Linguistic Comparison Analysis serves as a bridge between text analytics and text mining, facilitating a deeper understanding of both domains. Through this analysis, one can extract meaningful insights from textual data, highlighting the distinct characteristics and methodologies each field employs.
When exploring text analytics, focus centers on the role of semantics and syntax to derive insights. Techniques such as sentiment analysis and keyword identification enable the extraction of relevant patterns. In contrast, text mining delves into structured processes that draw upon algorithms and statistical methods to uncover hidden relationships within the data.
These two approaches, while interconnected, serve various objectives and methodologies. Linguistic Comparison Analysis ultimately helps decipher the nuances that differentiate text analytics from text mining, providing clarity for businesses aiming to utilize these techniques effectively. Understanding these distinctions equips practitioners with the tools to better harness text data for strategic decision-making.
Introduction to Linguistic Comparison Analysis in Text Analytics and Text Mining
Linguistic Comparison Analysis serves as a crucial tool in enhancing our understanding of both text analytics and text mining. As we delve into these two realms, we find that both methodologies aim to extract valuable insights from vast amounts of textual data. However, they approach this goal through different strategies. Text analytics emphasizes the extraction of meaning, while text mining focuses on the discovery of patterns and relationships within the data.
By employing Linguistic Comparison Analysis, practitioners can better discern the nuances that differentiate text analytics from text mining. This analysis helps to reveal how language is utilized differently in each approach, thereby facilitating improved decision-making. In an era where data-driven strategies are paramount, understanding these distinctions not only enhances comprehension but also empowers organizations to utilize these techniques effectively for their goals.
Generate visualizations from your qualitative data. At Scale.
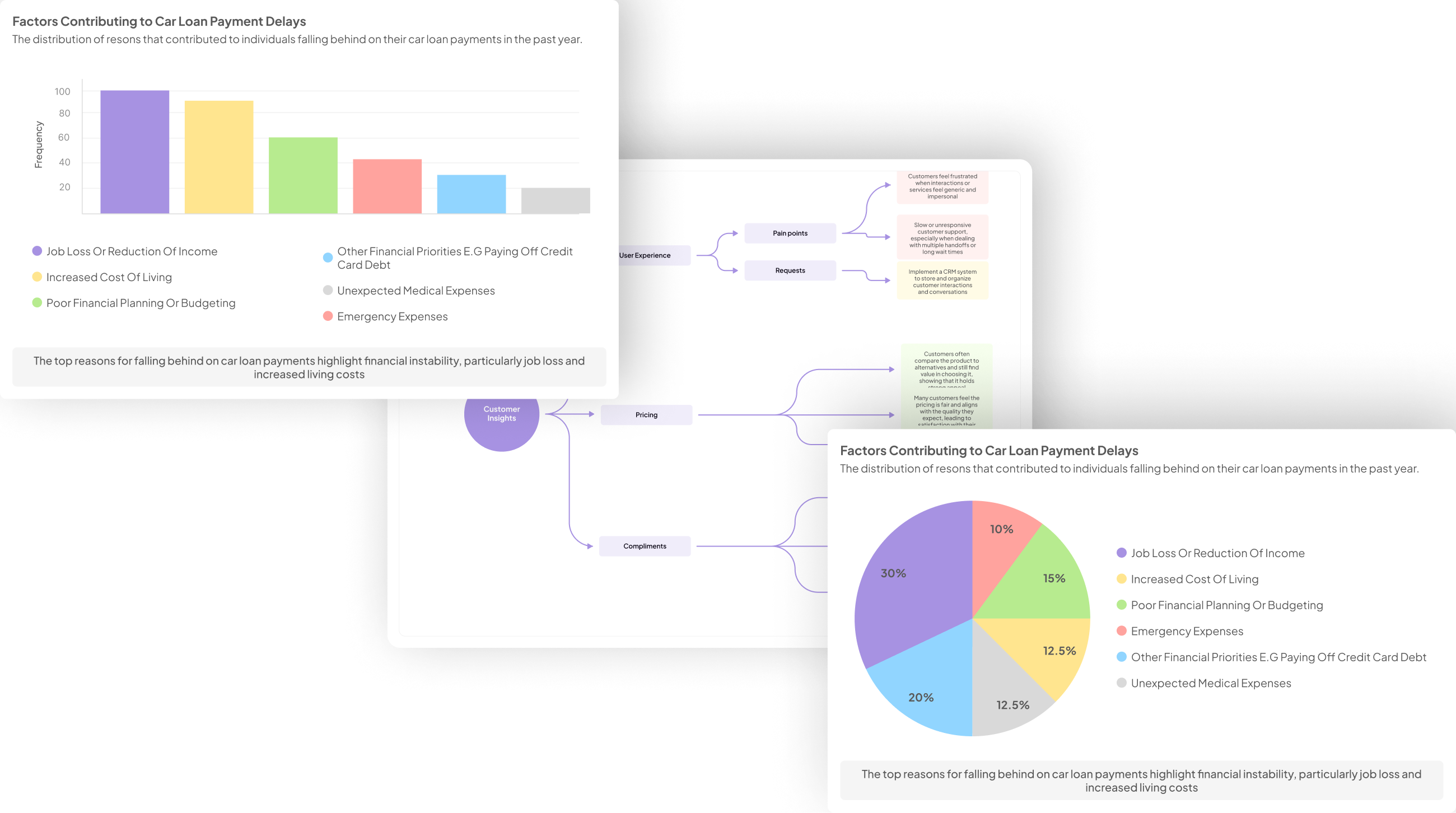
Linguistic Comparison Analysis: Understanding Text Analytics
Linguistic Comparison Analysis focuses on interpreting and deriving meaning from text data through various analytical techniques. By examining language patterns, sentiment, and contextual nuances, this analysis plays a pivotal role in text analytics. The process involves assessing how words interact, the emotional undercurrents they carry, and the overall intent behind written content. This understanding aids businesses in gauging customer feedback, preferences, and trends efficiently.
