What’s the Difference Between Sentiment Score and Emotion Index?
-
Bella Williams
- 10 min read
Understanding the distinctions between sentiment and emotion is essential for effective communication and analysis. When we delve into sentiment vs. emotion, we encounter two concepts that, while interconnected, serve different purposes in interpreting human responses. Sentiment generally refers to a broader opinion or attitude expressed in a statement, while emotion deals with specific feelings that may drive those sentiments.
Recognizing these differences enables individuals and businesses to leverage insights more effectively. Sentiment scores can measure the overall positivity or negativity of a collection of comments, whereas an emotion index captures a range of feelings such as joy, anger, or sadness. Understanding these fundamentals lays the groundwork for deeper exploration into their applications and implications for decision-making.
Transcribe & extract insights from interviews. At Scale.
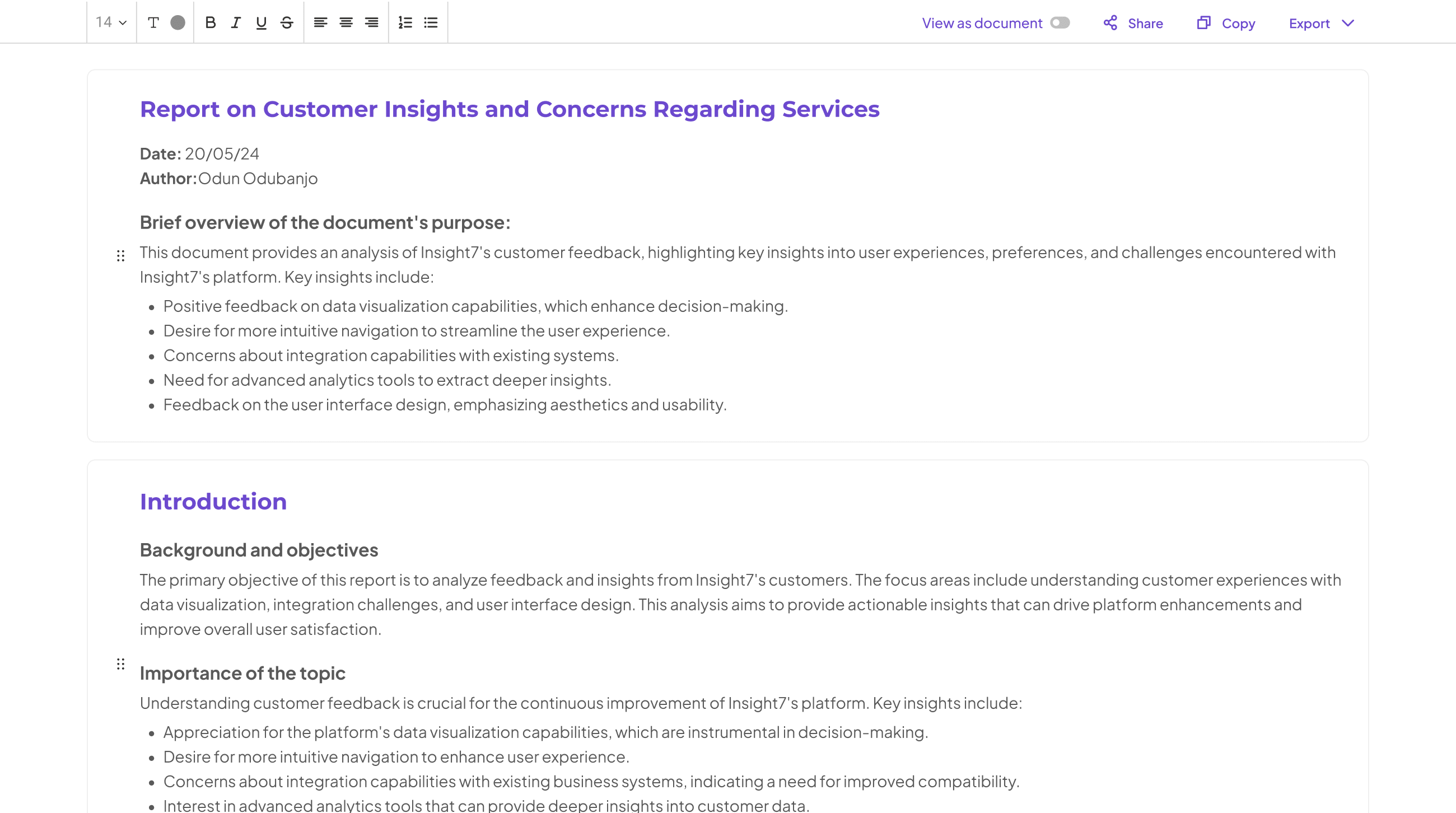
Sentiment vs. Emotion: Key Differences
Sentiment vs. Emotion highlights significant distinctions in analyzing human experiences. Sentiment refers to a general attitude, often categorized as positive, negative, or neutral. It provides a broad understanding of public opinion based on textual input, such as reviews or social media mentions. Conversely, emotion delves deeper into specific feelings like joy, anger, or sadness. This level of analysis reveals the nuances behind a person's emotional response to content or experiences.
