Top 10 AI Agents for Interview Analysis in Qualitative Research
-
Hello Insight
- 10 min read
Interview Analysis AI is revolutionizing the way qualitative research is conducted by enabling researchers to extract meaningful insights from interviews efficiently. In a digital age driven by vast data volumes, traditional methods often fall short, making AI an invaluable tool. By leveraging advanced algorithms, these AI systems streamline the analysis process, allowing researchers to focus on interpreting results rather than sifting through extensive data.
Furthermore, Interview Analysis AI enhances accuracy by identifying patterns and themes that may not be immediately apparent to human analysts. This improved precision is critical in maintaining reliability in qualitative research, ensuring that insights are not skewed by personal biases. As we explore the top AI agents available for interview analysis, the immense potential of these tools will become increasingly clear, paving the way for more robust and insightful research practices.
Analyze & Evaluate Calls. At Scale.
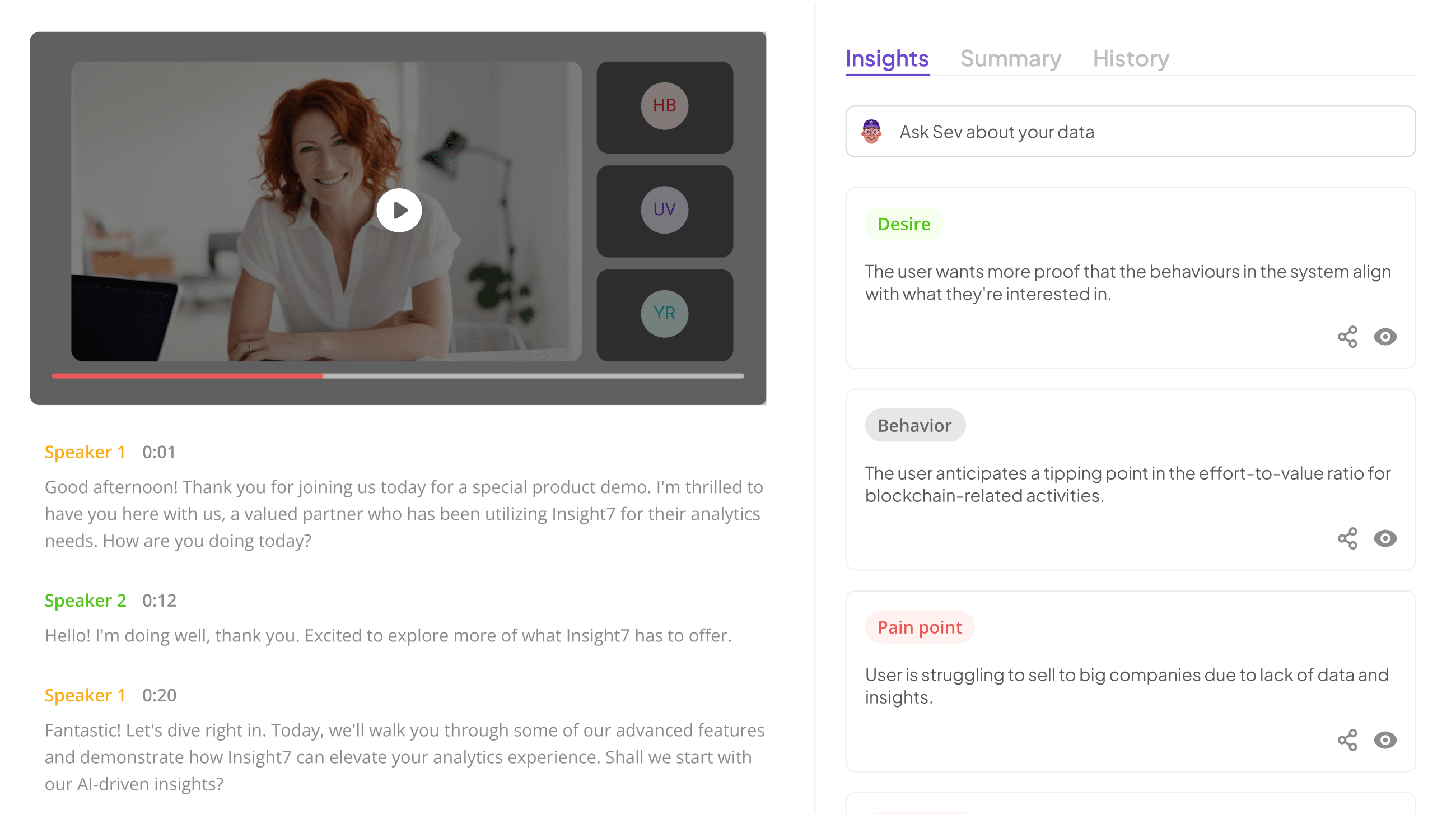
The Significance of AI Agents in Interview Analysis
AI agents are transforming the landscape of interview analysis in qualitative research. By utilizing Interview Analysis AI, researchers can handle large sets of data more efficiently, which is especially important in today's fast-paced environment. Traditional methods of qualitative analysis often struggle to keep up with the sheer volume of interviews collected, leading to delays in deriving actionable insights. AI technologies streamline this process, enabling researchers to focus on interpreting the data rather than getting bogged down in manual processing.
