What Is the Role of Sentiment AI in QA Evaluation?
-
Bella Williams
- 10 min read
Sentiment Analysis Integration plays a crucial role in enhancing quality assurance (QA) evaluations. By analyzing customer feedback and interactions, organizations can derive meaningful insights into user experiences, identifying strengths and weaknesses in their services. This integration fosters a deeper understanding of customer attitudes and emotions, enabling teams to refine their approaches and address issues more effectively.
Incorporating sentiment analysis into QA processes transforms raw data into actionable insights. It empowers evaluators to assess calls and communications against predefined standards while also capturing not just what customers say, but how they feel. Consequently, businesses can align their strategies to improve user satisfaction and ensure compliance with quality metrics, setting a benchmark for excellence in customer service.
Transcribe & extract insights from interviews. At Scale.
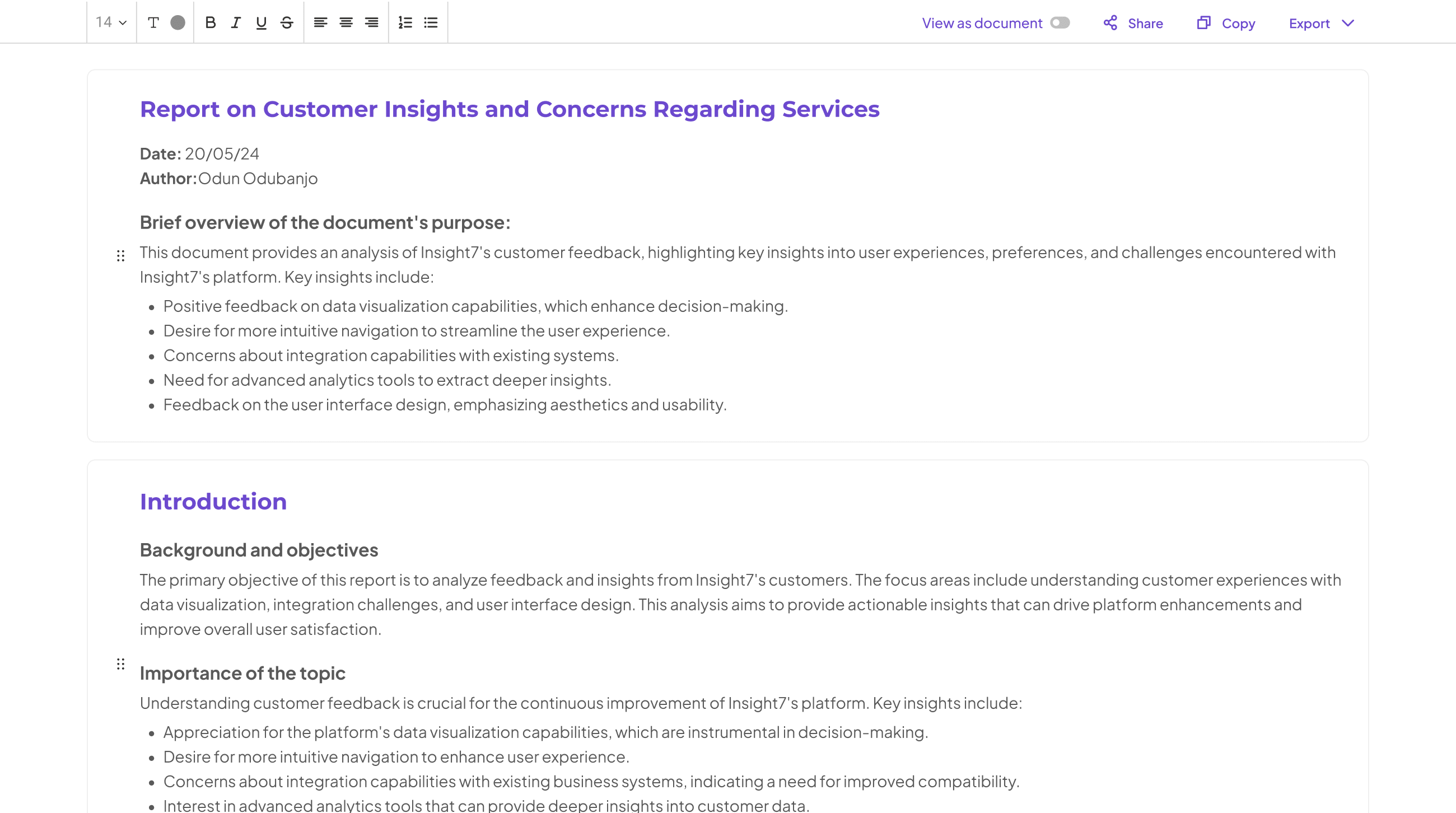
Understanding Sentiment Analysis Integration in Quality Assurance
Sentiment analysis integration plays a crucial role in enhancing quality assurance (QA) evaluations. By incorporating sentiment analysis into QA processes, organizations can gauge customer emotions and attitudes more accurately. This integration allows for a deeper understanding of customer interactions and experiences, leading to improved service and product quality.
