Top 7 AI Agent Types for Predictive Qualitative Insights
-
Hello Insight
- 10 min read
Predictive AI Agents have revolutionized the way we gather and interpret qualitative insights. By intelligently analyzing vast amounts of data, these agents provide researchers with nuanced understandings of customer sentiments and behaviors. This technology enables businesses to identify trends that humans might overlook, ultimately enhancing decision-making processes.
In this section, we will explore various types of Predictive AI Agents tailored for qualitative analysis. Understanding their functionality and applications will empower organizations. As we delve deeper, the focus will remain on how these innovative tools can effectively transform qualitative data into actionable insights, streamlining research efforts and improving outcomes.
Analyze & Evaluate Calls. At Scale.
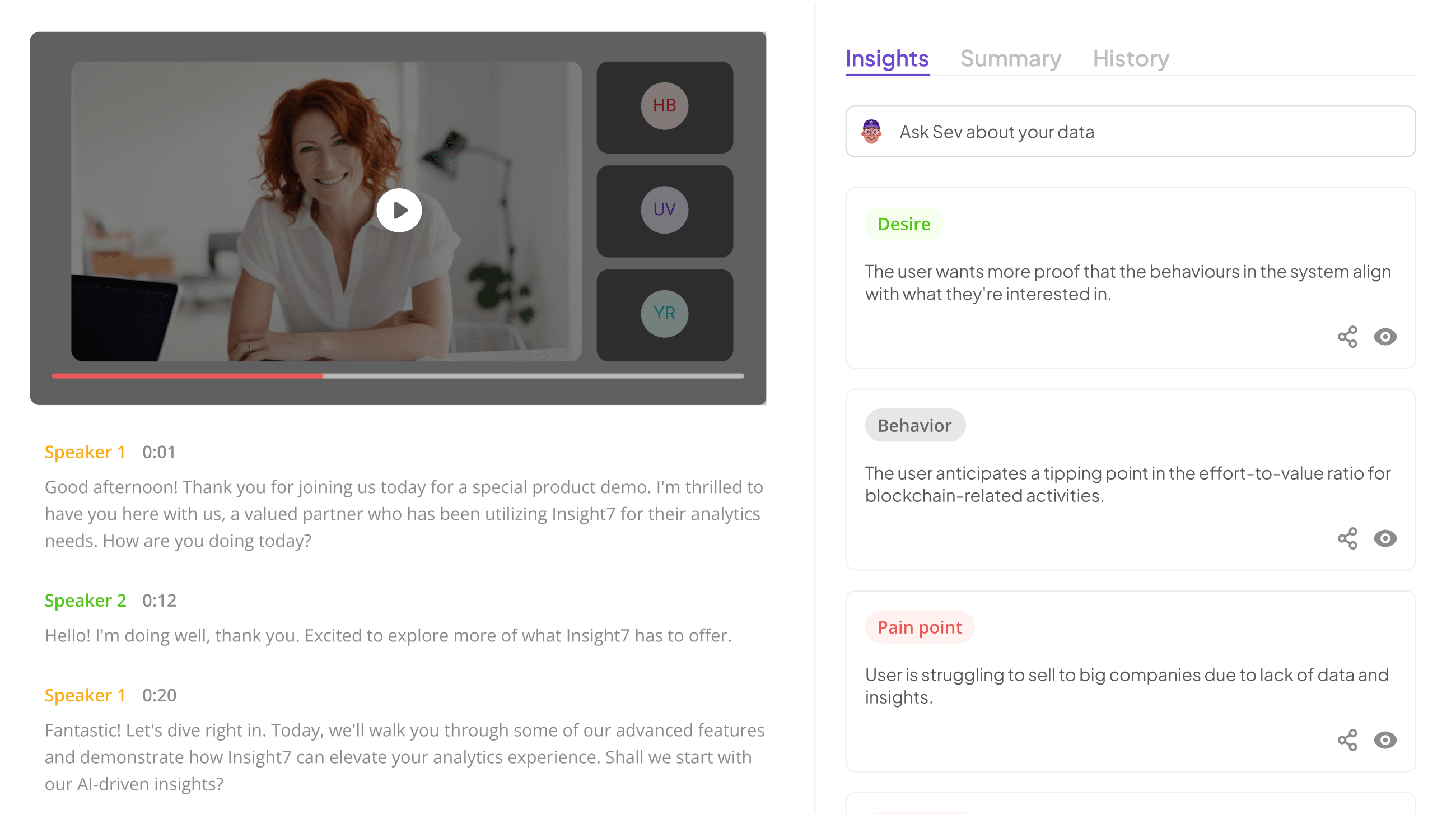
Exploring Predictive AI Agents for Qualitative Analysis
Exploring Predictive AI Agents for Qualitative Analysis reveals how technology enhances our understanding of complex data. Predictive AI Agents offer unique capabilities that streamline qualitative research processes. These agents can analyze large sets of qualitative data, identifying patterns, themes, and insights that might be overlooked by traditional methods.
