AI-Driven Product Management is transforming how product managers approach their roles. As teams face increasing complexity and competition, understanding customer needs through data becomes crucial. Traditional methods of gathering insights often fall short, prompting the need for innovative strategies that harness artificial intelligence.
By integrating AI tools, product managers can derive actionable insights from vast datasets, enabling better decision-making. These technologies not only streamline product development but also foster collaboration among teams. As the demand for rapid adjustments grows, embracing AI-driven strategies is essential for maintaining a competitive edge in the market.
Analyze qualitative data. At Scale.
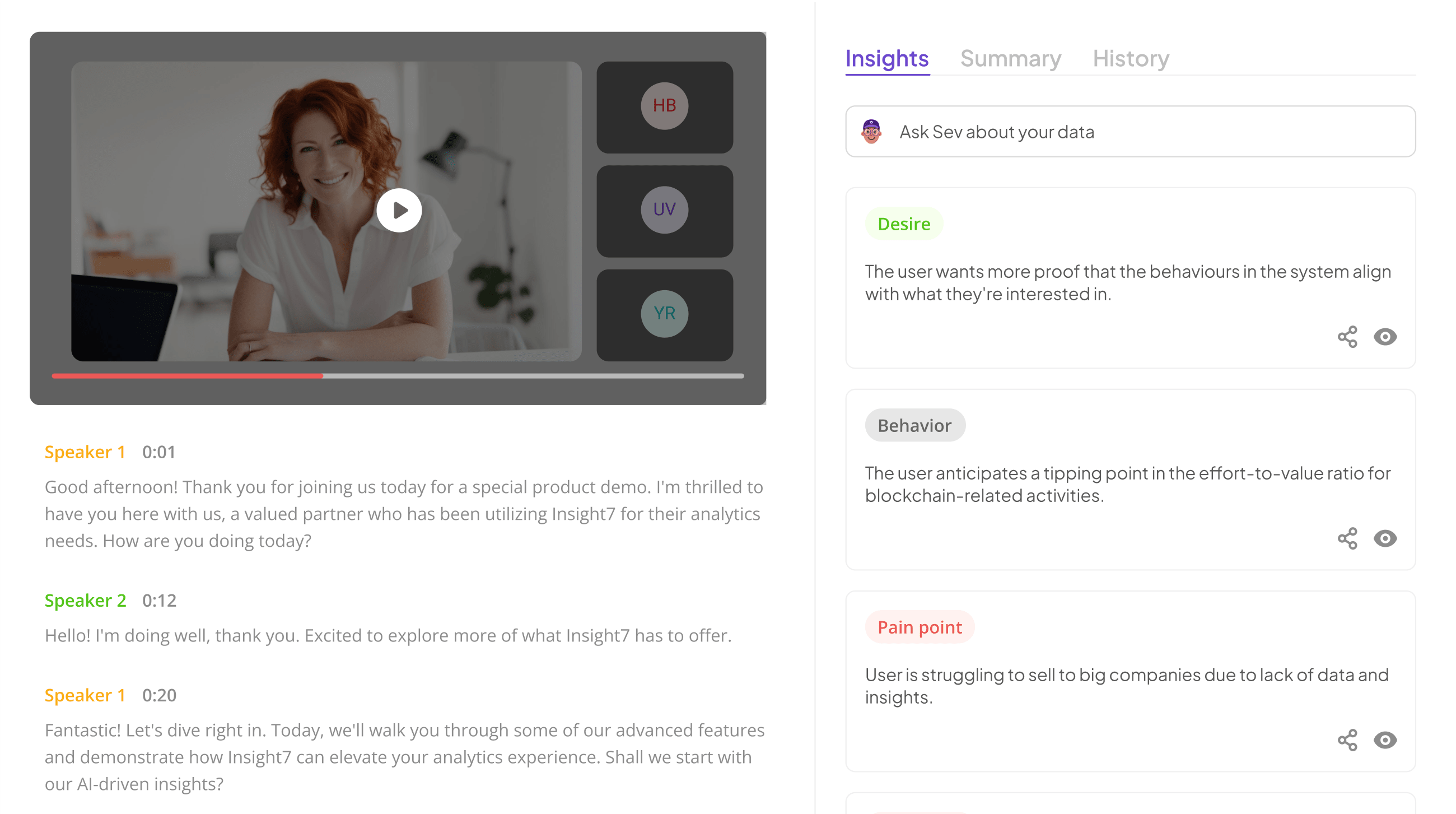
The Role of AI-Driven Product Management in Modern Business
AI-Driven Product Management plays a pivotal role in shaping modern business strategies. By harnessing advanced analytics and predictive capabilities, product managers can make informed decisions that align with market demands. This approach enables a deeper understanding of customer needs and preferences, which is essential in todayโs competitive landscape. Furthermore, AI tools not only streamline product development processes but also help in identifying potential disruptions before they occur.
