The Influence of Social Media Algorithms on Research Findings
-
Bella Williams
- 10 min read
Algorithmic Research Bias refers to the inherent issues that arise when social media algorithms dictate the accessibility and visibility of information. Social media platforms utilize complex algorithms that prioritize certain content, often narrowing down the type of data researchers encounter. This phenomenon can lead to skewed research outcomes since the information collected may not represent a comprehensive view of public sentiment or opinion.
Moreover, the echo chamber effect created by algorithms further complicates the research landscape. Users are frequently exposed to a limited scope of perspectives, which can stifle diverse opinions that are critical in shaping accurate research findings. Therefore, understanding Algorithmic Research Bias is vital for researchers aiming to draw valid conclusions from social media data. Recognizing these challenges can enable more effective methodologies and improve the overall reliability of research outcomes.
Analyze & Evaluate Calls. At Scale.
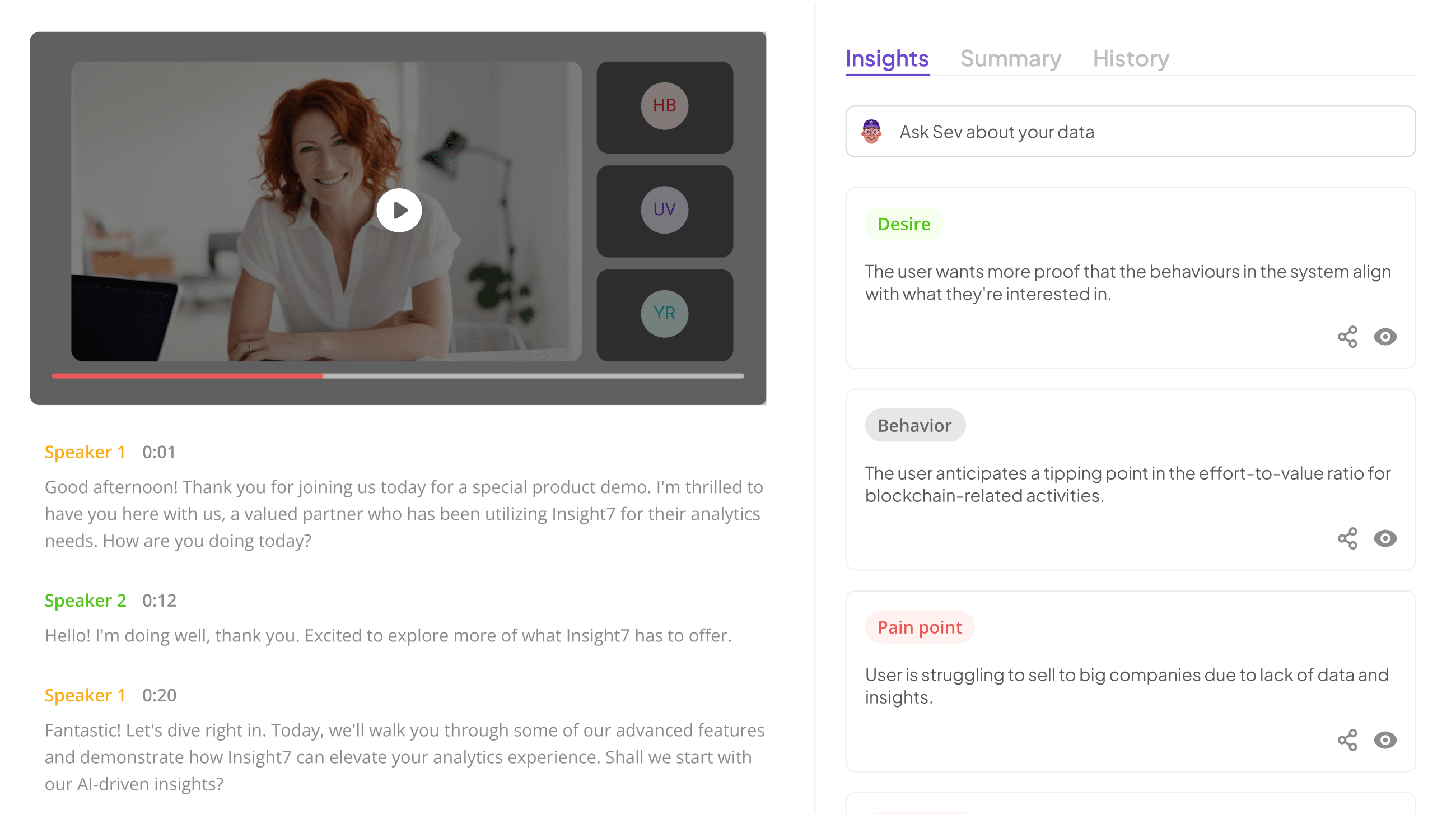
The Role of Algorithmic Research Bias in Shaping Study Outcomes
Algorithmic Research Bias plays a significant role in shaping study outcomes, particularly within social media research. As algorithms curate and filter online content, they inherently influence the information a researcher encounters. This selective prioritization can skew the breadth of data collected, often omitting valuable insights from diverse perspectives. Consequently, research findings may only reflect a narrow viewpoint, diminishing the legitimacy and applicability of the conclusions drawn.
