Top 10 AI Agents for Emotional Sentiment Analysis
-
Bella Williams
- 10 min read
Emotional sentiment analysis has become a cornerstone in understanding consumer behavior, emotions, and preferences. As organizations strive to make data-driven decisions, having the right tools for emotional sentiment analysis is crucial. Leading sentiment analyzers are revolutionizing how businesses and researchers interpret emotional data, providing valuable insights into customer experiences.
Understanding the nuances of human emotions can be challenging, particularly across diverse cultures and languages. However, these advanced tools are designed to navigate such complexities. They help identify subtle emotional cues, enabling organizations to engage more effectively with their audiences and refine their strategies based on authentic emotional data. In the sections that follow, we will explore the top AI agents driving these innovations in emotional sentiment analysis.
Analyze & Evaluate Calls. At Scale.
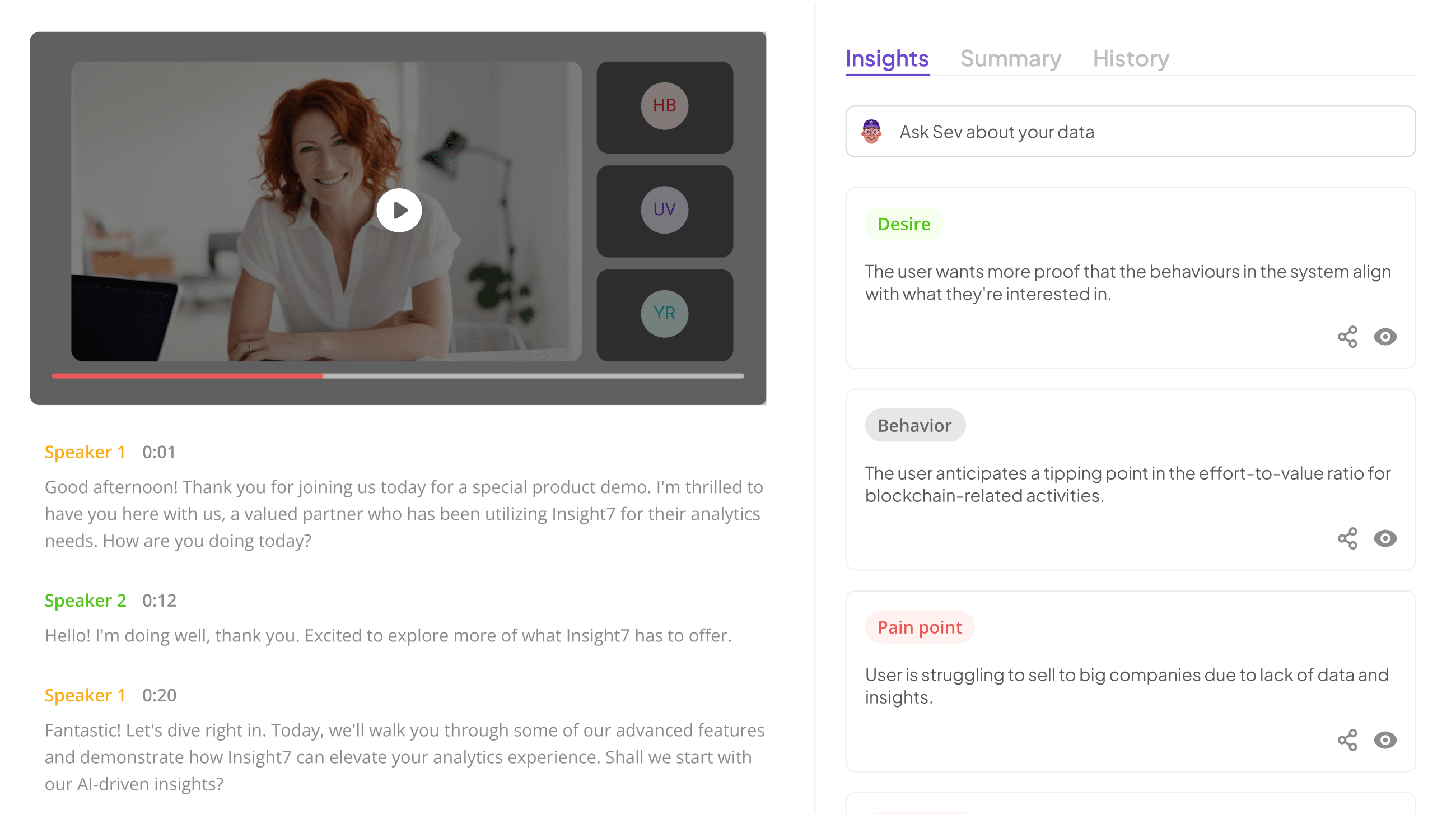
Overview of Emotional Sentiment Analysis
Emotional sentiment analysis plays a crucial role in understanding human emotions in various contexts. This analytical approach involves interpreting and categorizing emotions expressed in textual data, such as customer feedback or social media posts. By utilizing advanced algorithms, leading sentiment analyzers can identify sentiments ranging from joy and anger to sadness and confusion. These insights can significantly impact business strategies, enhancing customer satisfaction and engagement.
The importance of emotional sentiment analysis evolves as organizations face challenges like the nuances of human emotions and cultural differences. Accurate sentiment interpretation requires a sophisticated understanding of language, tone, and context. Additionally, multilingual analysis can complicate sentiment extraction. However, advancements in AI technology continue to improve accuracy, helping businesses navigate these challenges effectively. As we explore leading sentiment analyzers, their potential to transform communication and decision-making becomes clear, paving the way for a deeper understanding of emotional insight in various sectors.
Understanding Sentiment Analysis