Two key types of Predictive AI Agents are Classification and Clustering Agents. Classification Agents categorize qualitative data into predefined groups, making it easier to glean insights. In contrast, Clustering Agents group similar data without prior categorization, allowing researchers to discover new relationships and meanings within the data. Together, these agents create a powerful toolkit for extracting valuable insights across various domains, from market research to social media analysis. Their ability to automate insights generation significantly enhances the efficiency and accuracy of qualitative analysis, providing a competitive advantage in todayโs data-driven landscape.
Types of Predictive AI Agents
Predictive AI agents come in various forms, each designed to handle specific tasks in qualitative analysis. Understanding these types allows organizations to implement the best fit for their research needs. Among the prominent ones are classification agents and clustering agents. Classification agents categorize data based on predefined labels, ensuring insights are targeted and relevant. In contrast, clustering agents group data based on similarities, making it easier to identify patterns hidden within large datasets.
Each type of predictive AI agent serves a unique role in enhancing qualitative insights. Classification agents streamline the process of organizing information, guiding decision-making processes. Clustering agents facilitate deeper analysis by revealing connections between seemingly unrelated data points. By effectively leveraging these predictive AI agents, organizations can unlock more profound insights, driving better strategies and decision-making in their qualitative research efforts.
- Classification Agents
Classification agents play a pivotal role in the realm of predictive AI agents, especially when addressing qualitative insights. These agents excel at organizing data into predefined categories based on specific characteristics. This process enables researchers to derive meaningful patterns and trends from the vast amount of qualitative data available. For instance, classification agents can analyze customer feedback and sort it into categories like satisfaction, dissatisfaction, or suggestions, making it easier for businesses to identify emerging issues and opportunities.
Moreover, the effectiveness of classification agents lies in their ability to enhance decision-making processes. By accurately categorizing qualitative data, these agents allow organizations to focus on targeted areas for improvement. This not only streamlines the research process but also provides valuable context for strategic planning. Utilizing classification agents ensures that stakeholders can rely on structured insights, ultimately leading to better outcomes and more informed actions in the realm of qualitative analysis.
- Clustering Agents
Clustering Agents play a pivotal role in predictive AI agents, particularly in qualitative research. They excel at categorizing data into meaningful groups based on shared attributes, allowing researchers to uncover hidden patterns and insights. By analyzing large datasets, clustering agents can identify segments that might otherwise go unnoticed. This segmentation is crucial for tailoring strategies, as it helps businesses understand diverse customer needs and preferences.
These agents utilize various algorithms, such as K-means and hierarchical clustering, to process and organize data effectively. The primary goal is to simplify complex information while highlighting significant trends and relationships. For instance, in customer feedback analysis, clustering agents can group similar responses, enabling teams to focus on specific themes or issues. This targeted analysis enhances decision-making and supports a more insightful approach to qualitative research, ultimately driving improved strategies and outcomes.
