Predictive Consumer Analytics is transforming how businesses engage with their customers. In an era dominated by technology, understanding consumer behavior has become central to effective marketing strategies. By harnessing machine learning techniques, brands can analyze vast amounts of data to uncover patterns and anticipate future behaviors. This proactive approach allows marketers to tailor their campaigns, ensuring they resonate with the intended audience.
As we dive deeper into the significance of Predictive Consumer Analytics, it's essential to recognize its role in shaping personalized experiences. By analyzing historical data, businesses can develop insights that not only boost sales but also enhance customer satisfaction. Ultimately, the integration of these analytics into marketing practices is a game-changer, leading to more informed decisions and improved customer relationships.
Analyze qualitative data. At Scale.
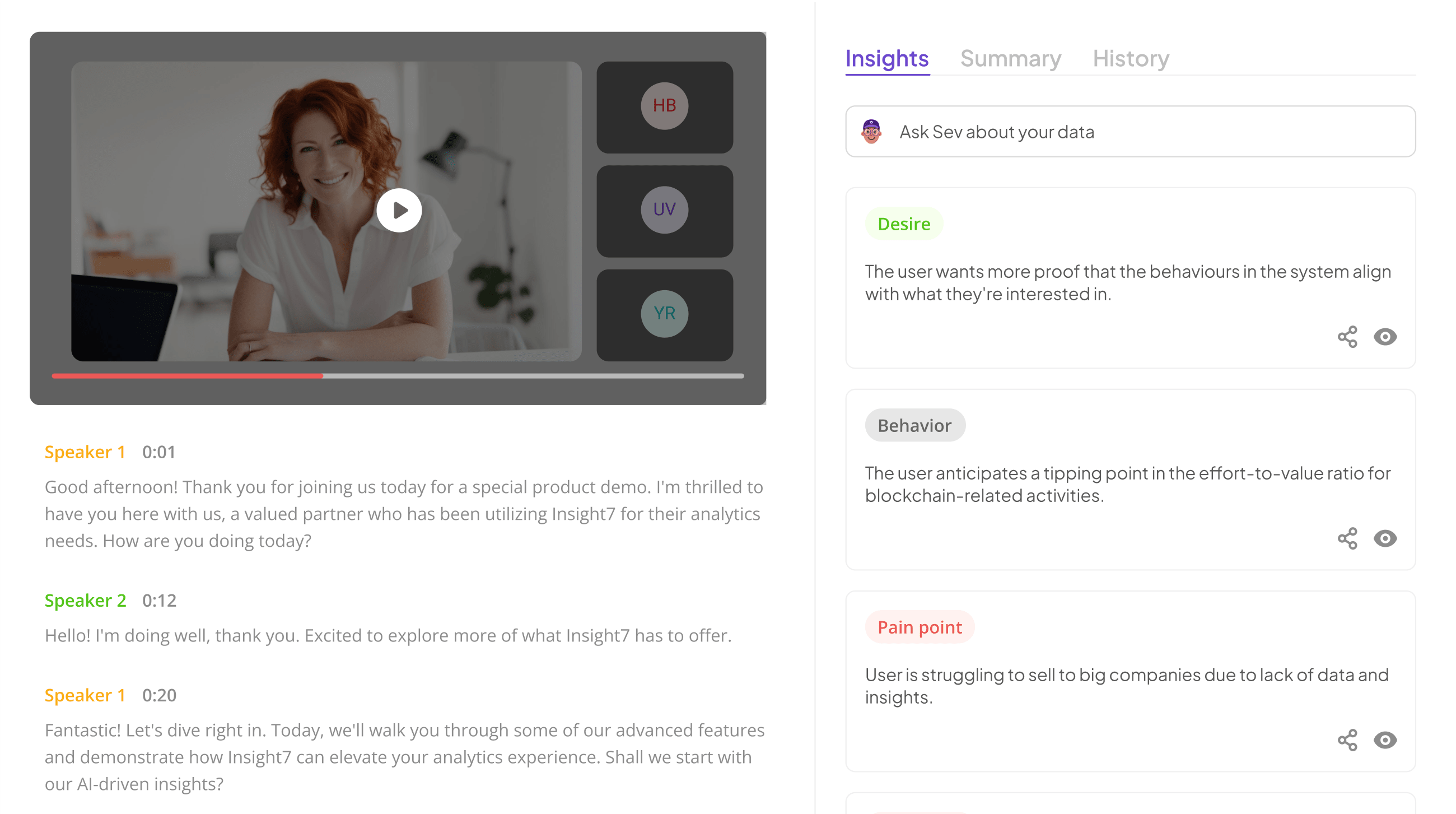
Understanding Predictive Consumer Analytics in Machine Learning
Predictive Consumer Analytics plays a vital role in understanding consumer behavior in todayโs market. It enables businesses to analyze historical data, identify patterns, and forecast future buying habits. By tapping into large datasets, organizations can extract meaningful insights that inform marketing strategies and product development. This process often utilizes algorithms and machine learning techniques to enhance accuracy and efficiency in predictions.