Sentiment analysis is a vital tool for understanding human emotions in textual data. It involves the use of AI algorithms to classify and interpret the sentiments expressed in communications, such as social media posts, customer feedback, and product reviews. By employing leading sentiment analyzers, businesses can gain critical insights into customer emotions, enhancing their decision-making processes.
Applications for sentiment analysis span various domains, including marketing, finance, and customer service. In marketing, it helps gauge public reaction to campaigns, allowing companies to adjust strategies for better engagement. In finance, sentiment analysis can predict market trends, influencing investment decisions. Understanding sentiment thus plays a crucial role in responding to consumer needs, refining products, and fostering better relationships with audiences. As companies increasingly rely on emotional data, leveraging leading sentiment analyzers becomes essential in staying competitive.
Definition and significance
Emotional Sentiment Analysis refers to the process of identifying and categorizing emotional responses expressed in text. This involves the utilization of advanced algorithms to discern feelings such as joy, sadness, anger, and surprise. Understanding these sentiments is essential for businesses and researchers, as it provides deep insights into consumer behavior and emotional responses.
The significance of examining emotions through sentiment analysis lies in its ability to enhance customer engagement, drive marketing strategies, and influence decision-making. By leveraging leading sentiment analyzers, organizations can extract actionable insights from various data sources, including social media, customer reviews, and surveys. This not only aids in refining product offerings but also helps build a closer relationship with customers. Ultimately, the effective application of emotional sentiment analysis can lead to improved customer satisfaction and increased loyalty.
Application domains and impact
Emotional sentiment analysis has significant applications across various domains, impacting decision-making and user engagement strategies. Leading sentiment analyzers are invaluable in fields such as marketing, customer service, and social media monitoring. They help organizations understand consumer emotions, enabling more targeted campaigns and improving customer interaction experiences. By accurately identifying sentiments, businesses can enhance their products, services, and overall brand reputation.
