Data Insight Extraction is a vital component of text mining, enabling organizations to derive meaningful conclusions from unstructured data sources. In a world overflowing with information, understanding and utilizing this process becomes essential for making informed decisions. Integrating Data Insight Extraction into analytics empowers businesses to recognize patterns, identify trends, and ultimately enhance strategic initiatives.
The importance of Data Insight Extraction cannot be overstated; it transforms raw text into actionable intelligence. Through advanced analytical techniques, organizations can uncover insights relevant to customer satisfaction, operational efficiency, and market dynamics. By effectively harnessing text mining, businesses not only stay competitive but also drive innovation and growth in their respective industries.
Generate visualizations from your qualitative data. At Scale.
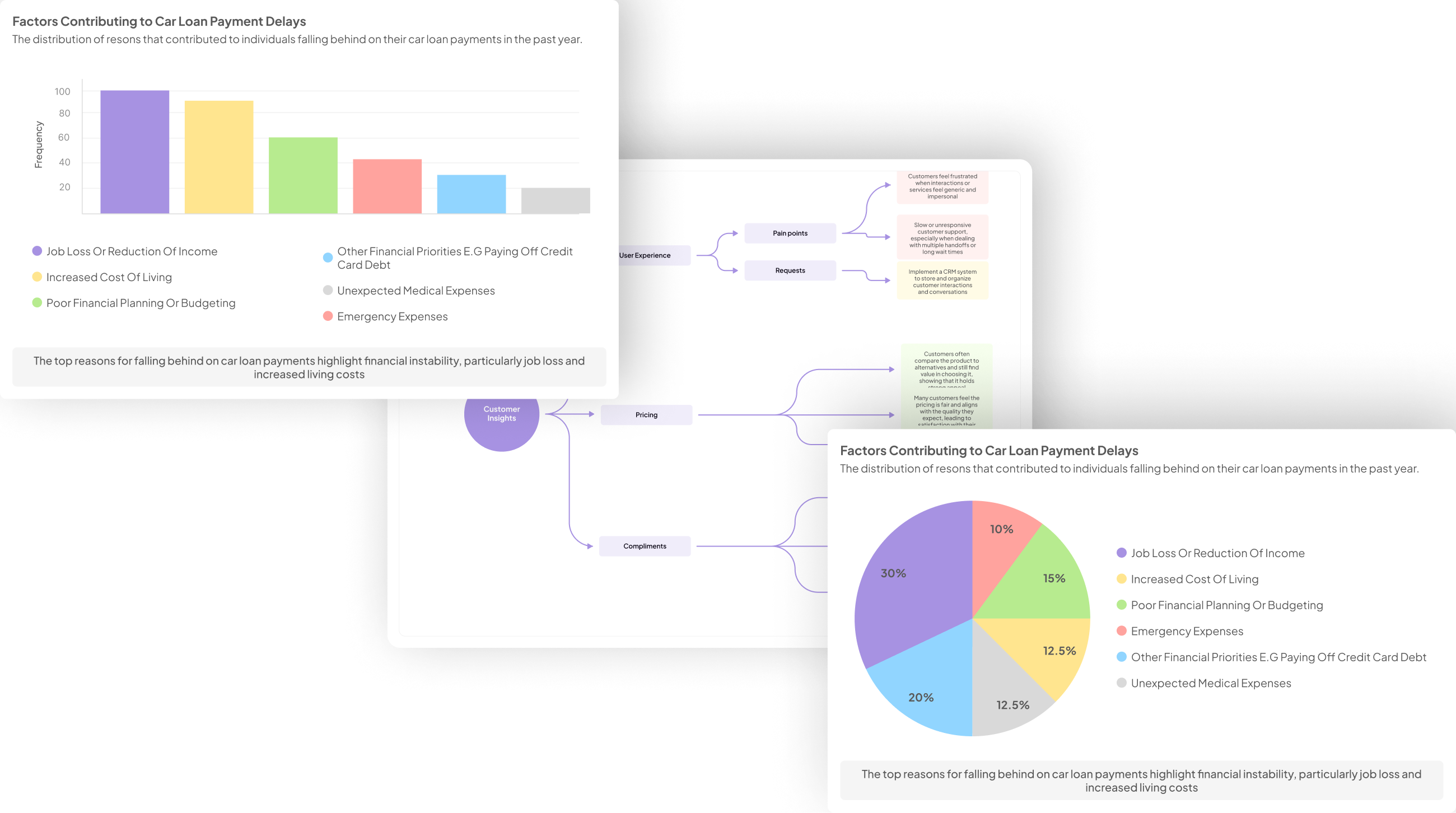
The Synergy between Text Mining and Data Insight Extraction
Text mining and data insight extraction work together to reveal actionable intelligence from unstructured data sources. This collaboration transforms raw text into meaningful insights, enabling organizations to make informed decisions. By applying natural language processing (NLP) techniques, text mining extracts essential patterns and trends from a wealth of textual information.