Moreover, algorithmic filtering fosters echo chambersโenvironments where users only encounter ideas that confirm their pre-existing beliefs. These echo chambers not only limit the diversity of information but also restrict researchers' exposure to contrasting opinions and critical discourse. As a result, the integrity of research can be compromised, leading to biased outcomes that fail to represent broader societal views. Researchers must be cognizant of these biases and take proactive measures to mitigate their effects in order to produce more reliable and comprehensive studies.
How Algorithms Affect the Diversity of Information Collected
Algorithms play a critical role in shaping the variety of information that researchers can access, often influencing outcomes significantly. Algorithmic filtering prioritizes specific content based on user behavior and engagement metrics, creating a landscape where certain voices and perspectives are amplified while others are diminished. This selective visibility affects the diversity of data available for research, leading to potential gaps in insight and understanding.
Additionally, algorithms can foster echo chambers, wherein users are exposed primarily to viewpoints that align with their existing beliefs. This phenomenon limits the breadth of information that researchers encounter, reducing exposure to contrasting ideas that may be essential for robust analysis. As a result, researchers must navigate the complexities of algorithmic research bias, being mindful of these dynamics to ensure comprehensive and diversified findings. By understanding how algorithms shape information accessibility, researchers can take proactive steps to mitigate bias in their research processes.
- Algorithmic Filtering: Discuss how algorithms prioritize certain content, affecting the breadth of research data.
Algorithms designed for content delivery on social media platforms often prioritize popular posts over others, which can significantly shape the breadth of research data. This algorithmic filtering may lead to the overrepresentation of certain viewpoints while entirely omitting diverse perspectives. As a result, such bias in content prioritization may skew the overall narrative, limiting researchers' ability to gather comprehensive insights.
Furthermore, algorithmic research bias plays a critical role in how information is consumed and shared. When algorithms focus heavily on engagement metricsโlikes, shares, and commentsโthey inadvertently create echo chambers, reinforcing existing beliefs rather than challenging them. Thus, researchers must be vigilant about the data they analyze, ensuring that they seek out diverse sources to counteract the inherent biases of algorithms. Engaging with a broader range of voices can illuminate underrepresented areas, ultimately leading to richer, more nuanced research findings.
- Echo Chambers: Examine how algorithms can create echo chambers, limiting exposure to diverse perspectives in research.
Algorithms play a significant role in shaping our online experiences, particularly in creating echo chambers that restrict our exposure to differing viewpoints. These algorithms, designed to prioritize content that aligns with users' preferences and past interactions, often lead to a narrow range of information being presented. Consequently, researchers may find themselves operating within a bubble, where alternative perspectives are overlooked. This phenomenon can skew research findings, reinforcing existing beliefs instead of fostering informed debates.
The limitations imposed by these echo chambers highlight the concept of algorithmic research bias. Researchers relying heavily on social media as a source of data may inadvertently present a skewed image of public sentiment and important issues. To mitigate this bias, itโs crucial for researchers to actively seek diverse perspectives and challenge algorithm-driven assumptions about what constitutes valuable information. By recognizing and adjusting for these biases, research can attain a more balanced representation that reflects the complexities of public opinion.
[before proceeding further, its essential to understand the tools that can assist researchers in mitigating algorithmic biases in their findings.]Tools for Mitigating Algorithmic Research Bias
Tools for mitigating algorithmic research bias play a crucial role in improving the reliability of research findings derived from social media. By utilizing specific tools, researchers can effectively counteract biases that arise due to algorithmic filtering and echo chambers. For instance, platforms like insight7 empower researchers to analyze vast datasets comprehensively, offering insights that span diverse content and perspectives.