In addition to marketing, sentiment analysis plays a crucial role in healthcare and mental health support. AI-driven tools assist in identifying emotional distress in patients, allowing for timely interventions. Furthermore, in political landscapes, sentiment analysis helps gauge public opinions and sentiments on key issues. The impact of effective sentiment analysis extends to improving compliance within various industries, aiding organizations in maintaining regulatory standards. Overall, the applications of these leading sentiment analyzers are vast and continue to grow in importance.
Key Challenges in the Field
Analyzing emotions accurately through technology presents substantial challenges. One key difficulty stems from the nuances of human emotion itself. Emotional expression varies widely across different cultures, and even among individuals within the same culture. These subtleties can confuse AI models, leading to incorrect sentiment categorization. This inconsistency makes it essential for leading sentiment analyzers to adapt their algorithms continually to accurately interpret emotional data.
Another considerable challenge is the complexity of multilingual analysis. Sentiment perception changes significantly across languages due to cultural interpretations and unique idiomatic expressions. Thus, AI tools must be trained on diverse linguistic datasets to ensure they perform effectively in various contexts. Overcoming these hurdles is crucial for advancing the efficacy of emotional sentiment analysis, ensuring sentiment analyzers not only understand language but also the cultural and emotional nuances that inform human communication.
Nuances of human emotion
Understanding the nuances of human emotion is critical in sentiment analysis. Emotions are complex and often overlapping, comprising various feelings that can be both subtle and pronounced. When analyzing sentiment, it’s essential to recognize these emotional layers as they influence how individuals express themselves. Leading sentiment analyzers must effectively parse these intricacies to provide meaningful insights.
One significant aspect is the context in which emotions are expressed. For example, sarcasm can easily distort the intended message if not recognized. Similarly, cultural differences can impact emotional expression, making it vital for sentiment analysis tools to be adaptable. A thorough grasp of these nuances leads to more accurate interpretations, which enhances the relevance and effectiveness of sentiment analysis. All in all, emotion is a multifaceted domain, and understanding its subtleties will profoundly impact the development of advanced sentiment analysis systems.
Multilingual analysis difficulties
Multilingual sentiment analysis presents unique challenges that can hinder accurate results. One major difficulty is the variation in emotional expressions across different languages. Words that convey similar meanings in one language may carry entirely different emotional weights in another. This disparity complicates how leading sentiment analyzers interpret feelings and sentiment across diverse cultures.
Additionally, idiomatic expressions can pose significant barriers. Phrases that are widely understood in one language may be lost in translation, leading to misinterpretations of sentiment. This issue is exacerbated by the nuances and subtleties inherent in languages, which can alter the intended meaning of a statement. Furthermore, the lack of training data in lesser-used languages can limit the effectiveness of these tools, resulting in skewed analysis or incomplete insights. As a result, professionals utilizing sentiment analysis must carefully consider the limitations presented by multilingual environments.
Leading Sentiment Analyzers: Top Tools for Emotional Analysis
Leading sentiment analyzers play a crucial role in understanding and interpreting human emotions in various contexts. These tools are essential for businesses that want to gauge customer feedback, analyze social media sentiment, or conduct market research. With advanced algorithms and machine learning techniques, these analyzers can sift through vast amounts of data to identify emotions, trends, and patterns that are otherwise hidden.
Several notable tools stand out in the realm of emotional analysis. IBM Watson Tone Analyzer provides a comprehensive set of features while Google Cloud Natural Language excels in delivering AI-driven insights. Amazon Comprehend utilizes machine learning to enhance sentiment analysis, making it practical across various sectors. Each of these tools has unique strengths that can cater to different analysis needs, empowering companies to make data-informed decisions. By leveraging these leading sentiment analyzers, organizations can better connect with their audience and adapt their strategies accordingly.
Insight7: A Pioneer in Sentiment Analysis
Sentiment analysis serves as a powerful tool for understanding consumer emotions and attitudes through data. This technology is transforming how organizations engage with their customers, enabling them to gain deeper insights into feedback and interactions. As leaders in the realm of sentiment analysis, some platforms are innovatively addressing the challenges of traditional methods, making it easier to interpret large volumes of data swiftly and accurately.
