The Impact of Social Media Algorithms on Research Findings
-
Bella Williams
- 10 min read
Algorithmic Bias Effects shape the landscape of social media research findings in profound ways. As researchers increasingly rely on social media data, they must acknowledge how algorithms influence which information is presented and subsequently analyzed. The inherent biases in these algorithms can filter diverse opinions, leading to skewed interpretations of public sentiment and behavior.
Understanding these effects is crucial, given that they can mislead researchers and result in unreliable conclusions. Furthermore, researchers often fall prey to cognitive and confirmation biases, where pre-existing beliefs overshadow objective analysis. Thus, identifying and addressing these biases is essential for ensuring the validity of social media research and fostering more reliable insights in future studies.
Analyze & Evaluate Calls. At Scale.
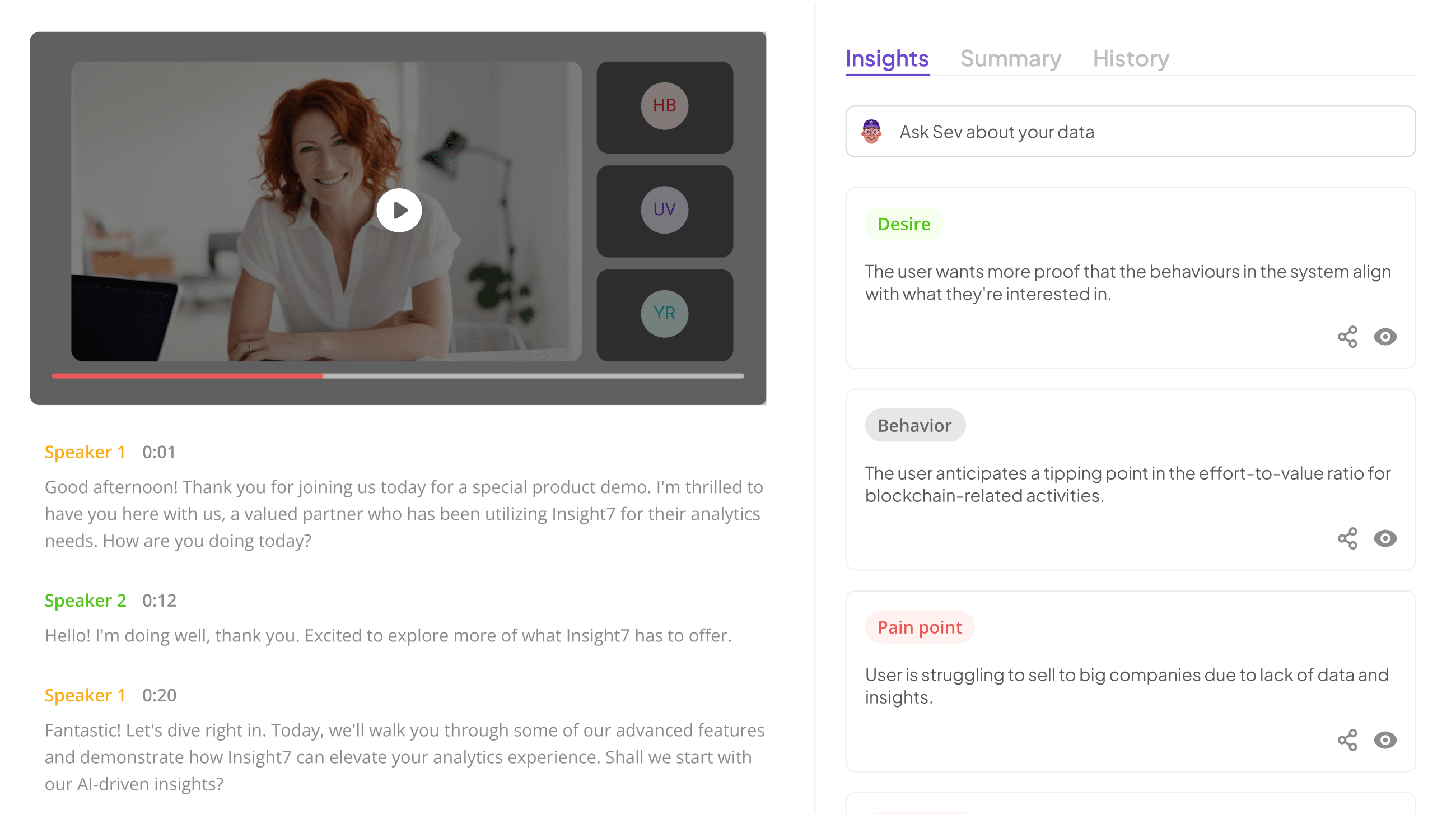
Understanding Social Media Algorithms and Their Mechanisms
Social media algorithms dictate which content users see, profoundly affecting research findings. Understanding how these algorithms work involves recognizing their core objectives: maximizing user engagement and retention. This leads to a content curation process that often prioritizes popularity over diversity, potentially resulting in algorithmic bias effects that skew the research landscape.
