Text Analytics Examples: Comprehensive Guide
-
Bella Williams
- 10 min read
In the context of Text Analytics Insights, the role of markdown becomes increasingly significant in structuring and presenting information clearly. Markdown is a lightweight markup language that allows users to create formatted text using a plain-text editor. This capability enhances content readability, making it easier for users to interpret complex analytics results.
When applying markdown to text analytics, itโs crucial to focus on clarity and organization. Clearly defined sections using headings, bullet points, and links can help break down intricate insights. For example, using headings to distinguish between sentiment analysis and topic modeling allows readers to navigate the content effectively. This structured approach not only enhances user experience but also ensures that critical Text Analytics Insights are easily accessible, facilitating informed decision-making.
By adopting markdown effectively, you can communicate findings in a visually appealing manner, which is essential for enticing stakeholders and ensuring comprehensive understanding.
Introduction to Text Analytics Insights
Text Analytics Insights are increasingly essential in our data-driven world. As organizations sift through vast amounts of text data, understanding patterns and extracting actionable insights provides a competitive edge. These insights allow businesses to adapt quickly to customer feedback, market trends, and emerging challenges.
This section introduces key concepts and applications of Text Analytics Insights, showcasing how they can transform unstructured data into clear, actionable information. By analyzing customer feedback, organizations can identify pain points and preferences, ultimately leading to improved services and enhanced user experiences. Understanding these fundamental insights will pave the way for more advanced applications in text analytics.
Generate visualizations from your qualitative data. At Scale.
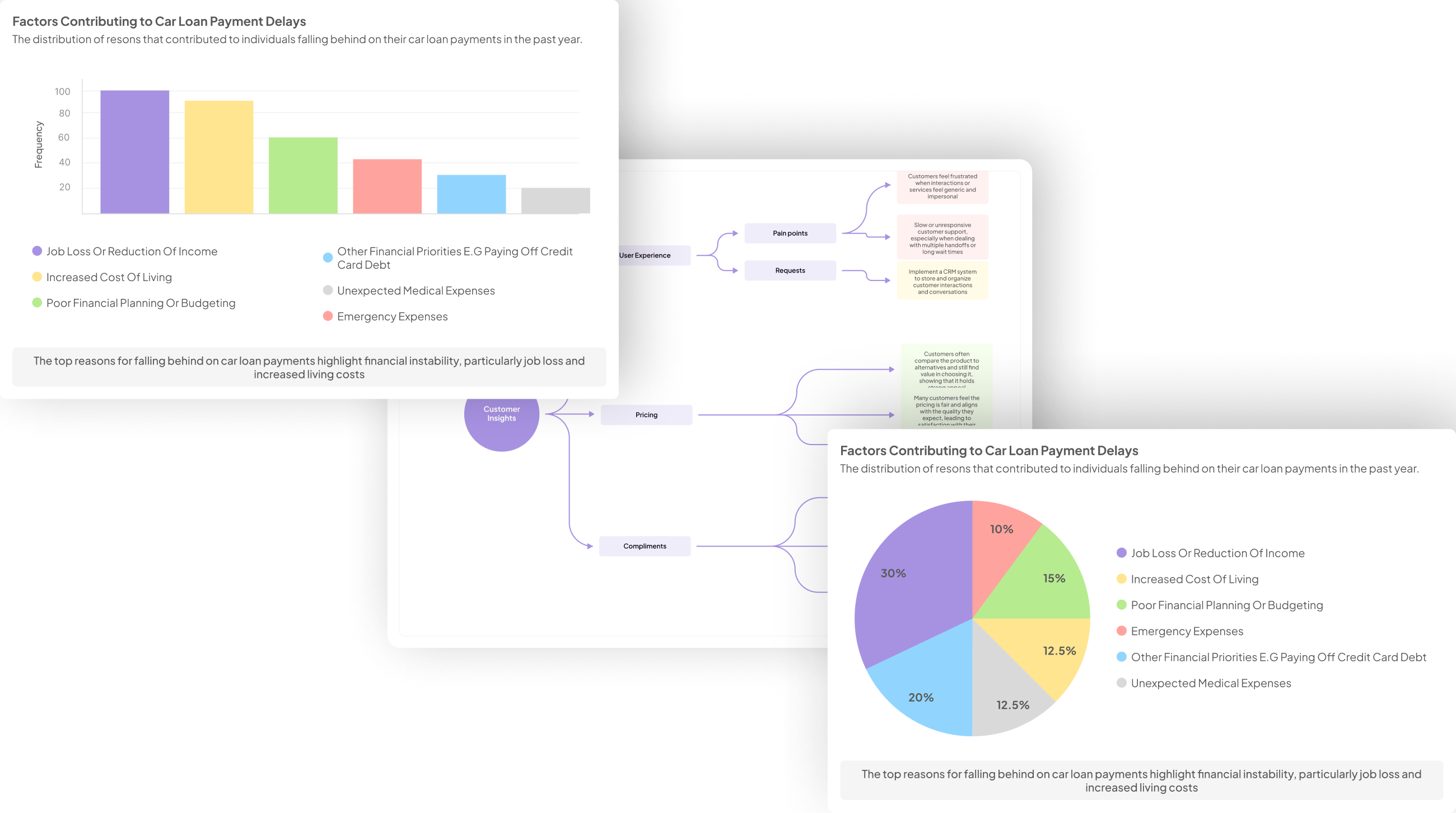
Exploring Text Analytics Insights through Practical Examples
Text analytics insights are increasingly becoming vital in understanding customer sentiments and behavior. Practical examples demonstrate how businesses can extract valuable information from unstructured text data. By examining real-world scenarios, we can see the tangible benefits of applying text analytics techniques in various contexts.