A standout feature of these leading sentiment analyzers is their ability to scale effortlessly. By offering self-service solutions, they empower users to analyze customer conversations in real-time, improving responsiveness and efficiency in decision-making. Moreover, they possess advanced capabilities such as multilingual support and emotion detection, allowing organizations to tap into rich customer insights across diverse markets. This evolution in sentiment analysis not only streamlines data interpretation but also fosters a proactive approach to customer engagement, ultimately providing a competitive edge in today’s fast-paced business environment.
Overview and unique features
Emotional sentiment analysis is a rapidly evolving field, marked by the emergence of leading sentiment analyzers. These powerful tools provide invaluable insights into human emotions, enabling businesses to better understand customer sentiments and improve their engagement strategies. By employing sophisticated algorithms, these AI agents can analyze large volumes of data efficiently, delivering actionable insights that inform decision-making.
The unique features of these sentiment analyzers include real-time monitoring, multilingual capabilities, and customizable analysis parameters. For instance, some tools focus on identifying pain points, desires, and customer behaviors, transforming raw data into clear insights. Others may offer visualization tools that simplify the interpretation of emotional data, making it accessible to all users, regardless of their technical expertise. Overall, the integration of these capabilities allows organizations to harness the full potential of emotional sentiment analysis in shaping their customer interactions and enhancing overall satisfaction.
Use cases and success stories
The applications of leading sentiment analyzers in emotional sentiment analysis are diverse and impactful. Organizations are utilizing these tools to enhance customer engagement and improve service quality. For instance, a company might analyze customer support calls to identify sentiment trends, which helps to better train their staff in addressing client needs. This real-time feedback loop fosters a deeper understanding of customer emotions, aiding in personalizing services effectively.
💬 Questions about Top 10 AI Agents for Emotional Sentiment Analysis?
Our team typically responds within minutes
Moreover, success stories abound across various industries. Retailers analyze online reviews and social media feedback to gauge consumer sentiment, informing product development and marketing strategies. In healthcare, sentiment analysis tools help monitor patient feedback on services, ensuring continual improvement in care quality. These use cases illustrate how sentiment analysis not only enhances operational efficiency but also creates a more empathetic connection between organizations and their audiences.
Extract insights from interviews, calls, surveys and reviews for insights in minutes
Detailed List of Top AI Sentiment Analysis Tools
The section titled “Detailed List of Top AI Sentiment Analysis Tools” presents a curated selection of advanced technologies that excel in emotional sentiment analysis. Each of these leading sentiment analyzers plays a critical role in interpreting human emotions from textual data, providing actionable insights for various industries. By employing sophisticated algorithms and machine learning techniques, these tools are designed to decode the nuances of language, offering a nuanced understanding of customer sentiments.
Among the most notable tools in this realm are IBM Watson Tone Analyzer, Google Cloud Natural Language, and Amazon Comprehend. The IBM Watson Tone Analyzer stands out with its comprehensive capabilities, allowing users to assess the emotional tone behind a series of texts and integrate with existing workflows for customized applications. On the other hand, Google Cloud Natural Language offers AI-driven sentiment insights that highlight specific tendencies in user feedback. Lastly, Amazon Comprehend utilizes machine learning to deliver personalized sentiment analysis, enhancing user experience through real-world application examples. These tools collectively represent the forefront of sentiment analysis technology, empowering businesses to make data-driven decisions.
IBM Watson Tone Analyzer
The IBM Watson Tone Analyzer offers a comprehensive suite of features designed to interpret emotional tones in text. This tool helps users understand the emotional undercurrents in communications, making it a vital resource for industries focused on customer sentiment. By assessing various emotional and language tones, it allows businesses to gain deeper insights into customer interactions and feedback.
