AI Evaluation Structuring serves as a transformative approach to managing open-ended evaluations, optimizing the analysis of qualitative data. As businesses seek to unearth deeper insights from their feedback and interviews, the challenge of consistency and speed often emerges. Many organizations struggle with the time-consuming nature of manual evaluation. This can lead to biases and hinder the efficacy of insights generated by diverse team members.
By adopting AI-driven tools for evaluation structuring, teams can enhance their analytical processes significantly. AI systems streamline data processing, improving accuracy and efficiency while allowing researchers to spot trends and themes more effectively. Embracing AI Evaluation Structuring not only expedites report generation but also supports a more coherent understanding of qualitative responses, thus empowering teams to make informed decisions quickly.
Analyze qualitative data. At Scale.
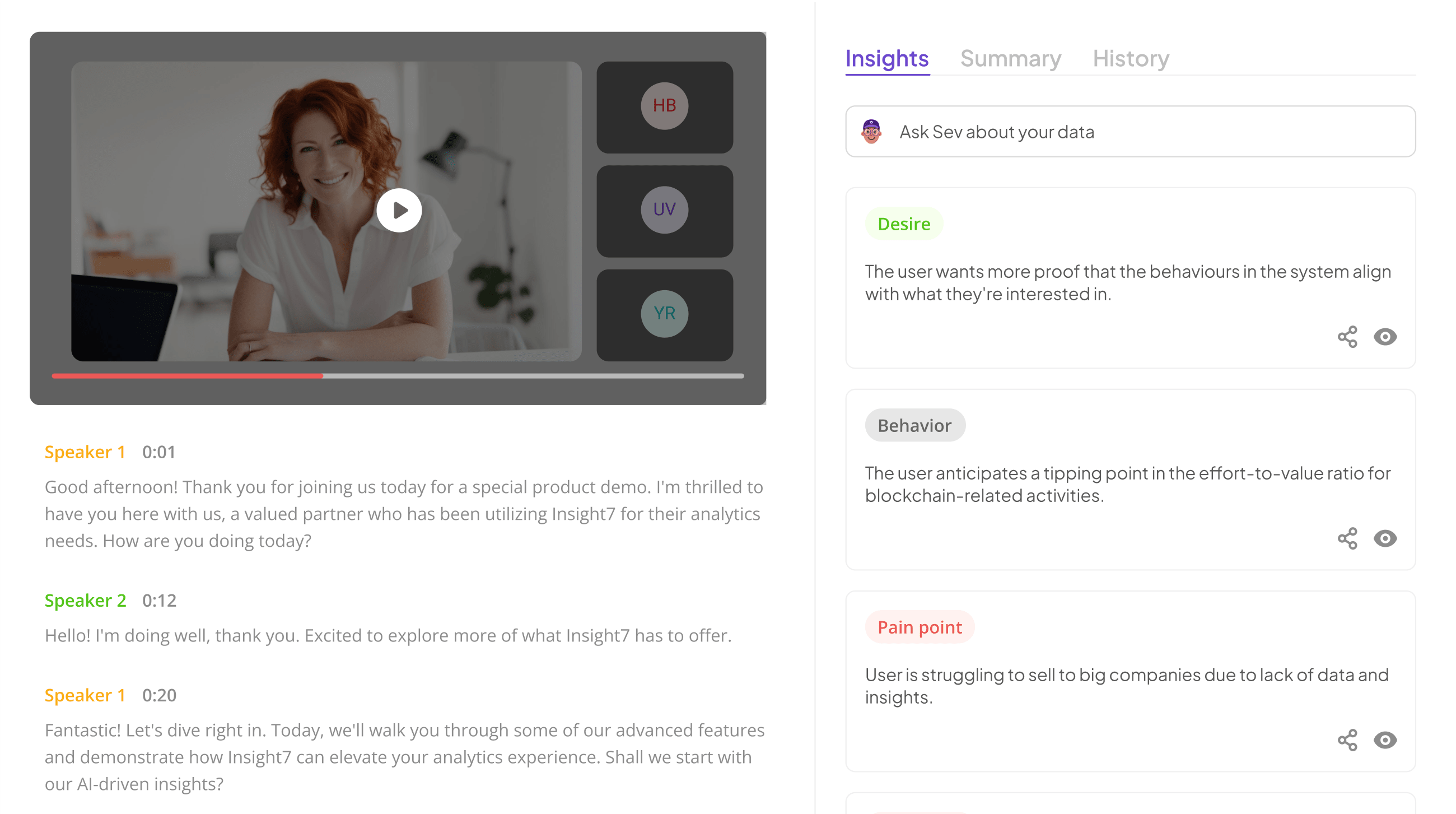
Unlocking the Potential of AI Evaluation Structuring in Open-Ended Feedback
AI Evaluation Structuring introduces a transformative approach to managing open-ended feedback. By harnessing artificial intelligence, organizations can systematically analyze qualitative data that is often rich but unstructured. The challenge lies in interpreting the complexity and variability of these responses. Without AI tools, this process demands significant resources, leading to inconsistencies and potential biases during analysis.
