In today's data-driven world, businesses are inundated with vast amounts of textual information. As organizations strive to derive actionable outcomes from this data, understanding the nuances between text mining and text analytics becomes crucial. Both methodologies play unique roles in extracting insights, yet they cater to different analytical needs and objectives.
Text insights comparison serves as a critical exploration for professionals aiming to harness the potential of unstructured data. While text mining focuses on discovering patterns and relationships within the data, text analytics emphasizes interpreting and transforming these findings into strategic decisions. Gaining clarity in these distinctions not only enhances analytical capabilities but also drives successful outcomes in various industries.
Generate visualizations from your qualitative data. At Scale.
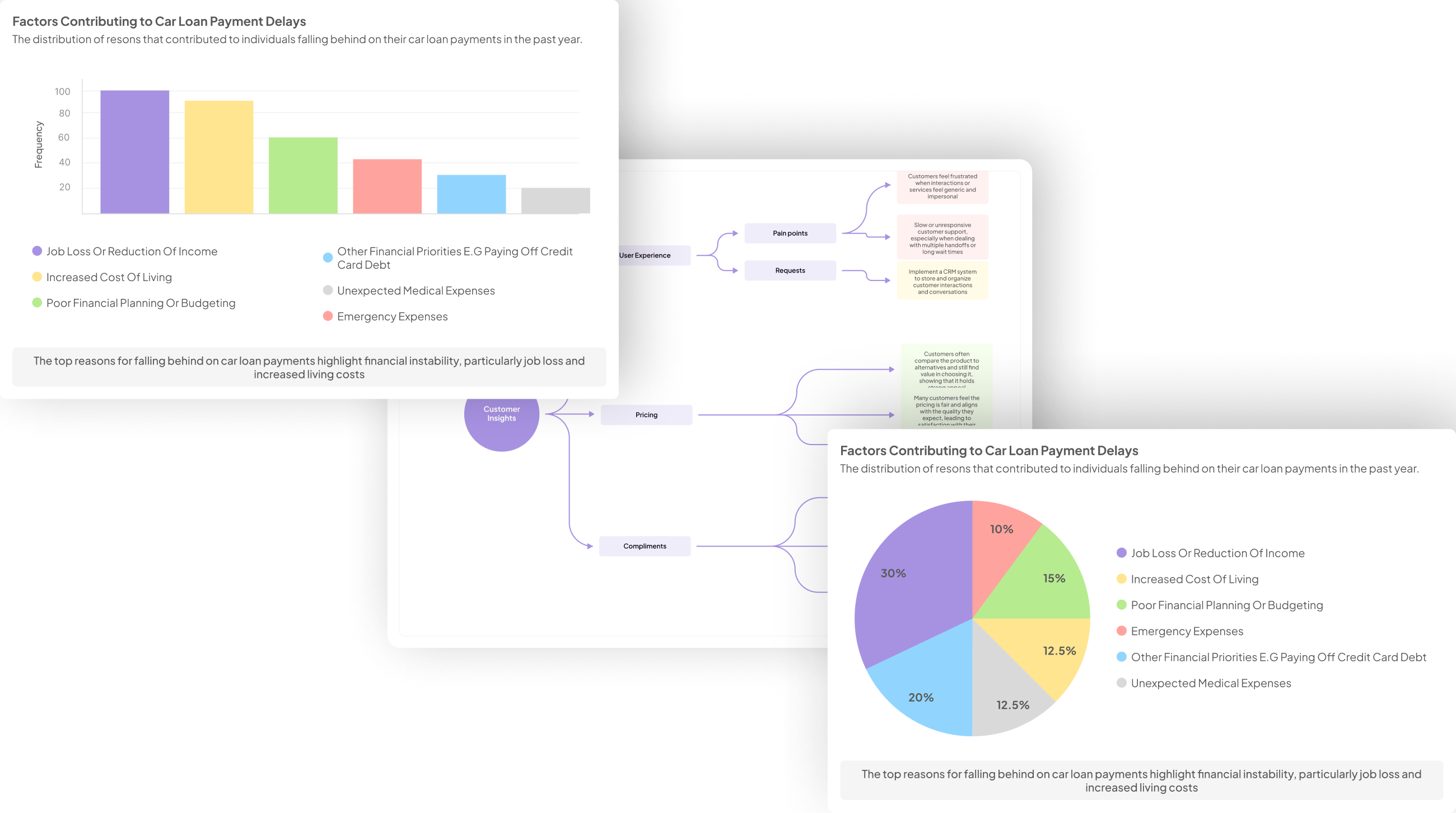
The Foundations of Text Insights Comparison: Text Mining and Text Analytics
Text insights comparison between text mining and text analytics highlights essential distinctions that frame their unique contributions to data analysis. Text mining focuses on the extraction of valuable information from unstructured data sources, such as social media, emails, and call transcripts. Through advanced algorithms, it helps identify patterns, trends, and sentiments to transform raw text into actionable insights.