One of the key strengths of this analyzer is its ability to seamlessly integrate with existing workflows and tools, enhancing its overall usability. Users can customize their analysis parameters, selecting specific emotions to focus on, such as joy, anger, or sadness. This capability ensures that organizations can tailor the insights to their unique needs, allowing for informed decision-making based on real emotional data. These features position this tool among the leading sentiment analyzers, setting a benchmark for understanding emotional sentiment in diverse applications.
Comprehensive features and capabilities
In exploring the comprehensive features and capabilities of leading sentiment analyzers, it becomes evident how these tools transform raw data into meaningful insights. At their core, these analyzers employ advanced algorithms, allowing for nuanced understandings of human emotions and sentiments, essential for businesses aiming to connect more deeply with their customers. Each tool offers unique functionalities, from real-time analysis to customizable reporting metrics that help organizations develop targeted strategies.
The effectiveness of these tools often hinges on the robustness of their integrations. They can seamlessly connect with various data sources, ensuring that the analysis reflects accurate sentiments from different platforms. Furthermore, many leading sentiment analyzers provide user-friendly interfaces that allow easy access to insights. This empowers stakeholders to make informed decisions swiftly based on the emotional feedback they receive from customers. Overall, the capabilities of these analyzers play a vital role in shaping better customer experiences and enhancing strategic planning.
Integration and customization options
Integration and customization options play a crucial role in enhancing the effectiveness of leading sentiment analyzers. These tools often provide APIs that allow businesses to seamlessly incorporate sentiment analysis into their existing systems. By enabling integration, organizations can analyze emotional responses in real-time, enhancing customer engagement and improving decision-making processes.
Customization is equally important as it allows users to tailor the sentiment analysis models according to specific industry needs. This involves configuring parameters like tone sensitivity and keyword focus for more accurate results. Many leading sentiment analyzers also offer flexible dashboards, making it easier for users to visualize data trends and iterate on their analytics strategies. This ensures companies not only adapt the tools to fit their unique requirements but also leverage emotional insights that drive impactful outcomes.
Google Cloud Natural Language
Google Cloud Natural Language is a powerful tool that provides AI-driven sentiment insights. It excels in analyzing text to determine the emotional tone and sentiment behind the words. Users can integrate this tool into various applications, from marketing analytics to customer feedback assessments. The platform processes a myriad of languages, allowing for timely analysis regardless of the source’s language.
Working with this tool, organizations can efficiently decipher users’ emotions and tailor their responses accordingly. However, while it offers robust features, there are considerations to keep in mind. For instance, it may sometimes struggle with nuanced expressions of sentiment, particularly in complex or ambiguous contexts. Understanding its strengths and limitations is essential for users looking to optimize their emotional analysis strategies. This tool stands out among leading sentiment analyzers while serving as a cornerstone for those focused on enhancing emotional engagement.
AI-driven sentiment insights
AI-driven sentiment insights have transformed the way businesses perceive customer emotions and feedback. These insights allow organizations to gauge emotional responses from vast amounts of data by harnessing advanced algorithms and machine learning techniques. The leading sentiment analyzers employ natural language processing to interpret the nuances of human emotion, revealing underlying sentiments that might not be immediately evident.
These tools can process customer conversations, social media interactions, and survey responses at an unprecedented scale. Moreover, the ability to extract actionable insights in real-time enables companies to adapt their strategies swiftly. By understanding the emotional drivers of customer behavior, businesses can innovate their products and enhance customer experiences. Ultimately, leveraging AI-driven sentiment insights equips organizations with the knowledge necessary to stay competitive in an ever-evolving marketplace.
Pros and cons in real-world applications
The use of leading sentiment analyzers in real-world applications comes with its share of pros and cons. On the positive side, these tools can significantly enhance customer understanding. They process large volumes of text quickly, extracting valuable sentiment insights that inform business decisions. This efficiency reduces the time and resources spent on manual analysis, enabling organizations to focus on more strategic tasks.
