“What are common mistakes when analyzing call center data?”
-
Bella Williams
- 10 min read
Call center analysis can be a powerful tool for enhancing customer service, but it comes with several pitfalls that can undermine its effectiveness. One major issue is the misinterpretation of metrics, where key performance indicators such as Average Handling Time (AHT) may be misunderstood. Without context, these numbers can lead organizations to inaccurate conclusions about their operations and staff performance.
Additionally, neglecting the importance of data cleansing can cause significant issues. Inaccurate or unclean data will compromise the integrity of the analysis. Common problems, such as duplicates and incomplete entries, can skew results and affect decision-making. Awareness and diligence in addressing these call center analysis pitfalls are essential to obtain valuable insights and improve service quality.
Generate visualizations from your qualitative data. At Scale.
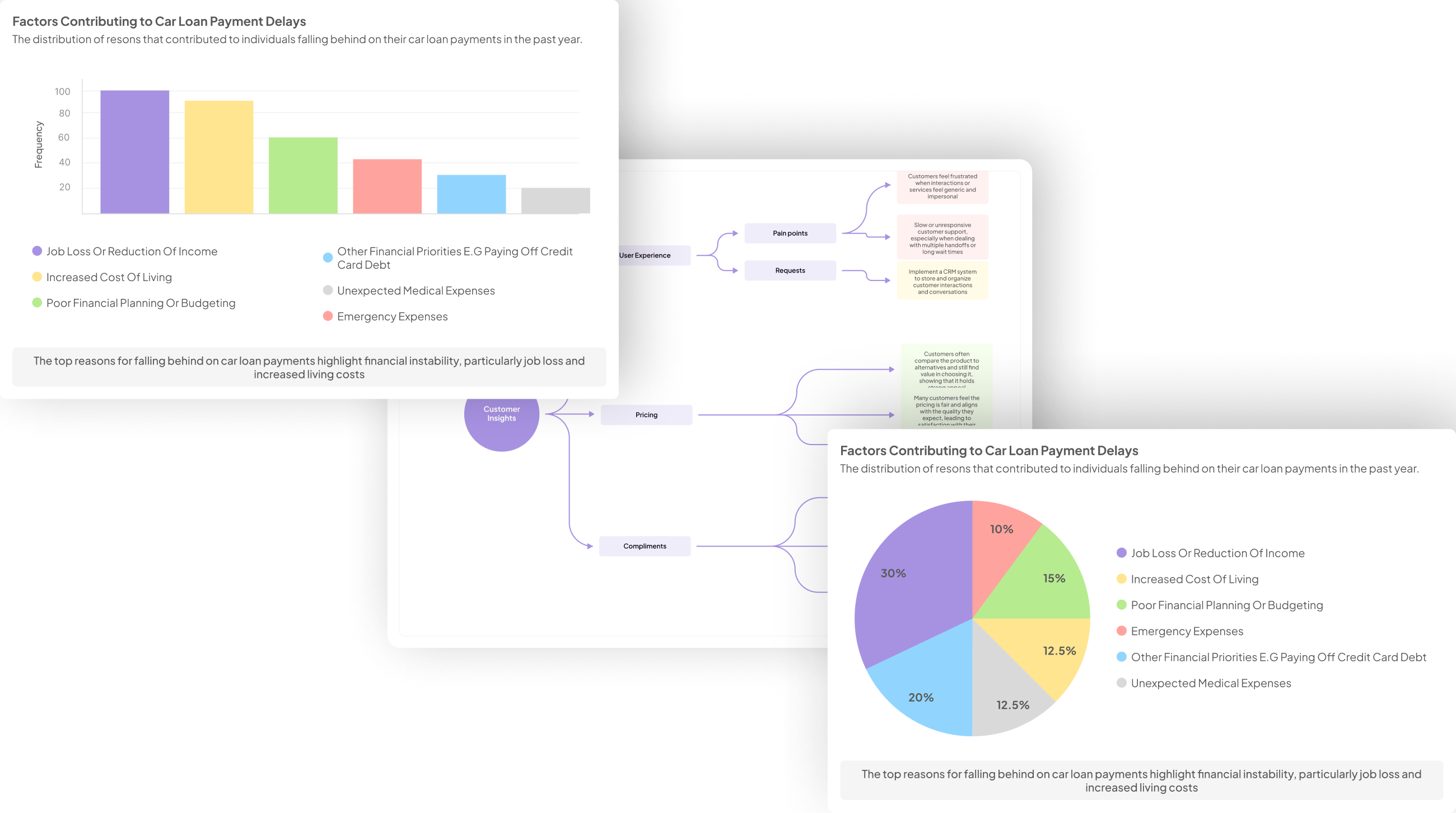
Common Mistakes in Call Center Analysis Pitfalls
In navigating common mistakes in call center analysis pitfalls, it is crucial to recognize the misinterpretation of metrics. Many analysts mistakenly view Average Handling Time (AHT) as the sole indicator of efficiency. However, without considering the context of each interaction, this can lead to flawed conclusions. For example, a longer call time could indicate a complex issue being resolved rather than inefficiency.