Understanding these differences is crucial for interpreting data effectively. Sentiment scores aggregate the overall mood, while an emotion index uncovers the intricate emotional layers present in communication. Each approach serves distinct purposes, allowing businesses to tailor their strategies accordingly. For instance, while sentiment analysis guides decisions based on public perception, emotion tracking helps enhance customer engagement through tailored experiences. By recognizing sentiment vs. emotion, organizations can better navigate customer insights and remain competitive in their markets.
Defining Sentiment Score
Sentiment analysis plays a crucial role in understanding customer feelings toward products or services. It involves assessing textual data to assign a numerical sentiment score, typically ranging from negative to positive. The process is rooted in natural language processing and machine learning, relying on algorithms that analyze the context of words within a sentence. This score provides insights into overall customer sentiment, helping businesses gauge public perception and make data-informed decisions.
To calculate sentiment scores, various factors are considered. These include keyword sentiment, sentence structure, and contextual meaning. To put it simply, sentiment scores transform qualitative emotions into quantitative data, enabling companies to understand how their audience feels at a glance. By contrast, while sentiment focuses on a general perception, emotion indexing dives deeper into specific feelings, revealing a broader emotional landscape. Understanding the distinction between Sentiment vs. Emotion is essential for effective market analysis.
- Explanation of sentiment analysis.
Sentiment analysis involves interpreting and classifying the emotional tone behind words to gain an understanding of the attitudes, opinions, and emotions expressed in text. This process is critical for businesses to identify customer sentiments toward products, services, or brands. Through various algorithms, sentiment analysis can categorize feedback as positive, negative, or neutral, providing essential insights into customer experiences and preferences.
The primary goal of sentiment analysis is to distinguish between feelings and emotions that individuals convey in their communication. While sentiment scores offer quick assessments of overall sentiment, emotion indexes dive deeper, revealing the specific emotions present in the text. By understanding the subtleties between sentiment vs. emotion, organizations can tailor their strategies to better engage with their audience and respond to their needs effectively. This differentiation empowers businesses to make more informed decisions and foster customer relationships.
- How sentiment scores are calculated.
Calculating sentiment scores involves several systematic steps to assess the emotional tone behind a text. Initially, the process begins with the collection of data, often sourced from customer reviews, social media posts, or feedback surveys. This data is then processed using Natural Language Processing (NLP) techniques that identify key linguistic features.
Next, algorithms analyze these features to assign numerical values to sentiments expressed within the text. Typically, scores are scaled on a range, with positive values indicating favorable sentiment, negative values representing unfavorable sentiment, and neutral values signaling ambivalence. Various factors, such as context and specific keywords, influence these scores significantly. Using sentiment scores helps differentiate between sentiment vs. emotion by providing clear, quantifiable insights into customer opinions and attitudes. Understanding these calculations not only aids businesses in refining their strategies but also enhances overall customer engagement.
Exploring Emotion Index
Understanding the Emotion Index sheds light on the complexity beyond the basic sentiment score. While sentiment scores provide a general impression—positive, negative, or neutral—emotion indexes delve deeper into the nuanced feelings expressed in the data. The Emotion Index categorizes emotions into various types such as joy, sadness, anger, and fear, allowing for a more refined analysis of user sentiment.
To explore the Emotion Index effectively, consider these key aspects:
- Granularity of Emotion: Unlike sentiment scores, emotion indexes identify specific emotions, offering more insight into customer experiences.
- Contextual Understanding: The Emotion Index evaluates the context behind emotions, enhancing the analysis of user reactions.
- Application in Product Development: Emotion analysis can guide product enhancements based on consumer feelings, addressing their concerns or desires directly.
This differentiation becomes crucial when companies seek to connect more meaningfully with their audiences, making the insight derived from emotional analysis invaluable.
- Explanation of emotion analysis.
Emotion analysis plays a crucial role in distinguishing the nuanced feelings expressed in text data. Unlike sentiment analysis, which categorizes reviews and comments as positive, negative, or neutral, emotion analysis goes deeper. It identifies a broader range of emotions such as joy, anger, sadness, and fear, allowing for a more comprehensive understanding of customer sentiments.
