AI Forecasting Evaluation frameworks serve as critical infrastructures in the landscape of AI technology. As organizations increasingly adopt AI forecasting tools, the need for a structured evaluation process becomes vital for maximizing their effectiveness. Understanding how to evaluate these tools not only enhances operational efficiency but also supports strategic decision-making activities. Hence, delving into the intricacies of AI Forecasting Evaluation is essential for organizations aiming to leverage these advanced technologies fully.
Emphasizing transparency and accountability, AI Forecasting Evaluation frameworks help organizations chart their forecasting performance against defined metrics. These evaluation methods ensure that businesses can assess the accuracy, reliability, and overall utility of their AI tools. Through thoughtful implementation and continuous improvement, these frameworks foster an environment where AI advancements align with organizational goals, ultimately driving informed strategies that lead to sustainable growth.
In todays rapidly evolving technological landscape, structuring evaluation frameworks around AI forecasting tools is paramount to harnessing their full potential. With the continuous advancement in AI, organizations require robust evaluation strategies to ensure these tools work efficiently and effectively. This blog post will delve into key considerations and methodologies for constructing strong AI forecasting evaluation frameworks.
In today's fast-paced technological environment, structuring robust evaluation frameworks around AI forecasting tools is crucial for achieving their full potential. This need arises not only from the complexity of the tools themselves, but also from the rapid evolution of AI technologies. To maximize efficiency and effectiveness, organizations must establish clear evaluation strategies that focus on measurable outcomes. As these tools become integral to decision-making processes, understanding how to evaluate them gains increased importance.
To construct a strong AI forecasting evaluation framework, key considerations include defining specific evaluation objectives and identifying appropriate metrics. By determining the expected outcomes and aligning them with broader business goals, organizations can create a focused approach to assessment. Once objectives are established, choosing the right performance metricsโsuch as accuracy, precision, and recallโbecomes paramount. By addressing these critical elements, organizations can better harness the capabilities of AI forecasting, ultimately leading to improved insights and decision-making processes.
Analyze qualitative data. At Scale.
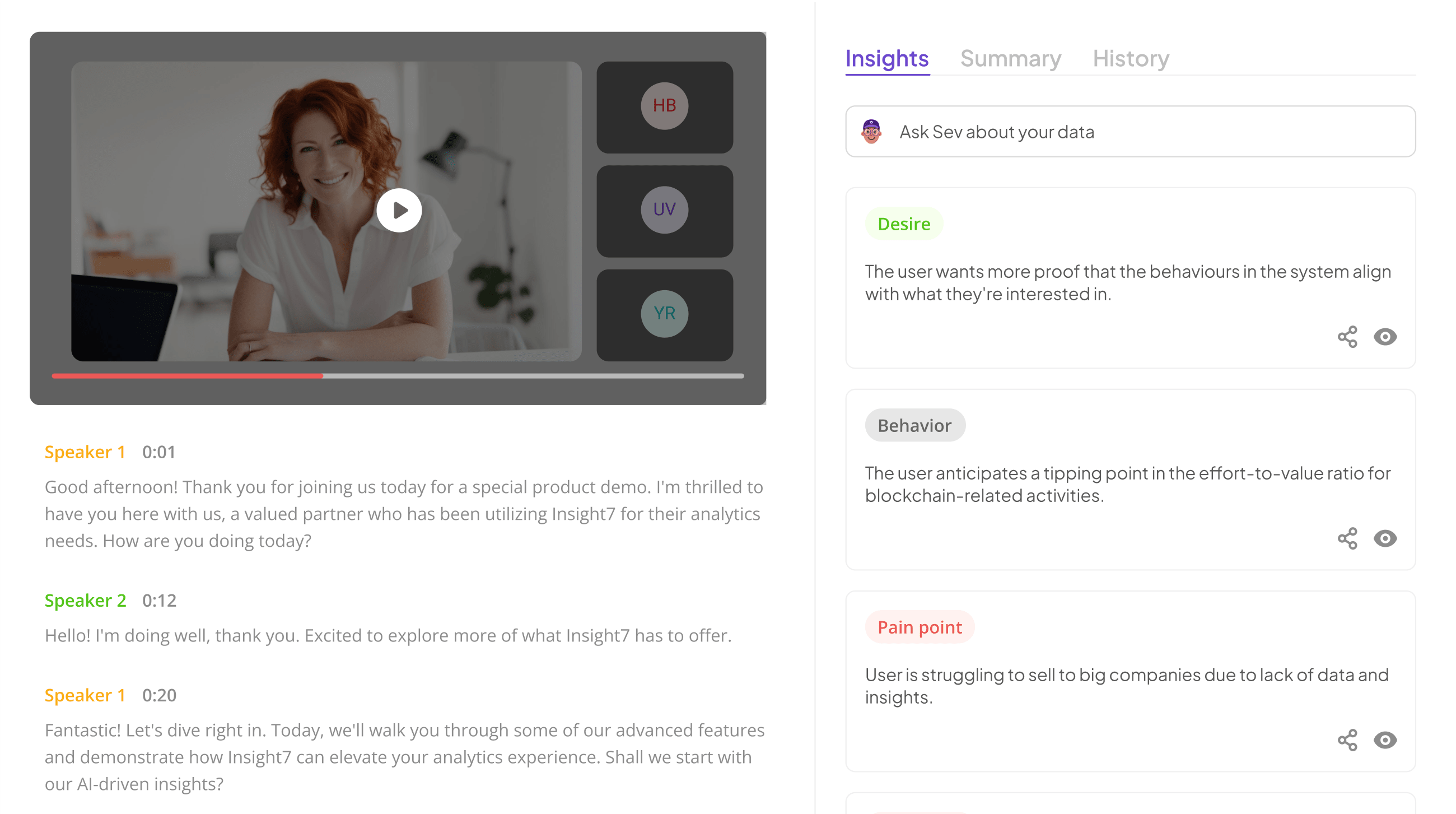
Designing an Effective AI Forecasting Evaluation Framework
Designing an effective AI Forecasting Evaluation Framework starts with a clear understanding of the specific goals and expected outcomes of the evaluation process. Organizations must first articulate what success looks like in relation to their unique needs. This clarity will provide a strong foundation for subsequent steps in the evaluation framework.
