In a competitive market, understanding customer conversations can be a game changer. Many businesses overlook the treasure trove of insights hidden within their customer interactions. By recognizing churn predictive patterns, organizations can take proactive steps to retain their clientele. The language, tone, and frequency of customer communication often signal their satisfaction levels and potential intentions to disengage.
By delving into these conversations, businesses can pinpoint specific issues that lead to dissatisfaction. Each snippet of dialogue may reveal critical insights into customer needs and frustrations, which can inform strategies to enhance their experiences. Analyzing these patterns not only facilitates early detection of churn signals but also equips companies with the necessary information to tackle the root causes of customer attrition.
Analyze & Evaluate Calls. At Scale.
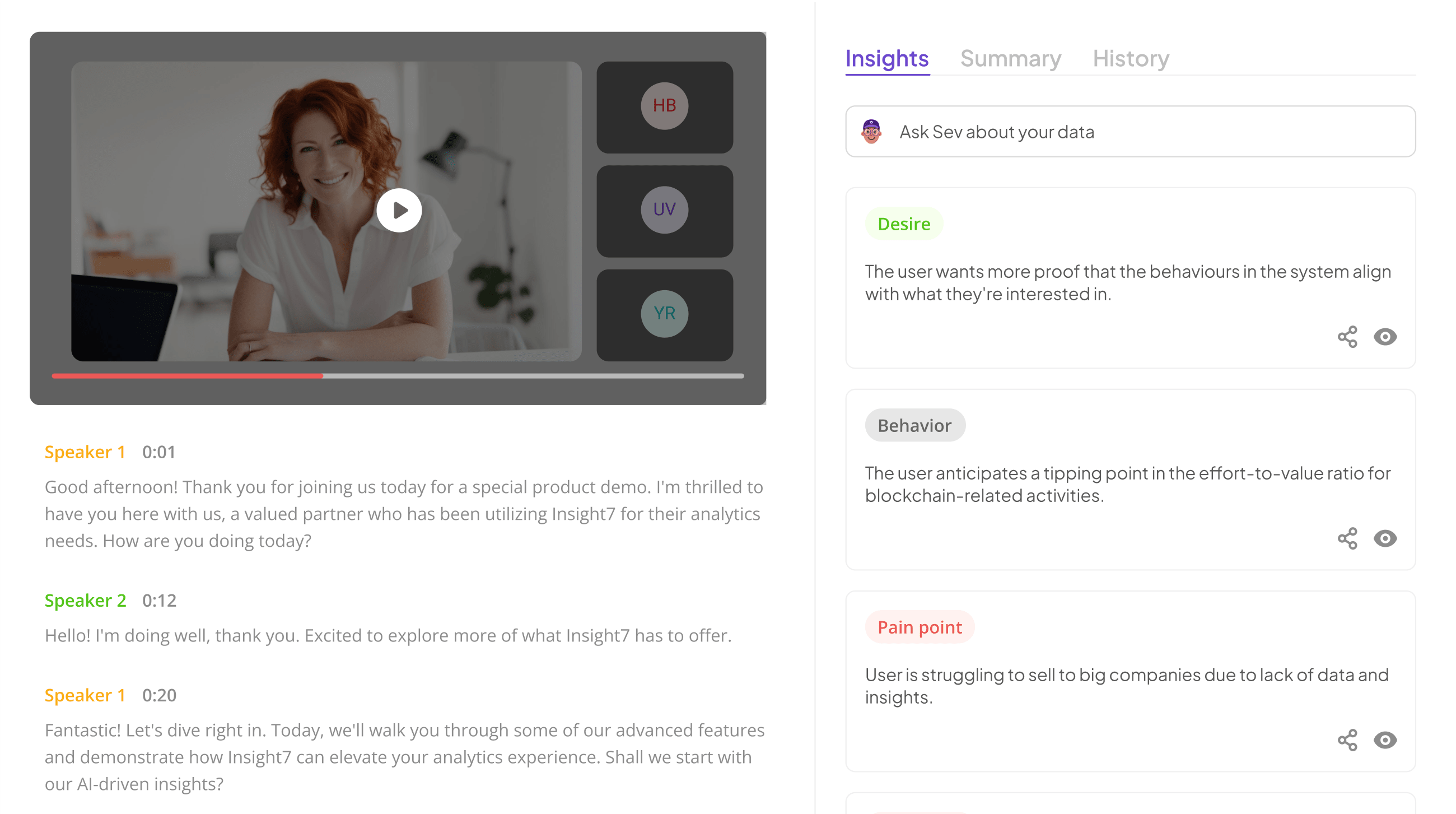
Identifying Churn Predictive Patterns in Communication
Identifying churn predictive patterns in communication is essential for understanding customer behavior. By analyzing conversations, businesses can spot early signs of churn, enabling proactive measures to retain customers. One critical aspect is recognizing negative sentiment and tone, which often indicates dissatisfaction. If a customer consistently uses negative language, it may signal an impending departure.
