Voice Analytics vs Speech Analytics: Core Differences
-
Bella Williams
- 10 min read
In today's data-driven world, understanding customer interactions is crucial for business success. Audio insights comparison plays a vital role in deciphering the intricacies of human communication, enabling companies to make informed decisions. As organizations increasingly turn to innovative technologies, distinguishing between voice analytics and speech analytics becomes essential for effective strategy development.
Both fields harness the power of audio data but serve distinct purposes. Voice analytics focuses on extracting sentiment and emotions from spoken interactions, while speech analytics emphasizes deciphering the content of conversations. Understanding these core differences not only helps businesses optimize customer experiences but also enhances overall communication strategies in an ever-evolving market.
Generate visualizations from your qualitative data. At Scale.
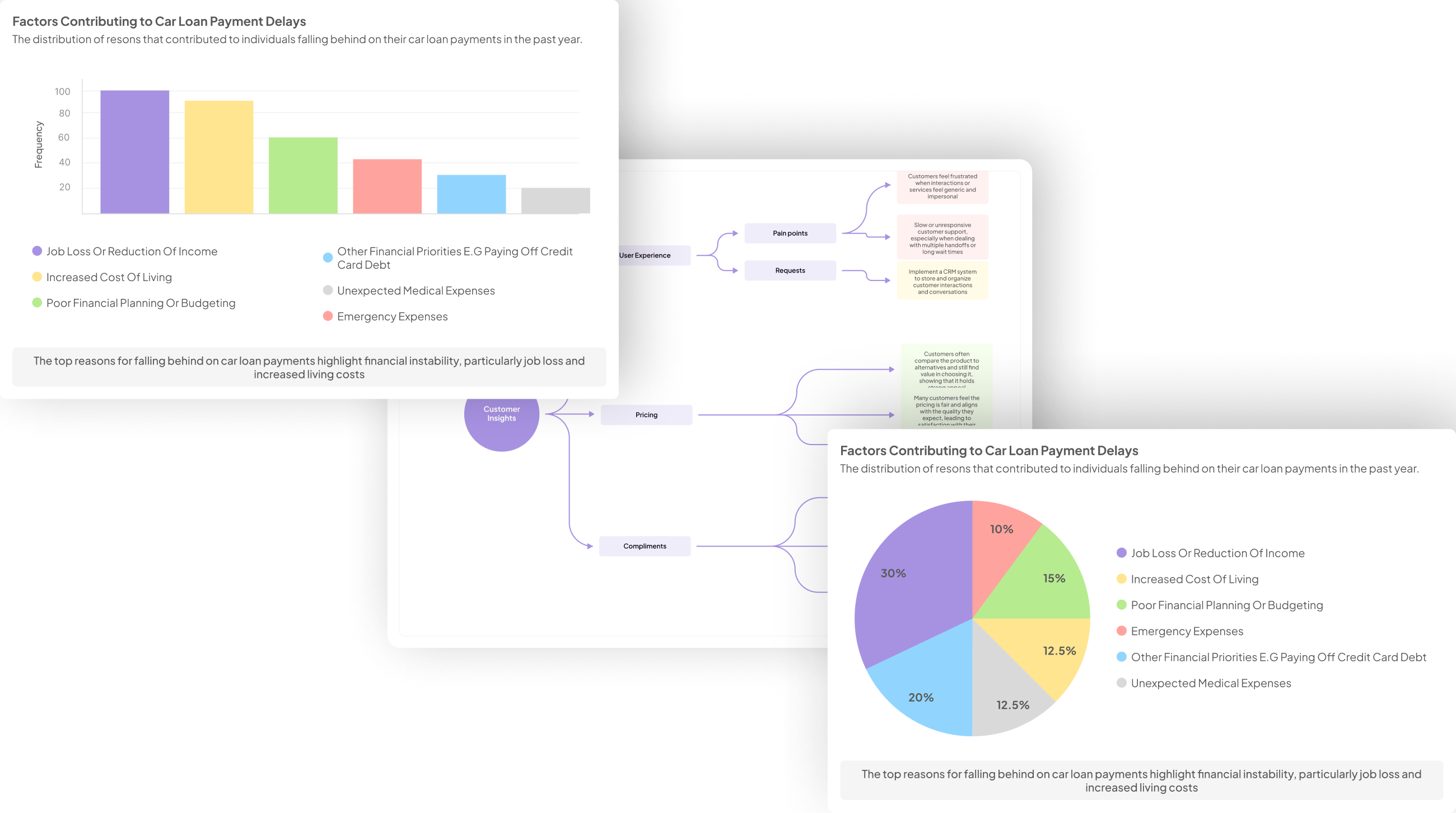
Exploring the Fundamentals of Voice and Speech Analytics
Voice and speech analytics are two essential tools for understanding audio data, yet they serve different functions. At the core, voice analytics focuses on the acoustic features of speech, such as tone, pitch, and emotion. This method allows organizations to gauge customer sentiment effectively and extract deeper insights from conversations. For instance, by analyzing voice tone patterns, businesses can identify customer satisfaction levels and adapt their strategies accordingly.