In predictive analytics, the first step is data collection, which includes customer interactions, sales transactions, and social media activity. The next phase involves data processing, where the information is cleaned and organized. Subsequently, businesses apply statistical models and machine learning algorithms to interpret the data. Finally, organizations can employ these insights to tailor their offerings, enhance customer experience, and ultimately increase sales. Such a systematic approach ensures that businesses stay responsive to changing consumer preferences and market dynamics.
How Predictive Consumer Analytics Leverages Data
Predictive Consumer Analytics plays a vital role in improving marketing strategies by utilizing vast amounts of consumer data. By analyzing patterns and trends in consumer behavior, businesses can anticipate preferences and make data-driven decisions. This process begins with the collection of relevant data, including purchase history, web interactions, and demographic information. Once this data is gathered, advanced algorithms and machine learning techniques are applied to gain insights.
The effectiveness of Predictive Consumer Analytics lies in its ability to segment customers into different groups based on behavior. These segments can then be used to create personalized marketing campaigns. Additionally, this approach can help identify potential churn risks and inform retention strategies. Ultimately, these insights allow businesses to target the right audience with the right message at the right time, significantly enhancing customer engagement and satisfaction.
The Process of Predicting Consumer Behavior
Predictive Consumer Analytics involves a systematic approach to understanding and forecasting consumer behavior by leveraging data-driven insights. The process begins with data collection, where businesses gather information from various sources, such as online interactions and purchase histories. This wealth of data is then cleaned and organized to ensure accuracy, allowing for effective analysis.
Next, data scientists utilize machine learning algorithms to identify patterns and trends within the data. These algorithms can analyze vast datasets much faster than traditional methods. As patterns emerge, predictive models are built and tested, ensuring they accurately forecast future consumer actions. By continuously refining these models based on new data, businesses can enhance their understanding of consumer behavior, ultimately leading to more tailored marketing strategies and improved customer satisfaction. Effective implementation of this process allows companies to anticipate consumer needs and make data-informed decisions that drive growth.
Tools for Predictive Consumer Analytics
Predictive Consumer Analytics relies on various tools to derive valuable insights from consumer data. These tools enable businesses to analyze trends, understand customer preferences, and forecast behaviors based on historical data. It begins with data collection, where organizations gather extensive information from various sources such as social media, customer feedback, and transaction records.
Several essential tools contribute to effective predictive consumer analytics. For instance, Google Analytics provides valuable insights into website traffic and user behavior. IBM Watson Analytics offers advanced data analysis capabilities, helping businesses uncover patterns. RapidMiner is acclaimed for its predictive modeling and machine learning features, allowing businesses to create data-driven strategies easily. Lastly, Microsoft Azure Machine Learning facilitates cloud-based analytics and deployment of predictive models, making it accessible for various businesses seeking robust analytics solutions. Understanding and utilizing these tools can significantly enhance the decision-making process, ultimately leading to improved customer engagement and satisfaction.
Extract insights from interviews, calls, surveys and reviews for insights in minutes
insight7: The All-in-One Tool for Consumer Insights
insight7 serves as a comprehensive solution for businesses seeking to capitalize on predictive consumer analytics. This all-in-one tool allows users to analyze customer data effectively, providing valuable insights into consumer behavior. By integrating advanced machine learning techniques, insight7 transforms raw data into actionable intelligence, enabling companies to make informed decisions quickly.
The platform addresses significant challenges faced by organizations, such as the inefficiency of manually analyzing customer conversations. Users can seamlessly gather and interpret insights from various channels, enhancing collaboration among teams. It's crucial for businesses to act on customer feedback swiftly to stay ahead of the competition. With insight7, companies can unlock vast amounts of customer signals, ultimately paving the way for improved strategies and better customer engagement. The toolโs user-friendly interface promotes efficient data analysis, enabling organizations to adapt strategies and meet evolving consumer needs.
Other Essential Tools for Consumer Prediction
Predictive Consumer Analytics relies on various essential tools that enhance the accuracy of consumer predictions. Each tool plays a fundamental role in the data analysis ecosystem, enabling businesses to gather insights and drive informed decisions. Here are some tools pivotal in shaping predictive analytics strategies:
Google Analytics: This platform offers extensive website traffic analysis, helping marketers understand user behavior and conversion patterns effectively. By tracking key metrics, businesses can tailor their marketing efforts for better engagement.
