The rise of voice communication technology has transformed how we interact, making clarity in conversations more essential than ever. Understanding how to analyze conversational clarity through data is pivotal for optimizing communication. Clarity Metrics Analysis provides a structured approach to assess and enhance the effectiveness of voice interactions.
In this section, we will explore various metrics that capture the essence of conversational clarity in voice data. By examining parameters such as signal-to-noise ratio and transcription accuracy, we can gain valuable insights into the clarity of dialogue. The objective is to equip readers with an understanding of these essential metrics, empowering them to improve communication outcomes through data-driven decision-making.
Transcribe & extract insights from interviews. At Scale.
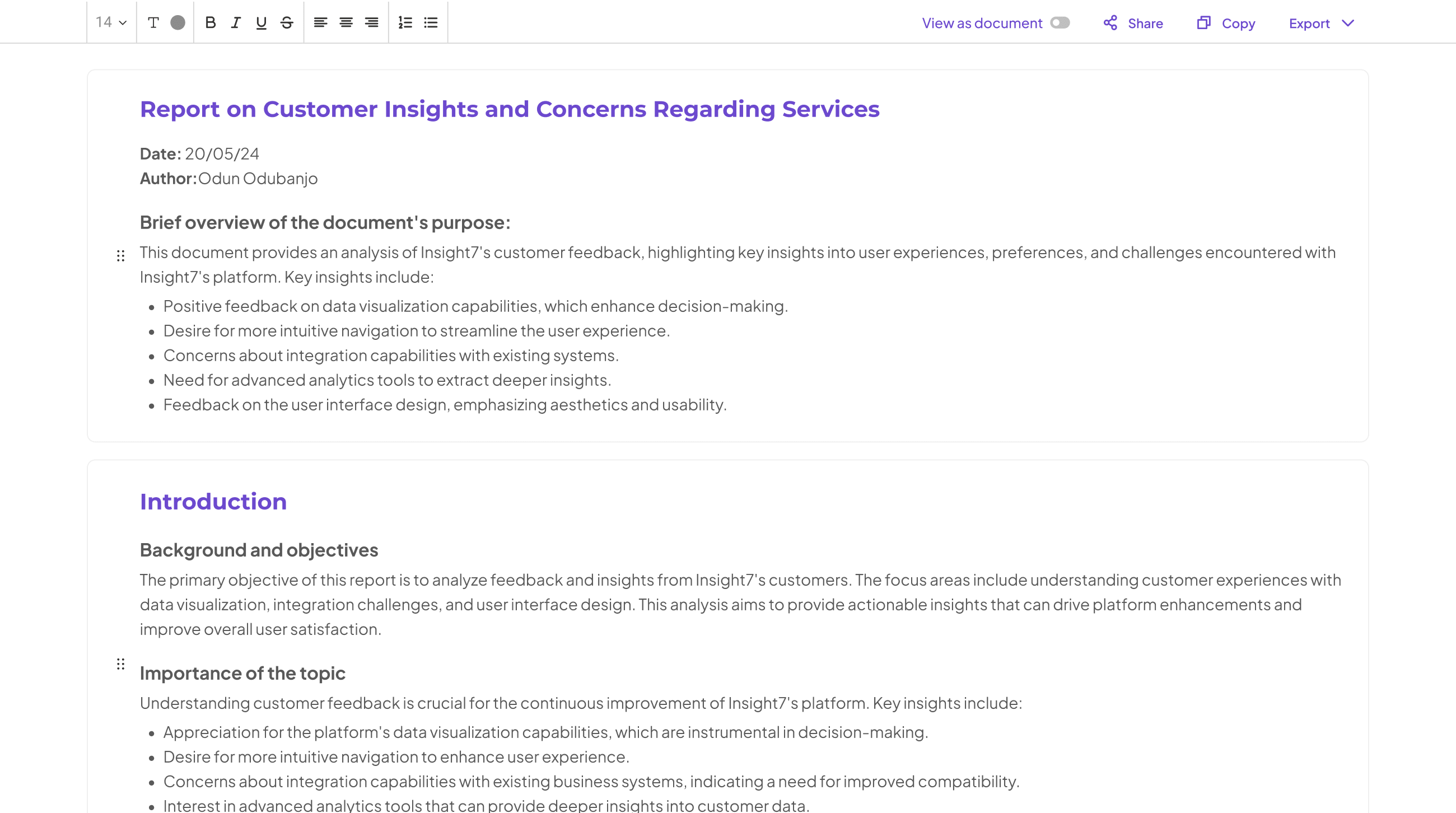
Essential Metrics for Clarity Metrics Analysis
Understanding the essential metrics for Clarity Metrics Analysis is crucial for evaluating conversational clarity in voice data. These metrics provide concrete ways to interpret audio quality, ensuring that discussions remain clear and effective. Among these metrics, Signal-to-Noise Ratio (SNR) plays a key role. A higher SNR indicates clearer audio, making it easier for listeners to understand the conversation without background distractions.