Incorporating AI into product management enhances collaboration among teams. It fosters a culture of data-driven insights, ensuring that all stakeholders are aligned with the product vision. AI facilitates the objective evaluation of user feedback, allowing for continuous improvement. As businesses adapt to rapidly changing environments, the need for AI-Driven Product Management becomes increasingly clear. Embracing these tools equips product managers with the insights and agility required to navigate the complexities of modern markets successfully.
Enhancing Decision-Making with AI Tools
In today's rapidly evolving market, decision-making for product managers can be complex. AI-driven product management techniques offer essential capabilities to enhance the quality of these decisions. Utilizing advanced analytics allows managers to interpret vast amounts of data efficiently, providing insights that are both timely and relevant. This not only streamlines the decision-making process but also enables a more proactive approach to addressing market needs.
Furthermore, AI tools help identify trends and patterns that may not be immediately apparent through traditional analysis. Enhanced predictive capabilities empower product managers to foresee customer behaviors and preferences. By integrating AI into their decision-making framework, they can make informed choices that align with customer demands while optimizing resources. This method fosters a culture of data-driven innovation, ensuring that product strategies remain competitive and customer-focused in an ever-changing landscape.
Streamlining Product Development with AI Integration
Integrating AI into product development transforms how teams operate, driving efficiency and innovation. AI-Driven Product Management enhances this process by automating routine tasks and providing valuable insights. As a result, teams can focus on strategic initiatives that propel product success. This integration not only streamlines workflows but also reduces the time spent on manual data analysis, allowing product managers to make informed decisions quickly.
Moreover, AI tools gather and analyze user feedback, revealing crucial pain points and user desires. This data helps teams refine their products based on real customer inputs, ensuring alignment with market needs. When product managers harness AI for data-driven insights, they enhance prioritization and resource allocation. Ultimately, the synergy between AI and product development fosters an agile environment where innovation can thrive, leading to improved outcomes and customer satisfaction.
Extract insights from interviews, calls, surveys and reviews for insights in minutes
Exploring the Benefits of AI-Driven Product Management
AI-Driven Product Management has revolutionized how product managers approach their work. First and foremost, it offers data-driven insights that enhance decision-making. With advanced analytics, product managers can sift through vast amounts of data to identify trends and customer preferences. This predictive analysis enables them to foresee market shifts and adapt their strategies accordingly, fostering a proactive approach rather than a reactive one.
In addition to data insights, AI tools significantly boost collaboration among team members. By streamlining workflows, AI systems help organize tasks and facilitate communication, ensuring that everyone stays on the same page. This collaboration extends to customer feedback as well, as AI tools can quickly analyze surveys and comments to uncover actionable insights. Ultimately, embracing AI-Driven Product Management equips product managers with the tools they need to create better products, respond agilely to market needs, and enhance overall efficiency.
Data-Driven Insights and Predictive Analysis
Data-driven insights and predictive analysis are essential components of AI-driven product management. By leveraging vast amounts of data, product managers can uncover deep insights that guide strategic decisions. For instance, analyzing customer feedback and behavioral patterns helps in identifying product strengths and weaknesses. This analysis provides a clearer understanding of market trends, enabling product managers to anticipate future demands and refine their offerings accordingly.
Moreover, predictive analysis empowers product teams to forecast upcoming trends and customer needs. Utilizing advanced algorithms, AI tools can analyze historical data to predict future outcomes. This allows for proactive decision-making, minimizing risks associated with product launches. When product managers embrace data-driven insights and predictive analysis, they significantly enhance their ability to make informed decisions that drive innovation and growth. Ultimately, the integration of AI in this process not only boosts efficiency but also ensures that products meet consumer expectations in a rapidly evolving market.
Boosting Team Collaboration Through AI
Effective communication is the backbone of any successful team, and AI is transforming how product managers enhance this aspect. AI-driven collaboration tools simplify information sharing and streamline workflows, allowing teams to focus on their core tasks. By utilizing AI-powered platforms, product managers can automate mundane tasks, freeing up time for creative brainstorming and problem-solving. This shift not only accelerates project timelines but also fosters a collaborative environment where innovation can thrive.
In addition to facilitating communication, AI tools provide real-time data analysis, enabling teams to make informed decisions quickly. For instance, these tools can gather feedback from various stakeholders and synthesize it into actionable insights. This dynamic enhances collective efforts within teams, empowering members to work together more effectively. With AI-driven product management, the synergy between team members deepens, and overall productivity sees significant improvement. Ultimately, embracing these AI solutions leads to a more cohesive and engaged team, ready to tackle any challenge.
Top AI Tools for Product Managers
In todayโs fast-paced market, the right AI tools can significantly enhance product management efficiency and effectiveness. Among the top AI tools available, insight7 stands out for its ability to provide actionable insights from customer feedback, helping product managers understand user needs better. Asana and Trello are excellent for streamlining project management, enabling teams to collaborate seamlessly and track progress in real time. Jira integrates well with development processes, offering tracking features for bugs and user stories, making it a favorite among tech-savvy product teams.