Another important pattern to watch for is the frequency of repeated support requests. When customers repeatedly reach out for assistance, it often highlights ongoing frustrations with the product or service. By identifying these churn predictive patterns, companies can better understand their customersโ needs. This information empowers businesses to initiate conversations that address issues before they escalate, ensuring a smoother customer experience. Engaging customers through meaningful communication can ultimately foster loyalty and mitigate churn.
Analyzing Negative Sentiment and Tone
Understanding negative sentiment and tone in customer conversations is crucial to uncovering churn predictive patterns. Effective analysis begins with using sentiment analysis tools to detect negative language that can indicate dissatisfaction. By monitoring the frequency and intensity of negative remarks, organizations can gain insight into customer emotions and sentiments.
Subsequently, correlating these negative sentiments with customer departure tendencies can reveal important trends. For instance, consistent negative comments may highlight recurring issues, allowing businesses to address them proactively. By analyzing the tone of conversations, companies can identify not only the potential for churn but also areas requiring improvement. Understanding these patterns ultimately aids in establishing strategies to reduce churn by enhancing customer satisfaction and retention.
- Step 1: Using sentiment analysis tools to detect negative language
To effectively utilize sentiment analysis tools, it's essential to focus on how they can detect negative language within customer interactions. Begin by selecting an appropriate sentiment analysis tool that can parse through customer conversations, extracting valuable insights regarding their emotional tone. These tools identify negative phrasing, slang, and emotional cues that indicate discontent, which can be instrumental in understanding churn predictive patterns.
Next, analyze the data yielded by these tools to highlight common negative sentiments. Group and categorize these findings according to specific themes or issues customers frequently mention. For instance, if multiple conversations reflect frustration regarding service delays, this is a telling sign of potential churn. By maintaining a closer look at these negative indicators, businesses can engage with their customers proactively, addressing concerns before they lead to disengagement. Thus, utilizing sentiment analysis not only aids in early detection of negative trends, but also empowers businesses to take timely corrective actions in the journey of customer retention.
- Step 2: Correlating negative sentiment with customer departure tendencies
Understanding how negative sentiment can correlate with customer departure tendencies is crucial in identifying churn predictive patterns. When a customer expresses dissatisfaction during conversations, it often signals a growing concern about the service or product. For instance, if customers frequently mention frustrations such as delays or product quality issues, it hints at their likelihood to leave. Companies should prioritize monitoring these conversations to identify consistent negative sentiments.
Another important aspect to consider is the context in which negative sentiments arise. By categorizing these sentiments, businesses can better understand the triggers for customer discontent. Specific expressions of frustration can indicate broader issues within the company. Timely intervention based on identified patterns can reduce churn rates and improve customer loyalty. Overall, recognizing these connections between negative sentiments and customer behavior is essential for developing effective retention strategies.
Recognizing Requests for Repeated Support
Recognizing requests for repeated support is a crucial conversation pattern in identifying churn predictive patterns. When customers repeatedly seek assistance, it often indicates underlying dissatisfaction or unresolved issues. Tracking the frequency of these support requests can help businesses pinpoint trends that may lead to customer disengagement.
For example, if a customer must contact support multiple times for the same issue, it highlights a flaw in the resolution process or a lack of effective communication. Additionally, identifying patterns, such as the nature of repetitive inquiries, can reveal specific areas of concern that need immediate attention. Addressing these issues promptly not only improves customer satisfaction but also reduces the likelihood of churn. Active monitoring and analysis of these patterns empower businesses to enhance their support systems, fostering loyalty and retaining valuable customers.