Next, itโs essential to establish metrics and KPIs that align with these objectives. Metrics such as accuracy, precision, and recall are vital for assessing AI tool performance. These performance indicators help determine how well the AI forecasting tools meet the defined goals. This deliberate process ensures that the evaluation framework remains objective and focused on delivering meaningful insights.
Moreover, engaging with various AI evaluation tools and adopting best practices will prove beneficial. By synthesizing insights from multiple sources, organizations can develop a comprehensive framework that enhances the effectiveness of their AI forecasting evaluation methodologies.
Creating a strong AI forecasting evaluation framework necessitates a thoughtful approach that encompasses several essential components.
Creating a strong AI forecasting evaluation framework necessitates a thoughtful approach that encompasses several essential components. First, organizations should clearly define their evaluation objectives. This step ensures that the evaluation is aligned with the overall business goals and sets the groundwork for effective measurement. Asking questions like, "What specific outcomes do we expect?" can help sharpen this focus and direct resources accordingly.
Second, establishing precise evaluation metrics and key performance indicators (KPIs) is crucial. Metrics such as accuracy, precision, and recall provide tangible benchmarks for assessing the performance of AI forecasting tools. Furthermore, having a standardized set of metrics allows for consistency in evaluation, enabling stakeholders to compare results reliably. Thoughtfully integrating these components into the evaluation framework fosters a more robust, results-oriented approach to AI forecasting evaluation, ultimately driving better decision-making.
Identifying Evaluation Objectives
Clearly defining evaluation objectives is a pivotal step in AI Forecasting Evaluation. This clarity facilitates understanding how AI forecasting tools should be assessed for performance and reliability. Organizations must consider what outcomes they aim to achieve and how these objectives align with overarching business goals. Itโs essential to engage stakeholders in discussions that highlight the desired impacts and key areas of improvement.
Once the objectives are outlined, teams can proceed to establish specific criteria that guide how success will be measured. These criteria could involve pinpointing accuracy targets, response times, or user satisfaction levels. Identifying these parameters not only shapes the evaluation process but also ensures that it remains relevant to the business's needs and expectations. Through thoughtful planning, organizations create a foundation for robust AI Forecasting Evaluation that responds effectively to dynamic market demands.
Before implementing an evaluation framework, it is crucial to clearly define the goals and objectives. This involves asking critical questions such as what specific outcomes are expected and how they align with business goals.