Key mechanisms include personalization, where individual user behavior shapes content visibility. When users interact with certain topics, algorithms favor similar content, creating echo chambers. Moreover, engagement metrics profoundly influence what researchers encounter. This focus on engagement may inadvertently reinforce existing biases, hindering the discovery of diverse perspectives. Consequently, researchers must remain vigilant about how these mechanisms can distort the representation of information, inviting a critical examination of algorithms to achieve more reliable insights in social media research. By recognizing these effects, researchers can better navigate the complexities introduced by social media algorithms.
How Algorithms Curate Content
Social media algorithms play a pivotal role in curating content viewed by users. By analyzing user behavior, preferences, and engagement levels, these algorithms prioritize posts that align with users’ patterns. This selective exposure often leads to a narrow view of topics, limiting diversity in content interaction. Consequently, the impact of algorithmic bias effects emerges as specific narratives gain traction while others remain marginalized.
The implications of these curated experiences can profoundly influence research findings. Researchers relying on social media as a data source may encounter skewed perceptions, inadvertently shaping their insights based on popularized content rather than a holistic representation of opinions. Recognizing this dynamic is crucial for researchers aiming to produce valid, reliable outcomes. By understanding how algorithms curate content, researchers can adopt more comprehensive strategies, ensuring their findings reflect a wider spectrum of perspectives rather than those filtered through biased algorithms.
The Role of Engagement Metrics in Shaping Research Perspectives
Engagement metrics significantly shape research perspectives by influencing researchers' access to information and the narrative they construct. These metrics, which measure user interactions like likes, shares, and comments, can skew visibility towards certain viewpoints or data while sidelining others. As a result, researchers might draw conclusions based on biased sources that prioritize popularity over accuracy, leading to distorted findings in the analysis of discussions around topics.
Furthermore, the reliance on these metrics complicates the integrity of social media research. It can foster algorithmic bias effects, where engagement becomes synonymous with relevance. Researchers must be aware that their work can perpetuate these biases if they do not critically evaluate the sources driving engagement metrics. By understanding how social media algorithms operate, researchers can better navigate these complexities and aim for more balanced, nuanced perspectives in their findings.
Algorithmic Bias Effects on Research Outcomes
Algorithmic Bias Effects on Research Outcomes are increasingly significant in today's digital landscape. As researchers rely more on social media data, the inherent biases in algorithms can alter findings fundamentally. This can lead to misleading conclusions based on skewed perspectives that manifest through content curation and engagement metrics. Furthermore, these algorithms often favor popular content, obscuring niche or critical viewpoints that may be vital for comprehensive research.
Moreover, cognitive biases, such as confirmation bias, can emerge when researchers engage with algorithmically curated content. This effect reinforces existing hypotheses and diminishes the opportunity for innovative thinking. Such patterns can prevent researchers from considering diverse data and alternative viewpoints, thereby limiting the depth and breadth of their inquiries. Understanding these biases is crucial for academics, businesses, and policymakers aiming to derive accurate insights from social media platforms. Addressing these challenges ensures more reliable research outcomes while promoting a thorough understanding of societal trends shaped by algorithmic influence.