In addition, several other tools are essential for researchers. One tool aids in balancing algorithmic bias during data collection, helping to ensure a more representative sample of the information available online. Another tool expands the research perspective by integrating various social media sources, thus overcoming the limitations imposed by any single platform. Finally, certain analytics tools specialize in detecting and rectifying discrepancies in data, thereby enhancing the overall validity of research outcomes. By implementing these tools, researchers can work toward minimizing algorithmic research bias and fostering more accurate insights.
insight7
Algorithmic Research Bias can profoundly influence how research findings are shaped in the realm of social media. Social media algorithms prioritize certain content, which can lead to significant gaps in understanding diverse perspectives. As researchers rely on insights gathered from these platforms, they may inadvertently amplify specific narratives while sidelining others, thus skewing their results.
Addressing Algorithmic Research Bias begins with recognizing the challenges it entails. One major issue is data skewness, where algorithms promote popular content over informative contributions. This filtering can create a misrepresentation of public sentiment and reduce the richness of the data collected. Furthermore, to counter this bias, researchers can adopt various strategies. Enhanced data sampling is essential, allowing for a broader array of voices and opinions to inform findings. Cross-platform analysis also proves beneficial, as it helps balance insights against different algorithmic influences. Embracing these practices can lead to more accurate and reliable research outcomes.
- Overview: Introduce insight7 and its capabilities in analyzing social media data in a comprehensive manner.
Insight7 offers a robust framework for analyzing social media data, catering to the complex needs of modern researchers. With the rise of algorithmic research bias, it is crucial to untangle how social media algorithms can inadvertently skew research findings. Insight7's platform enables researchers to extract meaningful insights from vast datasets, ensuring that diverse perspectives are represented. By streamlining the analysis of customer conversations, researchers can effectively turn qualitative data into actionable strategies.
The capabilities of Insight7 are particularly relevant in addressing the challenges posed by algorithms. The platform simplifies data collection, allowing users to navigate through the overwhelming amount of information generated online. It fosters collaboration by centralizing insights, which can easily be converted into business recommendations. As researchers strive to mitigate algorithmic bias in their studies, Insight7 stands out as an invaluable tool that empowers them to analyze social media data comprehensively and efficiently.
Other Essential Tools for Researchers
Researchers now have access to a variety of tools designed to address the challenges of algorithmic research bias. These tools can significantly enhance the quality and reliability of research outcomes by offering diverse perspectives on data. The first category of these essential tools focuses on data collection methods. Utilizing tools that incorporate multiple data sources allows researchers to gather broader insights and minimize the effects of algorithmic filtering.
Another category emphasizes data analysis capabilities, which are critical for recognizing and correcting biases. These technologies help researchers pinpoint biases embedded in the data, enabling them to make informed decisions. Lastly, tools for visualization play a vital role in presenting data in understandable formats, facilitating the identification of trends and anomalies that may arise from algorithm-driven systems. By combining these tools effectively, researchers can significantly reduce algorithmic research bias, leading to more accurate findings.
- Tool A: Explain its role in balancing algorithmic bias in data collection.
In the context of mitigating algorithmic research bias, Tool A plays a vital role by promoting fairness in data collection practices. This tool enables researchers to identify and address biases that may arise during the data gathering process. By offering features that assess and correct data skewness, Tool A empowers researchers to achieve a more representative sample, ensuring diverse perspectives are included in their findings.
Additionally, Tool A facilitates cross-platform analysis, allowing researchers to compare datasets across different social media platforms. This practice broadens the scope of data collected, mitigating the risk of echo chambers that might limit the variety of insights. Ultimately, integrating Tool A into research methodologies enhances the reliability of outcomes, thereby reducing the influence of algorithmic bias in data collection. By ensuring a balanced approach, Tool A supports researchers in achieving more accurate and equitable research results.
- Tool B: Discuss how it helps in broadening the research perspective.
Utilizing advanced tools can significantly broaden researchers' perspectives, particularly when examining the influence of social media algorithms. These tools help facilitate a more comprehensive understanding of how data is filtered and present, ultimately addressing issues of algorithmic research bias. By leveraging various analytic capabilities, researchers can uncover diverse viewpoints that may otherwise remain hidden due to the algorithms' inherent preferences.
One key advantage of employing such tools is their ability to aggregate data from multiple sources, thereby reducing dependency on singular platforms which can create echo chambers. This cross-platform analysis allows researchers to capture a fuller range of opinions and insights, fostering richer outcomes in their studies. Additionally, these tools often provide customizable queries that enable in-depth examinations of user sentiments and responses. As a result, researchers can challenge existing assumptions and explore contradictions, ultimately contributing to more robust and reliable research findings.
๐ฌ Questions about The Influence of Social Media Algorithms on Research Findings?
Our team typically responds within minutes
- Tool C: Highlight its features in detecting and correcting research bias.