Moreover, AI agents enhance the accuracy and consistency of data analysis. They are programmed to eliminate human bias, ensuring that insights are based solely on the information presented. This objectivity is crucial, as it allows researchers to draw reliable conclusions from interviews. In essence, AI agents not only accelerate the analysis but also improve the quality of insights derived, thus enabling organizations to make informed decisions swiftly and confidently.
Benefits of Using AI for Interview Analysis
Using Interview Analysis AI offers significant advantages for researchers in qualitative studies. One of the primary benefits is enhancing data accuracy and consistency. Traditional methods often involve manual processes that can introduce errors and bias. With AI, the analysis becomes more reliable, allowing for a clearer understanding of participant feedback.
Another major advantage is the speed at which AI can process data. AI tools can swiftly analyze vast amounts of information, significantly reducing the time required to interpret interviews. This efficiency enables researchers to derive insights quickly and respond to trends or changes in real time. Such timely insights can be pivotal in adapting strategies and staying ahead in a competitive landscape. By integrating Interview Analysis AI into qualitative research, researchers can transform their approach, leading to more profound insights and informed decisions.
- Enhancing data accuracy and consistency
In qualitative research, enhancing data accuracy and consistency is vital for meaningful insights. Interview Analysis AI helps achieve this by systematically processing large amounts of data while minimizing human errors. By utilizing advanced algorithms, these AI agents can transcribe and analyze interviews, ensuring that the information gathered reflects the participants' true sentiments. This level of precision is invaluable for researchers seeking to make informed decisions based on reliable data.
To further improve data accuracy, it is crucial to implement robust methodologies. First, employing AI tools for real-time transcriptions can immediately highlight discrepancies or errors in responses. Second, utilizing analytics dashboards allows researchers to visualize trends and patterns, ensuring that no critical insights are overlooked. Lastly, regularly updating the AI models with new data helps maintain their relevance and accuracy in analyzing diverse participant responses. Collectively, these strategies promote a seamless, consistent analytical process, ultimately enriching qualitative research outcomes.
- Speeding up the analysis process
In qualitative research, the analysis process can often be time-consuming and labor-intensive. Interview analysis AI significantly accelerates this workflow by automating several key tasks. With the ability to parse through large datasets, these AI tools can quickly identify themes and patterns in interview transcripts, saving researchers hours of manual work. By employing natural language processing (NLP), they accurately interpret sentiment and context, ensuring that insights are captured effectively.
Additionally, integrating interview analysis AI allows for a more streamlined approach to data management. Researchers can input numerous interviews simultaneously, enabling them to supplement their findings with diverse perspectives. The capability to compare and contrast data from different sources enriches the analysis, leading to deeper insights. In summary, those utilizing interview analysis AI will find themselves with more time to focus on drawing meaningful conclusions from their research, ultimately enhancing the quality and speed of their qualitative work.
Overcoming Challenges in Qualitative Research with AI
In the realm of qualitative research, overcoming challenges with Interview Analysis AI is essential for gaining valuable insights. One predominant issue researchers face is managing the overwhelming amount of data generated from interviews. Traditional methods often struggle to keep pace, making it difficult to extract meaningful insights in a timely manner. By utilizing artificial intelligence, researchers can efficiently process large datasets, transforming hours of manual analysis into streamlined, automated workflows.