In text analytics, techniques such as natural language processing and machine learning are often employed to automate data extraction. These methods enhance the accuracy and speed of analysis, thereby facilitating decision-making processes. By utilizing linguistic comparison tools, organizations can transform unstructured text into structured insights. This transformation not only drives strategic initiatives but also optimizes customer experience. Therefore, mastering linguistic comparison proves essential for anyone aiming to leverage text analytics effectively.
Role of Text Analytics in Extracting Meaning
Text analytics plays a vital role in extracting meaning from vast amounts of unstructured data. By employing techniques such as sentiment analysis, keyword extraction, and thematic analysis, organizations can derive actionable insights from text. These processes enable businesses to comprehend customer feedback, market trends, and competitive dynamics, thereby facilitating informed decision-making.
In the realm of linguistic comparison analysis, this becomes even more pronounced. It allows a detailed evaluation of linguistic patterns, enabling researchers to not only map the emotional tone of text but also identify underlying themes and opinions. Consequently, businesses can tailor their strategies based on nuanced understanding rather than surface-level interpretations. Thus, text analytics becomes an indispensable tool for extracting meaningful insights, significantly enhancing strategic initiatives across various sectors.
Techniques in Linguistic Comparison Analysis for Text Analytics
Linguistic Comparison Analysis encompasses various techniques that prove beneficial for text analytics. To effectively analyze language and discern meaning, several core methods are employed. These methods include tokenization, which breaks text into manageable units, and part-of-speech tagging to identify grammatical components within sentences. Such techniques allow analysts to understand the structural and semantic elements of language, making it easier to derive insights from customer interactions.
Another significant technique is sentiment analysis, which gauges the emotional tone behind a body of text. By identifying whether the language used is positive, negative, or neutral, businesses can better tailor their strategies. Additionally, named entity recognition helps in extracting relevant information by identifying specific names, dates, and locations. These tools facilitate a comprehensive understanding of text, enabling organizations to translate insights into actionable strategies. By emphasizing techniques in Linguistic Comparison Analysis, companies can effectively harness text analytics for enhanced decision-making and customer engagement.
