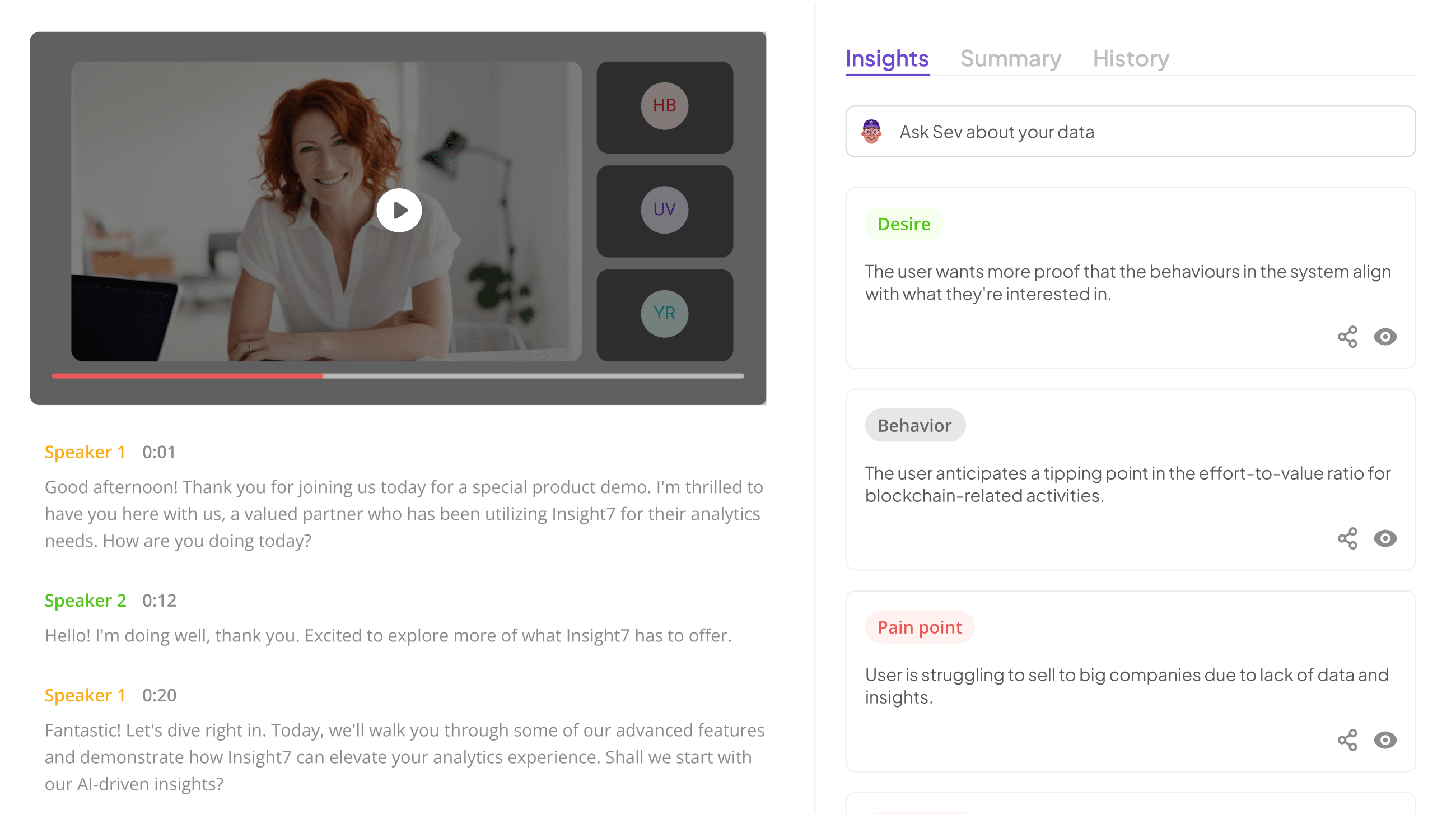
Understanding the Key Aspects of Human-AI QA Benchmarking
Human-AI QA Benchmarking encompasses several critical aspects that enable stakeholders to assess the performance differences between human reviewers and artificial intelligence systems. One essential element is understanding the defined roles of both human and AI in quality assurance. While human reviewers bring contextual understanding and emotional intelligence to evaluations, AI systems excel in processing vast amounts of data quickly and consistently.
Moreover, this benchmarking requires the identification of core metrics to measure effectiveness. Key performance indicators might include accuracy, consistency, speed, and user satisfaction. Each of these metrics contributes to a comprehensive understanding of how human judgments compare with AI assessments. By analyzing these aspects closely, organizations can make informed decisions about the integration of AI into their quality assurance processes. Ultimately, a clear grasp of Human-AI QA Benchmarking is vital for optimizing performance and ensuring quality in various applications.