Benefits of Using Predictive AI Agents in Qualitative Research
Predictive AI Agents play a pivotal role in enhancing qualitative research by offering efficient data analysis and insightful interpretations. One key benefit is their ability to process vast amounts of qualitative data quickly. This automation allows researchers to focus on meaningful insights rather than getting lost in the minutiae of data management. With predictive AI, user comments, testimonials, and feedback can be efficiently extracted and analyzed, revealing underlying patterns that might otherwise be overlooked.
Another significant advantage is their capability to provide real-time insights. Predictive AI Agents can generate summaries and highlight pivotal trends based on users' needs, aiding in prompt decision-making. By leveraging these advanced technologies, researchers can enhance their understanding of complex topics, leading to improved strategies and outcomes. Consequently, the integration of Predictive AI Agents into qualitative research not only streamlines processes but also enhances the overall quality of insights derived from the data.
- Enhanced Data Interpretation
Understanding Enhanced Data Interpretation is essential for extracting meaningful insights from qualitative data. Predictive AI Agents play a significant role in this process by automating data analysis and improving accuracy. By identifying patterns within large datasets, these agents provide nuanced interpretations that traditional methods often miss.
Enhanced Data Interpretation involves several key aspects. First, it allows for the integration of diverse data sources, offering a comprehensive view of customer signals. Second, Predictive AI Agents can uncover underlying trends, which can be vital for timely decision-making in competitive environments. Lastly, these agents streamline collaboration by presenting insights in an easily understandable format, reducing the time and effort needed to convert data into actionable strategies. With these capabilities, organizations can respond to market needs more effectively, transforming qualitative insights into real business outcomes.
- Automated Insights Generation
Automated insights generation is a transformative process that enhances the efficiency and depth of qualitative research. When integrated with predictive AI agents, this approach allows researchers to swiftly analyze vast amounts of data and draw actionable insights. Rather than spending countless hours aggregating information, stakeholders can effortlessly access a well-organized overview of key findings, enabling informed decision-making based on customer feedback and market trends.
This process typically involves several critical steps. First, predictive AI agents gather and process data from various sources, such as customer interviews or call transcripts, pulling out themes, pain points, and desires. Next, these insights are presented in a user-friendly format, often accompanied by clear evidence such as direct quotes from participants. This ensures that the insights are contextually relevant and reliable. By facilitating rapid insights generation, organizations can respond to changing market dynamics effectively and maintain a competitive edge.
Extract insights from interviews, calls, surveys and reviews for insights in minutes
Top AI Agent Tools for Predictive Qualitative Insights
In the landscape of qualitative research, selecting the right tools is crucial for gathering predictive insights. AI agent tools are at the forefront of transforming how organizations interpret data. By utilizing innovative technologies, these tools can analyze customer interactions, identify themes, and generate actionable insights, paving the way for informed decision-making.
Among the standout tools for predictive qualitative insights are insight7, MonkeyLearn, RapidMiner, KNIME, and IBM Watson. Each offers unique features designed to simplify data analysis. For instance, insight7 excels in transcribing and summarizing calls, revealing customer pain points and desires through detailed insights. MonkeyLearn provides a user-friendly interface for text analysis, allowing users to uncover patterns efficiently. Meanwhile, RapidMiner and KNIME are versatile platforms that support extensive data workflows, enabling deep analysis of large datasets. IBM Watson leverages sophisticated machine learning capabilities to deliver advanced qualitative findings. By integrating these tools, businesses can enhance their understanding and responsiveness to customer needs in today's dynamic market.
insight7
In the realm of advanced analytics, the role of predictive AI agents is vital for decoding qualitative insights. These agents, characterized by their ability to process and interpret large volumes of data, serve as digital catalysts for decision-making. Organizations often face the challenge of extracting actionable insights from fragmented data sources. Predictive AI agents synthesize this information, enabling businesses to foster informed strategies and gain a competitive edge.