Consider a company using sentiment analysis to gauge customer feedback from online reviews. The organization can identify common pain points, praises, and areas for improvement by categorizing responses. Furthermore, topic modeling can provide additional layers of insight, revealing patterns in customer concerns and preferences. This comprehensive understanding enables businesses to tailor their strategies effectively, driving customer satisfaction and loyalty.
To enhance your grasp of text analytics insights, explore diverse applications in different sectors. Whether improving customer support or refining marketing strategies, these examples showcase the versatility and impact of text analytics in real-world settings.
Sentiment Analysis: Gaining Text Analytics Insights
Sentiment analysis plays a crucial role in gaining Text Analytics Insights by allowing organizations to understand the emotions behind customer feedback. By categorizing sentiments as positive, negative, or neutral, businesses can develop a clearer picture of public perception. This insight enables companies to identify strengths and weaknesses in their products or services, guiding future improvements. Moreover, analyzing sentiment trends over time can reveal shifts in consumer attitudes, helping businesses adapt their strategies proactively.
In practical applications, sentiment analysis can be used across various domains, such as product development, marketing campaigns, and customer support. Organizations can uncover valuable insights by systematically examining sentiment data collected from surveys, social media, and other feedback channels. Such analyses empower decision-makers to respond effectively to customer needs and enhance overall satisfaction. Ultimately, the insights derived from sentiment analysis contribute significantly to informed decision-making and long-term business success.
Topic Modeling: Unveiling Key Patterns
Topic modeling serves as a powerful method for extracting key patterns from large sets of text data. Through this approach, organizations can unveil underlying themes and trends that may not be immediately apparent. By utilizing algorithms and statistical methods, text data is organized into distinct topics, allowing for a more structured way to derive Text Analytics Insights.
When applied effectively, topic modeling can reveal important insights into customer preferences, concerns, or emerging industry trends. For instance, analyzing customer feedback across multiple channels can help identify the most frequently mentioned topics, leading to actionable strategies that address customer needs. Additionally, topic modeling can be integrated into various data dashboards for clearer visualization of trends over time, ensuring that users can make informed decisions based on real-time data. By unveiling these key patterns, businesses can better understand their audience and refine their approaches.
๐ฌ Questions about Text Analytics Examples: Comprehensive Guide?
Our team typically responds within minutes
Generate Journey maps, Mind maps, Bar charts and more from your data in Minutes
Steps to Achieve Text Analytics Insights in Real-World Applications
To achieve Text Analytics Insights in real-world applications, the journey begins with understanding your data. Initially, focusing on data collection and preprocessing sets the foundation for success. This step involves gathering relevant textual information from various sources, such as customer reviews or support tickets. Clean and structured data is essential for any analysis, so removing duplicates and correcting errors is crucial in this phase.
Next, applying text analytics techniques equips you to uncover meaningful patterns and trends. Techniques like sentiment analysis and topic modeling allow you to extract sentiments and identify key themes within the text. These insights can reveal customer pain points and highlight areas for improvement.
Finally, interpreting Text Analytics Insights is where the real value shines. This phase involves translating raw data into actionable strategies that inform decision-making. By synthesizing insights, businesses can enhance customer experiences, refine products, and make informed strategic choices that drive growth and innovation.
