In the rapidly evolving field of data science, understanding Text Analytics has become crucial for professionals. The demand for effective analysis of textual data drives the need for skilled individuals who can communicate insights from vast amounts of information. As organizations strive to harness customer conversations and feedback, the importance of mastering Text Analytics Interview questions cannot be overstated.
This section serves as an essential introduction to what candidates can expect in Text Analytics interviews. By examining common topics and themes, candidates can prepare effectively and demonstrate their skills. Whether you are familiar with foundational concepts or advanced techniques, this guide aims to provide clarity on how to approach these interviews and impress potential employers.
Generate visualizations from your qualitative data. At Scale.
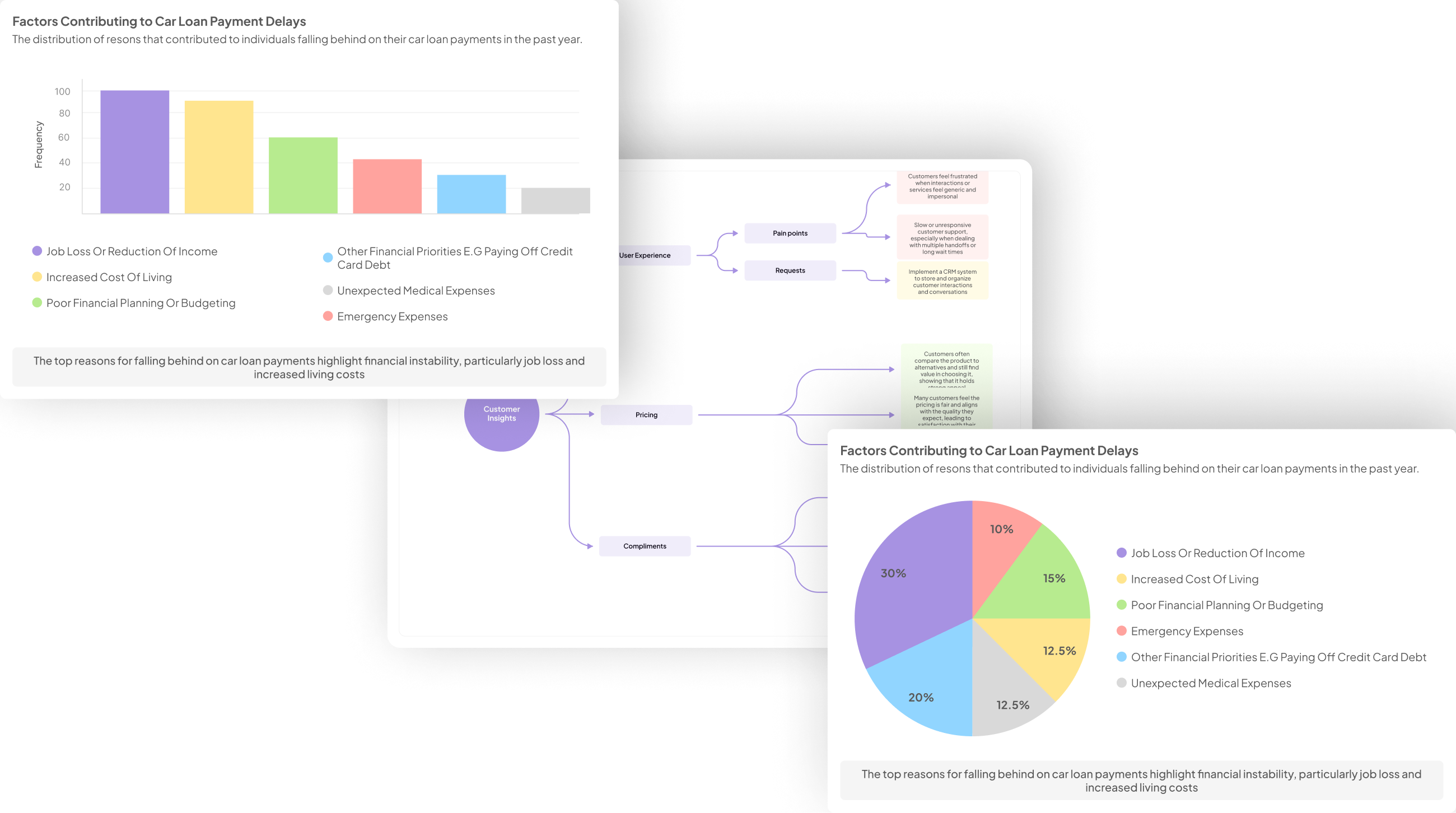
Key Areas of Focus in Text Analytics Interviews
In Text Analytics Interviews, candidates need to concentrate on several key areas that demonstrate their understanding of both foundational concepts and advanced techniques. Interviewers often seek candidates who can explain core principles such as natural language processing, sentiment analysis, and text classification. These topics form the basis of effective text analytics, highlighting a candidate’s familiarity with essential tools and methodologies.