Another challenge is ensuring the integrity of the analysis, as biases can skew results. AI tools help mitigate bias by employing standardized algorithms that treat all data points uniformly, leading to more objective outcomes. This promotes a more accurate understanding of participant sentiments and motivations. Ultimately, by addressing these challenges, Interview Analysis AI empowers researchers to translate insights into actionable strategies, fostering informed decision-making in their organizations.
- Handling large volumes of data
Handling large volumes of data in qualitative research can be quite challenging. Traditional methods may prove inefficient as the amount of information grows. However, Interview Analysis AI provides the tools necessary to streamline this process, making data handling more manageable and insightful. By automating the transcription and organization of audio recordings, these AI agents allow researchers to focus on deriving meaning from the data instead of getting bogged down by its sheer volume.
Effective handling of large data sets involves several key strategies. First, implementing organized project management within the AI platform helps categorize and analyze interviews efficiently. Next, utilizing features that summarize data trends aids in quickly identifying significant patterns. Lastly, enabling advanced search functions allows researchers to retrieve specific information rapidly, facilitating more focused analysis. By embracing these strategies, qualitative researchers can unlock the full potential of the data they collect, ensuring their studies are both thorough and insightful.
- Ensuring unbiased analysis
Ensuring unbiased analysis in qualitative research is essential for garnering valid insights. When using Interview Analysis AI tools, effective measures must be in place to mitigate bias. Firstly, it is crucial to analyze data from a diverse set of sources to capture a range of perspectives. This helps prevent skewed interpretations and enhances the reliability of findings.
Moreover, employing consistent coding frameworks during data analysis aids in maintaining objectivity. Automated algorithms can support standardized approaches, reducing human error and minimizing subjective influences. Additionally, incorporating anonymous feedback can bring to light diverse viewpoints, further ensuring an unbiased evaluation process. Ultimately, investing in intuitive AI tools designed for interview analysis can promote transparency in qualitative research, fostering trustworthy insights that drive informed decision-making.
Top AI Agents for Interview Analysis in Qualitative Research
In the realm of qualitative research, Interview Analysis AI agents are transforming how researchers process and extract insights from interviews. These tools streamline the analysis of large datasets, speeding up the review process and enhancing data accuracy. Researchers benefit from AI’s capability to minimize human error, enabling them to focus on interpreting the results rather than getting lost in data.
When selecting top AI agents for interview analysis, it's essential to consider a range of features. Notable AI agents excel at recognizing speech patterns and conducting semantic analysis. For example, Otter.ai provides real-time transcription services, while MonkeyLearn specializes in text mining. By utilizing these advanced technologies, researchers can derive meaningful insights and translate them into actionable strategies, significantly enhancing their decision-making capabilities in qualitative studies.
Extract insights from interviews, calls, surveys and reviews for insights in minutes
List of Top AI Tools
In the domain of qualitative research, utilizing the right Interview Analysis AI tools can profoundly enhance the quality of insights derived from interview data. These tools offer diverse functionalities, from automatic transcription to semantic analysis, allowing researchers to uncover themes and patterns quickly. Their efficiency eliminates the tedious manual work often associated with analyzing interviews, enabling researchers to focus on drawing actionable conclusions from their findings.
Among the top AI tools for interview analysis, several stand out due to their unique features and user experiences. For instance, some tools excel in transcribing conversations accurately, while others utilize advanced algorithms for semantic analysis. Identifying specific capabilities that align with your research needs is crucial. This ensures that the selected Interview Analysis AI tools not only simplify data processing but also enrich the overall qualitative research experience, ultimately transforming informative results into impactful decisions.
Insight7
Insight7 delves deep into the transformative role of Interview Analysis AI within qualitative research. As data-driven insights become increasingly critical, the demand for efficient analysis tools grows. Interview Analysis AI empowers researchers to sift through vast amounts of interview data, uncovering essential themes and patterns that might be overlooked through traditional methods. This capability allows teams to act decisively, turning raw data into actionable insights.