Defining Quality Assurance in Human and AI Contexts
Quality assurance (QA) in both human and artificial intelligence (AI) contexts operates on the fundamental principle of ensuring product reliability and performance. In human QA processes, the emphasis lies on expertise, intuition, and the ability to assess nuanced scenarios. Conversely, AI QA systems leverage algorithms and data analytics to evaluate outcomes based on set parameters quickly. Recognizing these distinctions is crucial when considering Human-AI QA benchmarking.
To effectively compare performance between human and AI QA reviews, specific metrics come into play. First, accuracy is paramount; it reflects the extent to which both systems produce correct results. Next, efficiency measures how swiftly QA processes occur, highlighting the time taken by human reviewers versus AI systems. Finally, adaptability assesses how well both methods respond to changes in criteria or context. By examining these aspects, organizations can establish robust benchmarks that facilitate effective Human-AI QA benchmarking.
Core Metrics to Evaluate in Human-AI QA Benchmarking
To effectively compare performance in Human-AI QA benchmarking, it is vital to focus on several core metrics. These metrics provide insights into accuracy, efficiency, and user satisfaction, allowing for a balanced evaluation of both human and AI capabilities. The first critical metric is accuracy, which assesses how well responses align with expected outcomes. This measure highlights the precision of both human reviewers and AI systems in addressing queries.
The next metric involves response time, evaluating how quickly both entities can deliver answers. This is crucial for understanding operational efficiency. Another significant aspect is user satisfaction, often gauged through feedback and engagement levels. A final metric to consider is the ability to handle context and nuances, which tests both human intuition and AI adaptability. By analyzing these metrics, organizations can determine strengths and weaknesses, ultimately leading to more effective quality assurance practices and improved human-AI collaboration.
Extract insights from interviews, calls, surveys and reviews for insights in minutes
Strategies for Effective Human-AI QA Benchmarking
To achieve effective Human-AI QA Benchmarking, establishing clear benchmarks and goals is essential. Begin by defining what quality means in your context, including the criteria you will evaluate. Setting specific targets allows you to measure performance accurately and provides a clear framework for comparison. These goals should align with the strategic objectives of your organization and the domains you are assessing.
Next, implementing comparative analysis methods will enhance your benchmarking strategy. This involves gathering data from both human and AI performance on the defined criteria. Identifying patterns, strengths, and weaknesses in both approaches informs decision-making and drives improvements. Additionally, various tools can assist in automating and optimizing this process. Platforms like Insight7, TestComplete, and QA Symphony can simplify data collection and analysis. By employing these methods, organizations can ensure a robust and effective benchmarking strategy that results in insightful performance comparisons.
Step 1: Establishing Benchmarks and Goals
Establishing effective benchmarks and goals is critical in the process of Human-AI QA Benchmarking. First, it is essential to identify the key performance indicators that will guide your evaluation. These benchmarks should include metrics such as error rate, turnaround time, and customer satisfaction scores, enabling a comprehensive comparison between human and AI reviews.
Next, defining clear goals tied to these benchmarks is equally important. For instance, aim to reduce error rates by a certain percentage over a specified period or enhance review efficiency through improved technology integration. By setting achievable and measurable goals, organizations can better track progress and make data-driven decisions to improve both human and AI performance over time. This foundational step paves the way for deeper insights and more effective quality assurance strategies.
Step 2: Implementing Comparative Analysis Methods
In Step 2: Implementing Comparative Analysis Methods, we explore how to measure and compare the effectiveness of Human-AI QA reviews systematically. This phase involves establishing a framework to quantitatively assess performance across both human and AI reviewers. To begin, identifying the key performance indicators (KPIs) relevant to your specific QA process is crucial. These metrics might include accuracy rates, response times, and consistency in identifying errors or inconsistencies.
Once you have determined the KPIs, utilize comparative analysis methods to interpret the data clearly. This can involve statistical techniques such as A/B testing, which allows you to compare results from human reviewers against those from AI systems directly. Visualization tools can also be instrumental in displaying these comparisons, enabling stakeholders to grasp insights at a glance. Ultimately, the goal of this step is to foster a better understanding of Human-AI QA benchmarking and clarify how each approach contributes to overall quality assurance.
List of Tools for Human-AI QA Benchmarking
To conduct effective Human-AI QA Benchmarking, utilizing the right tools is essential. These tools facilitate the assessment of performance differences between human reviewers and AI systems, enabling organizations to derive meaningful insights. Each tool offers unique features tailored for various aspects of quality assurance, such as data analysis, compliance evaluation, and automated testing.
Among the recommended tools, Insight7 excels in transcribing and analyzing call data, streamlining the quality assurance process. TestComplete automates functional testing across various platforms, enhancing the efficiency of evaluations. QA Symphony provides a centralized platform for test management and collaboration, ensuring seamless workflows. Meanwhile, Sikuli employs visual recognition for UI testing, making it valuable for assessing user interfaces. Finally, Applitools focuses on visual testing, verifying that applications appear correctly across different devices. Together, these tools enable comprehensive benchmarking necessary for optimizing quality assurance.