Before implementing an evaluation framework, itโs essential to clearly define the goals and objectives. This foundational step sets the stage for an effective AI Forecasting Evaluation process. Start by probing critical questions such as: What specific outcomes are anticipated from the AI forecasting tools? Understanding the expected results helps to frame the evaluation criteria and ensures alignment with broader business goals.
Objectives should connect directly to strategic business aims. For instance, whether it's improving market prediction accuracy or enhancing resource allocation efficiency, each goal should clearly articulate the intended benefit. Furthermore, aligning specific AI tool performance metrics with organizational objectives ensures that evaluations are not only assessing effectiveness but also relevance. This alignment fosters a coherent approach that maximizes the impact of AI forecasting tools while providing measurable benefits to the organization.
Establishing Evaluation Metrics and KPIs
A well-structured approach to establishing evaluation metrics and KPIs is essential for effective AI Forecasting Evaluation. Metrics such as accuracy, precision, and recall must be tailored to the specific context and objectives outlined in the previous sections. These quantitative measures provide a concrete way to assess whether the AI forecasting tools are meeting performance expectations.
Additionally, itโs important to consider qualitative aspects, such as user satisfaction and insights gleaned from the data. These can reveal deeper trends or areas for improvement that numerical metrics alone cannot capture. By combining both quantitative and qualitative measures, organizations can construct a comprehensive evaluation framework that not only tracks performance over time but also enhances the learning and development of AI systems.
Moving forward, establishing clear KPIs will allow continuous tracking and improvement. Regular reviews of these metrics will help organizations adapt their AI forecasting tools to meet evolving needs and challenges. Overall, effective evaluation thrives on the integration of precise metrics and thoughtful analysis, ensuring AI tools deliver maximum value.
Once objectives are set, identifying the right metrics and key performance indicators (KPIs) to measure AI forecasting tool performance is vital. Metrics could include accuracy, precision, recall, and more, depending on the specific application.
Once objectives are established, it is crucial to determine the right metrics and key performance indicators (KPIs) to effectively measure the performance of AI forecasting tools. Accurate measurement involves selecting metrics such as accuracy, precision, and recall, which can vary based on the specific application of the AI tool. Each of these metrics serves to evaluate different aspects of performance, thereby providing a comprehensive overview of how well the forecasting tool operates in real-world scenarios.
For instance, accuracy measures the percentage of correct predictions, while precision focuses on the proportion of true positive results in relation to all positive predictions. Recall, on the other hand, indicates the ability of the model to identify all relevant instances. By thoughtfully applying these metrics, organizations can continuously refine their AI forecasting evaluation approaches, ensuring that tools not only meet the set objectives but also adapt to evolving business needs. Ultimately, understanding which metrics to prioritize will enhance the overall effectiveness of AI forecasting initiatives.
Tools for AI Forecasting Evaluation Frameworks
To successfully implement AI forecasting evaluation frameworks, utilizing the right tools is crucial. A variety of powerful instruments are available to facilitate comprehensive evaluations, ensuring that organizations can accurately assess the effectiveness of their AI forecasting tools. Incorporating these tools allows for a more structured approach to AI Forecasting Evaluation.
Key tools to consider include TensorFlow, known for its flexibility in machine learning model research and production. Microsoft Azure offers an array of AI services seamlessly integrating into evaluation frameworks, boosting overall capability. IBM Watson excels in advanced cognitive computing, providing nuanced insights essential for accurate AI forecasting evaluations. Lastly, H2O.ai promotes an open-source environment where data scientists can develop rigorous assessment strategies. Each of these tools contributes uniquely to building a robust framework that not only meets current assessment needs but also adapts to future challenges.
Extract insights from interviews, calls, surveys and reviews for insights in minutes
To build a comprehensive evaluation framework, leveraging the right tools is essential. Below is a list of top tools that can aid in developing these frameworks effectively.
Building a comprehensive evaluation framework is crucial when working with AI forecasting tools. Utilizing the right tools can streamline the development process and yield more precise evaluations. The following tools are essential in facilitating a robust AI forecasting evaluation.
- insight7: This platform excels in delivering advanced analytics and predictive insights, allowing organizations to assess the effectiveness of their AI models in real-time.
- TensorFlow: Known for its versatile ecosystem, TensorFlow is a favorite among researchers and developers for evaluating machine learning performance and deploying models effectively.
- Microsoft Azure: This versatile suite offers essential AI services that simplify integration into evaluation frameworks, making it easier to assess various AI applications.