On the other hand, text analytics emphasizes the interpretation of this extracted data. It involves the application of various analytical techniques to understand context, relationships, and user behavior. Text analytics serves to connect qualitative insights with quantitative data, enabling organizations to make informed decisions based on comprehensive understanding.
Both methodologies complement each other while offering distinct advantages. Text mining uncovers hidden insights, while text analytics provides the context needed for effective decision-making. Understanding the foundations of these two disciplines allows organizations to harness their full potential in the ever-evolving data landscape.
The Core Process of Text Mining
The process of text mining begins with collecting and preparing the data, often from unstructured sources like documents and audio transcripts. This initial step is crucial as it sets the foundation for extracting meaningful insights. For example, transcribing recorded conversations can reveal customer sentiments and pain points that might be buried in the raw data. It is important to ensure that these transcripts are accurately generated, as they serve as the primary resource for analysis.
Once the data is prepared, the next phase is to analyze these transcripts to extract valuable information. Different tools allow users to pull specific insights from the data, such as identifying common themes or trends. For instance, summarizing key points from a series of calls can help highlight customer needs and preferences. This systematic approach enables organizations to gain critical insights from large volumes of text, showcasing the power of text mining in driving data-informed decision-making.
The Framework of Text Analytics
The framework of text analytics serves as a structured approach to derive meaningful insights from vast amounts of text data. This process begins with data collection, where various text sources, such as reviews or social media posts, are gathered. Once data is compiled, it undergoes preprocessing, which includes text cleaning and normalization. This crucial step eliminates noise and prepares data for analysis.
Following preprocessing, the analytic techniques are applied, allowing businesses to uncover patterns and trends within the text. Common methods include sentiment analysis and topic modeling, which help organizations understand customer sentiments and prevalent themes. Lastly, the insights generated are translated into actionable strategies, enabling companies to respond effectively to customer needs. This structured progression illustrates how the framework of text analytics enhances decision-making and fosters competitive advantage in today's data-driven world.
Generate Journey maps, Mind maps, Bar charts and more from your data in Minutes
Text Insights Comparison: Key Differences and Overlaps
Understanding the Text Insights Comparison reveals both distinct features and shared traits between text mining and text analytics. Text mining focuses on extracting valuable insights from unstructured data sources, such as social media posts and customer reviews. It identifies trends and patterns, enabling businesses to make informed decisions. On the other hand, text analytics primarily aims to analyze the extracted insights, generating meaningful interpretations and visual representations that inform strategy.
Despite their differences, there are overlaps in objectives and tools between the two fields. Both utilize natural language processing (NLP) to interpret textual data and employ similar algorithms for data analysis. Additionally, they share a common goal of enhancing customer experience through insights, improving products, or tailoring marketing strategies. Ultimately, recognizing these key differences and overlaps helps organizations utilize both approaches effectively for better-informed decision-making.
Distinguishing Features of Text Mining
The distinguishing features of text mining highlight its unique capabilities compared to text analytics. Text mining primarily focuses on extracting valuable insights from large volumes of unstructured text data. This process often involves various techniques such as natural language processing (NLP), machine learning, and statistical analysis. By identifying patterns and trends, text mining provides a way to turn raw text into actionable information.
In contrast, text analytics often aims at interpreting the extracted insights to support decision-making. While both areas deal with text data, text mining is about discovering hidden information, whereas text analytics is about contextualizing and applying that information to drive business strategies. Understanding these distinctions fosters a more comprehensive view of text insights comparison and clarifies how organizations can leverage both approaches effectively to gain a competitive edge in their respective fields.
Unique Characteristics of Text Analytics
Text analytics is characterized by its focus on deriving actionable insights from unstructured data. Unlike text mining, which primarily concentrates on extracting raw information, text analytics emphasizes understanding context, sentiment, and relationships within the data. This process enables organizations to interpret customer feedback, social media interactions, and other forms of textual data more meaningfully.
One unique characteristic of text analytics is its ability to harness advanced techniques such as natural language processing (NLP) and machine learning. These technologies allow for more nuanced analysis, thus providing insights that are not only descriptive but also predictive. Furthermore, text analytics often incorporates visualizations and reporting tools, which can help decision-makers quickly grasp complex data patterns. Ultimately, the goal is to transform data into knowledge that drives strategic actions, facilitating a proactive approach to business challenges.
Conclusion: Final Thoughts on Text Insights Comparison
In evaluating the nuances of text insights comparison, it's clear that both text mining and text analytics offer unique advantages. Text mining focuses on extracting patterns and knowledge from vast datasets, while text analytics emphasizes interpreting and contextualizing that information for practical application. Understanding these distinctions allows businesses to harness the full potential of data-driven decision-making effectively.
Ultimately, the choice between text mining and text analytics depends on specific organizational needs. Companies can create strategies that align with their goals by integrating elements from both disciplines. Businesses can maximize insights, improve efficiency, and drive innovation when they appreciate the value of each approach in the text insights comparison.