To conduct emotion analysis effectively, one might follow several essential steps. First, data collection involves gathering customer feedback, social media interactions, or survey responses. Next, data preprocessing is necessary to clean and prepare the data for analysis. Finally, advanced machine learning algorithms or natural language processing techniques are employed to analyze the data and assign specific emotions to the text. By focusing on the nuances of emotions, businesses can better understand and address customer needs, enhancing their overall experience. This is a significant consideration in the ongoing discussion of sentiment vs. emotion in data analysis.
- How emotion indexes are derived.
The derivation of emotion indexes involves a nuanced analysis that goes beyond basic sentiment evaluation. To create these indexes, algorithms typically begin by identifying emotional cues within the text, which are known as linguistic indicators. These cues are analyzed to classify emotions such as joy, anger, fear, or sadness. This classification is based on pre-established emotional lexicons or databases that associate specific words or phrases with particular emotions.
Next, the intensity of these emotions is assessed by weighing the occurrence of these indicators. By applying various statistical techniques, the resulting scores are then normalized to produce an emotion index. This process allows for a more intricate understanding of emotional responses compared to a simple sentiment score, making it crucial for businesses aiming to understand customer feelings. Thus, distinguishing between sentiment and emotion is essential for generating actionable insights from emotional data.
Generate Detailed Reports from Your Qualitative Data in Minutes.
Tools for Analyzing Sentiment vs. Emotion
Analyzing sentiment vs. emotion requires specific tools to understand the nuances behind customer feedback. The primary distinction between sentiment analysis and emotion detection lies in how they interpret data. Sentiment analysis generally categorizes text as positive, negative, or neutral. In contrast, emotion analysis digs deeper, identifying specific feelings like joy, anger, or sadness.
💬 Questions about What’s the Difference Between Sentiment Score and Emotion Index??
Our team typically responds within minutes
Several tools excel at differentiating sentiment from emotion. For sentiment analysis, popular tools include social media monitoring platforms that aggregate customer feedback and provide sentiment scores. These scores reveal overall customer perceptions, guiding businesses in strategizing their market approach. On the other hand, specialized emotion detection tools can analyze nuances in tone and context, offering a richer understanding of customer experiences. By using these tools effectively, businesses can craft targeted strategies to address customer needs, ultimately improving engagement and satisfaction.
Top Tools for Sentiment Analysis
When choosing the right tools for sentiment analysis, it's essential to understand how these technologies differentiate sentiment from emotion. The landscape of sentiment analysis is populated by various tools that each bring unique capabilities to the table. Some tools focus on real-time data processing, while others emphasize accuracy and depth of analysis. Users should evaluate tools based on their specific needs, whether that is monitoring brand sentiment, analyzing customer reviews, or tracking social media conversations.
Among the top tools for sentiment analysis are those that utilize machine learning algorithms to provide nuanced insights into customer attitudes. For instance, Tool 1 excels in processing vast data sets, enabling users to capture both positive and negative sentiments efficiently. Tool 2, on the other hand, offers advanced features that allow users to visualize sentiment trends over time. These tools not only enhance the ability to differentiate between sentiment and emotion but also empower users to make informed decisions based on the data analysis.
- insight7
Understanding insight7 involves recognizing the nuances between sentiment and emotion in data analysis. While sentiment scores focus primarily on the positive or negative tone expressed in text, the emotion index digs deeper into the specific feelings conveyed, such as joy, anger, or sadness. This differentiation is essential because it informs how businesses interpret customer feedback and shape their strategies accordingly.
To truly grasp the impact of this distinction, consider these key points:
- Purpose of Analysis: Sentiment scores are often used for quick assessments of customer perspectives, while emotion indexes provide a rich, detailed framework for understanding customer motivations.
- Data Interpretation: Accurate sentiment analysis can identify trends in customer satisfaction, but emotion detection reveals the underlying emotional context behind those sentiments.
- Actionable Insights: Organizations may implement strategies based on sentiment alone, but failing to account for specific emotions may lead to missed opportunities for deeper engagement with their customers.
In summary, both sentiment scores and emotion indexes serve valuable roles, but aligning the right approach with specific business goals is crucial for success.
- Sentiment Analysis Tool 1
Sentiment Analysis Tool 1 provides a robust framework for understanding consumer opinions through sentiment scores. This tool focuses primarily on gauging the positive or negative sentiment expressed in various texts, such as customer reviews or social media posts. By assessing these sentiments, businesses can derive valuable insights into how their products or services are perceived, aiding in decision-making and strategy formulation.