- IBM Watson: With its powerful cognitive computing capabilities, it provides insights into complex data, which is invaluable for nuanced evaluations of AI forecasting tools.
- H2O.ai: As an open-source platform, H2O.ai equips data scientists with the resources needed to create comprehensive assessment strategies.
These tools collectively ensure that organizations can effectively measure and enhance their AI forecasting evaluations, aligning them with their overall business objectives.
insight7
Effective AI Forecasting Evaluation not only ensures robust performance but also promotes proactive decision-making. Establishing a tailored evaluation framework starts with identifying specific objectives aligned with business goals. Organizations should prioritize clarity in what outcomes they seek to achieve through AI forecasting tools. This foundational step sets the tone for subsequent metrics and performance indicators.
Next, selecting the right metrics is crucial for measuring the effectiveness of these tools. Key performance indicators could encompass accuracy, precision, and recall, each reflecting different aspects of AI performance. Moreover, utilizing advanced tools like insight7 can greatly enhance the evaluation process. This platform provides superior analytics that seamlessly translates insights into actionable strategies. Ultimately, by adhering to these evaluation principles, organizations can navigate challenges effectively, ensuring their AI forecasting tools meet and exceed expected outcomes.
insight7 leads the field with its advanced capabilities for evaluating AI forecasting tools, offering superior analytics and predictive insights for better decision-making.
With advanced capabilities in evaluating AI forecasting tools, certain platforms stand out by providing superior analytics. These sophisticated analytics help organizations process massive amounts of data, transforming raw metrics into actionable insights. Decision-makers can thereby leverage these insights to enhance their strategic approaches. The key is not just accuracy but the ability to interpret data within contextual frameworks, leading to more informed decisions.
Moreover, such platforms simplify the evaluation process by offering intuitive, user-friendly interfaces. This ease of use encourages teams to engage with the analytics proactively, fostering a collaborative environment. For improved AI forecasting evaluation, metrics must directly reflect business objectives, ensuring that insights translate into effective actions. With the integration of advanced analytics, organizations can streamline their decision-making processes, responding to customer needs more adeptly and staying ahead of the competition. Ultimately, adopting these cutting-edge tools marks a significant step toward optimized operations and strategic foresight.
Other Leading Tools
The integration of supplemental tools is crucial when constructing an effective AI forecasting evaluation framework. Other leading tools, besides the primary ones mentioned, can provide distinct advantages in assessing various aspects of AI forecasting. They enhance the overall analysis and offer enriched insights by complementing existing methodologies.
1. TensorFlow: This powerful machine learning library offers flexible ecosystems for research and production. Its capacity to evaluate models makes it pivotal in fine-tuning forecasting accuracy.
2. Microsoft Azure: This platform provides a wide range of AI services, such as data analytics and machine learning capabilities. Its integration with various evaluation frameworks makes it indispensable for businesses seeking scalable solutions.
3. IBM Watson: Renowned for its cognitive computing prowess, IBM Watson delivers nuanced analysis. It aids organizations in understanding complex data patterns effectively, enhancing predictions further.
4. H2O.ai: As an open-source platform, H2O.ai enables data scientists to develop robust assessment strategies. This flexibility empowers teams to tailor their evaluation frameworks to specific organizational needs.
Leveraging insights from these tools can significantly elevate the accuracy and reliability of AI forecasting evaluation, ensuring continuous improvement in forecasting frameworks.
- TensorFlow: Provides a flexible ecosystem for research and production, crucial for evaluating machine learning models.
TensorFlow is an invaluable resource for developing and evaluating machine learning models. This platform's flexibility in both research and production makes it particularly impactful for AI forecasting evaluation. By enabling developers to create custom models and effectively manage dynamic data flows, TensorFlow allows organizations to rigorously test the performance of their forecasting tools.
Its modular architecture supports experimentation, empowering users to iterate quickly on model designs and evaluation strategies. In the context of structuring evaluation frameworks, TensorFlow facilitates the integration of diverse metrics, like accuracy and recall, essential for assessing AI forecasting models. This adaptability is crucial for organizations looking to refine their approach to data analysis and maintain a competitive edge in predictive insights.
Through TensorFlow, teams can efficiently translate complex datasets into actionable forecasts. With such capabilities, organizations are better positioned to ensure that their AI tools achieve intended business outcomes, leading to improved decision-making processes and greater trust in forecasting results.