However, challenges exist. Leading sentiment analyzers may struggle with the nuances of human emotion, especially in complex contexts where sarcasm or dialects are involved. Additionally, while many tools perform well in English, multilingual capabilities can lead to inaccuracies in sentiment interpretation across different languages and cultures. Organizations must weigh the benefits of efficiency against the potential for misinterpretation to ensure they use these tools effectively. Balancing these factors is crucial for maximizing the success of sentiment analysis initiatives.
Amazon Comprehend
Amazon Comprehend excels as a machine learning service designed for sentiment analysis, making it one of the leading sentiment analyzers available today. It specializes in understanding emotions conveyed within text, offering profound insights into customer opinions and feedback. By utilizing natural language processing, it effectively categorizes sentiments into positive, negative, neutral, or mixed, enhancing the ability to gauge customer feelings.
Users can apply this tool across various sectors, from marketing campaigns to customer service interactions. Real-world applications showcase its effectiveness in analyzing social media sentiments, product reviews, and even internal communications. Feedback indicates that businesses leveraging this technology gain valuable insights, keeping them attuned to customer needs and preferences. Ultimately, Amazon Comprehend stands out as a powerful resource for organizations looking to harness emotional sentiment analysis in their operations.
Use of machine learning for sentiment analysis
Machine learning has revolutionized the way we approach sentiment analysis, transforming vast datasets into meaningful insights. By employing sophisticated algorithms, analysts can accurately detect emotional nuances present in text, be it social media conversations or customer feedback. This allows businesses to gauge public sentiment and adjust strategies accordingly, fostering better customer experiences.
Leading sentiment analyzers harness machine learning techniques to identify patterns, categorize emotions, and track sentiment over time. Some tools leverage natural language processing (NLP) to enhance their effectiveness, ensuring they understand context and subtleties of human expression. The integration of machine learning facilitates improved accuracy and efficiency, making sentiment analysis a powerful tool for decision-makers. As organizations increasingly rely on data-driven insights, understanding and utilizing machine learning in sentiment analysis becomes essential for success in the digital landscape.
Examples of application and user feedback
User feedback and practical applications of leading sentiment analyzers offer a glimpse into their effectiveness in diverse settings. For example, businesses utilize these tools to refine customer service interactions. Insights gleaned from sentiment analysis help companies understand the emotional tone of customer calls, leading to improved training programs for representatives. When users report increased satisfaction from tailored services, it indicates that sentiment analyzers are delivering valuable insights.
Furthermore, many organizations leverage sentiment analyzers to enhance their marketing strategies. By analyzing customer feedback and engagement, companies can adjust their messaging and content effectively. This results in targeted approaches that resonate better with their audiences. Users have expressed that these tools provide actionable data, and the continuous improvement processes lead to significant improvements. With ongoing advancements, the potential of leading sentiment analyzers continues to expand, ensuring they meet evolving market demands.
Analyze & Evaluate Calls. At Scale.
