The rise of Multimodal AI Evolution heralds a new era in transcription and quality assurance (QA). As businesses realize the limitations of traditional single-modality approaches, they turn to advanced techniques that integrate audio, visual, and text data. This evolution enhances the capabilities of transcription services, ensuring precision and clarity, essential for effective communication. The convergence of various data formats empowers organizations to analyze interactions in real-time, thus elevating the standards of customer engagement.
Moreover, the transformative impact of Multimodal AI on QA systems cannot be overstated. By embracing this integrated approach, organizations can streamline their workflows and improve overall output quality. Enhanced algorithms can now process diverse formats seamlessly, enabling more accurate assessments. As technology continues to evolve, businesses will increasingly rely on these innovative solutions, shaping the future of transcription and QA. The journey into this new frontier is just beginning, creating exciting possibilities for enhanced efficiency and effectiveness.
Analyze & Evaluate Calls. At Scale.
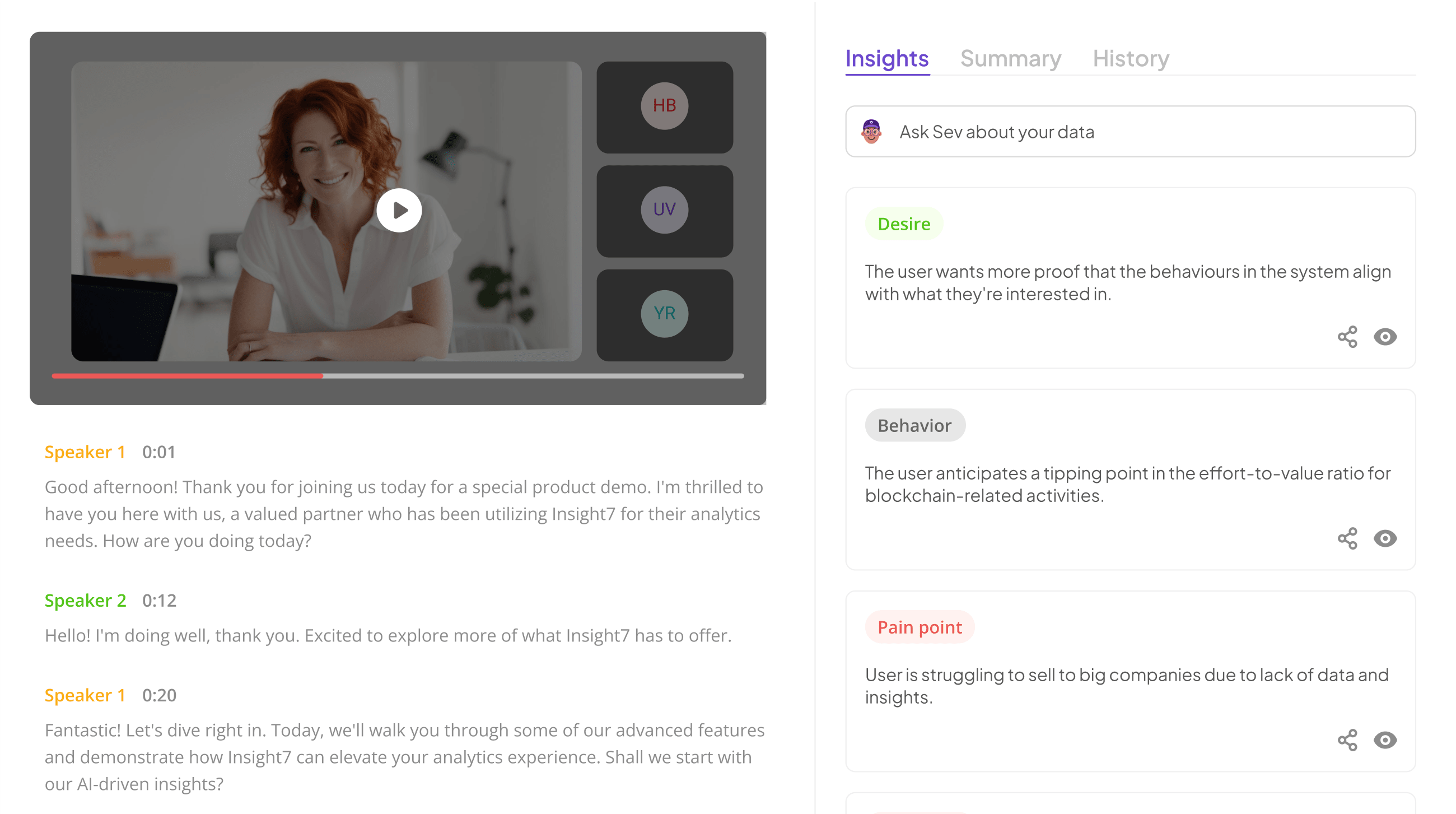
Understanding the Multimodal AI Evolution in Transcription
The Multimodal AI Evolution in transcription marks a significant shift in how we handle audio and text data. At the core of this phenomenon lies the convergence of various data formats that seamlessly collaborate to enhance processes. Instead of relying solely on traditional speech-to-text methods, modern systems integrate text, audio, and even visual inputs. This convergence allows for much greater precision and nuance in understanding transcribed content.