Cognitive Bias: Skewing Researchers Perceptions
In research influenced by social media algorithms, cognitive bias significantly skews researchers’ perceptions. Cognitive bias occurs when preconceived notions or preferences distort judgment and interpretation. This can lead researchers to pay undue attention to data that aligns with their beliefs while neglecting contradicting information. The effects of algorithmic bias exacerbate this issue, as algorithms often amplify certain viewpoints and data, shaping the research narrative.
Moreover, researchers may unconsciously favor data that they encounter more frequently on social media platforms, creating an echo chamber effect. This can further skew their understanding and lead to flawed conclusions. To counteract cognitive biases, it is crucial for researchers to actively seek diverse data sources and remain aware of their preconceived notions. Implementing robust methodologies that incorporate a variety of perspectives can help mitigate these biases, fostering a more reliable research environment.
Extract insights from interviews, calls, surveys and reviews for insights in minutes
Confirmation Bias: Reinforcing Existing Hypotheses
Confirmation bias occurs when researchers favor information that supports their pre-existing beliefs or hypotheses. This cognitive bias can be particularly pronounced in settings where algorithmic bias effects are present, such as social media platforms. The algorithms that curate content often amplify material that aligns with users' previous interactions, effectively narrowing their exposure to diverse viewpoints.
As researchers engage with social media data, they may unknowingly reinforce their biases by focusing on content that affirms their hypotheses. This can lead to skewed research findings, impacting the validity of conclusions drawn from their analysis. To counteract this, it is essential for researchers to adopt strategies that promote objectivity, such as diversifying data sources and being mindful of the potential influence of algorithmic bias effects. By recognizing and addressing confirmation bias, researchers can enhance the reliability of their findings and contribute to a more nuanced understanding of the data they analyze.
Tools and Strategies for Mitigating Algorithmic Bias Effects
To address the challenges posed by algorithmic bias effects, researchers must employ strategic tools and reliable methodologies. First, identifying potential sources of bias is crucial. This involves examining the algorithms themselves while acknowledging their inherent limitations. Once these biases are uncovered, researchers can better understand how they affect their findings.
Second, diversifying data sampling methods can significantly reduce algorithmic bias effects. Utilizing a variety of social media platforms ensures exposure to a broader range of perspectives and insights. This broadens the dataset and provides a more balanced view, which is vital to achieving accurate research outcomes.
Lastly, employing cross-platform analyses allows researchers to compare data from different sources. This strategy not only highlights inconsistencies but also provides a more holistic view of social media interactions. Together, these steps form a robust framework for mitigating algorithmic bias effects, fostering more trustworthy and insightful research findings.
Top Tools for Analyzing Social Media Data
Analyzing social media data requires the right tools to gain meaningful insights and mitigate potential distortions resulting from algorithmic bias effects. The landscape has several powerful tools that can enhance understanding of patterns and sentiments across user interactions. Each tool offers unique features tailored to address different research needs.
One notable option is Brandwatch, which excels in social listening and competitive analysis. Its advanced analytics help researchers decode conversations for a clearer view of public sentiment. Hootsuite provides a user-friendly dashboard for monitoring multiple platforms, making it easy to track engagement levels and narrative trends. Meanwhile, TweetBinder can offer valuable insights specifically for Twitter data, focusing on tracking hashtags and user interactions effectively. Sprout Social stands out with its emphasis on collaboration, enabling teams to analyze data collectively. Finally, tools like insight7 offer a comprehensive suite for detailed analytics, ensuring that researchers can interpret trends while remaining aware of potential biases in the dataset. These tools collectively empower researchers to navigate the complexities of social media algorithms and enhance the reliability of their findings.