Productboard is another key tool designed specifically for product management, allowing teams to prioritize features based on user feedback and market demands. Each of these tools plays a crucial role in empowering product managers to leverage AI-driven product management strategies effectively. By integrating these tools into their workflows, product managers can gain valuable insights, prioritize effectively, and drive successful product outcomes.
1. insight7
In the realm of AI-driven product management, insight7 stands out as a valuable resource for product managers aiming to understand customer signals at scale. This platform facilitates the analysis of extensive customer data drawn from interviews and conversations, significantly improving the decision-making process. Product managers need to harness such tools to stay competitive and adapt quickly to ever-changing market demands.
Traditional methods of data analysis often fall short, especially when it comes to managing the volume and complexity of insights generated today. With insight7's user-friendly, self-service interface, product managers can swiftly translate customer insights into actionable business strategies. This ability to analyze data quickly and efficiently allows teams to work collaboratively, minimizing inefficiencies and ensuring that insights are easily accessible. Ultimately, embracing AI tools like insight7 empowers product managers to unlock the potential of their customer conversations.
2. Asana
As product teams face increasing complexity, effective project management tools become essential. A robust platform can transform workflows and enhance communication. By harnessing AI-driven product management solutions, product managers can better aggregate and analyze data, leading to improved project execution.
Working within such systems allows product managers to streamline tasks in a user-friendly interface. Insights gleaned from customer interactions, stored in libraries, can reveal vital pain points and desires that guide project prioritization. The integration of AI tools automates the distillation of information, enabling teams to make informed decisions quickly. This analysis not only saves time but also enhances collaboration by ensuring everyone is aligned on objectives. Embracing AI-powered tools helps product managers navigate their roles more effectively, driving successful product outcomes while maintaining focus on customer needs.
3. Trello
Trello serves as a vital tool in AI-Driven Product Management, enabling product managers to organize tasks efficiently. Utilizing Trello's intuitive boards, teams can visualize their workflow and track progress in real time. This visual organization not only enhances transparency but also fosters collaboration among team members. With AI tools integrated within Trello, managers can automate repetitive tasks, allowing them to focus on more strategic initiatives.
Moreover, Trello's flexible structure supports various methodologies, including Agile and Kanban, thus accommodating diverse project needs. Managers can set deadlines, assign responsibilities, and monitor task completion, streamlining the product development process. By harnessing AI capabilities, Trello can analyze team performance over time, providing insights that inform better decision-making. In this way, it transforms data into actionable strategies, making it an indispensable asset for modern product management.
4. Jira
In today's dynamic product management environment, tools like Jira have become essential for teams striving to implement AI-driven product management effectively. Jira streamlines project tracking, allowing product managers to harness data insights for better decision-making. By integrating AI capabilities into Jira, teams can analyze performance metrics, assess backlog priorities, and identify potential obstacles quickly. This results in more agile and efficient workflows, where teams can respond to market changes with precision.
Furthermore, with the emphasis on collaboration in product development, Jira acts as a central hub for communication among team members. The software's customizable dashboards provide transparency and real-time visibility into task progress. This fosters a more collaborative environment, enabling product managers to harness collective insights and make informed decisions swiftly. Ultimately, leveraging AI within tools like Jira elevates the product management process, enabling teams to deliver better products that meet consumer needs.
5. Productboard
Product management thrives on effective communication, data analysis, and team collaboration. AI-driven product management transforms how product managers understand customer feedback and refine their offerings. By integrating AI tools, product managers can quickly analyze vast amounts of data from various sources, gaining valuable insights that drive informed decisions. This approach not only speeds up the decision-making process but also enhances responsiveness to market changes.
Utilizing AI tools, product managers can uncover trends and cluster feedback based on project attributes, creating a clear picture of customer needs. This allows for real-time questions and answers about customer perceptions, facilitating smoother communication among teams. Moreover, embracing AI-driven product management encourages a consultative approach, helping teams evolve from simply taking orders to genuinely understanding and addressing customer challenges. This shift adds significant value to product development, ultimately leading to greater customer satisfaction and business success.
Conclusion: Embracing AI-Driven Product Management for Future Success
As organizations navigate the complexities of modern markets, embracing AI-Driven Product Management has become essential. This innovative approach empowers product managers to harness data more effectively, transforming insights into actionable strategies. By leveraging AI tools, teams can optimize workflows and enhance collaboration, ensuring they remain competitive in a rapidly evolving landscape.
The future of product management lies in its ability to integrate advanced technologies. By adopting AI-driven methodologies, product managers can anticipate customer needs, streamline processes, and make informed decisions. Investing in AI tools today is not just a choice; it is a strategic imperative for achieving long-term success. Ultimately, embracing AI-driven methodologies ensures organizations are prepared for the challenges ahead.