Conversely, speech analytics prioritizes the semantic content of conversations, enabling organizations to analyze the words and phrases used by customers. This form of analysis highlights specific topics, trends, and insights that stem from actual dialogue. By transcribing and analyzing calls or meetings, organizations can harness information to make informed decisions. Understanding the fundamental principles of these technologies facilitates a comprehensive grasp of their potential applications and enhances the overall audio insights comparison within any analytical framework.
Understanding Voice Analytics: An Overview of Audio Insights Comparison
Voice analytics focuses on extracting useful insights from spoken interactions, primarily to enhance customer experience. By analyzing voice data, businesses can identify pain points, desires, and behavioral patterns, all of which are critical for understanding customer feedback. This analysis allows organizations to address customer needs effectively, transforming raw audio into actionable insights.
In contrast, speech analytics involves a broader approach, utilizing not just audio but also related textual data. This enables a more comprehensive understanding of conversations, allowing organizations to compare performance metrics and trends over time. Audio insights comparison plays a vital role in distinguishing the two fields, showcasing how each contributes differently to customer engagement and response strategies. Although both methods aim to improve user experience, their techniques and applications differ significantly, offering unique perspectives based on specific business needs.
Delving into Speech Analytics: How It Differs and Compares
Speech analytics focuses on extracting meaningful insights from spoken language, often to enhance customer interactions. By analyzing customer conversations, organizations can identify trends, sentiments, and areas for improvement in service. Unlike general voice analytics, which encompasses all audio data, speech analytics zooms in on the words spoken and the context behind those words.
Understanding the distinctions in audio insights comparison between these two analytics types is vital. For example, speech analytics uses advanced techniques like natural language processing to interpret emotions in conversations. Additionally, it categorizes feedback and tracks compliance with service standards, which can be highly beneficial for training staff. Conversely, voice analytics may deal with larger sets of data that include music, background noise, or other audio elements, making it less tailored for specific conversations. Thus, organizations can better align their strategies by recognizing how each analytics method serves their goals.
💬 Questions about Voice Analytics vs Speech Analytics: Core Differences?
Our team typically responds within minutes
Evaluate Performance on Customer Calls for Quality Assurance.
Key Differences in Audio Insights Comparison Between Voice and Speech Analytics
In the realm of audio insights comparison, understanding the distinctions between voice analytics and speech analytics is crucial. Voice analytics primarily focuses on analyzing the tonal elements and emotions conveyed through speech. It delves into the speaker's delivery, pitch, and stress levels, which can reveal hidden sentiments or emotional states during a conversation. On the other hand, speech analytics is more about transcribing and deciphering the actual content of the conversation. It emphasizes word choice, clarity, and the utilization of language to extract meaningful information from the dialogue.
These differences highlight varying objectives within audio insights comparison. While voice analytics seeks to understand the "how" behind the speech, speech analytics aims to clarify the "what." Both approaches provide valuable insights, but they cater to distinct analytical needs. Knowing when to apply each method can lead to more effective outcomes in understanding customer interactions and enhancing overall communication strategies.
The Role of Context in Audio Insights Comparison
In any analysis comparing audio insights, the context plays a critical role in determining the applicability and relevance of the data. Understanding the background of conversations—such as the speaker's intent, emotional tone, and situational specifics—significantly enhances the accuracy of insights derived from both voice and speech analytics. In this framework, context not only shapes the data being analyzed but also informs decisions on how to respond to customer needs effectively.
Moreover, incorporating contextual information allows businesses to identify patterns and trends that may otherwise go unnoticed. For example, recognizing the environment in which customer interactions occur can lead to more tailored service responses. Thus, the role of context enhances the quality of audio insights comparison by bridging the gap between raw data and actionable intelligence, ultimately guiding businesses in crafting effective strategies.
Technical Distinctions: Algorithms and Outputs
The algorithms behind voice and speech analytics play a crucial role in how data is processed and utilized. Voice analytics primarily relies on machine learning models that focus on interpreting emotional tones and nuances in human speech. This helps in analyzing customer sentiment and engagement during interactions. In contrast, speech analytics emphasizes the structure of spoken language, such as keyword recognition and conversation flow. This approach allows for a deeper understanding of dialogue patterns, thereby improving communication strategies.
Outputs from these analyses differ significantly based on the algorithmic approach. Voice analytics often produces sentiment scores and engagement metrics, which guide responses in customer service scenarios. On the other hand, speech analytics yields transcriptions and topic extraction, aiding in understanding recurring themes and customer inquiries. By comparing these audio insights, businesses can tailor their strategies in ways that are both effective and responsive to customer needs.
Conclusion: Synthesizing Audio Insights Comparison
In synthesizing the audio insights comparison, it's essential to recognize the unique roles that voice and speech analytics play in extracting valuable information from spoken language. While both methodologies aim to analyze audio data, they target distinct elements of the conversation, thereby providing diverse qualitative insights. Voice analytics often focuses on emotional tone and speaker behavior, while speech analytics emphasizes the content and intent behind the dialogue.
Both approaches are crucial for understanding customer experiences and improving service delivery. By comparing these audio insights, organizations can choose the appropriate analytics that best fits their objectives. Ultimately, a nuanced understanding of these techniques enhances decision-making, leading to better strategies that meet customer needs effectively.
💬 Questions about Voice Analytics vs Speech Analytics: Core Differences?
Our team typically responds within minutes