Delving deeper, data insight extraction enhances these findings by interpreting and analyzing the content. This process not only identifies significant themes but also connects the dots between various data points. As a result, organizations gain a comprehensive view that highlights customer sentiments and market trends. This synergy ensures that companies are not merely gathering data but are also adept at deriving insights that can drive strategic initiatives. Such sophistication in approach can lead to improved customer engagement and operational efficiency.
Understanding Text Mining
Text mining is a transformative process that allows organizations to extract valuable insights from vast amounts of unstructured data. It serves as a bridge between raw data and actionable knowledge. By employing natural language processing techniques, text mining can identify patterns, trends, and insights embedded within the data. This ability to sift through information leads not only to better decision-making but also supports deeper understanding of customer sentiments and behaviors.
At the core of text mining lies the principle of data insight extraction, which focuses on turning complex data sets into understandable, actionable conclusions. This extraction process typically involves various stages, including data collection, preprocessing, and structured analysis. Each stage plays a crucial role in refining the data, enhancing the quality of insights derived, and ultimately guiding strategies that align with consumer needs. Understanding text mining is not just about technical execution; it’s about recognizing its power to illuminate pathways to success in any data-driven environment.
The Role of Data Insight Extraction in Text Analytics
Data Insight Extraction plays a crucial role in text analytics by transforming raw textual information into understandable and actionable insights. This process involves examining text data to uncover patterns, trends, and sentiments. As businesses strive to enhance their decision-making, extracting meaningful insights from text becomes increasingly vital.
The first step in Data Insight Extraction is collecting data from various sources, such as customer feedback, social media, and interviews. Next, preprocessing the data ensures that it is clean and ready for analysis, eliminating noise that can obscure valuable information. Finally, analyzing the curated data reveals key insights, which can guide businesses in refining strategies, addressing customer needs, and improving overall user experiences. Ultimately, this synergy between Data Insight Extraction and text analytics enables organizations to harness the wealth of information contained in text, driving better outcomes across all facets of their operations.
Generate Journey maps, Mind maps, Bar charts and more from your data in Minutes
Practical Applications and Techniques in Data Insight Extraction
In the realm of data insight extraction, practical applications are pivotal for transforming raw data into actionable insights. Organizations can harness the power of text mining to systematically analyze customer interactions, such as call transcripts and feedback forms. For instance, by compiling data from various sources, businesses can identify common pain points or frequently mentioned desires, thereby gaining a better understanding of customer needs.
To effectively implement data insight extraction, organizations can follow a structured process. First, data collection involves gathering information from diverse media, such as surveys, interviews, and online interactions. Secondly, preprocessing ensures the data is cleaned and organized for meaningful analysis. Finally, the analysis phase employs various techniques to extract valuable insights, revealing trends and patterns that can inform strategic decisions. Using these approaches, companies can cultivate a comprehensive understanding of their audience and enhance their overall performance.
Steps to Implementing an Effective Data Insight Extraction Process
Implementing an effective data insight extraction process requires a systematic approach to transform raw data into meaningful insights. The first step is data collection, where relevant datasets are gathered from various sources like customer feedback, surveys, and social media interactions. This phase sets the foundation for successful analysis, ensuring the data is comprehensive and relevant.