In practice, the integration requires defining clear metrics that highlight customer sentiment. Key factors such as tone, satisfaction levels, and emotional responses are critical. Once identified, selecting suitable sentiment analysis tools is essential for effectively analyzing data. These tools process large volumes of feedback, providing insights that can drive continuous improvement. With this approach, businesses not only address immediate concerns but also anticipate future needs, significantly elevating customer satisfaction and loyalty.
Ultimately, sentiment analysis integration is pivotal in transforming data into actionable insights, thereby enhancing the overall quality assurance framework.
The Basics of Sentiment AI
Sentiment Analysis Integration helps organizations understand the emotional tone of interactions, especially during the quality assurance (QA) evaluation process. By detecting positive, negative, or neutral sentiments in customer interactions, businesses gain insights into user experiences and agent performances. This foundational aspect of Sentiment AI not only enhances the evaluation process but also informs strategies for improvement.
Implementing Sentiment AI in QA begins with data collection from various sources such as customer feedback, recordings, or chat transcripts. Next, algorithms analyze these inputs to identify emotional cues and trends related to customer satisfaction. This integration not only provides real-time feedback on service interactions but also supports teams in pinpointing specific areas for enhancement, thereby streamlining training and operational adjustments. By embracing Sentiment Analysis Integration, organizations can cultivate a deeper understanding of their clients, leading to more effective quality assurance practices.
- Definition and Overview
Sentiment Analysis Integration refers to the application of artificial intelligence to evaluate emotions derived from user feedback, comments, or other forms of communication. This process revolves around understanding the sentiment behind textual data, which can include positive, negative, or neutral sentiments. In the context of Quality Assurance (QA), such integration proves invaluable as it allows organizations to gain insights directly from customer interactions, revealing their sentiments regarding products or services.
The primary goal of integrating sentiment analysis into QA is to enhance the evaluation process. By analyzing customer sentiments, businesses can identify areas needing improvement and understand the overall customer experience. This can lead to more informed decisions and strategies, ensuring that quality outputs align well with consumer expectations. Moreover, incorporating these insights helps organizations predict future trends and make modifications in real-time, significantly improving product quality and user satisfaction.
- How Sentiment AI is Implemented in QA
Sentiment analysis integration plays a crucial role in quality assurance by evaluating customer interactions. Implementing this technology allows organizations to quantify sentiment, offering insights into customer experiences and agent performances. First, data from customer interactions, such as calls or chats, is gathered. This data is then analyzed using sentiment AI, which detects positive, negative, or neutral sentiments through natural language processing.