Another significant metric in this analysis is Spectral Clarity, which examines the spectral flatness of sound. Tools such as spectral analysis software can help quantify this aspect, offering insights into the uniformity of sound distribution across frequencies. By analyzing these acoustic features, organizations can enhance their understanding of voice data clarity, ultimately leading to improved communication strategies and outcomes.
Acoustic Features in Clarity Metrics Analysis
Acoustic features play a vital role in clarity metrics analysis as they assess the quality of audio signals in voice data. One primary aspect to examine is the signal-to-noise ratio (SNR), which measures the level of a desired signal against background noise. A higher SNR indicates clearer audio, while lower values suggest muddiness in communication, affecting the ease with which listeners can interpret the spoken words.
Another critical acoustic feature is spectral clarity, which evaluates the flatness of the audio spectrum. Tools that analyze the frequency distribution of sound can provide insights into the clarity of conversation. For example, a spectrum that demonstrates even energy across frequencies indicates higher clarity, whereas uneven distributions often correlate with issues in audio quality. Thus, understanding these features is essential for effective clarity metrics analysis, enabling enhancements in communication strategies and training for improved conversational interactions.
- Signal-to-Noise Ratio (SNR)
Understanding Signal-to-Noise Ratio (SNR) is crucial in clarity metrics analysis, particularly when evaluating voice data. SNR represents the level of desired signal compared to the level of background noise. A higher SNR indicates that the voice signal stands out more clearly against unwanted noise, which enhances the overall clarity of the conversation. It essentially filters out co-existing noise, allowing listeners to discern messages more effectively.
Measuring SNR involves analyzing audio recordings to assess how much of the sound is useful compared to interference. Techniques such as spectral analysis can be employed to quantify SNR levels accurately. When companies utilize SNR as a metric, they can significantly improve customer interactions by ensuring clear and engaging communication. This attention to signal clarity reduces misunderstandings and enhances customer satisfaction, ultimately facilitating better outcomes in business strategies and communication approaches.
- Importance of SNR in determining clarity.
Signal-to-Noise Ratio (SNR) plays a crucial role in clarity metrics analysis, particularly in voice data. It is fundamental for understanding the quality of audio. A higher SNR indicates clearer audio, where the desired signal is much more distinguishable from background noise. This distinction significantly enhances the listener's ability to comprehend and engage with the content being conveyed. Conversely, a lower SNR can lead to confusion and miscommunication, emphasizing the importance of achieving a favorable SNR in any audio recording.
To accurately measure and interpret SNR levels, tools and methodologies can be employed to assess audio recordings effectively. Techniques may include comparing the amplitude of the signal against the noise present in the background. By refining the SNR, organizations can enhance conversational clarity in voice interactions. This focus on SNR is essential for ensuring that conversations remain engaging and yield the desired insights and feedback, ultimately transforming how communication is perceived and understood.
- How to measure and interpret SNR levels.
To gauge the effectiveness of voice data clarity, Signal-to-Noise Ratio (SNR) is a critical metric. SNR measures the level of desired signal compared to background noise, allowing analysts to evaluate how clearly a conversation is perceived. A higher SNR suggests that the voice audio is distinct, which generally contributes to a more intelligible dialog. Lower SNR levels indicate higher background noise and, consequently, can hinder clarity. Thus, understanding SNR levels is vital for ensuring effective communication.
