What forecasting models can AI generate for contact centers?
-
Hello Insight
- 10 min read
AI Forecasting Models have emerged as transformative tools for contact centers, reshaping how businesses predict customer interactions and manage resources. As customer demands evolve, traditional forecasting methods can often fall short, leading to inefficiencies and missed opportunities. AI-powered models offer advanced analytics that can process vast amounts of data, allowing centers to anticipate call volume, customer needs, and operational challenges.
Understanding these models is essential for any contact center looking to enhance performance. They utilize machine learning and data analysis to generate accurate forecasts, ultimately improving customer satisfaction and operational efficiency. By incorporating AI Forecasting Models, organizations can not only streamline their processes but also create more engaging customer experiences.
Generate visualizations from your qualitative data. At Scale.
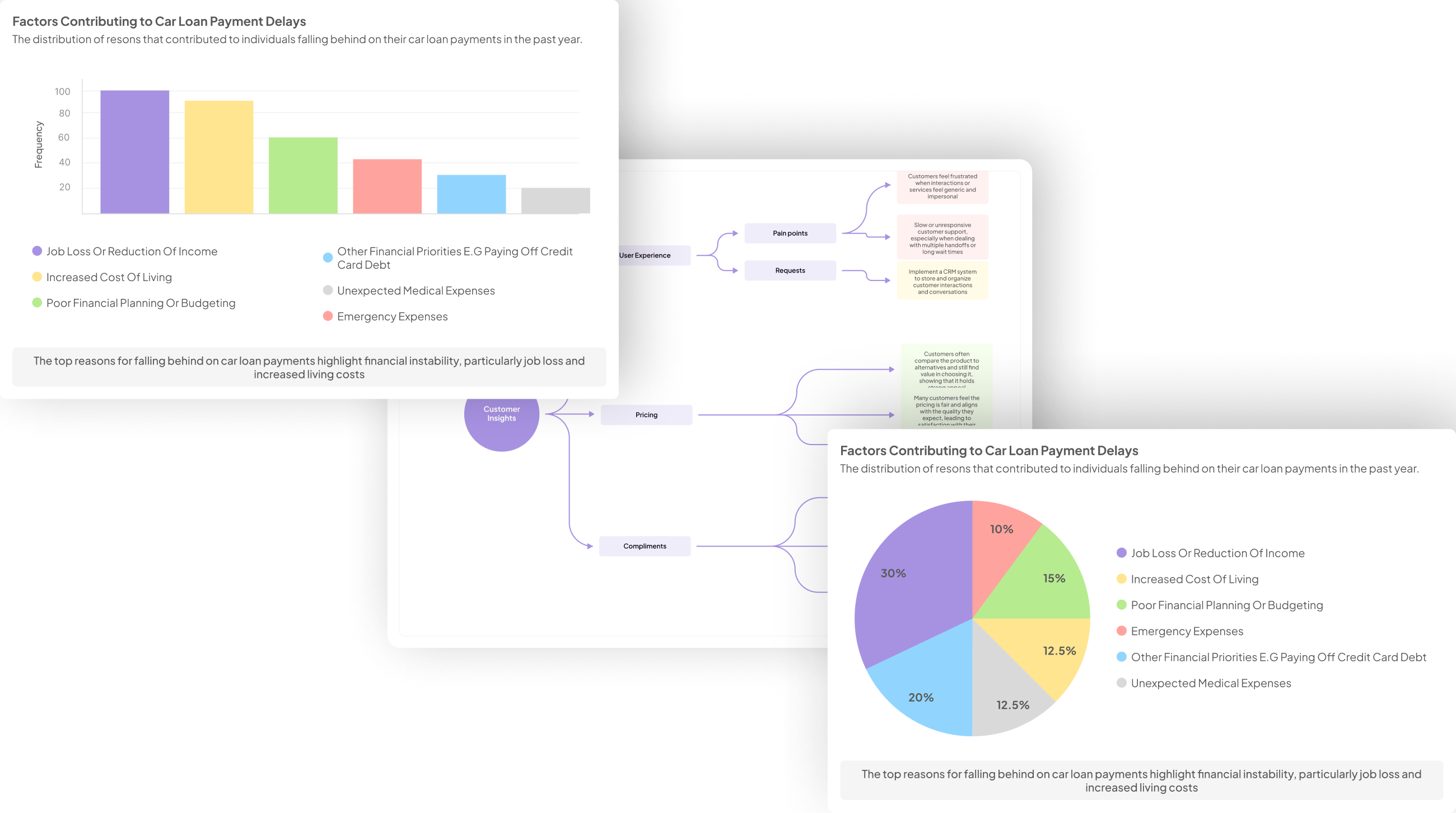
Understanding AI Forecasting Models in Contact Centers
AI Forecasting Models play a crucial role in enhancing the efficiency of contact centers by providing data-driven insights. These models use historical data to predict future call volumes, customer interactions, and agent performance. Understanding how these models work helps organizations prepare effectively for varying contact scenarios, ensuring they can adequately staff and manage workloads.