Next, preprocessing is essential for data insight extraction. This involves cleaning the data by removing inconsistencies and irrelevant information, ensuring that only significant information is retained for analysis. Finally, analyzing the prepared data allows for uncovering patterns and trends. By applying techniques such as text mining and natural language processing, organizations can extract actionable insights that drive decision-making. Establishing these steps enhances the ability to derive valuable information from data, ultimately improving strategies and outcomes.
- Step 1: Data Collection
Data collection is an essential first step in the process of data insight extraction. This stage involves gathering relevant information from various sources, ensuring that the dataset is comprehensive and representative. Utilizing diverse data sources, such as surveys, social media, and customer feedback, helps create a rich data pool for subsequent analysis. In this initial phase, attention should be paid to data quality, as inaccurate or irrelevant information can significantly impact the outcomes of later stages.
Once the data is collected, it sets the foundation for further analysis. The goal is to convert raw data into meaningful insights, guiding decision-making processes. It's crucial to organize and store the collected data systematically, facilitating easier access and analysis later on. Proper data collection not only enhances the reliability of insights derived but also empowers organizations to make informed decisions based on the extracted data insights.
- Step 2: Preprocessing for Data Insight Extraction
Preprocessing for Data Insight Extraction involves cleansing and organizing raw data to unveil meaningful insights. A crucial initial step, this process ensures that the most relevant information is emphasized while reducing noise. During preprocessing, inconsistencies and redundancies in text data are identified and corrected, allowing for a clearer analysis. Additionally, techniques such as tokenization, stemming, and removing stop words help to distill text into manageable units suitable for further examination.
Moreover, effective preprocessing sets the foundation for deeper analytics and ultimately enhances the data insight extraction process. By transforming unstructured data into a structured format, researchers can employ algorithms and analytical tools to identify patterns and trends. This approach not only streamlines the workflow but also improves the accuracy of insights gathered from text mining, making preprocessing an indispensable step in harnessing the full potential of data-driven decision-making.
- Step 3: Analyzing Data for Meaningful Insights
Analyzing data for meaningful insights is a crucial step in the text mining and analytics process. This stage focuses on extracting valuable information from vast amounts of textual data, transforming it into a format that can inform decision-making. By applying various analytical tools and methods, organizations can identify trends, sentiment, and patterns present within the data, enabling them to derive actionable insights.
To begin the analysis, it is essential to establish clear objectives for what the analysis aims to uncover. This could range from understanding customer feedback to identifying gaps in the market. Next, employing techniques like sentiment analysis and entity recognition allows you to categorize and interpret the data effectively. Finally, reviewing these findings in context helps to ensure that the insights are meaningful, relevant, and can directly support strategic goals. By mastering this step, businesses can turn data into a powerful ally for growth and innovation.
Case Studies: Successful Use of Text Mining and Data Insight Extraction
Companies increasingly utilize text mining and data insight extraction to transform raw data into actionable insights. For instance, a client leveraged text mining to analyze customer feedback across multiple communication channels. The insights extracted revealed common pain points and areas for improvement, enabling the team to craft targeted marketing strategies. In this case, the process unlocked valuable customer sentiment, aligning products more closely with user expectations.
Another case involved the analysis of internal communication data, with an aim to enhance team collaboration. By applying data insight extraction techniques, the organization uncovered themes and patterns that highlighted communication gaps. Addressing these issues led to improved interdepartmental interactions and overall productivity. These examples illustrate how text mining, combined with effective data insight extraction, can illuminate important trends and drive strategic decisions that benefit organizations.
Conclusion: Unlocking the True Potential of Text Mining and Data Insight Extraction
The journey towards unlocking the true potential of text mining and data insight extraction opens vast opportunities for organizations. By effectively applying these techniques, businesses can transform raw data into valuable insights, guiding decision-making and strategies. Understanding patterns, trends, and sentiments allows organizations to address customer needs more directly and innovatively.
Data insight extraction not only streamlines processes but also democratizes access to critical information across teams. As various stakeholders harness these insights, they become empowered to drive meaningful change. In a world inundated with data, prioritizing effective text mining strategies will ultimately position organizations for sustainable growth and success.