Tool C plays a crucial role in detecting and correcting research bias, especially in the context of algorithmic influences on social media data. One key feature is its ability to analyze data from diverse sources, ensuring that varied perspectives are included. This reduces the risk of algorithmic bias by broadening the data pool beyond what algorithms typically prioritize. Moreover, Tool C employs advanced statistical methods to identify anomalies in research data, which can indicate bias created by social media algorithms.
Additionally, it offers correction mechanisms that allow researchers to adjust their findings based on detected biases. This ensures that the outcomes are more representative of the actual user sentiments and behaviors, rather than skewed by algorithmic filtering or echo chambers. By enhancing research integrity, Tool C significantly aids in minimizing algorithmic research bias, ultimately leading to more reliable and comprehensive insights.
Extract insights from interviews, calls, surveys and reviews for insights in minutes
Algorithmic Research Bias: Challenges and Solutions in Data Collection
Algorithmic Research Bias presents significant challenges in data collection, often resulting in skewed findings that can misrepresent social phenomena. One of the primary hurdles is data skewness, where algorithms filter and prioritize specific content, leading to an incomplete representation of opinions and behaviors. This filtering creates research findings that may inadvertently emphasize certain narratives while overlooking others. Additionally, temporal bias arises when algorithms prioritize recent content, which can distort the historical context necessary for comprehensive analysis.
To mitigate these challenges, researchers can adopt several strategies. Enhanced data sampling techniques, for instance, can help ensure a more representative selection of data that reflects diverse perspectives. Furthermore, conducting cross-platform analysis allows researchers to gather insights from multiple social media platforms, balancing the impact of any single platform's algorithm. By implementing these solutions, the integrity of research findings can be preserved, ultimately leading to more reliable and actionable insights.
Recognizing Challenges Imposed by Algorithms
Algorithms play a crucial role in shaping the insights we gather from social media. However, recognizing challenges imposed by algorithms is essential for accurate research findings. One significant issue is the phenomenon of data skewness, where algorithms prioritize certain content over others. This filtering can lead to an incomplete or biased representation of opinions, ultimately distorting research outcomes.
Moreover, temporal bias emerges when algorithms favor trending content, which may overlook long-term sentiments. As a result, researchers face significant hurdles in capturing a holistic view of public opinion. To counteract these challenges, strategies such as enhanced data sampling and cross-platform analysis become vital. By understanding these limitations, researchers can take proactive steps to mitigate algorithmic research bias, ensuring their findings reflect a diverse and accurate perspective.
- Data Skewness: Analyze how algorithms cause data skewness and impact research findings.
Algorithms play a crucial role in shaping the data we encounter on social media, often leading to algorithmic research bias. This bias results from the way algorithms prioritize specific content, which can create data skewness. When researchers rely on socially curated information, they may inadvertently overlook diverse viewpoints and miss critical insights. The filtering process often leads to a concentration of certain opinions, distorting overall findings.
Moreover, the phenomenon of echo chambers exacerbates the issue. By continuously presenting similar perspectives, algorithms limit exposure to contrasting views, impacting the comprehensiveness of research. As a consequence, studies may present skewed results that reflect only a narrow slice of reality, hindering the potential for informed decision-making. To mitigate these biases, researchers must adopt strategies that enhance data sampling and incorporate cross-platform analysis. By actively acknowledging and addressing algorithmic research bias, they can develop more nuanced and representative conclusions.
- Temporal Bias: Examine the effect of algorithms on the timeliness of data.
Algorithms are integral in shaping the timeliness of data that researchers rely on, often creating a phenomenon known as temporal bias. They influence which data points are presented and how recent they are, significantly affecting research findings. For instance, social media platforms prioritize trending topics, which may overshadow crucial information that is less current yet essential for comprehensive analysis. This prioritization can lead to a skewed understanding and perpetuate the dominance of popular narratives over critical insights.
Additionally, temporal bias can constrain researchers, limiting their access to longitudinal data that may provide essential context. As algorithms evolve, they may inadvertently favor instantaneous reactions over curated, valuable information. It is essential for researchers to recognize these biases and actively seek methods to counteract them. By employing diverse sampling techniques and looking beyond the immediate trends, researchers can ensure that their findings are grounded in a robust temporal framework and reflect a more holistic view of the subject at hand.
Steps to Minimize Algorithmic Research Bias
To minimize algorithmic research bias, researchers must adopt strategic methods that enhance their dataโs integrity and representativeness. One essential step involves enhanced data sampling, which encourages the collection of a diverse array of data from varied demographics. This practice helps to ensure that the findings reflect a broader spectrum of perspectives rather than being shaped by a select group favored by algorithms.