Next, detailed reports are created that summarize agent performance and customer feedback. These reports highlight key areas for improvement and compliance issues, enabling organizations to identify training needs or adjust strategies effectively. Furthermore, this integration not only streamlines QA processes but also promotes a culture of continuous improvement based on real user emotions and experiences. By integrating sentiment analysis into quality assurance evaluations, companies can enhance customer satisfaction and drive operational excellence.
Importance of Sentiment Analysis Integration
Integrating sentiment analysis into quality assurance processes is vital for enhancing decision-making. By implementing sentiment analysis integration, businesses can transform raw data from customer interactions into actionable insights. This approach significantly improves user experience, as organizations gain a deeper understanding of customer emotions and feedback. Moreover, a continuous evaluation of sentiments allows for timely adjustments, enabling companies to respond effectively to emerging trends and issues.
The predictive capabilities of sentiment analysis integration also contribute to quality improvement. By analyzing patterns and shifts in sentiment over time, businesses can proactively identify areas in need of enhancement. Implementing a robust sentiment analysis framework empowers organizations to optimize their products and services based on customer perceptions. Ultimately, such integration fosters a more responsive and agile business culture, where quality assurance is informed by real-time emotional feedback.
- Enhancing User Experience
Integrating sentiment analysis into quality assurance processes significantly enhances the user experience. By analyzing customer interactions, organizations can gain insights into user emotions and preferences. This allows them to tailor products and services to better meet customer needs, creating a more personalized experience. Improved feedback mechanisms can help identify pain points, enabling teams to address issues proactively.
Moreover, sentiment analysis integration supports ongoing quality improvements. By continuously monitoring sentiments expressed in user interactions, teams can refine their approach and align offerings with user expectations. This evolving understanding fosters stronger relationships between businesses and their customers, ultimately driving engagement and satisfaction. As organizations embrace this approach, they will strengthen their commitment to delivering exceptional user experiences and ensure continual growth.
- Predictive Insights for Quality Improvement
Predictive insights play a crucial role in quality improvement by providing organizations with a deeper understanding of customer sentiments. By integrating sentiment analysis, companies can transform raw data into actionable intelligence. This allows teams to identify trends and patterns that may be overlooked through traditional evaluation methods.
Implementing sentiment analysis empowers businesses to proactively address customer concerns and enhance service delivery. With the ability to analyze vast amounts of feedback, organizations can pinpoint specific areas requiring improvement. This data-driven approach not only improves customer satisfaction but also fosters a culture of continuous improvement. Ultimately, the integration of sentiment analysis in quality assurance leads to better decision-making and more effective strategies for meeting customer needs.
Generate Detailed Reports from Your Qualitative Data in Minutes.
Leveraging Sentiment Analysis Integration: Applications and Best Practices
Integrating sentiment analysis into quality assurance (QA) evaluation greatly enhances the understanding of user experiences. By systematically analyzing customer sentiment, organizations can uncover valuable insights that inspire actionable improvements. Applications of sentiment analysis span various areas, from evaluating customer support to optimizing product features, directly influencing satisfaction and brand loyalty.
To effectively employ sentiment analysis integration, consider the following best practices:
Identify Key Sentiment Metrics: Determine the specific metrics that align with your organization's goals, such as customer satisfaction scores or net promoter scores. This focus will guide your analysis.
Choose the Right Tools: Select sentiment analysis tools that fit your needs, ensuring ease of use and comprehensive features. Solutions like IBM Watson or MonkeyLearn provide robust options for different analysis requirements.
๐ฌ Questions about What Is the Role of Sentiment AI in QA Evaluation??
Our team typically responds within minutes
By employing these strategies, organizations can harness the full potential of sentiment analysis integration, transforming raw data into meaningful insights that drive continuous improvement in QA processes.
Steps to Implement Sentiment AI in QA Evaluation
To effectively implement Sentiment Analysis Integration in QA evaluation, follow a systematic approach that ensures a thorough understanding of customer sentiments. The initial step is to identify key sentiment metrics vital for your evaluation process. These may include customer satisfaction scores, sentiment polarity, and emotional tone. By focusing on these specific metrics, you can tailor your analysis to capture the most relevant feedback from your interactions.