Various AI forecasting approaches include time series analysis, machine learning algorithms, and demand forecasting. Each method has distinct mechanics; some focus on identifying patterns in past behavior, while others use advanced analytics to consider numerous influencing factors. By utilizing these AI forecasting models, contact centers can minimize costs while maximizing customer satisfaction. Ultimately, adopting a systematic approach to AI forecasting empowers organizations to adapt to changing customer needs and improve overall service quality.
The Importance of AI Forecasting Models
AI forecasting models play a pivotal role in the realm of contact centers by offering enhanced predictive capabilities. These models analyze historical data to forecast future contact volumes, staffing requirements, and customer needs, enabling efficient resource allocation. The precision that AI provides significantly minimizes the chances of under or over-staffing, directly impacting operational costs and service delivery. As contact centers strive for greater efficiency and customer satisfaction, implementing these advanced forecasting techniques becomes essential for success.
Moreover, AI-driven forecasts contribute to improved decision-making processes, allowing managers to respond swiftly to changing dynamics. By accurately predicting call patterns and customer engagement, businesses can tailor their strategies to enhance service experiences. The integration of AI forecasting models not only boosts productivity but also fosters a proactive approach, ensuring that contact centers are prepared to meet customer demands effectively. Thus, recognizing the importance of such models is crucial for any contact center aiming to thrive in today's competitive environment.
- Role and benefits of forecasting models in optimizing contact center operations.
Optimizing contact center operations significantly benefits from AI forecasting models. These models analyze historical data and provide insights that enhance decision-making processes. By accurately predicting call volumes, customer inquiries, and service trends, contact centers can allocate resources more effectively, ensuring that staff levels match service demand.
Moreover, utilizing AI-generated forecasts helps improve customer satisfaction. By anticipating peak periods and common customer queries, teams can prepare more thoroughly, provide timely responses, and enhance overall service delivery. This proactive approach fosters a better customer experience and can lead to increased loyalty and retention.
In summary, the role of AI forecasting models in contact centers is pivotal. They not only streamline operations but also create a more responsive and customer-centric service environment. Integrating these models allows organizations to stay ahead of customer needs, ultimately supporting their growth and success.
- How AI-driven forecasts enhance accuracy and customer satisfaction.
AI-driven forecasts significantly enhance accuracy and customer satisfaction within contact centers. By harnessing the power of historical data and advanced algorithms, these models provide precise predictions regarding customer demands and service requirements. This increased accuracy helps minimize wait times and reduces the likelihood of overstaffing or understaffing. Consequently, contact centers can operate more efficiently while maintaining high-quality service levels.
Moreover, improved forecasting directly contributes to a better customer experience. When agents are better prepared for incoming inquiries, customers receive timely responses and tailored solutions. This leads to higher satisfaction rates and fosters customer loyalty. AI forecasts not only inform staffing decisions but also assist in creating more personalized experiences for clients. In summary, embracing AI forecasting models transforms operations and enhances overall customer engagement in contact centers.