Moreover, these AI tools streamline the interview process by providing features like speech recognition and semantic analysis. They not only speed up the analysis but also enhance accuracy, minimizing the risk of human bias in interpreting responses. With AI systems, teams can efficiently manage collaboration, integrating insights across various platforms and ensuring that important information is easily accessible. Adopting Interview Analysis AI is not merely a technical enhancement; it represents a crucial strategic shift in how organizations engage with qualitative data.
- Description and Key Features
In the realm of qualitative research, Interview Analysis AI serves as a transformative tool, enhancing the efficiency and accuracy of data interpretation. This section outlines the key features that define these advanced agents. The remarkable capability to automate the transcription and analysis of interviews stands out. By converting audio recordings into text, these tools eliminate the drudgery of manual transcription, allowing researchers to focus on deriving insights from the data.
Another essential feature is the extraction of themes and sentiment from responses. Interview Analysis AI can identify recurring patterns, pain points, and customer sentiments by processing large volumes of qualitative data swiftly. This functionality not only provides a comprehensive understanding of participant perspectives but also offers valuable evidence, such as quotes from respondents, supporting the insights. This blend of automation and analytical prowess empowers researchers to drive informed decisions based on reliable data. Ultimately, Interview Analysis AI democratizes access to insights, making it easier for teams to collaborate and share findings in an accessible manner.
- Use Cases and Success Stories
AI agents for interview analysis have found numerous applications across various sectors, transforming how qualitative research is conducted. A few compelling use cases include the evaluation of customer service interactions and the extraction of actionable insights from user interviews. For example, AI tools can effortlessly transcribe and analyze large quantities of audio data, enabling researchers to distill essential themes, sentiments, and trends. This sophisticated capability not only saves time but also enhances the quality of insights obtained from interviews.
Success stories abound, with organizations reporting significant improvements in both efficiency and accuracy when employing AI agents. By automating the analysis process, researchers can focus on strategic decision-making rather than being bogged down by tedious manual transcription tasks. Enhanced data accuracy and the ability to effortlessly generate reports have allowed many to elevate their qualitative research efforts, driving impactful outcomes in their projects.
Other Notable AI Agents
In the realm of interview analysis AI, several notable agents stand out beyond our top ten list. These tools offer unique functionalities that enhance qualitative research further. For instance, Otter.ai is renowned for its speech recognition capabilities, providing a seamless transcription process that captures nuances in conversations. This accuracy allows researchers to focus on analyzing content rather than manually revising transcripts.
Similarly, Trint excels in semantic analysis, enabling users to search and edit transcripts efficiently. Its intuitive interface makes it easy to visualize key themes from interviews, thus supporting comprehensive qualitative data synthesis. Additionally, tools like MonkeyLearn and NVivo bring advanced text mining features to the table, facilitating in-depth analysis of sentiment and trends within interview data. These agents contribute significantly to improving the effectiveness and reliability of qualitative research, making them invaluable assets in the interview analysis AI landscape.
- Recognizing Speech Patterns in Interviews
Analyzing speech patterns in interviews is crucial for extracting meaningful insights from qualitative research. When we speak, our words, tone, and rhythm reveal significant details about our thoughts and emotions. Recognizing these patterns assists researchers in identifying underlying themes and sentiments that may not be directly expressed in responses.
Interview Analysis AI tools can automate the process of auditory analysis, making it easier to spot trends and anomalies in conversation. For instance, AI agents like Otter.ai and Trint excel at transcribing audio, allowing researchers to focus on the implications of what was said rather than the mechanics of transcribing. These tools can highlight inconsistencies, pauses, and emotional cues, which can lead to a deeper understanding of user perspectives. By leveraging such technologies, researchers can efficiently convert spoken data into actionable insights, thus improving the overall quality of qualitative research.
- Otter.ai
In the realm of interview analysis AI, one standout tool offers users a seamless experience for capturing and transcribing conversations. The platform utilizes advanced algorithms to transcribe interviews accurately, which saves researchers both time and effort. With features tailored to qualitative research, it allows users to highlight key sections, add comments, and organize content effectively.