Unlocking the potential of AI involves several key steps. First, these AI systems enhance efficiency by automating the evaluation process, allowing teams to focus on high-impact tasks. Second, AI-powered analytics identify trends and themes, ensuring that insights are not overlooked. These tools turn qualitative feedback into quantifiable data, facilitating informed decision-making. Ultimately, embracing AI Evaluation Structuring not only streamlines the open-ended evaluation process but also enriches the overall feedback quality, making it more actionable and strategic for businesses.
Challenges in Structuring Open-Ended Evaluations
Open-ended evaluations can be especially challenging to structure due to the inherent complexity of qualitative responses. Each response can vary significantly, which complicates consistent analysis. This variability can lead to misinterpretations and inconsistencies if evaluators are not aligned on analysis methods. Furthermore, capturing nuanced insights that emerge from open-ended feedback requires a systematic approach, one that often ends up being incredibly manual and time-consuming.
Another challenge is the necessity for timely reporting. Clients increasingly demand faster insights, which adds pressure to evaluators who are already wrestling with the intricacies of qualitative data. In many cases, traditional methods may fall short, necessitating the integration of AI Evaluation Structuring tools to enhance efficiency. These tools can streamline the analysis process, ensuring that evaluations are not only faster but also more reliable. By effectively utilizing AI, teams can turn the challenges of structuring open-ended evaluations into opportunities for deeper, more actionable insights.
- Understanding the complexity and variability in qualitative responses
Understanding the complexity and variability in qualitative responses is essential for optimizing AI evaluation structuring. Qualitative responses often exhibit diverse perspectives, influenced by individual experiences and contexts. This variability can complicate data analysis, making it vital to employ structured approaches that enhance clarity and reliability. AI evaluation structuring can simplify these nuances by systematically organizing responses, revealing underlying themes that may otherwise be overlooked.
To effectively grasp the impact of qualitative variability, consider these key points:
- Diverse Perspectives: Individual viewpoints shape responses, creating a wide range of interpretations that must be harmonized.
- Contextual Influences: External factors such as environment and timing can affect how respondents articulate their ideas.
- Response Bias: Personal biases can skew the data, highlighting the need for objective analysis methods.
- Analytical Consistency: Consistent methods are crucial for ensuring that insights drawn from qualitative data are reliable and actionable.
- Improved Insights: AI can unearth hidden trends and connections, transforming complex data into valuable insights.
By embracing these points, AI evaluation structuring can facilitate a more coherent understanding of qualitative data, ultimately leading to more informed decision-making.
- The importance of consistent analysis for insightful data
Consistent analysis plays a pivotal role in extracting meaningful insights from qualitative data. When working with open-ended evaluations, relying on varied methodologies can lead to fragmented results, undermining the reliability of the conclusions drawn. In this context, AI Evaluation Structuring becomes essential. It enables evaluators to apply systematic approaches repeatedly, ensuring that insights are both relevant and actionable. By maintaining a cohesive framework for analysis, patterns in responses can be discerned, enhancing the understanding of user sentiments.