To measure SNR, you can utilize various audio analysis tools that capture both the signal and noise levels within recorded conversations. After calculating these values, interpreting SNR involves knowing benchmarks typical within your specific context or industry. For example, in voice data, an SNR of 20 dB or higher often indicates excellent clarity. By conducting a Clarity Metrics Analysis that incorporates SNR evaluations, you can refine communication strategies and enhance the overall user experience, maximizing presentation clarity in any interaction.
- Spectral Clarity
Spectral clarity plays a vital role in determining the quality and understandability of voice data. This concept revolves around the frequency characteristics of audio signals, helping us analyze how clear a voice is during conversation. Increased spectral clarity implies that the sound is more distinguishable and free from distortion or background noise, which directly affects user comprehension and engagement.
To measure spectral clarity, two essential components come into focus: spectral flatness and the overall energy distribution across frequencies. Tools such as Fast Fourier Transform (FFT) allow researchers to visualize and quantify these parameters. By doing so, professionals can gain insights into speech clarity that can guide coaching, training, and adjustments to communication strategies. This assessment not only improves individual performance but also aligns the communications more closely with audience expectations. Ultimately, spectral clarity serves as a foundational aspect of clarity metrics analysis in voice data.
- Explanation of spectral flatness and its relevance.
Spectral flatness is a crucial concept in the analysis of voice data, particularly concerning clarity metrics analysis. It quantifies the "flatness" of a sound spectrum, which can indicate the degree of noise versus tonal content in an audio signal. Higher spectral flatness values suggest a noise-like characteristic, while lower values indicate more tonal qualities. Understanding these characteristics is essential for identifying the clarity of speech in various contexts, including customer interactions.
The relevance of spectral flatness lies in its ability to provide insights into conversational clarity. When assessing voice data, a lower spectral flatness suggests a clearer signal, allowing listeners to better perceive spoken words. This metric can be particularly beneficial in applications where understanding and retaining information is paramount, such as customer service calls. By evaluating spectral flatness alongside other clarity metrics, organizations can improve their communication strategies and enhance the overall customer experience.
- Tools and techniques for spectral analysis.
To evaluate voice data effectively, spectral analysis is paramount. Employing various tools and techniques can help extract meaningful insights regarding clarity metrics analysis. Key techniques include Fourier transforms, which decompose sound waves into their constituent frequencies. This method aids in understanding the spectral characteristics of voice recordings. Another crucial approach is the use of spectrograms, which provide visual representations of frequency changes over time. By analyzing these spectrograms, one can pinpoint specific issues affecting clarity, such as background noise or inconsistent volume levels.
Moreover, integrating machine learning algorithms into spectral analysis can enhance the accuracy of clarity assessments. These algorithms can identify patterns and anomalies in voice data that may not be immediately recognizable. This holistic approach allows for a deeper understanding of conversational clarity and the underlying factors influencing it. In conclusion, leveraging advanced tools for spectral analysis is essential for achieving precise clarity metrics analysis in voice data.
Assessing Clarity Through Linguistic Metrics
Assessing clarity through linguistic metrics is crucial for understanding the effectiveness of voice data communication. This process involves analyzing various aspects, such as transcription accuracy and semantic coherence, to evaluate how well conversations convey intended messages. By examining these linguistic elements, organizations can enhance customer interactions and align communication strategies with the needs of their audience.
Key components of clarity metrics analysis include error rate analysis and understanding semantic relationships. Error rates indicate the frequency of transcription mistakes, which directly affect conversational clarity. Lower error rates typically lead to improved understanding and engagement. Additionally, assessing semantic coherence helps evaluate how well ideas flow in dialogue, ensuring relevance and logical connection in conversations. Utilizing these metrics allows for a comprehensive approach to improving clarity in voice data, ultimately fostering better customer experiences and more effective communication.
Generate Detailed Reports from Your Qualitative Data in Minutes.
Transcription Accuracy and Clarity Metrics Analysis
Transcription accuracy and clarity metrics analysis play a pivotal role in understanding the effectiveness of conversational voice data. By focusing on how accurately voice recordings translate into text, we can identify discrepancies and improve the overall clarity of conversations. Transcription accuracy is measured through error rate analysis, where the word error rate (WER) reveals the percentage of incorrect words in a transcript, subsequently affecting the comprehensibility of the dialogue.