Another significant pitfall is inadequate data cleansing. Utilizing unclean data can distort analysis results and ultimately lead decision-makers astray. Common data quality issues include duplications, irrelevant entries, and inconsistent formatting. These flaws not only obscure legitimate insights but also misguide training and operational strategies. Ensuring high data accuracy is essential for reliable analysis and actionable insights. Recognizing these pitfalls is the first step toward improving overall call center performance.
Misinterpretation of Metrics
Misinterpreting key metrics in call center analysis can significantly skew results, leading to misleading conclusions. For instance, Average Handling Time (AHT) might be misread as a sole indicator of efficiency, ignoring nuances like agent training needs or customer complexity. Similarly, Customer Satisfaction (CSAT) scores can be misleading if not contextualized. A high CSAT might seem positive, but if it’s paired with low retention rates, there's a problem that needs addressing.
Moreover, overlooking the context surrounding these metrics can distort their real significance. Without understanding factors like call volume trends or customer demographics, analysts may miss critical insights. Metrics should always tell a story; if you focus solely on numbers without considering the bigger picture, you'll fall into common call center analysis pitfalls. Analyzing metrics in context allows for more informed decisions and improvements in service delivery, ultimately enhancing the overall customer experience.
- Discuss how misinterpreting key metrics like Average Handling Time (AHT) and Customer Satisfaction (CSAT) can lead to incorrect conclusions.
Misinterpreting key metrics like Average Handling Time (AHT) and Customer Satisfaction (CSAT) can significantly mislead call center operations. For example, focusing solely on AHT may prompt management to rush calls, inadvertently compromising the quality of service. Consequently, customer satisfaction may decline, leading to a paradox where efficiency metrics suggest improvement while actual customer experiences worsen.
Similarly, high CSAT scores may falsely imply effective service when they might merely reflect unsustainable practices. Inaccurate interpretations of these metrics can distort the understanding of performance dynamics, resulting in misguided strategies. A comprehensive approach, considering both AHT and CSAT in context, is essential to avoid common call center analysis pitfalls and to drive meaningful improvements.
- Explain how neglecting the context can distort data interpretation.
Neglecting context when analyzing call center data can significantly distort data interpretation. For example, if a spike in call volume occurs during a holiday season, interpreting it as a decline in service quality might lead to misguided strategies. Similarly, if customer satisfaction ratings drop after a service change, it’s crucial to consider the specific circumstances contributing to this feedback. Without contextual understanding, analysts may jump to conclusions that veer their focus away from the real issues.
In call center analysis pitfalls, it’s essential to ask the right questions. Analysts should examine not just the quantitative metrics but also the qualitative factors influencing those metrics. Are there external events affecting customer behavior? Are there unique aspects of particular datasets, such as geographical differences? By incorporating these contextual factors, organizations can derive more accurate insights and craft effective strategies that address actual service deficiencies. Hence, recognizing the broader context ensures that data interpretation aligns effectively with reality.
Inadequate Data Cleansing
Inadequate data cleansing poses significant challenges during call center data analysis, often leading to misleading insights. The importance of having accurate and reliable data cannot be overstated. When organizations use unclean data, the results may reflect errors rather than the true performance of the call center. Common issues include duplicate entries, missing values, and inconsistent formats, all of which cloud analysis efforts.
These data quality issues distort metrics and can lead to misguided decisions. For instance, if duplicate entries inflate call volume metrics, decision-makers might misinterpret the center's efficiency. Additionally, not addressing inconsistencies can skew assessments of customer satisfaction. Prioritizing data cleansing before analysis not only enhances accuracy but also builds trust in the insights derived from the data. Ensuring data integrity is critical in avoiding the call center analysis pitfalls that arise from inadequate data cleansing.