Next, select the appropriate sentiment AI tools that align with your defined metrics. Consider tools that offer robust capabilities for transcription and data analysis, enabling seamless integration into your existing QA processes. Many tools also include customizable templates for evaluating various call types, enhancing compliance and quality assurance efforts. By combining the right metrics with effective tools, you will set a solid foundation for integrating sentiment analysis into your QA evaluations, thereby elevating your overall quality assurance framework.
- Step 1: Identify Key Sentiment Metrics
To effectively utilize Sentiment Analysis Integration in quality assurance evaluation, the first critical step is identifying key sentiment metrics. These metrics offer a structured way to assess customer feedback, highlighting both positive and negative sentiments. Understanding what to measure is essential for garnering valuable insights from the data collected during the QA process.
Start by focusing on specific metrics like sentiment score, customer satisfaction ratings, and net promoter score. Sentiment score indicates overall positivity or negativity in feedback, while customer satisfaction ratings provide direct insight into user experience. The net promoter score helps gauge customer loyalty and likelihood to recommend a product. By analyzing these metrics, organizations can uncover patterns related to product performance and user interactions, which in turn informs quality improvement initiatives. With clear metrics established, teams can more effectively implement data-driven enhancements to elevate their offerings.
- Step 2: Choose the Appropriate Sentiment AI Tools
Choosing the appropriate sentiment AI tools is a crucial step in effective sentiment analysis integration for quality assurance evaluations. The right tools can transform how you interpret customer feedback and enhance evaluation accuracy. Begin by identifying your specific needs, such as the types of data and sentiments you want to analyze. Make a list of potential tools that align with your requirements and assess their compatibility with existing systems.
Once you have shortlisted the tools, consider aspects such as ease of use, integration capabilities, and the level of analytics provided. It's essential to choose a tool that allows for clear sentiment categorization, enabling your team to derive actionable insights from the data collected. Ultimately, the selected sentiment AI tools should empower you to improve overall quality assurance processes, leading to enhanced customer experiences.
Top Sentiment Analysis Tools for QA Evaluation
In the growing field of quality assurance, effective sentiment analysis integration plays a vital role in improving user experience and product quality. Organizations are turning to advanced sentiment analysis tools to glean insights from customer feedback. These tools enable users to analyze both positive and negative sentiments expressed in vast data sets, guiding decisions and strategies for product enhancement.
Several top sentiment analysis tools have emerged as favorites for quality assurance evaluation. Insight7 offers comprehensive metrics and visualizations, allowing teams to dissect customer opinions effortlessly. MonkeyLearn provides powerful text analysis features, facilitating sentiment classification with ease. Lexalytics stands out for its linguistic intelligence, empowering businesses to understand customer emotions deeply. Aylien focuses on extracting key insights from news and social media, while IBM Watson Natural Language Understanding excels at interpreting complex language structures. Utilizing these tools significantly enhances the effectiveness of sentiment analysis integration, paving the way for improved QA processes.
- Insight7
Integrating sentiment analysis into quality assurance evaluation can greatly enhance the understanding of customer interactions. By utilizing sentiment analysis integration effectively, organizations can capture not just the feedback, but also the emotions behind the words of their customers. This deep understanding allows businesses to pinpoint specific pain points in their services or products, leading to clearer data-driven improvements.
One primary benefit of this integration is its capability to transform qualitative data into actionable insights. For instance, recognizing trends in customer sentiment can enable teams to address recurring issues before they escalate. Additionally, it helps forge a more engaged customer service framework, where proactive measures can be taken based on customer feedback. By analyzing the emotional context of interactions, QA teams can prioritize efforts and streamline processes to enhance overall user satisfaction and business outcomes.