- Insight7
In the realm of Human-AI QA Benchmarking, it is key to recognize the unique strengths and weaknesses of each reviewer. Human reviewers often excel in areas requiring nuanced understanding and empathy, bringing a level of context and interpretation that AI may struggle to replicate. Conversely, AI systems can process vast amounts of data quickly, lending efficiency to quality assurance tasks. This interplay between human intuition and AI precision shapes the future of QA processes.
To effectively compare their performances, one should consider specific criteria. Firstly, accuracy is vital; it assesses each reviewerโs ability to identify errors correctly. Secondly, speed determines how quickly these findings are reported. Lastly, consistency evaluates how reliable the reviewer is across multiple tasks. By analyzing these dimensions, organizations can make informed decisions on integration strategies between human and AI efforts, ultimately refining their QA processes.
- TestComplete
TestComplete is a pivotal tool in the realm of Human-AI QA Benchmarking. It allows users to automate testing for various applications efficiently, thereby streamlining the evaluation process. This tool provides a comprehensive environment where both human testers and AI can contribute to quality assurance efforts, allowing for more rigorous and reliable outcomes.
Utilizing TestComplete, teams can design, execute, and analyze tests across different platforms, such as web, mobile, and desktop applications. The flexibility it offers empowers users to customize their testing strategies based on specific evaluation criteria. By harnessing its capabilities, organizations can gain meaningful insights into their quality assurance processes, ultimately ensuring a higher standard of product reliability. This convergence of human intuition and AI precision underlines the value of TestComplete in establishing effective benchmarks for performance evaluation.
- QA Symphony
In the grand orchestration of quality assurance, the interplay between human and AI contributions creates a harmonious QA Symphony. Understanding this relationship is essential, especially when conducting Human-AI QA Benchmarking. By recognizing how each performs different roles, organizations can enhance their QA processes significantly. This synergy allows for complementary strengths where AI handles data-driven tasks while humans provide the nuanced judgment essential for complex evaluations.
To effectively compare performance, it's important to focus on several key elements. Firstly, assess accuracy and consistency in reviews conducted by both humans and AI. Secondly, analyze the speed and efficiency of each approach in addressing QA needs. Lastly, consider the adaptability of both systems in handling unique challenges. This approach helps to identify strengths and weaknesses, ultimately ensuring that quality assurance is not only robust but also aligned with organizational goals and customer expectations.
- Sikuli
Sikuli is a powerful automation tool that utilizes image recognition to facilitate user interface interactions. In the context of Human-AI QA Benchmarking, Sikuli allows for effective comparisons between human reviews and AI evaluations by automating repetitive tasks in the quality assurance process.
By leveraging Sikuliโs capabilities, QA teams can run visual tests that simulate user behavior, capturing both strengths and weaknesses in the AI system. This will not only help in monitoring the performance of AI-driven reviews but also aid in understanding areas where human reviewers excel. Additionally, Sikuli's functionality supports diverse testing environments, offering flexibility in analyzing performance across various applications.
Using Sikuli as part of the benchmarking process can illuminate discrepancies between human insights and AI responses. This understanding ultimately drives continuous improvement in AI systems, enhancing their accuracy and reliability in delivering quality outcomes.
- Applitools
In the journey of Human-AI QA Benchmarking, the role of effective tools is crucial for performance evaluation. Applitools offers advanced visual testing capabilities that enhance quality assurance by bridging the gap between human oversight and AI efficiency. By providing a comprehensive analysis of user interfaces, it allows teams to compare results seamlessly, pinpointing visual discrepancies that might escape traditional methods.
Utilizing this tool enables QA professionals to establish benchmarks that reflect both human judgment and AI assessments. The visual insights provided by Applitools serve to augment the traditional checklist approach used by human testers. This combination of AI-driven analysis and human intuition fosters a more robust QA process, ultimately leading to higher quality outcomes and improved user experiences.
Conclusion: Drawing Insights from Human-AI QA Benchmarking
The process of Human-AI QA Benchmarking provides valuable insights into the performance differences between human reviewers and AI systems. By systematically comparing these two approaches, organizations can assess strengths and weaknesses, informing future improvements. This benchmarking allows for a clearer understanding of where AI can enhance efficiency and where human discernment still plays a critical role.
Moreover, the insights gained from Human-AI QA Benchmarking not only highlight performance metrics but also offer a pathway for optimizing workflows. By identifying specific areas for training and development, businesses can better integrate AI into their quality assurance processes, ultimately leading to improved outcomes and more effective collaboration between human and machine.
๐ฌ Need Help? Chat with Support
Our team typically responds within minutes