In addition to error rates, semantic coherence enhances our understanding of how conversational elements connect meaningfully. Evaluating semantic relationships ensures that spoken dialogue maintains relevance and coherence, making it easier for listeners to follow along. By integrating both transcription accuracy and clarity metrics, organizations can refine their communication strategies, enhancing user experience and promoting clearer interactions. This analysis serves as a significant step toward achieving more effective and understandable voice data communication.
- Error Rate Analysis
Error Rate Analysis is essential for quantifying the accuracy of voice data and its impact on conversational clarity. By examining the word error rates (WER), organizations can identify patterns of miscommunication and determine which aspects of the conversation may be unclear. The calculations involve comparing the number of errors in transcriptions against the total number of words spoken, offering invaluable insights into the quality of the conversation.
Furthermore, a low error rate signifies higher clarity, which can significantly enhance user experience. When users can easily understand each other without confusion, their interactions become more productive and efficient. Being aware of the error rates allows teams to pinpoint areas needing improvement, refine their dialogue strategies, and ultimately foster better communication. By focusing on Error Rate Analysis, we can create clearer and more effective conversations that benefit all parties involved in voice data interactions.
- Methods for calculating word error rates (WER).
Calculating the word error rate (WER) is pivotal in clarity metrics analysis as it quantifies the accuracy of transcribed speech. WER is determined by comparing the number of errors in a transcription against the total number of words in the original speech. The formula for WER is simple: it involves counting substitutions, deletions, and insertions necessary to match the transcribed text with the original. High WER indicates poor clarity, while a lower value suggests effective communication.
To delve deeper, practitioners often rely on specific methodologies for accurate WER calculations. First, they meticulously align the reference text and transcription to pinpoint differences. Second, incorporating various contextual factors, such as tone and speech rate, enhances insights into conversational clarity. Tracking these error rates is vital for improving voice recognition systems and assessing overall communication effectiveness in conversational settings.
- Impact of lower error rates on conversational clarity.
Lower error rates in conversational analysis play a pivotal role in enhancing clarity. When the error rate decreases, the accuracy of transcriptions significantly improves, allowing nuances and context to shine through more clearly. This improved understanding fosters better interactions, as users can trust the system to accurately capture their dialogues, leading to more effective communication.
In Clarity Metrics Analysis, it becomes evident that a sound error rate analysis directly influences user engagement and satisfaction. Conversations become less punctuated by misunderstanding, reducing frustration and confusion in exchanges. Consequently, organizations can benefit from a higher level of service quality and efficiency, as representatives can focus on content rather than correcting errors. Enhanced clarity ultimately leads to richer, more meaningful interactions, underscoring the importance of consistently striving for reduced error rates in voice data analysis.
- Semantic Coherence
Semantic coherence is essential in determining the clarity of conversations in voice data. It examines how well the dialogue flows, revealing the relationships between different segments of speech. Effective communication hinges on logical connections and relevant exchanges, which significantly impact understanding and engagement.
To achieve a comprehensive Clarity Metrics Analysis, it is vital to focus on specific aspects of semantic coherence. First, identifying the presence of coherent themes throughout the conversation ensures that the messages conveyed resonate well with listeners. Secondly, evaluating the relevance of responses in the dialogue framework helps in assessing whether each speaker's contribution aligns with the ongoing topic. Finally, analyzing transitions between ideas provides insights into how smoothly speakers move from one point to another. These elements collaboratively offer a clearer understanding of conversational clarity, guiding improvements in communication strategies.
- Understanding semantic relationships in conversation.
Understanding semantic relationships in conversation is crucial for enhancing clarity in voice data. This understanding helps reveal how different concepts within dialogues relate to one another, which is vital for effective communication. By analyzing these relationships, we can assess whether conversations are coherent and whether the intended messages are conveyed clearly to the listener.
In conversational clarity metrics analysis, evaluating semantic coherence is a key aspect. It scrutinizes how well the topics and ideas flow, ensuring that the conversation remains relevant and understandable. This analysis often involves examining the context of statements and the relationships between them, thereby identifying areas where confusion might arise. Ultimately, grasping these semantic connections plays a significant role in refining communication strategies, aiding in the delivery of clearer messages tailored to diverse audience needs.