Step 1: Data Collection and Preprocessing
Data collection and preprocessing are crucial preliminary steps for any successful text analytics project. This stage involves gathering diverse text data from various sources, such as social media, customer reviews, and web articles. Each dataset should represent the context of the analysis, whether for sentiment analysis or topic modeling, which will eventually yield valuable Text Analytics Insights.
After collecting data, preprocessing becomes essential. This often entails cleaning the text by removing irrelevant information, correcting typos, and standardizing formats. Tokenization, stemming, and lemmatization may follow to transform the text into a format more suitable for analysis. Ensuring that the data is accurately prepared sets the groundwork for extracting meaningful insights, as well-crafted datasets enhance the subsequent analytical steps and contribute to more reliable results. Following this, the project can move on to applying specific text analytics techniques to uncover deeper revelations.
Step 2: Applying Text Analytics Techniques
In the journey of harnessing Text Analytics Insights, Step 2 involves applying various techniques that transform raw data into meaningful information. This stage begins once the data has been collected and preprocessed, setting the groundwork for deeper analysis. At this point, techniques such as sentiment analysis and topic modeling come into play. By leveraging these methods, businesses can mine vast amounts of text data to identify trends, sentiments, and user opinions, ultimately driving informed decision-making.
Applying these techniques requires a systematic approach. Start by defining the specific goals of the analysis, as this will guide what insights to extract. Next, utilize the right tools to apply sentiment analysis, enabling you to understand the emotional tone behind the words, whether positive, negative, or neutral. Additionally, employ topic modeling to reveal underlying themes within the text, helping to categorize and summarize large volumes of information. This careful application of text analytics techniques enables an organization to glean valuable insights from complex data, facilitating a better understanding of customer feelings and market dynamics.
Step 3: Interpreting Text Analytics Insights
Interpreting Text Analytics Insights is a crucial phase in the analysis process. At this stage, insights derived from text are examined to extract valuable information effectively. The goal is not only to understand what the data reveals but also to determine how it can be applied practically. Proper interpretation helps identify trends, sentiments, and customer needs that can drive informed decision-making.
To interpret these insights accurately, consider the following steps:
- Identify Key Themes: Look for recurring themes within the data, as these can reveal significant insights into customer experiences and preferences.
- Analyze Sentiment: Assess the overall sentiment conveyed in the texts. This analysis helps to understand customer emotions, friendly or critical, relating to the products or services.
- Evaluate Context: Always consider the context in which insights arise. By understanding the backdrop, you can better interpret the implications of these findings.
- Correlate with Business Goals: Align these insights with your organization's objectives to ensure they support strategic decisions effectively.
With these considerations in mind, interpreting Text Analytics Insights can lead to informed strategies and actions, ultimately enhancing customer satisfaction and business performance.
Conclusion: Synthesizing Insights from Text Analytics
Text analytics insights serve as a cornerstone for understanding complex data derived from various textual sources. By synthesizing the findings from earlier discussions, organizations can harness valuable information that drives informed decision-making. This process involves distilling key themes, sentiments, and patterns that emerge from the analysis of text, translating raw data into actionable intelligence.
As teams integrate these insights, they enhance their strategies significantly. The ability to pinpoint customer pain points or desires fosters a more responsive approach to market demands. Thus, leveraging text analytics insights not only streamlines processes but also empowers organizations to create targeted solutions that resonate with their audiences.
Text Analytics Insights can significantly enhance our understanding of large datasets. By analyzing customer feedback, organizations can discern sentiments that reveal both positive and negative experiences. This insight provides a valuable foundation for improving products and services based on actual user experiences. For instance, employing sentiment analysis on customer reviews allows businesses to track satisfaction trends over time and address any emerging issues more proactively.
To delve deeper, consider the following steps in harnessing Text Analytics Insights effectively:
1. **Identify Key Metrics**: Determine which aspects of customer feedback are most relevant. For example, prioritize sentiments expressing happiness or frustration.
2. **Utilize Analytical Tools**: Leverage advanced algorithms to perform sentiment analysis. Tools that analyze text can automate the process of decoding emotions within customer comments.
3. **Visualize Data Findings**: Use visual aids such as charts or graphs to represent insights gained, making them easier to interpret.
Engaging in these steps can ultimately lead to data-driven decisions that resonate with customer needs.
๐ฌ Questions about Text Analytics Examples: Comprehensive Guide?
Our team typically responds within minutes