Furthermore, consistent analysis allows for comparisons across different datasets, offering a clearer view of trends over time. This is critical for organizations seeking to adapt strategies based on evolving consumer behavior and preferences. With the right AI-powered tools, analysis becomes not just efficient but also deeply insightful. The interplay of consistent analysis and advanced technology helps organizations to navigate complexities in qualitative feedback, promoting informed decision-making and strategic planning.
Benefits of AI Evaluation Structuring
AI Evaluation Structuring facilitates a transformative approach to handling open-ended evaluations. The primary benefit of this structuring is enhanced efficiency, which enables quicker processing of qualitative data. With accurate AI-driven analysis, the time-consuming task of manually sifting through responses is significantly reduced. Users can focus on delivering insights rather than merely gathering data.
In addition, AI Evaluation Structuring excels at identifying trends and themes, which may be overlooked in traditional analyses. This ability to uncover patterns within qualitative responses leads to more informed decision-making and richer insights. By structuring evaluations with AI tools, organizations can not only improve the quality of their analyses but also adapt more swiftly to evolving consumer preferences. Ultimately, AI Evaluation Structuring empowers businesses to derive actionable insights faster and more reliably, enhancing overall strategic planning and execution.
- Enhanced efficiency and accuracy in data processing
The integration of AI in structuring evaluations significantly boosts the efficiency and accuracy of data processing. By employing advanced technologies, organizations can streamline their approach to gathering insights from open-ended feedback. This automation reduces the time spent on manual analysis, allowing teams to focus on interpreting data meaningfully. AI-driven tools can aggregate and analyze large volumes of qualitative data, making the identification of trends and themes seamless.
Moreover, these tools enhance accuracy by minimizing human error in interpretation. Users benefit from detailed reports generated quickly, presenting well-structured data that aids in strategic decision-making. This method not only ensures that no insights are overlooked but also supports organizations in staying compliant with data privacy regulations, ensuring safe handling of sensitive information. In essence, leveraging AI for evaluation structuring provides an invaluable edge in today's data-driven environment, leading to informed actions and targeted business strategies.
- Ability to identify trends and themes within qualitative data
The ability to identify trends and themes within qualitative data plays a crucial role in effective evaluation. By employing AI evaluation structuring, organizations can systematically process open-ended feedback to uncover underlying patterns. This process involves leveraging advanced algorithms to sift through large volumes of qualitative responses, extracting meaningful themes that might otherwise go unnoticed.
To enhance this analysis, several key aspects come into play. First, coding the data allows users to categorize responses efficiently, helping to clarify themes like challenges and desires. Second, defining specific goals ensures that insights are relevant and targeted, aligning with business objectives. Lastly, sentiment analysis enriches the understanding of participant feedback by providing context around emotional tones associated with various themes. By focusing on these elements, AI evaluation structuring becomes an invaluable tool for deriving actionable insights from qualitative data.
Extract insights from interviews, calls, surveys and reviews for insights in minutes
Top Tools for AI Evaluation Structuring
In the realm of open-ended evaluations, selecting the right tools for AI evaluation structuring is crucial. These tools streamline complex qualitative data, facilitating the extraction of meaningful insights. Moreover, they enhance the accuracy of analysis, ensuring that organizations can effectively capture diverse perspectives. Choosing the right technology establishes a foundation for robust data interpretation, ultimately leading to better decision-making.
A variety of advanced tools are available today that specialize in AI evaluation structuring. For instance, MonkeyLearn offers versatile text analysis that adapts through machine learning, enabling users to uncover underlying patterns efficiently. Qualtrics stands out with its comprehensive surveying features, providing AI-driven insights that enhance respondent engagement. Then there's IBM Watson, known for its sophisticated natural language processing capabilities, which help in understanding nuanced responses. SurveyMonkey Genius employs AI to improve survey analysis, while Clarabridge focuses on integrating AI for customer feedback interpretation. These tools collectively empower organizations to harness the power of qualitative data with greater efficiency and effectiveness.
insight7: Setting the Benchmark
In any evaluation process, establishing a reliable benchmark is essential to ensure the quality and relevance of insights gathered. When we discuss insight7: Setting the Benchmark, it becomes crucial to understand how AI Evaluation Structuring can facilitate this objective. By employing advanced algorithms, AI systems can systematically analyze large volumes of qualitative data, thereby creating a robust framework for evaluation. This benchmark not only enhances the consistency of analyses but also ensures that decision-makers have access to quality insights that accurately reflect the sentiments and needs of respondents.