IBM Watson Analytics: Known for its advanced data analysis capabilities, this tool utilizes natural language processing and machine learning to deliver actionable insights. It empowers users to visualize complex data sets effortlessly, aiding strategic decision-making.
RapidMiner: With its user-friendly interface, RapidMiner simplifies the process of data preparation and modeling. It enables businesses to build predictive models without the need for extensive programming knowledge.
Microsoft Azure Machine Learning: This cloud-based service provides robust predictive analytics capabilities. Users can create, deploy, and manage predictive models at scale, allowing for efficient adaptation to market changes.
By incorporating these tools into a predictive analytics strategy, businesses can significantly improve their understanding of consumer behavior, which is critical in todayโs fast-paced market environment.
- Google Analytics
Google Analytics plays a pivotal role in predictive consumer analytics by providing actionable insights derived from vast amounts of user data. This tool tracks website traffic and user behavior, enabling businesses to understand their audience better. By leveraging these insights, companies can identify patterns and trends that forecast future consumer actions. For instance, if a specific product consistently attracts more visitors, businesses can predict an increase in sales and adjust their marketing strategies accordingly.
To effectively utilize Google Analytics for predictive analytics, consider the following key aspects:
- User Behavior Tracking: Analyze how consumers interact with your website to identify patterns.
- Conversion Goals: Set clear objectives to measure the effectiveness of marketing campaigns.
- Audience Segmentation: Break down user demographics and behaviors to tailor strategies for targeted groups.
- Data Insights: Use the reports generated to forecast trends and inform decision-making.
By focusing on these elements, businesses can enhance their predictive capabilities, allowing for more informed strategies in meeting consumer needs.
- IBM Watson Analytics
In the realm of predictive consumer analytics, advanced tools enable businesses to decipher vast amounts of data for actionable insights. One prominent tool utilizes machine learning to streamline the analytical process, allowing companies to transform raw data into meaningful patterns. This technology focuses on uncovering customer sentiments and behaviors, making it essential for understanding market dynamics.
By leveraging natural language processing and automated data mining, this tool enhances the accuracy of predictions regarding consumer choices. It facilitates the identification of positive and negative sentiments across numerous datasets, enabling businesses to refine their products and marketing strategies effectively. Furthermore, the capability to compare data across different regions helps identify trends and preferences unique to specific audiences. Through such insights, organizations can forecast consumer behavior with increased reliability, shaping strategies that resonate with their target markets effectively.
- RapidMiner
In the realm of predictive consumer analytics, RapidMiner stands out as a powerful platform. This tool simplifies the process of data analysis and model development, allowing users to harness vast datasets effectively. It provides an intuitive interface, making it accessible even to those without extensive programming expertise. With its robust suite of machine learning algorithms, RapidMiner enables businesses to uncover hidden patterns and correlations within consumer data.
Moreover, RapidMiner supports seamless integration of various data sources. Users can easily import and analyze data from spreadsheets, databases, and cloud storage. By providing valuable visualizations, the platform enhances the understanding of consumer behavior trends. Through its project-based approach, users can manage multiple analyses in one space, making it easier to draw insightful conclusions that inform marketing strategies. Overall, RapidMiner plays a crucial role in empowering organizations to engage in predictive consumer analytics effectively and efficiently.
- Microsoft Azure Machine Learning
Microsoft Azure Machine Learning provides powerful capabilities for predictive consumer analytics. By harnessing vast amounts of data, it allows businesses to identify patterns and trends in consumer behavior. Through advanced machine learning algorithms, Azure enables the development of predictive models that can foresee consumer actions based on historical data. This functionality is essential for tailoring marketing strategies and improving customer experiences.
The tools available in Azure facilitate data exploration and model training, making it accessible for businesses of all sizes. Analyzing past interactions and feedback can lead to actionable insights, guiding product development and enhancing customer engagement. By understanding predictive consumer analytics, organizations can leverage data-driven decisions. Ultimately, this enhances their ability to anticipate market needs and stay ahead of the competition. The integration of machine learning not only streamlines analysis but also empowers businesses with foresight into consumer trends.
Conclusion: The Future of Predictive Consumer Analytics in Shaping Consumer Experiences
Predictive Consumer Analytics is set to revolutionize how businesses understand and engage with their customers. As machine learning continues to advance, companies will increasingly utilize these tools to uncover nuanced consumer patterns. This shift will not only drive more personalized marketing strategies but also enhance overall customer satisfaction.
Looking ahead, the integration of real-time analytics will empower brands to adapt quickly to changing consumer preferences. By harnessing the potential of advanced algorithms, businesses will improve their decision-making processes. Ultimately, embracing predictive analytics will lead to a richer, more meaningful consumer experience that fosters loyalty and drives growth in the market.