Real-world applications of these agents are diverse, spanning industries from retail to healthcare. For instance, a retail brand might utilize clustering agents to segment customer feedback, revealing unique preferences that drive sales. Similarly, classification agents assist healthcare providers in predicting patient outcomes based on historical data. By incorporating these AI-driven tools into their analysis processes, companies can significantly enhance their operational efficiency and responsiveness to market trends. The integration of predictive AI agents marks a transformative step towards more refined qualitative research and actionable insights.
- Features and Capabilities
Predictive AI agents offer diverse features and capabilities essential for qualitative research. These sophisticated algorithms analyze data to identify patterns, which can lead to actionable insights. By employing natural language processing (NLP), these agents understand and interpret complex language, allowing them to extract relevant information from large datasets efficiently. Additionally, intuitive user interfaces enhance the user experience, making it easier to access and comprehend the results generated.
The integration of machine learning capabilities enables predictive AI agents to evolve continually. As they process more data, their accuracy improves, refining the insights they provide. Real-time data analysis allows organizations to respond quickly to shifts in consumer behavior or market trends. Overall, these features contribute to a more nuanced understanding of qualitative data, assisting businesses in making informed decisions that align with their strategic objectives.
- Real-world Applications
Predictive AI Agents are increasingly being utilized across various industries, revolutionizing how organizations analyze qualitative insights. These agents are adept at processing vast amounts of data, surfacing patterns that human analysts might overlook. For instance, in customer service, Predictive AI Agents can assess phone calls to ensure compliance and enhance quality assurance. By analyzing conversations against predefined criteria, organizations can refine their training methods and improve overall service quality.
Another powerful application is in market research. Here, Predictive AI Agents sift through customer feedback, allowing businesses to gain profound insights into customer preferences and sentiment. By transforming unstructured data into actionable insights, they enable companies to adapt their strategies swiftly. The versatility of these agents in diverse applications underscores their critical role in enhancing decision-making and fostering a customer-centric approach across many sectors.
Other Leading Tools
In addition to the dominant Predictive AI Agents, various other leading tools are making significant contributions to qualitative insights. First on the list is MonkeyLearn, known for its powerful text analysis capabilities. This tool allows users to extract key themes and sentiments from customer feedback, making it invaluable for businesses seeking to understand consumer perceptions.
Next, RapidMiner stands out with its user-friendly interface, enabling data preparation and machine learning processes without deep technical expertise. This tool provides extensive analytical capabilities, helping users uncover hidden patterns in qualitative data. Following that is KNIME, a platform renowned for its modular approach to data analytics. It facilitates easy integration of diverse data sources, enhancing the richness of insights derived from customer interactions. Lastly, IBM Watson offers advanced AI and machine learning features, ensuring businesses obtain predictive insights from complex data sets. These tools collectively expand the toolkit available for extracting meaningful insights from qualitative research.
- MonkeyLearn
Predictive AI agents serve a critical role in transforming qualitative insights by automating data analysis. One such tool specializes in simplifying the complexities of data extraction and interpretation. With its robust capabilities, this agent streamlines the processing of customer feedback, making it easier for businesses to derive actionable insights.
๐ฌ Questions about Top 7 AI Agent Types for Predictive Qualitative Insights?
Our team typically responds within minutes
Users can leverage this agent to analyze vast streams of qualitative data, such as social media comments, reviews, and survey responses. The intuitive interface allows for customization according to the specific needs of a research project. By employing advanced natural language processing, it helps uncover patterns and sentiments that may not be immediately apparent.
Additionally, the effective use of predictive AI agents can enhance decision-making processes and improve stakeholder communication. Adopting this technology not only saves time but also enriches the quality of insights derived from qualitative research, ultimately guiding businesses toward informed strategies and operational improvements.
- RapidMiner
RapidMiner serves as a vital tool for creating Predictive AI Agents aimed at deriving qualitative insights. This platform allows users to seamlessly bring together data from various sources, facilitating deeper analysis. By utilizing features like data mining and machine learning, users can create predictive models that offer valuable insights based on historical data.