- insight7
Algorithmic Bias Effects can significantly influence research outcomes, particularly in the context of social media. Researchers often find themselves navigating a landscape shaped by algorithms that prioritize specific content. This curation can inadvertently lead to skewed perceptions and misinterpretations of findings, amplifying certain viewpoints while silencing others.
💬 Questions about The Impact of Social Media Algorithms on Research Findings?
Our team typically responds within minutes
To address these challenges, researchers should consider several strategies. First, identifying potential bias sources is crucial; understanding how algorithms operate allows for greater awareness of their limitations. Second, diversifying data sampling methods helps ensure a more comprehensive view of the user experience, mitigating narrow perspectives. Finally, employing cross-platform analyses can reveal inconsistencies in data, providing a fuller understanding of the implications of algorithmic bias. By implementing these steps, researchers can enhance the reliability and accuracy of their findings in a rapidly evolving digital landscape.
- Brandwatch
Brandwatch provides an essential framework for understanding social media dynamics and their impact on research findings. In a landscape increasingly shaped by algorithms, the study of algorithmic bias effects is crucial. By tracking social interactions and content patterns, it reveals how algorithms can favor certain narratives while suppressing others. This selective visibility can significantly distort research outcomes, misleading marketers and researchers alike.
Understanding these dynamics allows for more informed decision-making. Utilizing such analytical tools enables researchers to identify biases entrenched in data collection and interpretation. By doing so, they can address the cognitive and confirmation biases that often skew perceptions. Researchers can enhance their methodologies by diversifying data sampling methods and employing cross-platform analyses, which ultimately leads to more accurate and reliable insights. Addressing algorithmic bias effects in social media research is vital for creating balanced perspectives and driving effective marketing strategies.
- Hootsuite
In the context of understanding algorithmic bias effects on social media research findings, tools that assist in monitoring and analyzing social media platforms play a critical role. These tools offer researchers crucial insights on how content is shaped by algorithms. By identifying patterns in user engagement, researchers can better understand the impact of algorithmic biases on their findings.
One such tool enables users to efficiently gather data from various social media channels. Researchers can customize their data collection strategies, which helps mitigate biases inherent in social media algorithms. This ability to tailor data collection is essential when analyzing diverse populations and perspectives. It ultimately aids in developing a more comprehensive understanding of research outcomes influenced by social media. By utilizing these tools, researchers can address algorithmic biases more effectively, ensuring their findings reflect a broader range of insights and experiences.
- TweetBinder
In today's social media-driven world, tools that facilitate the analysis of online data have become indispensable. One such tool helps researchers distill insights from vast amounts of social media activity. This platform simplifies the process, enabling users to visualize interactions and extract key insights that can inform research decisions. By making access to data user-friendly, it empowers researchers to focus on the significance of their findings rather than the complexities of data analysis.
The challenge lies in understanding the algorithmic bias effects that can skew these insights. Algorithms determine the content visibility, which can inadvertently favor certain perspectives over others. When researchers rely solely on these inputs, they must consider the resultant biases. Recognizing this, researchers need to implement strategies that ensure a balanced view. By actively addressing potential biases, the integrity of research findings can be upheld, fostering a more accurate understanding of social media dynamics.
- Sprout Social
When examining the impact of social media algorithms on research findings, understanding their implications is crucial. Algorithmic bias effects shape what data researchers encounter and ultimately influence their conclusions. Some platforms excel at data analysis and provide insights into customer behavior, allowing for a better understanding of prevailing trends.
To navigate algorithmic bias effects effectively, several strategies can be employed. First, it’s vital to identify potential sources of bias within the data collection process. Recognizing how algorithms prioritize specific types of content can lead to a more comprehensive view of consumer sentiment. Next, diversifying data sampling methods ensures that varied perspectives are captured, reducing skewed insights. Lastly, employing cross-platform analyses can help draw more accurate conclusions by providing a fuller picture of social media interactions. By implementing these steps, researchers can minimize the distortion caused by algorithms and create more reliable findings.