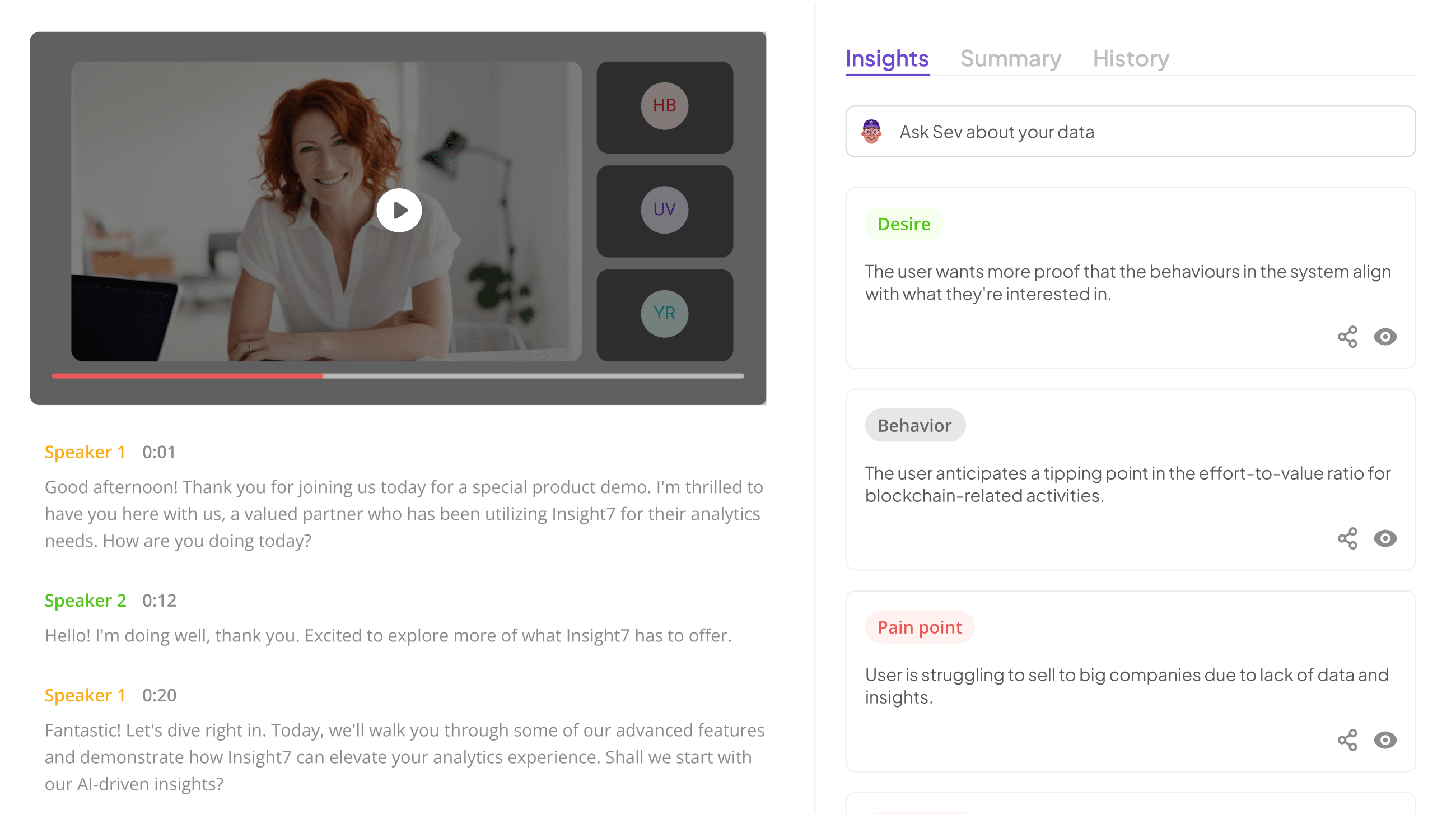
Conclusion: The Future of Leading Sentiment Analyzers in Emotional Sentiment Analysis
As we look ahead, the future of leading sentiment analyzers in emotional sentiment analysis is poised for remarkable advancements. With rapid developments in artificial intelligence and machine learning, these tools are becoming increasingly adept at discerning complex human emotions. This evolution enables businesses to harness deeper insights from customer interactions, paving the way for greater personalization in marketing and customer service.
The ongoing integration of more sophisticated algorithms will enhance the accuracy and reliability of sentiment analysis. As leading sentiment analyzers continue to refine their capabilities, we can expect improved emotion detection across languages and cultural contexts. Such progress holds the promise of transforming how organizations engage with their audiences, ensuring that empathy and understanding are at the forefront of their communication strategies.
Analyze & Evaluate Calls. At Scale.