One engaging aspect of RapidMiner is its project management capabilities. Users can upload audio transcripts, enabling them to analyze customer feedback efficiently. The platform simplifies data interpretation, allowing researchers to identify trends and patterns that inform marketing strategies. With user-friendly interfaces and powerful analytical tools, RapidMiner empowers businesses to transform raw data into actionable insights, enhancing their understanding of customer behavior and preferences.
Ultimately, employing RapidMiner in the journey of utilizing Predictive AI Agents fundamentally improves research outcomes, making it a preferred choice in the realm of qualitative analysis.
- KNIME
The platform provides a user-friendly environment ideal for exploring predictive AI agents and their capabilities in qualitative analysis. Its straightforward design allows anyone in a business to engage with the system, eliminating the need for specialized expertise. This democratization of insights enables teams to convert raw customer dataโlike calls and interviewsโinto valuable reports for decision-making, enhancing overall understanding of customer experiences.
In this setting, predictive AI agents excel at analyzing vast amounts of qualitative data. Users can easily access a library of recorded conversations, generate transcripts, and visualize key insights through an efficient interface. Sharp features allow these agents to identify pain points, desires, and behaviors, presenting evidence through customer quotes and specific examples. By integrating these insights into a project, businesses can systematically address common themes and improve customer relations, creating a more informed decision-making process.
- IBM Watson
IBM Watson stands out as a unique tool in the realm of Predictive AI Agents for qualitative insights. This AI system employs natural language processing and machine learning to analyze vast datasets effectively. Its ability to understand context and sentiment allows organizations to gain profound insights from qualitative data, making decision-making processes much more insightful.
This AI agent excels at identifying patterns and trends within data that may not be immediately apparent to human researchers. By automating the analysis of textual information, it significantly reduces the time needed for data interpretation. Furthermore, Watsonโs adaptability means that businesses can customize it to meet their specific needs, enhancing its effectiveness in various sectors, including customer service, marketing, and compliance. Organizations looking to improve their qualitative research can benefit from utilizing this advanced predictive technology, leading to more informed strategies and outcomes.
Steps to Implementing Predictive AI Agents
To implement predictive AI agents effectively, begin by clearly defining your research goals. This involves aligning the agents with your specific objectives and prioritizing the key insights you intend to obtain. A well-defined purpose will ensure that the AI agents you utilize are tailored to meet the needs of your analysis, enabling more focused and relevant outcomes.
Next, selecting the right predictive AI agent is crucial. You should assess criteria such as the agent's capabilities, the ease of customization, and its scalability to match your organizational requirements. The selected agent should not only be efficient in processing data but also robust enough to adapt as your needs evolve. Once these foundational steps are established, you can proceed to implement the predictive AI agents, ensuring you monitor their performance to refine and optimize their usage continuously. By following these steps, you set a solid foundation for leveraging predictive AI agents in your qualitative research endeavors.
Step 1: Define the Research Goals
To successfully navigate the world of Predictive AI Agents, the first crucial step is to define your research goals. Understanding your objectives lays the groundwork for effective analysis and ensures that your insights will be meaningful and actionable. Clearly articulated goals help in aligning AI tools with your specific needs, leading to more relevant outcomes.
When defining your research goals, consider the kinds of insights you want to gain. These could range from understanding customer behavior patterns to identifying emerging trends in your industry. Prioritizing the key insights you hope to achieve can also streamline your approach, allowing you to focus on the most impactful data. In this way, you set a precise framework that will guide your selection of the right Predictive AI Agents, ensuring alignment with your overall research objectives.
- Align Agents with Objectives
To align Predictive AI Agents with your specific objectives, it's crucial to clearly define what you aim to achieve from your qualitative analysis. Establishing precise goals helps determine which agents will be most effective in meeting your needs. By understanding your desired outcomes, you can better leverage the capabilities of the agents you select.
Next, prioritize the insights that matter most to your project. This will streamline your process and ensure that your Predictive AI Agents focus on generating actionable data relevant to your objectives. Regularly reassess these objectives to ensure alignment, allowing your agents to adapt as your research evolves. Success in predictive insights hinges on this alignment, creating a feedback loop that enhances the quality and relevance of your findings.