- Highlight the importance of data accuracy and the impact of using unclean data.
Data accuracy is paramount when conducting call center analysis. Utilizing unclean data can lead to misguided conclusions and ineffective strategies. Common issues with data accuracy include duplicate entries, incomplete records, and outdated information. These issues may distort key metrics, such as Average Handling Time (AHT) and Customer Satisfaction (CSAT), leading analysts to draw the wrong insights.
When unclean data skews results, the entire analysis can be compromised. For instance, a high AHT caused by erroneous records could be misperceived as inefficiency, prompting unnecessary changes in staffing or protocols. Therefore, it is vital to ensure data is thoroughly cleansed and validated before analysis to avoid these pitfalls. Accurate data not only reflects the real performance of the call center but also empowers teams to make data-driven decisions that enhance the customer experience and drive business growth.
- Share common data quality issues and how they affect analysis results.
Data quality issues can severely impact the outcomes of call center analysis, leading to misguided strategies and decisions. Common pitfalls include inaccurate data entry, which results in misleading insights. Errors in recording customer interactions, along with missing or incomplete data fields, can skew analysis results, often masking underlying trends and patterns.
Another significant issue is outdated data, which can create a false sense of operational efficiency. When analysts rely on stale data, they may perceive a decline in performance when the reality is merely due to irrelevant metrics. Additionally, inconsistency in data formats can lead to confusion and difficulty in aggregating insights. To avoid these pitfalls, it is crucial to implement thorough data cleansing processes. Regular audits and standardization efforts can help maintain data integrity, ensuring that analysis reflects actual customer experiences and interactions. By addressing these data quality issues, call center analysis can yield more accurate and actionable insights, supporting better decision-making.
💬 Questions about “What are common mistakes when analyzing call center data?”?
Our team typically responds within minutes
Generate Journey maps, Mind maps, Bar charts and more from your data in Minutes
Advanced Call Center Analysis Pitfalls: Avoiding Overcomplication
Overcomplication in call center analysis often leads to confusion and misdirection, making it one of the significant pitfalls in the process. Analysts can become overly focused on intricate models and algorithms, losing sight of the fundamental goals of their analysis. This can result in convoluted reporting, where insights are buried under layers of unnecessary details, making it challenging for stakeholders to make informed decisions. Keeping analysis straightforward allows for clearer insights that drive action.
Another common mistake is neglecting root cause analysis. When only surface-level issues are addressed, the underlying problems persist and can lead to recurring customer complaints. Effective root cause analysis involves asking the “why” behind trends and issues, thereby revealing critical insights. Additionally, ignoring customer feedback can stunt the potential for improvement. Integrating qualitative feedback enhances quantitative data analysis, painting a fuller picture of customer experiences. Emphasizing simplicity in analysis fosters better understanding and actionable outcomes, thereby avoiding the pitfalls of overcomplication.
Overlooking Root Cause Analysis
Failing to perform a thorough root cause analysis is a common oversight in call center analysis pitfalls. Relying on surface-level data can lead to temporary fixes instead of addressing the underlying issues. For instance, if call abandonment rates rise, merely increasing staff or altering scripts may not resolve deeper systemic problems, such as inefficient processes or inadequate training. Overlooking the root causes can result in recurring challenges, frustrating both agents and customers.
To conduct an effective root cause analysis, consider a structured approach. Begin by collecting relevant data from various sources, including call recordings and agent feedback. Next, engage teams in brainstorming sessions to identify potential reasons for customer dissatisfaction or operational shortcomings. Finally, prioritize these causes based on their impact and feasibility for resolution. By doing so, organizations can not only enhance operational efficiency but also improve customer satisfaction, leading to better overall performance in the call center.
- Explain why failing to perform a detailed root cause analysis can lead to surface-level solutions.
When analyzing call center data, a critical mistake is bypassing a thorough root cause analysis. Failing to identify the underlying issues often results in implementing surface-level solutions, which may only provide temporary relief. For instance, if call volume spikes due to customer dissatisfaction, addressing only staffing levels may neglect the core issue driving complaints. This approach can lead to recurring problems, as superficial solutions do not foster long-term improvements.
