Using AI to Analyze User Onboarding Conversations for Drop-Off Signals
-
Bella Williams
- 10 min read
Every onboarding journey is unique, yet many users share similar roadblocks that lead them to drop off early. Effective onboarding drop-off analysis can unveil these critical pain points, providing insights into user experience and satisfaction. Utilizing AI in this process enables companies to analyze user conversations, identifying trends and signals that highlight where users struggle during onboarding.
This introduction sets the stage for a deeper exploration of onboarding drop-off analysis. By examining user interactions and engagement metrics, companies can tailor their onboarding strategies, ultimately reducing drop-off rates. With AI, organizations can not only faster identify problem areas but also implement solutions that facilitate a smoother onboarding experience.
Analyze & Evaluate Calls. At Scale.
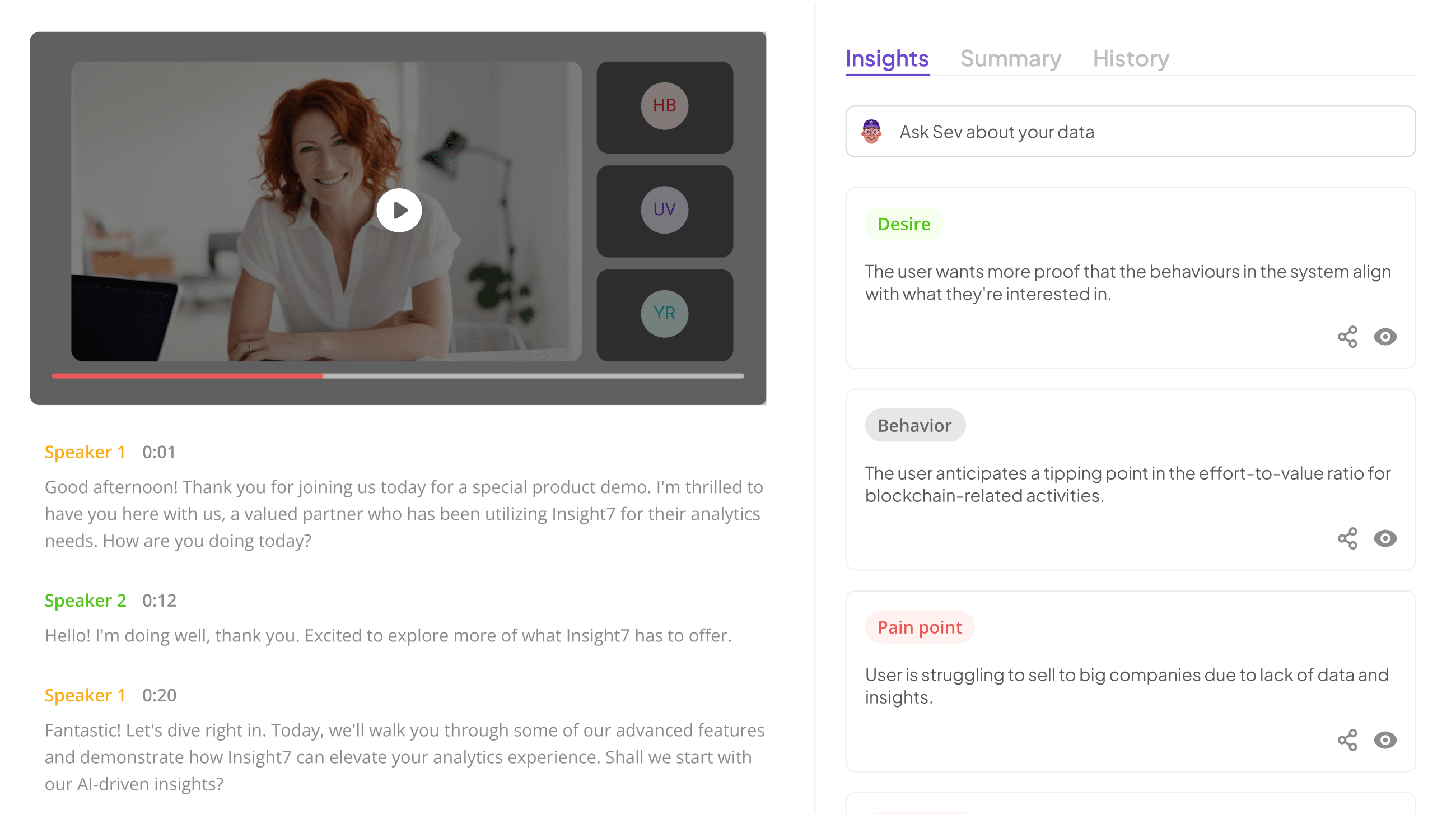
Identifying Key Patterns in Onboarding Drop-off Analysis
To effectively analyze Onboarding Drop-off Analysis, it is essential to identify key patterns in user interactions. By closely examining user conversations, organizations can uncover specific moments that contribute to onboarding failures. This analysis requires understanding how users express their difficulties or confusion during conversations, allowing for targeted improvements in the onboarding process.