Another driving factor is the advancement of machine learning algorithms, which have become increasingly proficient in analyzing, interpreting, and generating data. This capability translates into enhanced accuracy in transcription services, ensuring that the rendered text accurately reflects spoken language nuances. Furthermore, real-time transcription capabilities are being realized, enabling users to interact with content instantly. Consequently, the Multimodal AI Evolution not only revolutionizes transcription practices but also opens new avenues for data analysis and insight extraction, redefining the transcription landscape.
Key Drivers of Multimodal AI Evolution
The evolution of multimodal AI is significantly driven by the integration of various data formats, such as text, audio, and visual elements. This convergence allows for a richer understanding of information by leveraging context from multiple sources. In transcription services, for instance, combining spoken words with visual cues enhances the accuracy of transcriptions and enables quicker responses to user queries.
Advancements in machine learning algorithms play a crucial role in refining these processes. Improved algorithms enable machines to process and analyze vast amounts of data from multiple modalities efficiently. As a result, organizations can derive actionable insights much faster than traditional methods allow. Consequently, companies that adapt to this evolution quickly will maintain a competitive edge, capitalizing on timely insights to drive decision-making strategies. Embracing these key drivers will continue to shape the capabilities of transcription and question-answering systems in the near future.
- The convergence of multiple data formats
The evolution of multimodal AI is fundamentally reshaping how we interact with and interpret various data formats. As technology progresses, we're witnessing the blending of textual, audio, and visual data into cohesive insights. This convergence allows AI systems to process information more holistically, enhancing their understanding and response capabilities. Multimodal AI evolves by integrating these diverse data types, creating richer contexts that inform both transcription and question-answering systems.
In practice, this means that AI can analyze and summarize content more effectively. For instance, a transcript from a meeting can be enriched with contextual audio cues and visual elements, enabling a deeper analysis of key themes. Patterns and trends emerge from what was once disparate data, providing actionable insights. This convergence not only facilitates better communication and understanding but also empowers organizations to make data-driven decisions that were previously unattainable. Overall, the future of multimodal AI promises to redefine our engagement with information in more profound ways.
- Advances in machine learning algorithms
Recent advancements in machine learning algorithms have significantly contributed to the enhancement of multimodal AI capabilities. These cutting-edge algorithms facilitate the integration of various data types, such as text, audio, and visual inputs, allowing AI systems to better understand and process information. The fusion of these modalities suppresses traditional boundaries, elevating the performance of AI applications, especially in transcription and question answering.
Machine learning techniques like deep learning, transfer learning, and reinforcement learning have become more refined and versatile. Deep learning models are adept at extracting features from multiple data sources, improving accuracy. Transfer learning enables models to adapt knowledge gained in one domain to perform effectively in another, while reinforcement learning aids in continually optimizing AI systems through real-time feedback. Together, these advances in machine learning algorithms power the evolution of multimodal AI, preparing it for broader applications across industries.