Another critical approach is cross-platform analysis. By examining data across multiple social media platforms, researchers can uncover trends and insights that may be overlooked when relying solely on one source. This practice diminishes the risk of echo chambers and biases that emerge from platform-specific algorithms. Collectively, these steps promote a more comprehensive understanding of research findings, thereby improving the credibility and reliability of the work produced.
Step 1: Enhanced Data Sampling
To address algorithmic research bias effectively, enhanced data sampling serves as a foundational approach. By strategically designing the data collection process, researchers can mitigate the inherent biases caused by algorithms on social media platforms. This involves diversifying sources to ensure a more representative dataset. An effective data sampling strategy incorporates various demographics, interests, and geographic locations, aiming to reflect the broader population rather than a narrow segment influenced by algorithmsโ filtering effects.
Moreover, leveraging robust sampling techniques can reveal hidden insights that might otherwise be overlooked. Researchers should actively seek out underrepresented voices and perspectives, as this inclusion curtails the impact of echo chambers that algorithms often create. Effectively balancing diverse inputs not only enriches the data but also provides a more accurate picture of social phenomena. Ultimately, enhanced sampling allows for a deeper understanding of the research subject while reducing the likelihood of skewed findings stemming from algorithmic preferences.
- Strategy: Describe strategies for obtaining a more representative data sample.
To obtain a more representative data sample, researchers must implement targeted strategies that counteract algorithmic research bias effectively. A crucial first step is enhanced data sampling. This involves selecting diverse participant groups that reflect the wider population. Researchers should consciously include individuals from various demographics, socio-economic backgrounds, and geographic locations to capture a holistic view of the subject matter. Such diversity ensures that the data collected is not skewed toward a singular perspective.
Another essential strategy is conducting cross-platform analysis. By gathering data from multiple social media platforms, researchers can examine varying audience interactions and sentiments, thereby gaining a broader understanding of trends. This approach also helps mitigate the echo chamber effect frequently caused by platform-specific algorithms. Lastly, constant evaluation and adjustment of data collection methods is imperative to remain aligned with changing algorithm dynamics and user behaviors, ultimately leading to more reliable research findings.
Step 2: Cross-Platform Analysis
By engaging in cross-platform analysis, researchers can counteract the impact of social media algorithms on their findings. This step involves examining data collected across various platforms, allowing for a more holistic understanding of user behaviors and preferences. The diversity of inputs reduces the risk of algorithmic research bias, as it captures a broader spectrum of voices and experiences.
During this analysis, researchers can identify common themes and differences in user engagement influenced by specific algorithms. By cross-referencing insights from platforms like Twitter, Facebook, and Instagram, scholars can assess how algorithmic curation may skew the data towards particular demographics or opinions. This approach not only enhances the reliability of research findings but also encourages transparency and a more accurate representation of the subjects studied. Ultimately, cross-platform analysis serves as a vital practice for minimizing the inherent biases introduced by social media algorithms.
- Practice: Explain how examining data across multiple platforms can provide a more balanced view.
A comprehensive examination of data across various platforms offers researchers a more balanced view and helps mitigate aspects of Algorithmic Research Bias. Different platforms may employ differing algorithms that shape the information users encounter. By diversifying data sources, researchers can capture a wider array of perspectives, reducing the likelihood of skewed findings. A single platform may present a narrow range of opinions, and expanding the analysis to include multiple viewpoints can uncover biases inadvertently embedded in algorithms.
Cross-platform analysis enables the identification of patterns and discrepancies in user feedback. For instance, insights collected from Twitter might differ significantly from those obtained on Facebook due to algorithmic filtering and user demographics. This diverse set of data helps paint a fuller picture, allowing researchers to recognize areas of consensus as well as disagreement. By integrating these varied insights, the research becomes more robust, ensuring that findings are representative and not confined to a limited perspective influenced by social media algorithms.
Conclusion: Addressing Algorithmic Research Bias in Modern Research
To effectively address algorithmic research bias, researchers must first acknowledge its presence within their methodologies. Algorithms inherently filter information, which can lead to a narrowed perspective and skewed findings. By understanding how these biases operate, researchers can develop strategies that foster a more inclusive and diverse data collection process.
Implementing cross-platform analysis is one such strategy. It allows researchers to compare insights across various social media platforms, revealing patterns that might otherwise remain hidden. Additionally, enhancing data sampling methods can ensure that diverse voices are represented, ultimately leading to more reliable research outcomes. In recognizing and addressing algorithmic research bias, the integrity and relevance of research findings can be significantly strengthened.
๐ฌ Questions about The Influence of Social Media Algorithms on Research Findings?
Our team typically responds within minutes