- Evaluating coherence and relevance in dialogues.
Evaluating coherence and relevance in dialogues involves a systematic approach to understanding how conversations unfold and the meanings they convey. Effective dialogues should not only flow smoothly but also address the needs and topics relevant to the participants. This importance stems from the necessity of clarity metrics analysis, which seeks to identify the quality and efficiency of communication within voice data.
To assess coherence and relevance, two primary criteria can be considered: semantic coherence and contextual relevance. Semantic coherence refers to how well the meanings of the words used connect and relate to each other throughout the dialogue. A conversation filled with well-structured ideas lends itself to deeper understanding. Contextual relevance, meanwhile, examines whether the topics discussed align with the interests and needs of the dialogue participants. By analyzing these factors, one can better appreciate conversational clarity and its implications for various applications, including customer service and team collaborations.
Tools for Clarity Metrics Analysis in Voice Data
Analyzing clarity metrics in voice data is essential for understanding conversational quality. A variety of tools exist to assist researchers and analysts in this vital task. Each tool offers unique functionalities and focuses on different aspects of clarity. For anyone exploring clarity metrics analysis, becoming familiar with leading technologies is a key step.
1. Insight7 is a powerful platform for clarity analysis. It allows users to upload and transcribe voice data efficiently, enabling quick extraction of insights. Features like bulk analysis and customizable templates support users in filtering information and identifying pain points in conversations.
2. Google Cloud Speech-to-Text provides robust functionalities aimed at enhancing transcription accuracy and clarity. Its capability to handle diverse accents and dialects makes it versatile for various applications.
3. IBM Watson Speech to Text is known for its advanced natural language processing capabilities. It enhances clarity evaluation by providing insights into context and intent, crucial for deeper analysis.
4. Microsoft Azure Speech Services offers features specifically designed to assess voice data clarity. Its integration capabilities allow organizations to streamline clarity assessment within existing workflows.
These tools can significantly aid in clarity metrics analysis, leading to improved conversational effectiveness.
- insight7
Understanding insight7 reflects the growing need for effective methods to analyze conversational clarity in voice data. This section delves into the framework of Clarity Metrics Analysis, which is essential for interpreting spoken interactions in a meaningful way. As organizations generate vast amounts of customer conversations, the challenge remains to extract actionable insights from this data swiftly and accurately.
Key aspects of this process include the identification of critical metrics that contribute to overall clarity. These can be broken down into several focal areas: assessing acoustic features, evaluating transcription accuracy, and utilizing state-of-the-art tools. For example, methods like Signal-to-Noise Ratio can reveal how clearly audio is presented, while Error Rate Analysis helps determine the precision of transcriptions. By leveraging such metrics, businesses can create a roadmap to enhance their customer interactions, ensuring they remain competitive in a rapidly evolving market.
- Features and capabilities for clarity analysis.
In today's data-driven landscape, clarity analysis has become crucial for understanding voice interactions. The features and capabilities for clarity analysis provide essential insights into the effectiveness of communication. By employing various clarity metrics, businesses can decode conversational nuances that impact customer experiences. A strong clarity analysis framework focuses on both acoustic and linguistic factors, ensuring a comprehensive evaluation of voice data.
Key features of clarity analysis tools include transcription accuracy, automated sentiment detection, and keyword extraction. Acoustic features, like signal-to-noise ratio, help gauge audio clarity, while linguistic metrics, such as semantic coherence, assess contextual understanding. Tools like insight7 leverage user-friendly interfaces, enabling anyone to analyze numerous audio files seamlessly. By utilizing these capabilities, organizations can foster deeper engagement, identify customer pain points, and ultimately enhance their communication strategies.
- Unique advantages for measuring conversational clarity.
Assessing conversational clarity provides unique advantages that can significantly enhance understanding and communication. The application of Clarity Metrics Analysis allows organizations to gain insights into how clearly ideas are articulated in conversations. This, in turn, helps identify potential areas requiring improvement, whether in training customer service representatives or refining communication protocols. With advancements in technology, measuring these aspects has never been easier, leading to improved engagement in conversations.