- MonkeyLearn
MonkeyLearn stands out as a versatile tool in the realm of sentiment analysis integration, particularly for quality assurance evaluation. With its user-friendly interface, businesses can easily analyze customer feedback and interactions, extracting meaningful insights. This integration plays a crucial role in identifying trends and gathering data to enhance user experiences effectively.
The platform offers numerous features that allow companies to customize sentiment analysis according to their specific needs. Users can set key metrics to measure, ensuring that the evaluation aligns with overall quality expectations. Through its robust API, businesses can seamlessly incorporate sentiment analysis into their existing workflows. This capability empowers teams to adopt a data-driven approach, leading to improved decision-making processes. Ultimately, effective sentiment analysis integration not only streamlines QA evaluation but also fosters stronger customer relationships by proactively addressing concerns and adapting strategies accordingly.
- Lexalytics
In the realm of sentiment analysis integration, an effective platform plays a significant role in harnessing customer insights. By utilizing advanced algorithms, analysis tools can automatically extract key sentiments from large volumes of data, thus highlighting user experiences directly. This functionality offers companies a comprehensive view of customer feedback without requiring specialized teams to interpret the data.
Moreover, the integration enables businesses to respond promptly to evolving customer needs and preferences. Through features like call transcription and the visualization of conversations, actionable insights become readily available. These insights identify pain points and positive affirmations, driving improvements in quality assurance processes. Ultimately, such systems not only enhance user satisfaction but also guide strategic decisions within organizations seeking to optimize their operations. Embracing sentiment analysis integration allows companies to capture the voice of the customer effectively, fostering a more engaged and responsive business environment.
- Aylien
A powerful player in the realm of Sentiment Analysis Integration, Aylien offers advanced tools designed to refine quality assurance processes. By employing natural language processing and machine learning, Aylien enables users to dissect customer feedback in real time. This precise analysis helps teams gauge emotions and intentions behind customer interactions, providing nuanced insights that elevate service levels.
When integrated into QA evaluations, Aylien's capabilities extend beyond basic data interpretation. It allows organizations to identify sentiment trends, flag potential issues, and ultimately enhance user experience. By employing sentiment analysis, companies can make data-driven decisions that align their services with customer expectations, fostering stronger relationships and improving overall satisfaction. Utilizing such technology demonstrates a commitment to quality and continuous improvement in customer-facing interactions.
- IBM Watson Natural Language Understanding
IBM Watson Natural Language Understanding plays a significant role in transforming how sentiment AI is integrated into quality assurance evaluation. By analyzing text, audio, and even video content, this tool effectively identifies emotions and sentiments, providing valuable insights. Businesses can understand customer feedback more deeply and identify areas for improvement in their services.
The integration of sentiment analysis improves the quality evaluation process by pinpointing specific sentiments tied to customer interactions. For example, teams can analyze transcripts of support calls to gauge agent performance or customer satisfaction. This capability allows for more data-driven feedback and tailored strategies to enhance the quality of customer service. Moreover, such insights help organizations stay competitive in their markets, aligning quality assurance practices with overall customer satisfaction goals. Ultimately, using advanced sentiment analysis tools can considerably elevate the quality assurance process, leading to enhanced user experiences and deep insights.
Conclusion: The Future of Sentiment Analysis Integration in QA Evaluation
The evolution of sentiment analysis integration in quality assurance (QA) evaluation is poised to enhance the way organizations assess customer interactions. As companies increasingly rely on AI-driven tools, the integration of sentiment analysis will provide deeper insights into customer sentiment and behavior. This advancement will empower quality evaluators to identify potential areas for improvement and tailor their approaches accordingly.
Looking ahead, we can expect sentiment analysis integration to become more sophisticated. The use of advanced algorithms and machine learning will allow for a more nuanced understanding of emotional responses in customer interactions. This, in turn, could lead to more personalized customer experiences and improved service quality, significantly enhancing the overall effectiveness of quality assurance practices in the future.
๐ฌ Questions about What Is the Role of Sentiment AI in QA Evaluation??
Our team typically responds within minutes