Transformative Impact on Transcription Services
The evolution of multimodal AI is reshaping transcription services in remarkable ways. This transformation focuses on integrating various forms of data, enhancing functionality and user experience. One significant impact is the significant improvement in transcription accuracy, ensuring that spoken language is converted to text with high fidelity. The incorporation of real-time capabilities enables users to receive immediate transcripts, which streamlines workflows and saves valuable time.
Moreover, multimodal AI enhances the transcription process through visual cues alongside audio, adding context and clarity. It allows users to pull out insights efficiently from transcripts, transforming raw data into actionable information. This evolution not only optimizes traditional transcription methods but also empowers organizations to analyze conversations at scale. With these advancements, the potential for improved understanding of customer needs and other insights is significant, marking a pivotal shift in the transcription landscape.
- Enhanced accuracy in speech-to-text
Enhanced accuracy in speech-to-text technology is a significant milestone in the Multimodal AI evolution, transforming the way organizations handle transcription services. By integrating multiple data modalities, including audio and visual information, these systems enhance precision in capturing spoken language. This means fewer errors and improved understanding of context, which is vital for delivering high-quality transcripts.
Moreover, real-time transcription capabilities have emerged as a game changer. With advanced algorithms, users can obtain instant transcriptions, allowing for timely analysis and insights. These innovations empower businesses to unlock trends and pain points within conversations effectively. Ultimately, this progression underscores the importance of multimodal approaches, ensuring a seamless and accurate transcription experience that meets diverse user needs. As technology evolves, the potential for error-free, contextually rich text generation continues to grow.
- Real-time transcription capabilities
Real-time transcription capabilities are integral to the ongoing Multimodal AI Evolution. With rapid advancements in technology, these capabilities enable users to convert speech into text instantaneously. This immediate transformation enhances various fields, such as customer service, where quick response times are crucial. The ability to transcribe calls or meetings as they occur allows for seamless communication and information retention.
Moreover, the functionality to handle multiple files simultaneously represents a significant leap in efficiency. Businesses can now analyze several conversations simultaneously, extracting valuable insights swiftly. This is particularly beneficial for organizations seeking to mine large datasets for trends or customer feedback. As real-time transcription continues to mature, it supports more robust analytics tools, making it easier to identify key insights without extensive delays. Ultimately, these advancements set the stage for richer interactions and improved decision-making processes in a variety of sectors.
Extract insights from interviews, calls, surveys and reviews for insights in minutes
Multimodal AI Evolution and Its Effect on Question Answering Systems
The evolution of multimodal AI is significantly impacting question answering systems. This development incorporates various data formatsโtext, audio, and visualโthat enhance the understanding of user queries. As a result, systems now excel in providing personalized answers to complex questions, elevating user satisfaction. This evolution marks a shift from traditional text-based responses to richer, more contextual interactions. Users benefit from engaging with systems that can process visual and auditory inputs alongside text, thereby improving accuracy and relevance in responses.
Implementing multimodal AI in question answering involves several steps. First, effective data collection and processing ensure that diverse data formats are assembled for analysis. Next, training models with these multimodal inputs enhances system comprehension, allowing for nuanced responses. Finally, deploying the system requires continuous learning to adapt to user interactions and feedback, ensuring ongoing improvement. Thus, the multimodal AI evolution is reshaping the landscape of question answering, paving the way for smarter, more intuitive systems that cater to user needs.
Evolution from Text-Based to Multimodal Inputs
The transition from text-based inputs to multimodal inputs marks a significant milestone in the Multimodal AI Evolution. Initially, transcription and question-answering systems predominantly relied on textual data, limiting their ability to understand context and nuance fully. As technology advanced, integrating visual and audio data alongside text became essential. This shift allows for a richer understanding of user intents, making systems more responsive and effective.
The evolution has also fostered personalization in answering complex queries. Users now expect systems that can draw insights from various data formats, enhancing engagement and satisfaction. Moreover, as organizational needs grow increasingly complex, the demand for efficient, multimodal solutions will likely continue to rise. By embracing multimodal inputs, businesses can improve their customer interactions, ultimately leading to more meaningful insights and strategic decisions.
- Integration of visual and audio data with text
The integration of visual and audio data with text represents a significant step in the Multimodal AI Evolution. By uniting diverse data types, systems can better comprehend context and enhance the user experience. This combination allows for a richer understanding of information, as visuals, sound, and text can provide complementary cues that strengthen overall comprehension.