Additionally, surface-level solutions can mask the true nature of systemic issues within the call center. Without delving into root causes, teams may remain unaware of deeper operational flaws, resulting in wasted resources and diminished customer trust. To avoid these pitfalls, organizations should adopt a structured method for root cause analysis, integrating data insights with qualitative feedback to pinpoint problems accurately. This comprehensive approach ensures that solutions are effective and sustainable, enhancing overall customer satisfaction and operational efficiency.
- Describe steps for effective root cause analysis.
To engage in effective root cause analysis for common call center data pitfalls, one should start by gathering relevant data meticulously. Ensuring clean, accurate data lays the foundation for reliable insights. Next, it’s essential to involve cross-functional teams to gain diverse perspectives; this collaboration can uncover factors that may go unnoticed during individual analysis.
Once you've gathered the necessary information, identify patterns and potential issues. Delve deep into the causes behind the metrics rather than stopping at surface-level symptoms. Asking “why” multiple times can often reveal the true root causes of the problems faced. Lastly, document your findings clearly to facilitate better decision-making and future improvement initiatives. Skipping these steps can lead to superficial solutions, significantly hindering the call center's overall performance and effectiveness. By following these structured steps, organizations can mitigate the call center analysis pitfalls and drive impactful changes.
Ignoring Customer Feedback
In the realm of call center analysis pitfalls, one critical mistake is ignoring customer feedback. Customer feedback serves as a valuable source of qualitative data. Failing to consider this feedback can result in a skewed understanding of customer experiences and needs. When analysts solely rely on quantitative metrics, they overlook the nuanced insights that qualitative data provides.
Collecting customer feedback can take many forms, including surveys, call transcripts, and social media interactions. An effective way to analyze this feedback is to categorize comments into themes, such as service quality or product issues. Additionally, employing sentiment analysis can help quantify the emotional tone of customer interactions. Overall, integrating customer feedback into your analysis process enables a more holistic view of call center performance. By doing so, you can identify areas for improvement, ensuring that solutions are truly aligned with the customers' preferences and expectations.
- Emphasize the importance of integrating qualitative data from customer feedback into the analysis.
Ignoring customer feedback can significantly undermine the effectiveness of call center analysis. Integrating qualitative data provides critical context that numbers alone cannot convey. When analyzing data trends, it’s vital to delve into what customers are saying, as it offers insights into their emotions, thoughts, and behaviors. Data metrics like call durations and resolution rates do not tell the complete story without understanding customer sentiments expressed during calls.
Additionally, customer feedback can highlight underlying issues that quantitative data may overlook. By approaching call center analysis with a balanced perspective that includes qualitative insights, teams can foster a deeper understanding of customer needs. Methods such as categorizing feedback into themes or analyzing verbatim comments can help distill essential insights. This holistic approach can enable call center operations to implement more effective strategies, reducing the common mistakes in call center analysis pitfalls. Overall, integrating qualitative data becomes not just beneficial, but essential for achieving meaningful results in customer care analysis.
- Discuss methods for collecting and analyzing feedback effectively.
Collecting and analyzing feedback effectively is crucial to avoiding common call center analysis pitfalls. First, it's important to establish clear channels for gathering input from customers. Surveys, follow-up calls, and social media feedback are excellent methods to ensure diverse customer voices are heard. Make sure these channels are easy for customers to access, as this enhances participation rates.
Once feedback is collected, analyzing it should involve both quantitative and qualitative approaches. Quantitative analysis can include examining numerical ratings, while qualitative analysis encompasses sentiment analysis or thematic coding of comments. This dual-method approach enables a well-rounded understanding of customer experiences, allowing for actionable insights. Regularly revisiting and refining these processes can significantly improve the reliability of the analysis and aid in crafting effective responses to customer needs. By taking these steps, organizations can sidestep the pitfalls often seen in call center data analysis.
Conclusion: Navigating Call Center Analysis Pitfalls
In conclusion, navigating call center analysis pitfalls requires diligence and careful consideration. It's crucial to recognize that misinterpretations of key metrics can lead decision-makers astray, often resulting in misguided actions. Instead, a comprehensive understanding of metrics, like Average Handling Time and Customer Satisfaction, is vital to formulating effective strategies.