Generate Journey maps, Mind maps, Bar charts and more from your data in Minutes
Linguistic Comparison Analysis: Exploring Text Mining
Linguistic Comparison Analysis in text mining involves examining and interpreting language patterns within data to yield actionable insights. This analysis is crucial for transforming unstructured data into structured, meaningful information that can inform decisions. Text mining utilizes various techniques, such as natural language processing and sentiment analysis, to explore and uncover hidden patterns in communication.
When conducting a Linguistic Comparison Analysis, there are key processes to consider. First, data collection is essential; gathering diverse text sources enhances the quality of insights. Next, data preprocessing involves cleaning and filtering this data to ensure accuracy. Finally, applying algorithms and models allows for in-depth analysis, revealing trends and sentiments that can guide strategic planning. Emphasizing these processes enables organizations to leverage useful insights from their text data, ultimately enhancing their decision-making capabilities.
Core Text Mining Processes
Core text mining processes focus on extracting valuable insights from vast amounts of textual data. A fundamental stage involves data preprocessing, which includes activities like tokenization, stemming, and removing stop words. These techniques help clean and prepare the text for deeper analysis, ensuring that the data is structured correctly for mining. Once the data is preprocessed, various algorithms can be applied to discover patterns and relationships between the words and themes.
Another critical component is linguistic comparison analysis. This involves evaluating the differences and similarities within datasets to determine sentiment, intent, and context. Furthermore, by employing machine learning algorithms, analysts can uncover hidden trends and generate predictive insights, which are highly beneficial for businesses. Overall, these processes work together to transform unstructured text into actionable information that can drive decision-making and enhance overall understanding.
Applications of Linguistic Comparison Analysis in Text Mining
Linguistic Comparison Analysis plays a vital role in extracting deeper insights from text mining. By systematically comparing linguistic features across different text sources, analysts can identify patterns, trends, and variability in language use. For instance, this method can be applied to customer reviews, revealing sentiments that shape public perception of a brand or product.
Moreover, applications of Linguistic Comparison Analysis extend to competitive analysis and market research. By analyzing the language styles and terminologies utilized by competitors, businesses can adapt their messaging strategies effectively. Furthermore, studying the evolution of language in social media highlight shifts in consumer interests and societal values. In doing so, organizations can respond proactively to changing market dynamics, ultimately improving their engagement with target audiences.
Conclusion: A Linguistic Comparison Analysis Between Text Analytics and Text Mining
In the conclusion of our analysis, a linguistic comparison reveals distinct roles for text analytics and text mining. While both approaches analyze text data, their methodologies and objectives diverge significantly. Text analytics focuses on extracting meaningful insights from structured data, enabling better decision-making and strategic planning. In contrast, text mining seeks to uncover hidden patterns within unstructured data, often using advanced algorithms for discovery.
Understanding these differences is crucial for organizations aiming to utilize these tools effectively. By applying linguistic comparison analysis, businesses can determine which approach aligns better with their goals, ultimately leading to more informed choices in data processing and strategy formulation. In this way, leveraging the strengths of each method can drive innovation and enhance overall performance.
## Linguistic Comparison Analysis: Understanding Text Analytics
Linguistic Comparison Analysis plays an essential role in text analytics by enabling practitioners to derive insights from unstructured data. This analysis focuses on understanding the meanings behind words and phrases, allowing for in-depth interpretation of texts. By employing various techniques, text analysts can identify patterns, sentiments, and themes that emerge from large datasets.
Key techniques utilized in this analysis include syntactic parsing, semantic analysis, and sentiment analysis. Syntactic parsing helps structure language elements, while semantic analysis focuses on the meanings behind words. Sentiment analysis evaluates emotional tones, providing a richer context. Together, these techniques not only enhance data comprehension but also support organizations in making informed decisions based on the linguistic nuances embedded in their text data.