As audio, video, and text data converge, transcription accuracy improves dramatically. For instance, during customer interviews, visual cues can reveal non-verbal communication insights, while audio recordings capture tone and emotion. Together, these elements create a comprehensive understanding of the conversation, empowering organizations to derive meaningful insights from what was discussed. As this technology matures, we will witness a profound shift in how we approach transcription and answer complex questions, making AI-driven solutions increasingly essential in various industries.
- Personalization in answering complex queries
As organizations strive to meet the evolving needs of their users, personalization in answering complex queries becomes increasingly essential. Multimodal AI evolution plays a significant role in reshaping this process, enabling systems to process various data formats beyond text. The current landscape allows for the integration of voice, images, and other inputs, leading to smarter interactions. This capability not only enhances users' experiences but also streamlines the retrieval of information relevant to their inquiries.
To achieve effective personalization, there are a few key components to consider. First, understanding user preferences and behavior is crucial for tailoring responses. Second, advanced algorithms must analyze multimodal inputs, allowing the system to engage naturally. Lastly, continuous learning mechanisms should adapt to users' feedback over time. As a result, organizations can improve the accuracy and relevancy of answers provided, ultimately leading to higher satisfaction and more meaningful engagement.
Step-by-Step: Implementing Multimodal AI in QA
To effectively implement Multimodal AI in Quality Assurance (QA), begin with robust data collection and processing. The first step involves gathering diverse data types, including audio, video, and text files, which are pivotal to enriching the evaluation process. A clean and well-organized dataset enhances the AI system's learning capability, allowing it to recognize patterns across various formats. This is essential for ensuring comprehensive analysis in QA tasks.
Next, train your models with these multimodal inputs. This phase is crucial, as it involves teaching the AI to comprehend and analyze different data types simultaneously. Optimizing model architecture to handle diverse inputs can significantly improve performance. Finally, system deployment should incorporate continuous learning mechanisms to refine model outputs over time. Successful implementation of these steps will reflect the full potential of the Multimodal AI evolution, yielding more accurate and insightful evaluations in QA processes.
Step 1: Data Collection and Processing
Data collection and processing serve as the foundational step in the Multimodal AI evolution for transcription and quality assurance. This phase revolves around gathering diverse data sources, including audio files, transcripts, images, and video content. A robust dataset ensures that the AI system learns from multiple perspectives, enhancing its ability to provide accurate transcriptions and answers.
The initial task involves identifying the right data sources relevant to the specific needs of the task at hand. Gathering high-quality and varied datasets enhances the learning experience for the AI models used in transcription. Once collected, processing this data is essential; it requires cleaning, normalizing, and organizing the information into usable formats. This systematic approach not only streamlines the learning process but also helps in understanding user sentiments, a critical component in both transcription accuracy and effective question-answering systems.
Step 2: Model Training with Multimodal Inputs
Model training with multimodal inputs is a crucial step in refining the capabilities of Multimodal AI Evolution. This process involves integrating diverse data types, such as text, audio, and visual inputs, to enhance the model's overall learning and performance. By combining these varied inputs, models can better understand context, leading to more accurate outputs, particularly in transcription and question-answering tasks.
To effectively train models using multimodal inputs, three fundamental steps should be followed:
Data Alignment: Ensure that different data types are synchronized to allow the model to learn from them together. For example, pairing audio transcripts with their corresponding visual cues can enhance comprehension.
Feature Extraction: Identify and extract relevant features from each data modality. This involves using algorithms to capture distinctive characteristics from videos, audio clips, and text documents.
Integration and Training: Combine all extracted features into a unified model. This integrated model undergoes training to discern relationships across modalities, ultimately leading to more accurate predictions and insights in real-world applications.
Through this structured approach, the foundation for further advancements in Multimodal AI can be solidified, driving innovation in fields like transcription and quality assurance.
Step 3: System Deployment and Continuous Learning
Deploying a system is just the initial step in embracing Continuous Learning within the Multimodal AI Evolution. Once the transcription and question answering systems are operational, continuous monitoring and enhancement become essential. The insights gained from user interactions can guide iterative improvements, ensuring the technology adapts to changing needs and environments. This ongoing process facilitates better outcomes, as each cycle of feedback refines the system's performance.