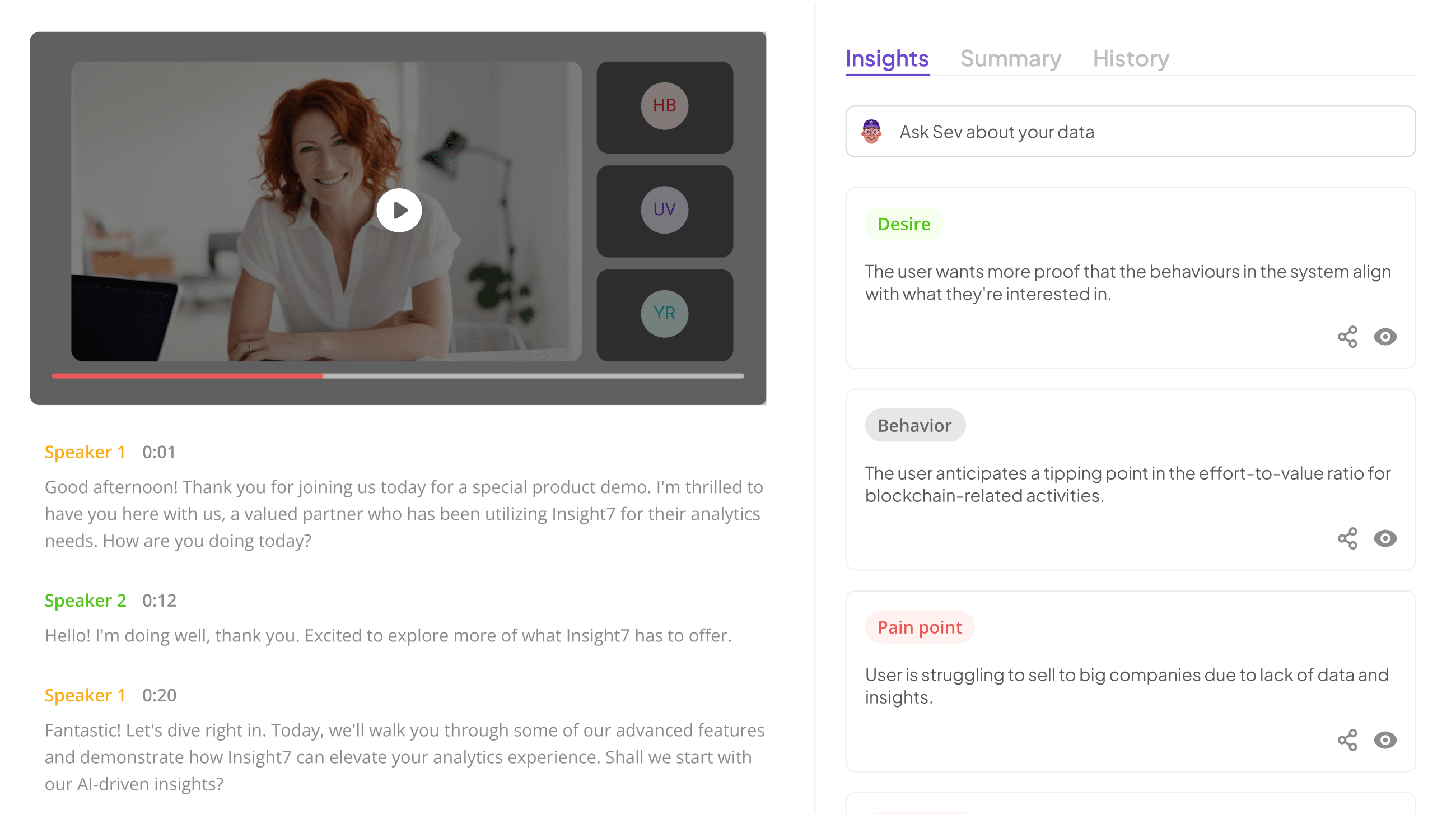
💬 Questions about Top 10 AI Agents for Emotional Sentiment Analysis?
Our team typically responds within minutes