- Prioritize Key Insights
To prioritize key insights effectively, one must first establish research goals that align with the objectives of the qualitative analysis. By defining these goals, you will clarify which insights matter most for your specific project. This step ensures that the predictive AI agents you choose are focused on extracting relevant and actionable information. With a clear direction, you can direct your efforts toward gathering insights that genuinely benefit your understanding and decision-making processes.
Next, it is essential to evaluate the insights produced by predictive AI agents critically. Not all gathered data will hold equal value; attention should focus on themes, patterns, and recurring mentions that highlight critical customer pain points or experiences. By systematically assessing these insights, organizations can make informed decisions that resonate with user needs. This approach transforms raw data into meaningful narratives that guide strategy and enhance overall impact.
Step 2: Select the Right AI Agent
Selecting the right AI agent is a crucial step in harnessing predictive AI for qualitative insights. Begin by identifying your specific research goals. Each AI agent excels in different aspects, and understanding their unique capabilities can guide your decision-making. For instance, classification agents are beneficial when you need to label and organize qualitative data, whereas clustering agents can reveal hidden patterns within your data set.
After defining your objectives, consider the criteria for selection. Look for scalability โ a good agent should evolve alongside your data needs. Additionally, customization options are essential. A flexible AI agent allows you to tailor its operations to suit your specific analytical needs, enhancing the overall effectiveness of your research. Ultimately, choosing the right predictive AI agent not only streamlines your processes but also improves the accuracy and relevance of your insights.
- Criteria for Selection
When selecting predictive AI agents for qualitative insights, several important criteria must guide your decision-making process. First, consider the agent's ability to align with your specific research objectives and how effectively it can translate complex data into actionable insights. The effectiveness of predictive AI agents often hinges on their capability for customization in response to varying datasets and research needs.
Next, assess the scalability of the agent. A robust solution should effectively handle growing data volumes and accommodate evolving analytical requirements over time. Additionally, ensure the predictive AI agent provides comprehensive support and features that streamline data interpretation. Take into account user-friendliness and integration capabilities with existing systems, which can significantly impact your team's overall efficiency in generating insights. By adhering to these criteria, you can confidently choose a predictive AI agent that meets your analytical demands.
- Customization and Scalability
Customization is crucial when integrating predictive AI agents into qualitative research. Each project may have unique specifications, requiring tailored adjustments to meet distinct goals. Predictive AI agents offer a range of customizable features, allowing users to modify variables according to their specific needs. By shaping these agents, teams can create a more intuitive interface that aligns with their research objectives and enhances user experience.
Scalability plays a vital role as businesses expand their analytical needs. Predictive AI agents are designed to grow alongside an organization, adapting to increasing data volumes and complexity without losing efficiency. Whether itโs adjusting processing capabilities or expanding features, scalability ensures that your insights remain accurate and actionable as projects evolve.
In the age of data, harnessing customizable and scalable predictive AI agents can significantly improve the quality and depth of qualitative insights. This adaptability ultimately drives better decision-making and a clearer understanding of customer needs.
Conclusion: The Future of Predictive AI Agents in Qualitative Insights
The future of predictive AI agents in qualitative insights promises to reshape how we understand and interpret human experiences. As technology evolves, these agents will employ advanced algorithms to deliver deeper and more nuanced insights into consumer behavior. By embracing specific criteria and evaluation methods, organizations can harness the full potential of predictive AI agents, driving innovation in qualitative research.
Furthermore, the integration of these agents into research frameworks will enhance decision-making processes. This ensures businesses remain agile in an ever-changing market landscape. As predictive AI agents continue to advance, they will become indispensable tools for uncovering meaningful patterns in qualitative data, ultimately leading to better customer understanding and stronger business strategies.
๐ฌ Questions about Top 7 AI Agent Types for Predictive Qualitative Insights?
Our team typically responds within minutes