- Step 1: Tracking frequency of support requests
Tracking the frequency of support requests is a vital step in uncovering churn predictive patterns among customers. Regularly monitoring how often clients reach out for assistance can reveal underlying issues that contribute to dissatisfaction. For instance, an increase in support inquiries may indicate unresolved problems that could drive customers away. By effectively tracking these requests, businesses can gauge the overall sentiment surrounding their service.
To optimize this tracking process, consider implementing a systematic approach. First, categorize support requests based on the type of issue reported. Second, analyze the frequency of these issues over time to identify recurring problems. Lastly, correlate these patterns with customer satisfaction metrics, which can provide deeper insights. This analysis not only highlights critical areas needing improvement but also helps predict potential churn, enabling companies to take proactive measures before customers decide to leave.
- Step 2: Identifying patterns of dissatisfaction through repetitive inquiries
To identify patterns of dissatisfaction through repetitive inquiries, itโs essential to examine customer interactions closely. When customers repeatedly reach out for support, it often indicates unresolved issues or dissatisfaction with the product or service. Engaging in frequent discussions with the same customer can reveal underlying frustrations that may lead to churn. By analyzing these conversations, companies can uncover patterns that predict potential customer exits.
To effectively identify these churn predictive patterns, consider the following steps:
Monitor Support Requests: Keep track of how often customers contact support. High frequency often signals dissatisfaction.
Analyze Inquiry Content: Look for recurring themes in inquiries. Are customers asking similar questions? If so, they're likely signaling a problem.
Evaluate Response Effectiveness: Determine if responses adequately resolve issues. Ineffective solutions can perpetuate dissatisfaction, increasing the likelihood of churn.
Understanding and acting upon these repetitive inquiries can greatly enhance customer retention efforts and improve overall satisfaction.
Extract insights from interviews, calls, surveys and reviews for insights in minutes
Implementing Effective Tools for Detecting Churn Predictive Patterns
Implementing effective tools for detecting churn predictive patterns begins with selecting the right analytics software. These tools must be sophisticated enough to sift through vast amounts of customer interaction data to identify key indicators of disengagement. By focusing on customer sentiment, frequency of support requests, and even the language used during conversations, businesses can get ahead of churn issues before they escalate.
To achieve this, itโs essential to employ a suite of analytics tools designed to process conversation data accurately. For instance, a comprehensive conversation analytics platform can highlight negative sentiment trends and reveal customers who may be at risk of leaving. Furthermore, integrating these tools with existing customer service systems provides seamless access to insights, maximizing their effectiveness. By taking these steps, organizations can not only detect churn predictive patterns but also develop strategies to retain valuable customers.
Best Tools for Analyzing Customer Conversations
To effectively analyze customer conversations for identifying churn predictive patterns, several tools stand out. These tools specialize in dissecting dialogue and uncovering insights hidden within customer interactions. The ability to systematically analyze conversations can highlight essential signals that indicate potential churn, allowing businesses to take proactive measures.
Insight7: This versatile platform offers comprehensive conversation analytics. It is designed to manage and synthesize large volumes of customer data. With intuitive dashboards, businesses can easily spot trends related to customer dissatisfaction.
Tool 2: This tool offers advanced sentiment analysis features that help in assessing customer emotions accurately. By correlating tone and sentiment with churn likelihood, teams can quickly address issues before they escalate.
Tool 3: Known for its unique applications, this option integrates seamlessly with existing service platforms. It enhances performance by providing actionable insights tailored to specific customer needs.
Tool 4: Taking advantage of advanced AI capabilities, this tool helps companies gain a deeper understanding of customer emotions. This understanding can guide strategic changes that influence retention.
Tool 5: This platform is especially beneficial for tracking customer queries and identifying repetitive patterns that may indicate dissatisfaction. It provides analytics for better engagement and service improvements.
Utilizing these analytical tools allows businesses to stay ahead, mitigating customer churn effectively.
- insight7: A versatile tool for comprehensive conversation analytics
insight7: A versatile tool for comprehensive conversation analytics empowers organizations to identify churn predictive patterns effectively. This platform enables businesses to analyze customer interactions at scale, transforming raw data into actionable insights. By systematically examining conversations, companies can uncover trends that may signal potential churn, such as recurring issues or increasing negative sentiment.