Types of AI Forecasting Models Used in Contact Centers
AI forecasting models available for contact centers vary in their approach and utility, each designed to enhance operational efficiency and improve customer interactions. The most common types include time series analysis, which leverages historical data to identify trends and predict future contact volumes. Another model is machine learning, where algorithms learn from past data patterns to forecast demand more accurately. These models use statistical techniques and complex algorithms to refine their predictions continuously.
Additionally, regression analysis is widely used in forecasting, allowing contact centers to understand relationships between different variables, such as call volume and seasonality. By combining these AI forecasting models, contact centers can gain a deeper understanding of customer needs and behaviors. Ultimately, the effective implementation of these models leads to more informed decision-making, streamlined operations, and enhanced customer satisfaction.
- Overview of various AI models applicable to contact centers.
Artificial Intelligence is revolutionizing how contact centers manage forecasting through various advanced models. These AI forecasting models analyze historical data, patterns, and customer interactions to predict future demand accurately. By harnessing machine learning algorithms, contact centers can prepare for fluctuations in call volumes and tailor their resources accordingly. This predictive capability not only enhances operational efficiency but also significantly boosts customer satisfaction by ensuring prompt responses during peak times.
Several key types of AI models are particularly effective in this domain. Time Series Analysis utilizes past data to forecast future activity, while Regression Analysis identifies relationships between variables to predict specific outcomes. Neural Networks apply complex computations to enhance forecast accuracy, and Natural Language Processing allows deeper insights into customer sentiments. These models work together to create a comprehensive forecasting strategy, enabling contact centers to optimize their performance and improve overall service delivery.
- A brief explanation of how these models function and their purpose.
AI forecasting models play a critical role in contact centers by analyzing historical data to predict future trends. These models utilize algorithms and machine learning to identify patterns in customer interactions, call volumes, and service demands. By processing past data, they can help forecast fluctuations in workload or customer inquiries, allowing centers to allocate resources more effectively.
The primary purpose of these models is to enhance operational efficiency and improve customer satisfaction. Through accurate forecasts, contact centers can optimize staffing levels, ensuring the right number of agents are available during peak times. Additionally, predictive insights can inform training programs and resource allocation, ultimately leading to better service quality. As such, AI forecasting models serve not only to streamline operations but also to elevate the overall customer experience in contact centers.
Evaluate Performance on Customer Calls for Quality Assurance.
Evaluate Performance on Customer Calls for Quality Assurance.
Evaluate Performance on Customer Calls for Quality Assurance.
Step by Step: Implementing AI Forecasting Models
Implementing AI forecasting models involves a structured approach aimed at enhancing the efficiency of contact centers. Start by evaluating your existing data and processes. This initial step is crucial, as understanding your current situation helps identify gaps and opportunities for improvement. Next, select the right AI model tailored to your specific business needs. Consider factors like scalability, integration capabilities, and the nature of the data you deal with.
Once you have chosen an appropriate model, proceed with its implementation. This phase may involve training the model using historical data and integrating it with your existing systems. Continuous monitoring of the model's performance is essential to ensure it meets your expectations. By following these steps, you can systematically implement AI forecasting models, ultimately improving your contact center's operational efficiency and customer satisfaction.
Steps to Integrate AI Forecasting Models in Contact Centers
Integrating AI forecasting models into contact centers involves a series of strategic steps that can transform operations. Firstly, it’s essential to evaluate existing data and processes. Understanding the current capabilities helps identify gaps and opportunities for enhancement. This assessment ensures that the chosen AI forecasting model will align effectively with the specific needs of the contact center.
Next, selecting the right AI model is critical. Organizations should consider models that cater specifically to their forecasting requirements, assessing factors like data inputs, predictive accuracy, and scalability. Once a model is selected, implementation follows, requiring careful integration into existing systems. This phase also involves monitoring the model's performance and making necessary adjustments based on real-time data. By following these steps, contact centers can efficiently harness the power of AI forecasting models, leading to improved operational efficiency and enhanced customer satisfaction.
- Step 1: Evaluate existing contact center data and processes.