Ongoing training and development of these models allow them to learn from new data continually. By analyzing various inputs like conversations, surveys, and customer feedback, the system accumulates knowledge that enhances its accuracy and responsiveness. Such a dynamic approach guarantees that the system remains relevant, efficiently addressing user inquiries and improving transcription quality over time. Ultimately, the success of Multimodal AI hinges on its ability to evolve in response to real-world applications.
Leading Tools Powering The Rise of Multimodal AI
The increasing demand for efficient transcription and question-answering systems has fostered a significant momentum in the Multimodal AI evolution. Leading tools in this space are making profound changes by integrating various data sources, including text, speech, and even visual content. These advancements empower organizations to understand customer insights in deeper, more nuanced ways.
Prominent tools such as Deepgram and AssemblyAI are enhancing transcription accuracy with robust machine learning models. Otter.ai and Rev.com excel in providing real-time transcription capabilities, allowing users to capture conversations more effectively. These tools not only streamline data analysis but also facilitate smooth collaboration by unifying insights into accessible formats. As organizations adopt these advanced solutions, they can bridge the gap between traditional data handling techniques and the needs of the modern, fast-paced business environment. This evolution is essential for remaining competitive and responsive to customer demands.
insight7
The evolution of multimodal AI is reshaping transcription and QA processes significantly. Its rise is driven by the need for more efficient data analysis methods that can process diverse inputsโsuch as audio, text, and imagesโsimultaneously. Businesses generating vast amounts of customer interactions often struggle to translate this data into actionable insights using traditional techniques. However, the integration of various data formats in a streamlined manner enhances both accuracy and speed.
To realize the benefits of multimodal AI in transcription and QA, organizations should focus on a structured implementation approach. First, data collection and processing should aggregate inputs from different sources effectively. Next, model training with these diverse datasets is crucial to improving system responses. Finally, continuous learning during deployment ensures that the system adapts to new information, maintaining its relevance and effectiveness. With these steps, companies can harness the potential of multimodal AI to drive insightful decision-making.
- Overview and features
The rise of Multimodal AI in transcription showcases a significant evolution that offers immense benefits. Understanding the overview and features of these technologies reveals how they function to improve user experiences. Multimodal AI pulls together various data formats such as text, audio, and visual elements, creating a cohesive framework for transcription and question-answering.
Key features of this evolution include enhanced accuracy in converting speech to text and real-time transcription capabilities. These advancements allow users to easily translate complex conversations into formatted reports. Additionally, the intuitive interface ensures that anyone can utilize the technology efficiently, requiring no specialized training. As organizations seek greater accessibility to insights, the democratization of data through such tools fosters a culture of data-driven decision-making. This transition is crucial for adapting to modern challenges in transcription and quality assurance.
Additional Tools
The development of Multimodal AI has spurred various additional tools tailored to enhance transcription and quality assurance processes. These tools harness cutting-edge technologies that integrate audio, visual, and text data, refining how information is captured and analyzed. A suite of tools like Deepgram, AssemblyAI, and Otter.ai stand out for their powerful capabilities, each contributing uniquely to the transcription landscape.
Consider Deepgramโs advanced speech recognition technology, delivering real-time transcription with impressive accuracy. AssemblyAI focuses on customizable AI models that adapt to various use cases, allowing users to tailor their transcription needs effectively. Otter.ai is particularly known for its collaborative features that make it easy to share, edit, and review transcripts. Rev.com combines human expertise with AI efficiency, offering a hybrid approach that ensures high-quality outputs. Sonix provides an intuitive platform for managing and distributing transcripts. Each of these tools illustrates how the Multimodal AI evolution is reshaping transcription and QA, paving the way for more efficient workflows.
- Deepgram
The advancements in transcription technology are being significantly enhanced by the rise of multimodal AI, enabling more intuitive analysis. Users can now interact with various data formats, making it easier to transcribe recordings at scale. This evolution allows for simple bulk processing, ensuring that insights from numerous conversations can be efficiently extracted and analyzed.