One of the core advantages is the ability to quantify clarity through specific metrics. Metrics such as Signal-to-Noise Ratio and transcription accuracy can provide actionable data that influences training and strategy. Evaluating these elements not only helps in ensuring better customer interactions but also strengthens the overall communication framework within an organization. By harnessing these metrics, organizations can better align their approaches to enhance conversational clarity and drive success.
- Google Cloud Speech-to-Text
Google Cloud Speech-to-Text offers advanced functionalities for transcription and analysis of audio data, significantly enhancing clarity metrics analysis. By converting spoken language into text, it enables businesses to assess conversational clarity through various metrics effectively. The platform's ability to transcribe recordings ensures that organizations can analyze multiple audio files quickly and efficiently, generating clear insights from dialogues.
Utilizing features like bulk transcription and customizable analysis templates simplifies the evaluation process. Users can easily select specific insights related to clarity, such as identifying common pain points within the conversation. The platform further allows for summary features that highlight key terms and phrases, assuring comprehensive understanding and relevance in discussions. By adopting such tools, organizations can effectively gauge the clarity of their voice data, ultimately improving their overall communication strategies.
- Overview of functionalities.
An understanding of the functionalities available for Clarity Metrics Analysis in voice data is paramount for assessing conversational clarity. Various tools and technologies provide unique capabilities that enhance the clarity measurement process. These functionalities enable users to monitor interactions and extract valuable insights from voice data, leading to improved customer service and marketing strategies.
For instance, tools like Google Cloud Speech-to-Text offer robust transcription services, ensuring that conversations are accurately converted into text. This accuracy is crucial as it directly influences the analysis of conversation dynamics. Additionally, platforms such as IBM Watson Speech to Text provide functionalities that assess tone and emotion, further enriching clarity metrics. Understanding these features helps organizations tailor their customer interactions and refine their response strategies. Thus, leveraging these functionalities can significantly enhance both the quality and effectiveness of communication in business contexts.
- Applications in assessing voice data clarity.
Applications in assessing voice data clarity involve a balanced use of various clarity metrics that can be effectively analyzed to enhance conversational quality. This comprehensive evaluation incorporates both acoustic features and linguistic metrics to provide actionable insights. Utilizing methods such as Signal-to-Noise Ratio (SNR) analysis can quantify sound clarity, enabling quick identification of potential issues in communication quality.
Additionally, the interpretation of transcription accuracy plays a vital role in assessing clarity. Analyzing metrics such as word error rate (WER) ensures an understanding of how accurately spoken words are captured and interpreted. Furthermore, evaluating semantic coherence allows us to gauge how well conversational elements connect with one another. By applying clarity metrics analysis, organizations can tailor their training and coaching strategies for improved communication outcomes, ultimately leading to higher customer satisfaction and engagement.
- IBM Watson Speech to Text
The service aims to improve clarity in voice data through a seamless transcription process. Users can upload multiple audio files, significantly enhancing efficiency when analyzing large volumes of conversation. Once the files are processed, it generates transcripts that become the basis for clarity metrics analysis.
This tool emphasizes various features that facilitate insights extraction, such as summarization capabilities and keyword identification. Users can easily select insights related to pain points and customer feedback, making it ideal for understanding conversational dynamics. Moreover, it supports the exploration of connections within dialogue, allowing for a deeper analysis beyond just transcription. This combination of powerful features and user-centric design provides an effective way to enhance clarity and enrich conversations.
- Key features for clarity evaluation.
Key features for clarity evaluation focus on understanding the elements that contribute to effective communication in voice data. When evaluating clarity, it's crucial to consider specific metrics that impact listeners' comprehension. These metrics can reveal how effectively information is communicated and understood, allowing for a comprehensive clarity metrics analysis.
Firstly, signal-to-noise ratio (SNR) is essential, as it measures the clarity of the audio signal in relation to background noise. Higher SNR values correlate with clearer audio, which enhances understanding. Secondly, transcription accuracy directly influences the clarity assessment, whereby evaluating the word error rate enables us to gauge the effectiveness of the spoken content. Additionally, semantic coherence needs to be examined as it reflects the logical flow of conversation, ensuring that dialogues remain relevant and comprehensible. Each of these features plays a pivotal role in forming a complete clarity evaluation.