To effectively forecast demand and improve service levels in contact centers, it is crucial first to evaluate existing data and processes. This initial step allows organizations to identify gaps, redundancies, and opportunities that may affect performance. By thoroughly analyzing existing contact center data, decision-makers can gain insights into customer interactions, call volumes, and agent performance. Understanding these elements sets a solid groundwork for implementing AI forecasting models.
This evaluation process can be broken down into several key activities. First, assess the types and sources of data currently collected, such as call logs, customer feedback, and agent metrics. Next, analyze the efficiency of existing processes, such as call routing and handling times. Finally, consider how these elements align with overall business goals. Thorough evaluations will pave the way for selecting the most appropriate AI forecasting models that can enhance accuracy and optimize operations, ultimately leading to improved customer satisfaction.
- Step 2: Choose the right AI model that aligns with business needs.
Choosing the right AI model that aligns with business needs is essential for maximizing the benefits of AI forecasting models in contact centers. Begin by assessing your specific requirements, such as the volume of incoming interactions, the complexity of customer inquiries, and desired service levels. Each AI model offers distinct advantages, whether it's enhancing predictive accuracy or streamlining operational efficiency. Understanding these capabilities ensures you can select a model that suits your contact center's unique challenges.
Next, consider the data available within your organization. Some AI models thrive with structured data, while others can leverage unstructured data for insights. This differentiation can guide you in selecting the model that not only meets your present needs but also adapts to future growth. By keeping business objectives and available resources in mind, you can make an informed choice that enhances performance, ultimately driving higher customer satisfaction and operational excellence in your contact center.
- Step 3: Implement the AI model and monitor its performance.
Once the appropriate AI forecasting model has been selected, the next step is implementation and performance monitoring. Begin by integrating the AI system with existing contact center operations, ensuring seamless data flow from customer interactions. This step involves configuring the AI system to analyze incoming data and produce forecasts tailored to your center's specific needs. Training staff on how to utilize these insights effectively will enhance overall productivity and accuracy.
To maintain the model's effectiveness, continuous performance monitoring is essential. Set key performance indicators (KPIs) to evaluate the AI forecasting model's accuracy and reliability. Regularly analyze the forecast results, feedback from agents, and overall customer satisfaction. Adjustments may be necessary based on the data collected to refine the model further. Adopting a proactive approach in monitoring and tweaking the AI forecasting models ensures that your contact center remains agile and capable of meeting ever-evolving customer demands.
Tools for AI Forecasting Models in Contact Centers
Creating effective AI forecasting models requires specialized tools designed for the unique challenges of contact centers. The selection of tools significantly impacts an organization's ability to analyze data and predict customer interactions. With the increasing amount of customer signals generated, relying on traditional methods is no longer sufficient.
Several prominent tools assist in generating AI forecasting models tailored for contact centers. Insight7 stands out as a premier option, enabling comprehensive data analysis and forecasting. Another tool, IBM Watson Analytics, harnesses AI to enhance predictive analytics capabilities, improving decision-making processes. Additionally, Google Cloud AI offers robust functionalities that facilitate more accurate forecasts. Salesforce Einstein provides AI-driven insights into customer interactions, while Amazon Forecast delivers highly customizable forecasting solutions powered by machine learning. By strategically implementing these tools, contact centers can enhance their forecasting accuracy and overall operational efficiency.
- Insight7: Top choice for comprehensive data analysis and forecasting.
In the quest for effective data analysis and forecasting, AI-powered tools like Insight7 stand out as preferred options for contact centers. These platforms efficiently synthesize large volumes of data, providing actionable insights that help businesses understand customer behavior and optimize their strategies. By employing advanced AI forecasting models, contact centers can anticipate demand and enhance service delivery, ensuring they remain competitive in a rapidly evolving landscape.
The versatility of these AI forecasting models is evident in their ability to analyze historical data, consumer signals, and trends. This multifaceted approach enables contact centers to make informed decisions that drive operational efficiency. Not only do these models predict future call volumes, but they also support resource allocation and training needs. By streamlining data analysis, organizations maximize customer satisfaction while minimizing response times, paving the way for success in customer engagement.