As organizations increasingly rely on open-ended evaluations, the tools that support AI Evaluation Structuring must adapt to meet their evolving needs. One key feature is the ability to identify trends and themes from diverse qualitative responses. Additionally, they can streamline the examination of interview transcripts, reducing the time analysts spend on initial readings. By setting these benchmarks, organizations can refine their approach to data analysis, leading to improved decision-making and fostering a culture of continuous improvement.
- Overview of features specific to open-ended evaluations
In exploring features unique to open-ended evaluations, AI Evaluation Structuring plays a pivotal role. These evaluations often yield diverse qualitative data, making it essential to have robust tools that can efficiently analyze responses. One significant feature is the analysis grid, allowing users to formulate multiple questions across various data sets simultaneously. This functionality not only saves time but also enhances the depth of analysis, summarizing key insights from numerous sources effectively.
Another crucial aspect is the capability to process large volumes of qualitative data, such as open-ended survey responses. Tools equipped with coding functionalities can tag and categorize information, streamlining the process of identifying trends and themes within the responses. This systematic approach ensures that evaluators can extract actionable insights from complex data sets, ultimately leading to more informed decisions and strategies in their projects.
- Real-world applications and success stories
Real-world applications of AI Evaluation Structuring have shown impressive results across various sectors. Companies have utilized AI-powered tools to streamline their qualitative data analysis, transforming raw feedback into actionable insights. For example, in a B2B context, organizations have successfully implemented AI to analyze win-loss data, revealing critical patterns that inform strategic adjustments. This approach has enabled teams to focus on key customer sentiments and enhance retention rates effectively.
Success stories highlight the efficiency gains and accuracy improvements achieved by utilizing AI in open-ended evaluations. Clients have reported substantial time savings, allowing them to make faster, data-driven decisions. In these scenarios, the ability to synthesize diverse qualitative inputs into structured outputs fosters informed strategies. Such examples illustrate the profound impact of AI Evaluation Structuring, empowering organizations to navigate complex data landscapes and improve customer engagement continuously.
Other Effective Tools
In addition to established platforms, several other effective tools can enhance your approach to AI evaluation structuring. Each tool serves specific functionalities that can significantly streamline your open-ended evaluation processes. For instance, MonkeyLearn offers versatile text analysis while using machine learning, allowing users to classify and extract insights from qualitative responses seamlessly.
Equally, Qualtrics provides a comprehensive survey platform enriched with AI-driven insights, enabling deep analysis of feedback. On the other hand, IBM Watson leverages advanced natural language processing to understand sentiment and themes in large datasets. Meanwhile, SurveyMonkey Genius automates the analysis of surveys, facilitating quicker decision-making. Lastly, Clarabridge effectively integrates AI for customer feedback analysis, enabling companies to harness valuable insights from their user data. Embracing these tools contributes to a more structured and effective approach to AI evaluation structuring in open-ended assessments.
- MonkeyLearn: Versatile text analysis with machine learning
Open-ended evaluations often contain a wealth of qualitative data that traditional methods struggle to analyze effectively. This is where innovative tools come into play. Utilizing versatile text analysis capabilities, machine learning streamlines AI evaluation structuring, transforming vast amounts of unstructured text into actionable insights. By capturing themes and trends from user responses, these tools enhance the consistency and depth of analysis, leading to more reliable evaluations.
One key advantage is the toolโs seamless integration of transcription and analysis processes. Users can upload various content types and receive transcribed text ready for deep analysis. This makes it easier to extract valuable insights without the need for multiple platforms. Furthermore, as the technology evolves, it promises to incorporate more nuanced feedback mechanisms, enhancing the overall quality of qualitative data interpretation, which ultimately benefits decision-making across various fields.