Moreover, the tool integrates with various video conferencing platforms, enhancing its usability across diverse research scenarios. This accessibility makes it especially valuable for teams conducting interviews remotely. Researchers can rely on this AI tool to generate accurate transcripts that form the backbone of their qualitative analysis. By streamlining the transcription process, users can focus on extracting meaningful insights from their interviews, thus enhancing the overall quality of their research.
- Trint
In the evolving realm of qualitative research, sophisticated AI tools significantly enhance the process of interview analysis. Among these tools, a leading option specializes in transcribing spoken language into text, making it highly beneficial for researchers. This capability allows users to create accurate records of conversations efficiently, transforming lengthy interview sessions into manageable, searchable text documents. Such insights are invaluable when stakeholders need to evaluate themes and patterns throughout their research.
Furthermore, the AI tool excels in producing high-quality transcripts with remarkable speed and precision. By automating transcription, it significantly reduces the time researchers typically spend on manual documentation. This efficiency not only streamlines data management but also enables a deeper focus on analyzing content and deriving meaningful insights from interviews. Overall, leveraging this tool enhances interview analysis, ultimately leading to richer qualitative research outcomes.
- Semantic Analysis and Text Mining
Semantic analysis and text mining are pivotal in refining qualitative research, especially in the context of interview analysis AI. These methodologies empower researchers to sift through subtle nuances in large text datasets, extracting meaningful insights and understanding underlying themes. By employing advanced algorithms, this process enables the transformation of raw conversational data into structured, actionable information.
Incorporating semantic analysis makes it possible to identify sentiments, key phrases, and relationships between concepts within interview transcripts. Text mining complements this by further organizing and categorizing content, allowing researchers to quickly pinpoint recurrent themes or sentiments across multiple interviews. This combination greatly enhances the efficiency and effectiveness of qualitative analysis, aiding researchers in deriving insights that are not immediately evident, thus ensuring a more comprehensive understanding of the subject matter.
- MonkeyLearn
MonkeyLearn serves as an advanced tool in the realm of interview analysis AI, particularly excelling in semantic analysis and text mining. This platform allows researchers to process and derive meaningful insights from vast amounts of qualitative data. By employing natural language processing, it effectively identifies patterns and trends in interview transcripts, significantly enhancing the overall analytical experience. Users can customize themes and categories based on their specific research needs, making it a versatile asset for qualitative studies.
Additionally, the platform's user-friendly interface simplifies the process of data management. Researchers can effortlessly upload and organize their interview data for analysis. This accessibility allows for quicker insights, which can be critical in qualitative research where timelines are often tight. Furthermore, it supports a range of integrations, ensuring compatibility with existing systems and workflows. In summary, utilizing this innovative technology can revolutionize the approach to interview analysis, making it indispensable for researchers aiming for thorough and insightful qualitative analysis.
- NVivo
In the realm of qualitative research, NVivo serves as a powerful tool for conducting interview analysis AI. This software supports researchers in processing, analyzing, and visualizing data efficiently. By transforming raw interview transcripts into structured information, NVivo aids in identifying key themes and patterns essential for qualitative insights.
Its project features allow users to upload audio files and transcriptions easily, fostering a seamless analytical experience. Researchers can categorize and code responses, facilitating a deeper understanding of participant feedback. Additionally, NVivo offers matrix querying, enabling teams to see trends and summarize responses across different projects. This capability is vital for constructing comprehensive narratives that reflect user experiences. By utilizing NVivo, researchers enhance their qualitative analysis, making the exploration of interview data more efficient and insightful.
Conclusion on the Impact of Interview Analysis AI
Interview Analysis AI has revolutionized the way qualitative research is conducted, introducing unparalleled efficiency and accuracy. By automating the analysis of interviews, researchers can quickly identify key themes and patterns in large datasets, which traditionally required extensive manual effort. This innovation not only saves time but also enhances the overall quality of insights, allowing researchers to focus on interpreting findings rather than getting bogged down in data organization.
Furthermore, Interview Analysis AI helps minimize biases that may arise during data interpretation. Its ability to process vast amounts of information objectively ensures that all voices from interviews are heard and represented accurately. As qualitative research continues to evolve, embracing Interview Analysis AI will likely become essential, paving the way for deeper insights and more informed decision-making in various fields.