- Success stories and use cases.
Success stories and use cases illustrate the practical applications of clarity metrics analysis in voice data, showcasing how organizations enhance customer interactions. By adopting advanced tools, businesses have identified actionable insights that significantly elevate conversational clarity. For instance, one company streamlined its customer support by implementing real-time speech analysis, reducing average response times and improving customer satisfaction scores.
Moreover, another initiative demonstrated the value of transcribing conversations to uncover hidden pain points. By analyzing the transcripts, the organization could identify common customer complaints, leading to targeted improvements in products and services. These success stories reveal that clarity metrics analysis not only transforms voice data into valuable insights but also fosters a more responsive and customer-centric approach. By embracing these use cases, businesses become better equipped to meet the evolving needs of their clientele, ensuring more meaningful and effective communication.
- Microsoft Azure Speech Services
Microsoft Azure Speech Services is an advanced platform designed for voice data processing, focusing on enhancing clarity metrics analysis. Its capabilities include transcription, where spoken language is converted into written text. This essential function allows organizations to analyze conversations and assess the quality of communication effectively. The platform is particularly known for its high level of accuracy, which plays a crucial role in the analysis of conversational clarity.
Additionally, Azure provides features for linguistic analysis, making it easier to evaluate dialogues' relevance and coherence. By integrating this service into your analytics workflow, you can gain valuable insights into customer interactions. Moreover, the ease of integration with various applications facilitates the seamless incorporation of clarity metrics into your existing systems. Overall, these capabilities make this service an invaluable tool for organizations aiming to improve their conversational clarity and customer engagement strategies.
- Highlighting clarity-focused features.
Clarity-focused features play a pivotal role in enhancing the effectiveness of voice data analysis. Recognizing these features helps identify how well conversations are understood. Key components include tools that measure elements like Signal-to-Noise Ratio (SNR), which assesses the strength of a voice signal against background noise. A higher SNR often indicates clearer audio, contributing to improved clarity perception. Additionally, spectral clarity, which involves analyzing the frequency components of a sound, informs how distinct words and phrases are perceived during discussions.
Moreover, linguistic metrics, such as error rates from transcripts and semantic coherence, also provide valuable insights. Analyzing the consistency of dialogue helps reveal whether messages are conveyed effectively and understood accurately. These clarity-focused features, integral to Clarity Metrics Analysis, ensure that conversational quality and listener engagement are optimized, ultimately leading to more insightful interactions in various settings.
- Integration strategies for clarity assessment.
Integration strategies for clarity assessment can significantly enhance the analysis of conversational clarity in voice data. By implementing a structured approach, organizations can ensure effective assessment and interpretation of clarity metrics. First, it is essential to identify the relevant acoustic and linguistic features that impact clarity. For instance, focusing on signal-to-noise ratio and transcription accuracy can provide insights into the overall quality of voice data, as these metrics reflect the clarity of communication.
Next, integrating advanced tools designed for clarity metrics analysis is critical. Utilizing platforms like IBM Watson Speech to Text or Google Cloud Speech-to-Text can streamline the data evaluation process. These tools not only assist in capturing voice nuances but also facilitate the analysis of semantic coherence within conversations. By leveraging technology to assess clarity more accurately, organizations can foster improved interactions and enhance customer experiences through data-driven insights. This multi-faceted approach ensures that clarity assessment is both thorough and effective, leading to actionable outcomes.
Conclusion on Clarity Metrics Analysis in Voice Data
In conclusion, clarity metrics analysis plays a crucial role in evaluating the effectiveness of conversational interactions in voice data. By employing acoustic and linguistic metrics, one can ascertain how well communication is being perceived. Key indicators such as signal-to-noise ratio and transcription accuracy serve as benchmarks for determining clarity levels, enabling businesses to adjust their approaches accordingly.
Moreover, utilizing sophisticated tools for analysis bolsters the ability to refine conversations. With accurate data feedback, organizations can transition from a transactional mindset to a more consultative approach. Ultimately, a thorough clarity metrics analysis not only enhances customer experience but also guides ongoing improvements in communication strategies.