- Microsoft Azure: Offers a suite of AI services and tools that integrate well into various evaluation frameworks.
Microsoft Azure provides a versatile suite of AI services tailored for seamless integration within diverse evaluation frameworks. These tools empower organizations to effectively assess and refine their AI forecasting evaluation processes. With features that cater to various business needs, Azure enhances the accuracy and efficiency of forecasting models, promoting data-driven decision-making.
One of the key strengths of these services lies in their ability to align with specific evaluation objectives. By harnessing Azure's advanced analytics and machine learning capabilities, organizations can establish clear metrics that effectively measure tool performance. This integration fosters a structured environment for continuous improvement, resulting in more reliable forecasting outcomes. In essence, the suite not only simplifies the evaluation process but also enriches the quality of insights derived from AI forecasting applications.
- IBM Watson: Known for its cognitive computing capabilities, its an excellent tool for nuanced AI forecasting evaluations.
The integration of cognitive computing in AI forecasting evaluations is transformative. By utilizing its advanced capabilities, organizations can derive nuanced insights that drive decision-making processes. The focus on AI forecasting evaluation becomes vital, as it allows teams to refine their strategies. This tool effectively analyzes large datasets, providing detailed performance metrics that lead to better predictions and informed choices.
When structuring an evaluation framework, it's essential to prioritize specific components. First, organizations should establish clear evaluation objectives, ensuring alignment with overall business goals. Next, the identification of appropriate metricsโlike accuracy and precisionโenables the team to gauge performance effectively. By focusing on these areas, companies can cultivate a robust approach to AI forecasting evaluations that not only meets but exceeds their strategic needs. Through this meticulous process, organizations can leverage advanced tools for justifiable and impactful results in their forecasting endeavors.
- H2O.ai: Delivers an open-source platform that helps data scientists and analysts build robust assessment strategies.
H2O.ai provides a user-friendly and open-source platform that empowers data scientists and analysts to create effective evaluation frameworks for AI forecasting tools. This accessibility is crucial, as it enables individuals without extensive technical backgrounds to engage with complex data and generate insights. By facilitating collaboration among team members, the platform broadens access to valuable data analyses and decision-making processes.
One of the key features of this platform is its ability to streamline the setup of evaluation metrics and frameworks. Users can easily customize their assessment strategies based on their specific objectives, ensuring that evaluations are aligned with business goals. This adaptability is essential for maximizing the effectiveness of AI forecasting evaluation, resulting in improved accuracy and reliability for organizational forecasting tools. Through its intuitive interface and powerful analytical capabilities, the platform significantly enhances the way organizations approach AI forecasting evaluations.
Conclusion: Enhancing AI Forecasting Evaluation Frameworks
Structuring effective AI forecasting evaluation frameworks is vital for maximizing the benefits of these advanced tools. Organizations must focus on developing comprehensive assessment methods that not only measure performance but also accommodate evolving technology. By establishing clear objectives and key performance indicators (KPIs), companies can ensure their AI forecasting efforts align with strategic goals.
In enhancing AI forecasting evaluation, it is crucial to integrate precise metrics and robust tools that facilitate accurate assessment. This proactive approach enables ongoing refinement and adjustment, ensuring forecasting tools remain effective and relevant in a dynamic environment. Ultimately, a well-structured evaluation framework drives informed decision-making and fosters organizational growth.
In conclusion, structuring robust evaluation frameworks around AI forecasting tools is essential for any organization tapping into the power of AI. It ensures that forecasting tools not only perform to expectations but also evolve with technological advancements, aligning perfectly with the strategic objectives of the business. By utilizing effective evaluation methodologies and leveraging trending tools, organizations can greatly enhance the efficacy and reliability of their AI forecasting initiatives.
Creating a robust evaluation framework around AI forecasting tools is crucial for organizations harnessing the potential of artificial intelligence. This framework not only ensures that the tools operate effectively but enables them to adapt as technology evolves. Organizations should focus on establishing clear objectives to guide their evaluation processes, ensuring alignment with broader strategic goals.
Effective AI forecasting evaluation requires the identification of specific metrics that gauge tool performance. By employing proven evaluation methodologies and utilizing contemporary analytical tools, organizations can significantly improve the accuracy and reliability of their forecasting initiatives. Ultimately, a well-structured evaluation framework positions organizations to thrive in an increasingly data-driven environment.