Additionally, ensuring data accuracy is paramount. Inadequate data cleansing can obscure valuable insights and skew results, leading to overall inefficiency. By integrating qualitative feedback from customers, analysts can obtain a more holistic view of performance. Ultimately, avoiding these pitfalls allows teams to develop a more robust and actionable analysis framework.
Generate visualizations from your qualitative data. At Scale.
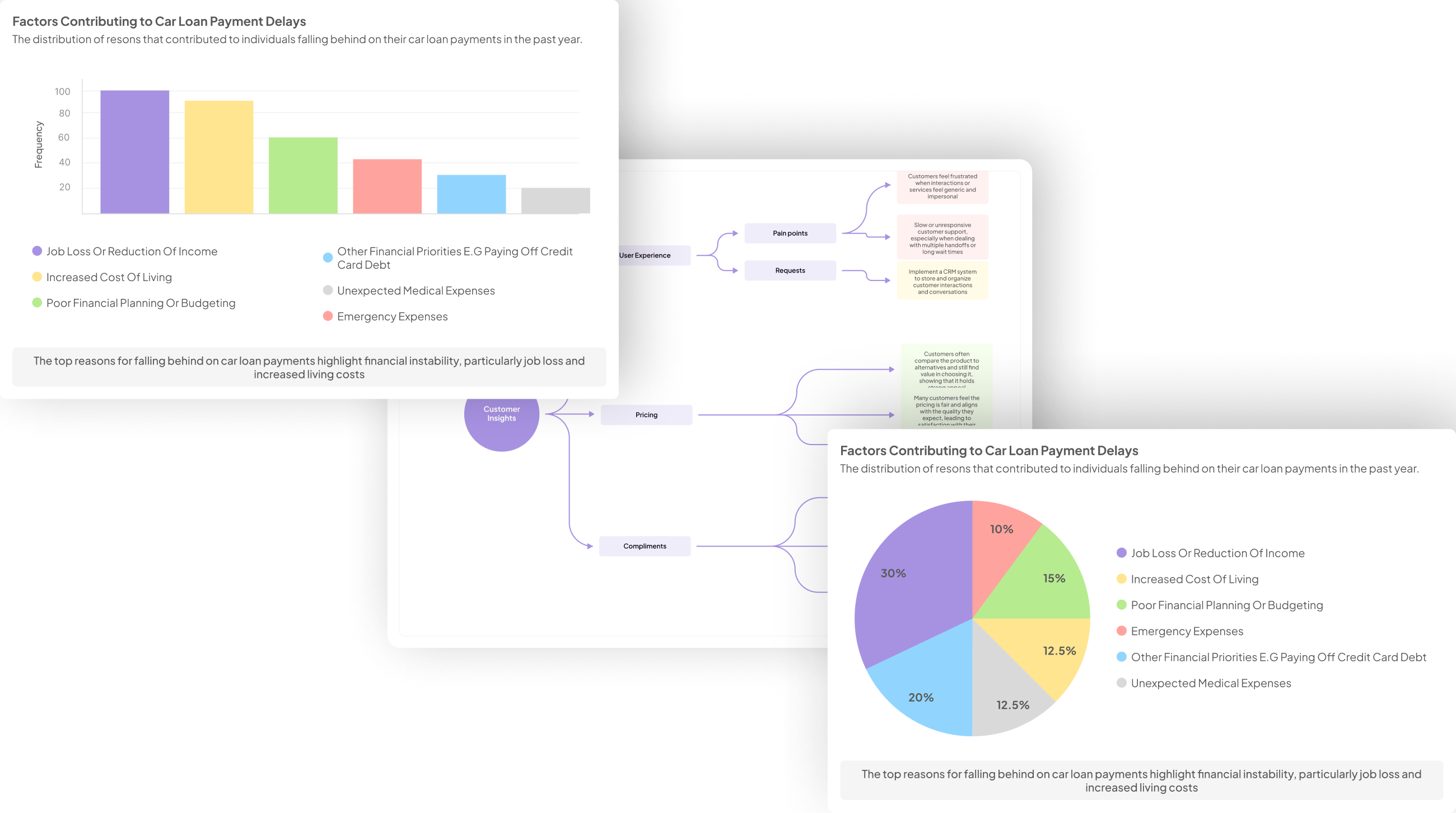
💬 Questions about “What are common mistakes when analyzing call center data?”?
Our team typically responds within minutes