Several significant patterns may emerge during onboarding interactions. Firstly, frequent mentions of specific features or tasks can signal potential bottlenecks. Secondly, expressions of frustration or hesitation before completing critical steps can indicate points of confusion. Finally, the timing of these drop-off signals is crucial; recognizing when users are most likely to disengage offers valuable insights into refining onboarding strategies. By capturing and analyzing these patterns, teams can develop more effective onboarding experiences tailored to user needs, ultimately reducing drop-off rates and enhancing overall user satisfaction.
Understanding User Conversations for Onboarding Drop-off Analysis
Analyzing user conversations during the onboarding process can reveal critical insights into drop-off patterns. Understanding User Conversations for Onboarding Drop-off Analysis involves closely examining interactions to identify where users feel confused or disengaged. These conversations often highlight the factors that contribute to users abandoning the onboarding experience. By pinpointing these moments, teams can implement targeted strategies to improve retention and enhance user satisfaction.
To effectively understand user conversations, consider these key aspects: first, identify common queries and pain points raised by users. Next, examine the tone and sentiment behind their messages to gauge frustration levels. Lastly, consider the context in which users engage with the onboarding content. This multi-faceted approach enables organizations to uncover meaningful insights that inform improvements in the onboarding process, ultimately driving better user engagement and reducing drop-off rates.
Leveraging AI to Detect Drop-Off Trends and Signals
Detecting drop-off trends and signals is crucial for enhancing onboarding processes. By analyzing user interactions during onboarding, you can identify specific moments where users disengage. AI plays a transformative role in this analysis, offering tools that can sift through large volumes of conversation data efficiently.
To uncover patterns, AI algorithms can identify recurring themes or pitfalls that lead to user drop-offs. For example, monitoring questions that frequently arise can illustrate where users feel confused or unsupported. Additionally, sentiment analysis can gauge user frustration or satisfaction during the onboarding experience. Understanding these signals allows organizations to refine their onboarding strategies, implementing best practices that retain user interest. Proactive measures, such as tailored content and timely check-ins, become achievable with insights generated from data analysis, enhancing user experiences and driving greater engagement throughout the onboarding journey.
Extract insights from interviews, calls, surveys and reviews for insights in minutes
Tools and Techniques for Effective Onboarding Drop-off Analysis
Effective Onboarding Drop-off Analysis is crucial for understanding user behavior during the onboarding process. It encompasses various tools and techniques that enable teams to identify patterns in user conversations. By analyzing these conversations, businesses can discern common points where users experience challenges or disengagement. The goal is to drive user retention by addressing these drop-off signals proactively.
One can employ AI-driven tools like Insight7, which offers robust quantitative analysis and transcription capabilities. Additionally, exploring platforms such as IBM Watson, Google Cloud Natural Language, and Microsoft Azure Text Analytics can significantly enhance the analysis process. These tools help in extracting meaningful insights from user interactions, thus facilitating refined strategies to improve the onboarding experience. When integrated properly, these techniques not only identify drop-off signals but also facilitate a user-centric onboarding journey, leading to higher satisfaction and retention rates.
Utilizing insight7 for Enhanced Onboarding Drop-off Analysis
Understanding user behavior during the onboarding process is essential for reducing drop-off rates. Utilizing insight7 enhances onboarding drop-off analysis by enabling the examination of user conversations at scale. This tool analyzes patterns in communication that may indicate where users struggle or disengage. Such insights can help identify common obstacles within the onboarding journey and offer strategies to address them effectively.
Additionally, employing insight7 allows teams to highlight critical touchpoints in the onboarding process. By examining interactions, businesses can determine the effectiveness of preset defaults and best practices established prior to onboarding. This proactive approach, complemented by scheduled check-ins and instructional videos, provides users with the support they need to fully engage. Identifying and addressing these drop-off signals ensures that users derive value quickly, fostering long-term retention and satisfaction.
💬 Questions about Using AI to Analyze User Onboarding Conversations for Drop-Off Signals?