With insight7, users can seamlessly navigate through vast amounts of customer communication. The toolโs intuitive design allows for easy categorization and analysis, making it simpler to spot detrimental patterns. Organizations can detect shifts in customer sentiment, providing them the ability to respond proactively and ultimately enhance customer retention strategies. Additionally, the integration capabilities ensure insight7 fits seamlessly into existing workflows, allowing companies to maintain efficient operations while deriving crucial insights for minimizing customer turnover.
- Tool 2: Features and benefits in identifying churn signals
Identifying churn signals is crucial for retaining customers and understanding their needs. Tool 2 offers features that enable businesses to pinpoint these signals effectively. By analyzing customer conversations, the tool highlights specific phrases and sentiments that indicate potential dissatisfaction. These insights empower companies to act swiftly before customers decide to leave. Recognizing patterns, such as repeated complaints or negative feedback, allows organizations to address issues proactively.
Additionally, this tool promotes a deeper understanding of customer behavior through comprehensive data analysis. It can track support interactions to reveal trends in customer frustration or confusion. By leveraging such insights, businesses can fine-tune their offerings and enhance customer experiences, ultimately reducing churn rates. This proactive approach not only helps retain existing customers but also fosters loyalty and satisfaction over time, transforming potential churn into ongoing engagement.
- Tool 3: Unique applications for predicting customer exit
Understanding unique applications for predicting customer exit involves examining innovative methods that organizations can employ to anticipate churn. Companies today are inundated with customer interaction data. By effectively analyzing these interactions, businesses can uncover underlying churn predictive patterns that may otherwise go unnoticed. Investing in the right tools enables teams to transform these insights into actionable strategies, fostering customer retention and satisfaction.
One unique application is sentiment analysis, which uncovers emotions embedded within customer conversations. By identifying shifts in tone, teams can proactively address customer concerns before they lead to exit. Similarly, monitoring the frequency of requests for support highlights recurring issues, signaling possible dissatisfaction. Additionally, employing conversation analytics tools offers comprehensive insights into dialogue patterns, enabling organizations to gauge which topics may precede a customerโs decision to leave. Understanding and implementing these applications can significantly enhance retention efforts and mitigate churn.
- Tool 4: Integration with existing customer service platforms
Integrating churn predictive patterns with existing customer service platforms can significantly enhance a business's ability to retain customers. By consolidating data streams from various sources, companies can create a rich tapestry of customer interactions. This integration allows for a holistic view of conversations, making it easier to spot patterns that may indicate customer dissatisfaction or potential churn.
To effectively harness this integration, it is crucial to focus on a few key areas: first, ensuring real-time data flow between customer service platforms and analytics tools is vital. This immediate access to customer interactions enables quicker responses to signs of distress. Second, training customer service representatives to identify and act upon these predictive patterns can further mitigate churn risks. Lastly, utilizing analytics tools to aggregate insights allows businesses to refine their support strategies continuously. Adopting these practices will empower organizations to proactively engage customers, safeguarding loyalty and minimizing churn.
- Tool 5: Advanced AI capabilities for nuanced understanding
Advanced AI capabilities serve as a game changer in identifying churn predictive patterns, enhancing an organization's ability to understand customer sentiment and behavior. These capabilities leverage machine learning to analyze vast amounts of data from customer interactions. By accurately interpreting nuances in tone, language, and context, AI can highlight potential signs of customer dissatisfaction, allowing proactive measures to be taken.
To effectively harness these capabilities, several strategies can be employed. First, deploying advanced sentiment analysis tools can help pinpoint negative language or tone across customer conversations. Second, utilizing predictive analytics enables organizations to correlate identified patterns with historical churn data. Lastly, ongoing training in AI models ensures a deeper understanding of evolving customer expectations and behaviors. Together, these strategies create a comprehensive approach to recognizing and addressing triggers of customer churn before they escalate.
Conclusion: Leveraging Conversation Patterns to Mitigate Customer Churn
In conclusion, understanding churn predictive patterns is crucial for businesses striving to retain customers. By attentively analyzing customer conversations, you can uncover underlying issues that may lead to churn, such as negative sentiment or repeated support requests. These insights empower companies to proactively address customer concerns, fostering a more engaging relationship.
Additionally, implementing effective tools to gather and analyze conversation data further enhances this process. With timely insights, businesses can refine their strategies and offer tailored solutions, ultimately reducing churn rates. Emphasizing customer engagement becomes essential, ensuring that conversations evolve from reactive to proactively addressing customer needs and expectations.