Consumers appreciate the seamless experience, where they can upload audio files and receive accurate transcripts in no time. The incorporation of templates aids in identifying key insights, such as customer pain points or preferred interactions, ensuring that relevant information is highlighted. By applying analysis tools to these transcriptions, organizations enhance their understanding of interactions, enabling better customer engagement strategies. As multimodal AI continues to evolve, its role in transcription will reshape how businesses leverage information for improved decision-making and customer satisfaction.
- AssemblyAI
As the field of transcription evolves, tools embracing multimodal AI are transforming the way we interact with audio data. These technologies enhance transcription accuracy by integrating visual and semantic cues. The result is an unparalleled ability to comprehend context, signifying a significant evolution in transcription capabilities. This transformation is driven by intensive research, leading to advanced algorithms that can process diverse data types, ultimately supporting more complex transcription tasks.
Furthermore, the implementation of multimodal AI can improve quality assurance systems that rely on verbal communication. By accurately identifying speakers and tracking performance metrics, organizations can streamline their evaluation processes. This not only enhances compliance but also enables dynamic feedback to optimize performance. The ongoing evolution of multimodal AI is set to reshape the transcription landscape, paving the way for more sophisticated and user-friendly solutions. As these innovations develop, organizations must remain attuned to how they can harness these technologies effectively.
- Otter.ai
The rise of multimodal AI has paved the way for innovative transcription solutions that enhance the user experience significantly. These advancements enable systems to integrate diverse data formats, improving the overall quality of transcriptions. By using sophisticated algorithms, this technology captures spoken words with remarkable accuracy, ensuring that every nuance and detail is documented.
One notable aspect of this evolution is its ability to provide real-time transcription capabilities. This feature is particularly valuable in fast-paced environments where immediate access to information can drive decision-making. As multimodal AI continues to evolve, users will benefit from better insights and improved efficiency, ultimately transforming the landscape of transcription services. The future seems bright for those seeking seamless integration between voice and text, as this innovative technology becomes more accessible in various applications.
- Rev.com
The evolution of multimodal AI has significantly reshaped transcription services, making them more efficient and accurate. Central to this evolution is a platform that prioritizes user accessibility and data analysis. By integrating advanced technologies, it helps organizations process customer interactions more effectively. Businesses are increasingly generating immense amounts of customer data, yet traditional methods struggle to keep pace. Consequently, the need for innovative solutions has never been greater.
This platform addresses critical challenges in data analysis by facilitating real-time insights from customer conversations. It enables users to transform scattered information into actionable strategies, enhancing collaboration and efficiency. Critics of conventional transcription systems often highlight time-consuming manual processes, which can delay insights crucial for competitive advantage. By embracing this multimodal approach, companies can streamline their transcription workflows and leverage timely insights to stay ahead in a rapidly evolving market.
- Sonix
As organizations strive to enhance their data processing capabilities, several innovative tools emerge as frontrunners in the realm of Multimodal AI evolution. One noteworthy tool within this landscape focuses on harnessing the power of various data inputs, shifting from traditional methods to smarter solutions. This platform exemplifies the transformative journey many companies undertake to keep pace with digital conversations and customer insights.
By integrating advanced algorithms, this tool offers exceptional accuracy in converting speech to text while processing multiple formats simultaneously. This capability not only improves the speed of transcription but also ensures that organizations can derive actionable insights from audio, visual, and text data, ultimately streamlining their operations. As businesses encounter an influx of customer signals, the necessity for such intelligent solutions becomes clearer, positioning them strategically in competitive markets.
Conclusion: The Future of Multimodal AI Evolution in Transcription and QA
The future of Multimodal AI evolution in transcription and quality assurance is poised for remarkable advancements. As technology continues to progress, the integration of various data types will enhance the way we capture, analyze, and interpret information. This evolution is not merely about improving efficiency; itโs also about enriching the user experience through more accurate and context-aware insights.
In the coming years, we can expect increased collaboration between audio and visual elements, which will foster deeper understanding in transcription services. Moreover, quality assurance processes will likely become more dynamic, relying on multifaceted data analytics to ensure compliance and elevate customer experiences. Ultimately, embracing this evolution will not only streamline workflows but also empower organizations to make informed decisions based on comprehensive insights.