Our team typically responds within minutes
Exploring Additional AI Tools for Onboarding Conversations
When exploring additional AI tools for onboarding conversations, it is essential to consider platforms that can provide deeper insights into user interactions. Tools like IBM Watson and Google Cloud Natural Language offer advanced natural language processing capabilities. These technologies can analyze conversations, extracting sentiments and identifying keywords that indicate where users might be struggling during onboarding.
Microsoft Azure Text Analytics can further enhance onboarding drop-off analysis by providing real-time feedback on user sentiment and engagement levels. Additionally, Chatbase enables teams to track user interactions across various channels, helping to pinpoint areas where conversations stall. By integrating these tools, businesses can create a comprehensive understanding of onboarding experiences, enabling them to adapt strategies and reduce drop-off rates effectively. Leveraging these AI technologies not only improves user engagement but ultimately drives customer success and satisfaction.
- IBM Watson
The integration of AI in analyzing user onboarding conversations is essential for identifying potential drop-off signals. By employing advanced AI technologies, businesses can harness the power of data to enhance their onboarding processes. One notable AI platform specializes in natural language processing, enabling the analysis of user conversations with remarkable accuracy.
This platform provides insights into user engagement and identifies key patterns that signal when and why users might abandon their onboarding journey. With its ability to process vast amounts of data quickly, it helps organizations understand user sentiment and pain points during the onboarding phase. This understanding is vital for optimizing communication strategies and tailoring follow-up actions, ultimately reducing drop-off rates and improving the overall user experience.
- Google Cloud Natural Language
Google Cloud Natural Language provides powerful text analysis features that can enhance onboarding drop-off analysis. By processing user conversations, you can uncover critical insights about customer experiences during the onboarding journey. This tool analyzes sentiment, entity recognition, and syntax to extract meaningful data from user interactions, enabling you to pinpoint areas where users might disengage.
Utilizing Google Cloud Natural Language allows teams to assess not just the content but also the emotional tone behind user feedback. This analysis can highlight specific phrases or issues leading to drop-offs. By understanding these patterns, businesses can implement targeted strategies to improve user engagement. As a result, Google Cloud Natural Language becomes an essential asset in monitoring and refining the onboarding process, turning conversations into actionable insights.
- Microsoft Azure Text Analytics
Microsoft Azure Text Analytics offers powerful capabilities for analyzing user onboarding conversations. This tool leverages natural language processing to provide insights into user sentiments and identify drop-off signals during the onboarding journey. By analyzing text data, organizations can pinpoint conversation patterns that may lead to user disengagement.
When conducting onboarding drop-off analysis, utilizing Azure's tools allows for the extraction of valuable information from customer interactions. The service categorizes sentiment, identifies key phrases, and offers language detection features. These insights help organizations better understand user concerns and enable proactive measures to address potential blockers. By integrating Azure Text Analytics into your analysis workflow, you can significantly enhance your understanding of user motivations and obstacles during onboarding. This approach ultimately supports improved user experiences and higher retention rates.
- Chatbase
Chatbase is an innovative tool that plays a pivotal role in analyzing onboarding conversations. By parsing through user interactions, it identifies critical points where customers may lose interest or face challenges. This deep analysis aids businesses in understanding user behavior during the onboarding process, providing insights into potential drop-off signals. Recognizing these patterns is essential for optimizing the onboarding journey and ensuring users receive the support they need to succeed.
With Chatbase, organizations can streamline their communication strategies based on data-driven insights. It allows teams to automate responses for common queries, thereby enhancing user engagement. Furthermore, by pinpointing frequent drop-off moments in onboarding conversations, businesses can proactively address concerns and improve the overall user experience. This results in significant enhancements in user retention rates, making Chatbase a valuable asset for effective onboarding drop-off analysis.
Conclusion: The Future of Onboarding Drop-off Analysis with AI
As we look toward the future of onboarding drop-off analysis, the integration of AI presents transformative opportunities. By analyzing user conversations, businesses can gain insights into the factors leading to drop-offs during the onboarding process. This understanding not only allows for identification of challenges but also facilitates the development of tailored solutions that improve user experience and drive retention.
AI technologies will enable organizations to analyze vast amounts of conversation data in real-time, uncovering patterns and signals that were previously difficult to detect. With tools like Insight7 and others, companies can refine their onboarding strategies based on actionable insights. Ultimately, embracing AI in onboarding drop-off analysis is key to enhancing user satisfaction and fostering long-term engagement.
💬 Questions about Using AI to Analyze User Onboarding Conversations for Drop-Off Signals?
Our team typically responds within minutes