- Qualtrics: Comprehensive survey tool with AI-driven insights
Designed to transform the survey process, this comprehensive survey tool empowers users to craft open-ended evaluations effortlessly. With a blend of intuitive design and powerful analytics, it addresses the complexities faced by researchers and teams. As organizations increasingly rely on qualitative data, having a systematic approach to AI evaluation structuring becomes essential for turning raw feedback into actionable insights.
The tool integrates advanced AI-driven insights, allowing for swift analysis of open-ended responses. This means faster identification of trends, themes, and sentimentsโcritical for crafting targeted strategies. By utilizing this tool, teams can alleviate the inconsistencies that often arise from manual evaluations, thus streamlining the reporting process. Ultimately, this comprehensive tool helps teams enhance efficiency and build a more cohesive understanding of customer perspectives. Embracing tools for AI evaluation structuring is crucial for any organization looking to leverage real-time feedback effectively.
- IBM Watson: Advanced natural language processing capabilities
Advanced natural language processing capabilities play a transformative role in AI evaluation structuring, especially for open-ended evaluations. These capabilities enable the analysis of complex qualitative data by discerning meaning and context within responses. By employing sophisticated algorithms, AI can categorize sentiments and identify key themes, significantly enhancing the reliability of insights derived from customer feedback.
One major advantage is the efficiency with which vast amounts of text data can be processed. This automation reduces the manual effort typically associated with qualitative analysis, allowing teams to focus on strategic decision-making. Furthermore, these advanced capabilities help ensure consistency in evaluations, making the results more actionable. As organizations continue to embrace these technologies, they can tap into valuable insights that guide product development and customer engagement, ultimately leading to improved outcomes in their evaluation processes.
This integration of advanced natural language processing shapes a new frontier in AI evaluation structuring, paving the way for more insightful and data-driven decisions.
- SurveyMonkey Genius: AI-powered survey analysis
AI Evaluation Structuring plays a crucial role in enhancing how open-ended survey responses are analyzed. The power of AI tools, particularly in survey analysis, transforms overwhelming data into structured insights. With cutting-edge technology, users can quickly identify trends, themes, and sentiment from qualitative responses, something traditionally accomplished through painstaking manual processes. This acceleration not only saves valuable time but also helps researchers maintain consistency in their findings.
In recent developments, a notable tool in AI-powered survey analysis has emerged, significantly streamlining this process. It utilizes advanced algorithms to dissect qualitative data and automatically categorizes insights. Users can upload open-ended responses and receive immediate feedback on key themes, ensuring more accurate and nuanced interpretations. This approach addresses long-standing challenges in managing qualitative responses, making the evaluation process both efficient and insightful. By embracing such innovative solutions, organizations can rapidly adapt to the increasing demand for timely and reliable insights.
- Clarabridge: Customer feedback analysis with AI integration
Customer feedback analysis with AI integration helps businesses transform qualitative insights into actionable strategies. This powerful approach utilizes advanced algorithms to organize and assess open-ended evaluations, making it easier to identify key themes and trends. As organizations increasingly rely on customer feedback to refine their products and services, efficiently structuring this data becomes paramount.
Through AI Evaluation Structuring, companies can streamline their analysis processes by automatically categorizing responses, which enhances both accuracy and speed. By leveraging such tools, businesses can unite scattered insights into cohesive reports that drive informed decision-making. Additionally, these AI solutions allow for deeper exploration of customer sentiments, enabling organizations to activate insights swiftly. This integration not only saves time but also empowers teams to focus on strategic actions that improve customer experiences and overall satisfaction.
Conclusion: The Future of AI Evaluation Structuring in Open-Ended Assessments
The future of AI Evaluation Structuring in open-ended assessments looks promising as technological advancements continue to evolve. Tools designed to enhance qualitative analysis are rapidly becoming more sophisticated, offering greater efficiency and reliability. This transformation enables researchers and evaluators to process vast amounts of data systematically, uncovering insights that were previously difficult to obtain. Such capabilities are essential for maintaining accuracy and reducing biases in findings, thereby increasing the overall value of qualitative research.
Moreover, as user needs become more complex, the tools will likely integrate features that provide real-time feedback and progress tracking. The importance of understanding the context around data remains paramount. By effectively structuring evaluations, organizations can derive actionable insights that drive meaningful change. Embracing these innovations in AI evaluation structuring will not only streamline the assessment process but also enhance decision-making across various fields.