In contrasting this tool with an emotion index, it's essential to note that while sentiment analysis captures the overall positive or negative feelings, an emotion index delves deeper into specific emotional states like joy, anger, or sadness. This nuanced understanding enhances the analysis, enabling businesses to tailor their approach based on the specific emotional feedback received. Understanding the distinction between sentiment score and emotion index empowers marketers to refine their strategies more effectively, ultimately leading to improved customer satisfaction and loyalty.
- Sentiment Analysis Tool 2
Sentiment Analysis Tool 2 provides a robust framework for understanding customer feedback by focusing on the nuances of sentiment vs. emotion. This tool allows businesses to analyze reviews, survey responses, and social media interactions to quantify feelings expressed by customers. By categorizing comments as positive, negative, or neutral, users can gain valuable insights which help in product development and marketing strategies.
Utilizing sophisticated algorithms, Sentiment Analysis Tool 2 can visualize data trends, enabling companies to discern patterns in customer feedback over time. Users can extract actionable insights by identifying recurring themes, allowing them to respond to customer sentiments effectively. This is crucial, as understanding sentiment alongside emotional context offers a comprehensive view of customer perspectives. Overall, Tool 2 enhances the ability to engage with customers meaningfully and can significantly impact decision-making processes.
Leading Tools for Emotion Detection
When it comes to understanding emotional nuances in text, various tools stand out for their effectiveness in emotion detection. These tools help analyze not just the overall sentiment but also the specific emotions expressed, creating a richer picture of user feedback. Among the leading tools are those specifically designed to parse complex emotional responses, offering insights into feelings such as joy, sadness, anger, and fear.
For instance, Emotion Detection Tool 1 utilizes machine learning to classify emotional content by analyzing word choice and context. It can effectively distinguish between nuanced emotions, differentiating, for example, between frustration and anger. Likewise, Emotion Detection Tool 2 employs advanced algorithms to decode emotional subtext in customer interactions, allowing businesses to tailor their responses more effectively. Utilizing these tools is crucial for a comprehensive understanding, where sentiment vs. emotion comes into play, ensuring that businesses can connect meaningfully with their audience.
- Emotion Detection Tool 1
Emotion Detection Tool 1 utilizes advanced algorithms to identify and analyze emotions expressed in text, allowing businesses to gain deeper insights. While sentiment analysis generally categorizes opinions as positive, negative, or neutral, the Emotion Detection Tool dives much deeper into human feelings. This tool often recognizes a range of emotions such as joy, anger, sadness, fear, and surprise, providing a comprehensive view of customer sentiment.
In the context of "Sentiment vs. Emotion," it is vital to understand that while sentiment scores give an overall impression, the emotion index reveals the underlying feelings that drive those impressions. This distinction is crucial for businesses aiming to improve customer experiences. By using Emotion Detection Tool 1, organizations can tailor their strategies based on precise emotional data, resulting in more effective engagement and communication. Ultimately, harnessing this technology helps bridge the gap between what customers say and what they truly feel.
- Emotion Detection Tool 2
Emotion Detection Tool 2 plays a crucial role in distinguishing human emotional responses from mere sentiment scores. While sentiment might capture a general attitude, an emotional detection tool digs deeper into specific feelings like joy, anger, or sadness. This nuanced insight allows businesses to tailor their strategies effectively, transforming generic feedback into actionable intelligence.
Understanding the functionality of Emotion Detection Tool 2 is essential. Its algorithms analyze language patterns, tone, and context, providing a multi-dimensional view of customer sentiment. This contrasts with traditional sentiment analysis, which may overlook the richness of human emotion. By utilizing such tools, companies can enhance user experience and engagement by understanding the emotional drivers behind feedback. Ultimately, integrating these insights not only enriches customer interactions but also sharpens overall brand perception.
Conclusion: Choosing Between Sentiment Score and Emotion Index
When deciding between sentiment score and emotion index, it is essential to comprehend their unique functions. Sentiment scores quantify overall attitudes, reflecting positive or negative perceptions. They are straightforward and excellent for basic sentiment analysis. In contrast, the emotion index delves deeper, categorizing the specific emotions behind sentiments. This allows for a richer understanding of customer feelings, which can enhance emotional engagement.
In practical applications, the choice between sentiment versus emotion depends on your objectives. If you seek general feedback or trends, a sentiment score suffices. However, if your goal is to grasp the nuances of customer experiences, opt for an emotion index to capture the full emotional spectrum. Ultimately, the right tool aids your analysis and drives meaningful insights.
💬 Questions about What’s the Difference Between Sentiment Score and Emotion Index??
Our team typically responds within minutes