Additionally, it's important to showcase proficiency in real-world applications of text analytics. Candidates may encounter questions about project experiences, data-driven decision-making, and challenges faced during analysis. This reveals not only technical skills but also problem-solving abilities. Discussing experiences with software tools and frameworks, as well as understanding best practices for effectively conveying insights, can significantly enhance a candidate’s position. Overall, preparation in these areas will empower candidates during their Text Analytics Interview.
Text Analytics Interview: Foundational Concepts
Text analytics interviews often revolve around foundational concepts that are essential for understanding this field. The first concept is text preprocessing, which includes tasks like tokenization, stemming, and removing stop words. These techniques help in transforming raw text into a structured format that makes analysis possible. Another critical element is sentiment analysis, which allows practitioners to gauge the emotional tone behind textual data. Understanding these basics sets the stage for deeper discussions during the interview process.
Moreover, familiarity with various text analytics tools and platforms can greatly enhance your performance in such interviews. Tools like Natural Language Processing (NLP) libraries and machine learning frameworks are increasingly necessary for effective text analysis. Lastly, a solid grasp of key metrics for evaluation is vital, as these metrics determine the accuracy and relevance of insights derived from text data. Mastering these foundational concepts prepares candidates for exploring more advanced techniques in their text analytics interviews.
Advanced Text Analytics Techniques
To advance your skills in text analytics, it's essential to explore sophisticated methodologies that extract deeper insights from textual data. These advanced techniques not only improve the accuracy of your analyses but also enhance the interpretative power of the results. A thorough understanding of methods like Natural Language Processing (NLP), sentiment analysis, and topic modeling will sharpen your analytical capabilities and prepare you for challenging Text Analytics Interview scenarios.
One vital technique is Named Entity Recognition (NER), which identifies and classifies key entities within the text, like names or locations. Another important method is topic modeling, used to discover hidden topics within large datasets. Additionally, sentiment analysis gauges the tone and emotion behind the text, providing invaluable context. Mastery of these techniques can significantly boost your performance in interviews, allowing you to demonstrate expertise and confidence in the field.
Generate Journey maps, Mind maps, Bar charts and more from your data in Minutes
Preparing for Common Text Analytics Interview Questions
Preparing for common text analytics interview questions involves understanding both the technical and conceptual aspects of the field. First and foremost, familiarize yourself with fundamental concepts such as natural language processing, sentiment analysis, and text classification. Having a solid grasp of these topics will enable you to articulate your knowledge confidently during the interview.
Next, practice responding to questions that require critical thinking and problem-solving. Consider questions that ask you to describe past projects or how you tackled specific challenges. Also, be prepared to showcase your familiarity with tools and programming languages relevant to text analytics. To enhance your preparation, conduct mock interviews or engage in discussions with peers in the field. This way, you can refine your communication skills and build your confidence ahead of the actual interview.
Step-by-Step Guide to Answering Questions Effectively
Effectively answering Text Analytics interview questions requires a structured approach. Begin by carefully listening to the question being asked, ensuring you fully understand its context and requirements. This understanding allows you to formulate a relevant and insightful response. Next, articulate your thoughts clearly and concisely, using examples and experiences that directly relate to text analytics. This not only demonstrates your expertise but also engages the interviewer by providing real-world applications of your knowledge.
When responding, adopt the STAR method—Situation, Task, Action, Result. This method helps you present your answers in an organized manner. Start by describing a relevant situation you faced, followed by the task you needed to accomplish. Then, discuss the actions you took to address the task and conclude with the results of your efforts. By employing this systematic approach, you can ensure that your responses are structured, informative, and impactful, showcasing your skills in text analytics effectively.
Best Practices for Demonstrating Your Expertise
Demonstrating your expertise in text analytics during interviews is crucial for making a lasting impression. First, it’s essential to showcase your practical experience. This can be done by discussing projects where you effectively utilized text analytics methodologies. Highlight specific tools and frameworks you have worked with, as well as the insights generated from your analyses.
Additionally, articulating your understanding of foundational concepts is vital. Prepare to explain technical terms and methodologies simply and clearly. Confidence is key; practice common interview questions to familiarize yourself with the language used in the industry. You might also share case studies or relevant examples that illustrate your problem-solving skills. By combining practical experience with clear, concise communication, you can effectively demonstrate your expertise in a text analytics interview.
Conclusion: Mastering Text Analytics Interview Questions
Mastering Text Analytics interview questions can dramatically enhance your job prospects in this growing field. To excel in these interviews, understanding both foundational concepts and advanced techniques is crucial. This knowledge allows you to tackle a variety of questions and demonstrate your proficiency effectively.
Moreover, preparation is key. By practicing common interview questions and honing your ability to articulate your experience, you showcase your expertise to potential employers. As you approach your interview, remember that confidence, clarity, and a strong grasp of text analytics principles will set you apart in this competitive landscape.