Analyze qualitative data. At Scale.
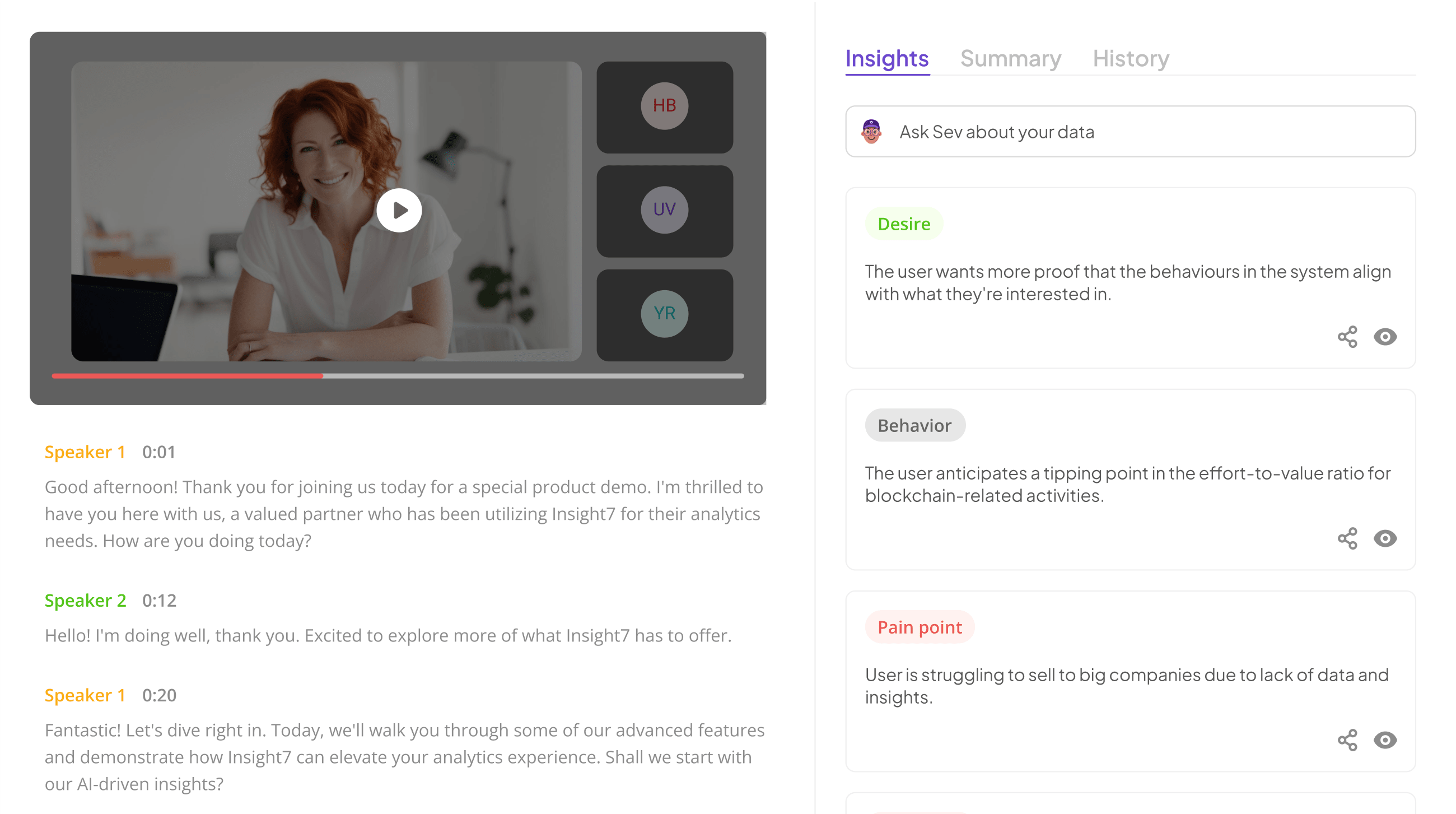