Steps to Implement Bias-Reduction Strategies
To effectively implement bias-reduction strategies, it is imperative to begin by identifying potential sources of bias within the data. This entails scrutinizing the data collection process to recognize where algorithmic bias could manifest, particularly focusing on skewed demographics or limited viewpoints. Understanding the origins of bias is the first step toward developing more objective research directives.
Next, diversifying data sampling methods is crucial for gathering a wide range of perspectives. By implementing varied sampling techniques, researchers can counteract the narrow focus caused by algorithms, ensuring that their findings are representative of the broader audience. Following this, employing cross-platform analyses allows researchers to validate their findings against multiple sources of social media data, further reducing the risks associated with algorithmic bias effects.
By adhering to these steps, researchers can foster a more holistic understanding of social media's impact on research findings, ultimately leading to more reliable and diverse conclusions.
- Step 1: Identify Potential Bias Sources
Identifying potential sources of bias is essential for achieving reliable research findings. It is particularly crucial in the context of social media algorithms, which can inadvertently skew perceptions and outcomes. Start by examining algorithmic influences that shape the content researchers encounter. These algorithms prioritize certain data based on user interactions, which may not represent a comprehensive view. For instance, if researchers consistently engage with specific topics, the algorithms will promote similar content, leading to a narrow perspective that lacks diversity.
Next, consider the broader implications of algorithmic bias effects on research. It’s vital to critically assess data sampling, as algorithms often favor popular or engaging posts, potentially sidelining less popular yet significant content. Thus, leading researchers may draw skewed conclusions based on limited information. By carefully identifying these potential sources of bias, researchers can take deliberate steps to ensure more balanced, accurate findings in their studies.
- Step 2: Diversify Data Sampling Methods
To effectively mitigate the impact of algorithmic bias effects, diversifying data sampling methods is crucial. By employing varied sampling techniques, researchers can gather a more comprehensive view of audience behaviors and sentiments across social media platforms. This approach helps ensure that data reflects diverse voices and experiences, addressing the skew that may arise when relying on a single source or method.
Key strategies for diversifying data sampling include random sampling, stratified sampling, and snowball sampling. Random sampling involves selecting participants randomly, providing a representative cross-section of the population. Stratified sampling focuses on specific subgroups within a population to ensure all demographics are represented. Snowball sampling allows researchers to tap into existing social networks to reach individuals who might otherwise remain overlooked. These methods collectively foster a richer understanding of diverse perspectives, lessening the likelihood of overlooking critical insights that could significantly influence research outcomes.
- Step 3: Employ Cross-Platform Analyses
Employing cross-platform analyses is a crucial step in mitigating algorithmic bias effects in social media research. Researchers should first gather data from diverse social media platforms to capture a wide range of user interactions and opinions. This approach provides a more comprehensive dataset, reducing the likelihood of being influenced by any single platform’s unique algorithmic bias.
Next, it’s essential to compare findings across these platforms. Each social media network operates on distinct algorithms, which can lead to varying representations of information. By conducting analyses on multiple platforms, researchers can identify and account for discrepancies in data outcomes. This step enhances the validity of research findings, allowing for a clearer understanding of the broader social media landscape. Ultimately, cross-platform analyses not only enrich research quality but also promote a fairer evaluation of algorithmic bias effects, leading to insights that are more representative and impactful.
Conclusion: Navigating Algorithmic Bias Effects for Accurate Research Findings
To effectively navigate algorithmic bias effects, researchers must remain vigilant about the influences of social media algorithms on their findings. Social media platforms prioritize content through algorithms, which can skew the data available for analysis. Such bias may lead to distorted perceptions and ultimately affect the validity of research conclusions.
Researchers should adopt strategies that include diversifying data sources and employing cross-platform analyses to mitigate these effects. By critically examining how algorithms curate information, researchers can enhance their understanding and ensure more accurate findings. This awareness is crucial for generating reliable and meaningful insights that reflect the true sentiments of users in the digital ecosystem.
💬 Questions about The Impact of Social Media Algorithms on Research Findings?
Our team typically responds within minutes