- IBM Watson Analytics: Utilizing AI for predictive analytics in contact centers.
Utilizing AI for predictive analytics in contact centers transforms how organizations gather insights from customer interactions. When implementing AI forecasting models, the goal is to enhance operational efficiency, boost customer satisfaction, and ultimately drive better business outcomes. Predictive analytics harnesses the capabilities of AI to analyze past and current data, enabling contact centers to anticipate trends and customer needs accurately.
AI forecasting models, such as time-series analysis and machine learning algorithms, allow for better resource management and workforce scheduling. By extracting valuable insights from vast amounts of interaction data, these models identify trends that traditional methods often overlook. Organizations can proactively address customer inquiries and optimize staffing levels, leading to improved service levels while minimizing operational costs. Embracing these AI-driven methodologies not only fosters a more responsive environment but also equips contact centers to thrive in today's competitive landscape.
- Google Cloud AI: Offering advanced AI functionalities for improved forecasts.
Advanced artificial intelligence functionalities can significantly enhance forecasting accuracy in contact centers. By employing these AI forecasting models, organizations can predict call volumes more precisely. Accurate predictions help in staffing decisions, ensuring that the right number of agents are available to handle customer inquiries efficiently.
A critical aspect of these models is their ability to analyze historical data and real-time interactions. They identify trends and patterns, which inform future staffing and operational strategies. By integrating these insights, businesses can transition from reactive to proactive management, ultimately driving better customer satisfaction and engagement. Furthermore, AI forecasting models empower contact centers to adapt to changing market conditions, enhancing overall performance. The utilization of such advanced technology positions organizations to thrive in an increasingly competitive landscape.
- Salesforce Einstein: AI-powered insights for customer interaction forecasts.
AI-powered insights transform how contact centers manage customer interactions. By utilizing advanced algorithms and data analysis techniques, these insights enable more accurate predictions of customer behavior and needs. As a result, organizations can optimize their operations and improve overall service quality. Understanding these forecasting models allows businesses to anticipate demand fluctuations and manage resources accordingly.
Several key AI forecasting models are commonly employed in contact centers. 1. Predictive Analytics utilizes historical data to forecast future trends. 2. Time Series Analysis examines past interaction data to identify patterns, providing insights into peak periods. 3. Machine Learning Models continually adapt and refine predictions based on new data, ensuring accuracy over time. Embracing these AI forecasting models empowers contact centers to enhance customer satisfaction and operational efficiency, ultimately leading to a competitive advantage in the market.
- Amazon Forecast: Customizable forecasting with machine learning technology.
In the realm of AI forecasting models, customizable forecasting with machine learning offers unique advantages for contact centers. These models utilize historical data and advanced algorithms to accurately predict future trends and customer needs. By tailoring the process to a contact center’s specific environment, organizations can achieve high levels of precision in forecasting call volumes, staffing requirements, and customer sentiment.
These sophisticated models enable contact centers to stay adaptable in an ever-changing environment. For instance, they can dynamically adjust forecasts based on seasonal trends or unexpected market shifts. With the ability to analyze large datasets in real time, decision-makers are equipped with actionable insights, facilitating timely interventions. Ultimately, customizable forecasting not only streamlines operations but also enhances customer satisfaction by ensuring that service levels meet demand accurately.
Conclusion: The Future of AI Forecasting Models in Contact Centers
AI Forecasting Models are poised to revolutionize contact centers in the coming years. As technology matures, these models will become increasingly sophisticated, allowing for more accurate predictions of customer behavior and call volumes. Not only will this enhance operational efficiency, but it will also lead to improved customer experiences, ultimately driving higher satisfaction rates.
Looking ahead, the integration of AI Forecasting Models will empower contact centers to make data-driven decisions swiftly. Enhanced analytical tools will facilitate real-time adjustments, ensuring that resources meet demand effectively. As organizations embrace these advancements, the focus will shift towards leveraging insights for strategic growth in an unpredictable market landscape.