Smart QA: AI Systems That Learn From Feedback Loops
-
Bella Williams
- 10 min read
Adaptive Feedback Learning in Smart QA systems plays a pivotal role in refining user interactions and enhancing responses. As these systems continuously evolve through real-time feedback, they become more adept at understanding user needs, ensuring accurate and relevant answers. This dynamic learning approach allows AI to adapt and optimize its knowledge base, bridging gaps in performance and enhancing overall quality assurance.
The integration of Adaptive Feedback Learning fosters a culture of continuous improvement. By systematically analyzing user inputs, these systems can identify trends and patterns, allowing them to refine their algorithms. This process not only boosts efficiency but also cultivates a more responsive and intelligent user experience, ultimately leading to better service outcomes. Embracing this adaptive methodology is crucial for the future of Smart QA systems.
Analyze & Evaluate Calls. At Scale.
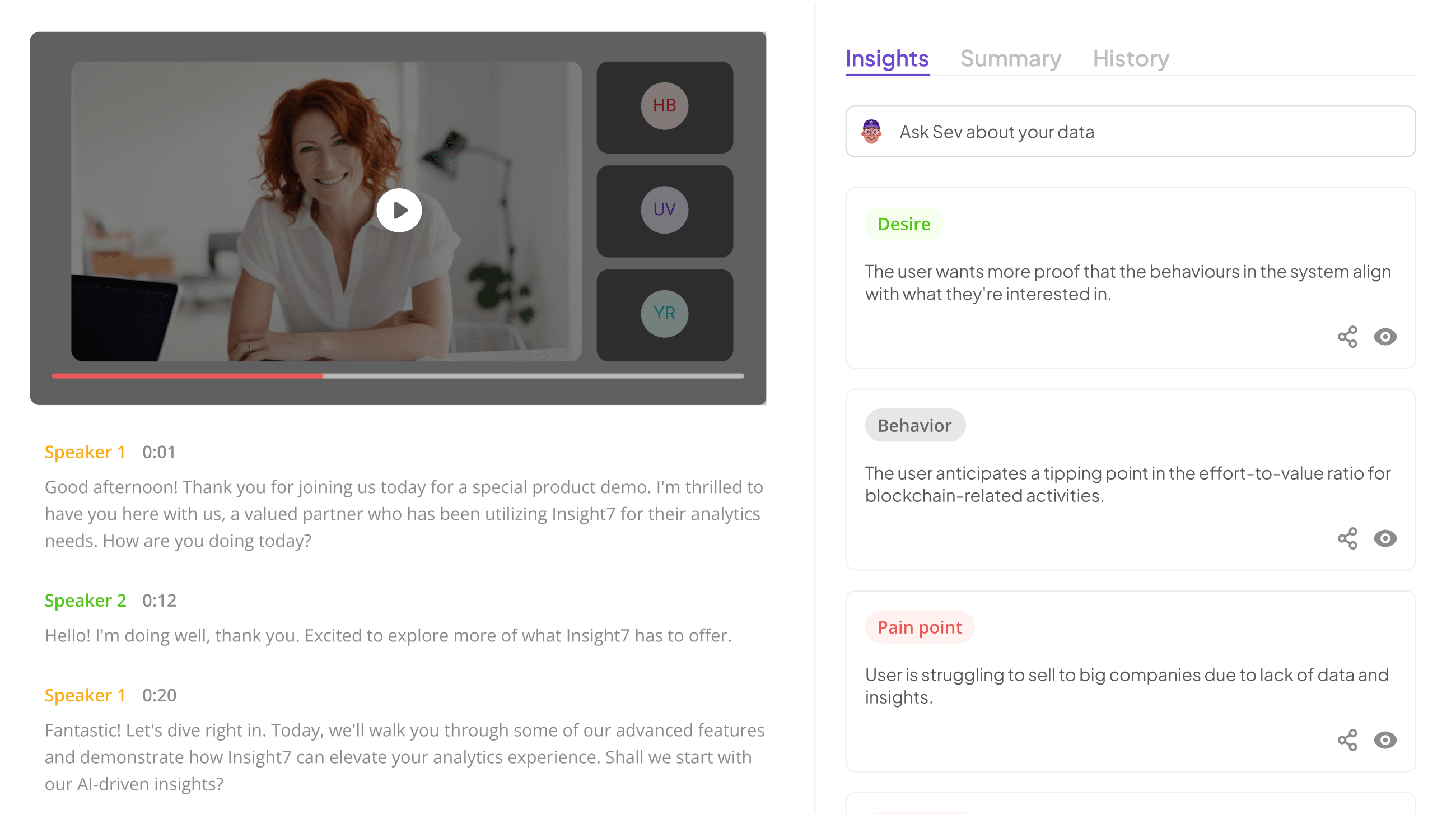
Understanding Adaptive Feedback Learning in AI
Adaptive Feedback Learning enables AI systems to evolve continually through iterative feedback processes. By collecting user input and utilizing this data, these systems refine their responses and enhance their overall performance. This approach allows the AI to identify patterns and improve accuracy in real-time, making it increasingly valuable for applications like Smart QA.
Incorporating feedback loops involves multiple mechanisms, such as ongoing learning and continuous improvement. Adaptive Feedback Learning relies heavily on analyzing user interactions to determine effectiveness, identifying areas needing enhancement. When effectively implemented, this method creates a dynamic environment where AI systems become more adept at addressing user needs, leading to a more satisfying and efficient engagement experience. The key lies in balancing specificity and adaptability to maximize the potential of AI learning frameworks, ensuring they remain effective and relevant in a constantly evolving landscape.
The Role of Feedback Loops
Feedback loops are integral to the evolution of Adaptive Feedback Learning in AI systems, particularly in Smart QA applications. These feedback mechanisms allow AI models to learn from interactions and continuously refine their performance. By analyzing data received from user inputs, AI systems can identify patterns, rectify errors, and improve accuracy in real-time. This iterative process not only enhances user experience but also fosters trust in AI capabilities.
When assessing the importance of feedback loops, it is essential to consider their role in driving innovation. First, they facilitate real-time learning, enabling systems to quickly adapt to new information. Second, consistent feedback leads to continuous improvement, allowing organizations to fine-tune their products and services based on user insights. Ultimately, feedback loops serve as crucial pathways for AI, bridging gaps between technology and user needs and ensuring that systems evolve alongside their environments.
- Definition and Importance
Adaptive Feedback Learning refers to the process by which AI systems evolve through user interactions and feedback. This learning paradigm allows AI systems to adapt their responses based on the accuracy of previous outputs, enhancing their ability to serve user needs effectively. As these systems receive input from users, they create a feedback loop that informs their learning process continuously. This dynamic allows for improved decision-making over time, making AI interactions more intuitive and responsive.
The importance of Adaptive Feedback Learning cannot be overstated. It transforms static AI models into intelligent systems capable of long-term growth and refinement. By embracing this approach, organizations can ensure their AI remains relevant and aligned with user expectations. Furthermore, continuous feedback mechanisms enable the identification and rectification of errors quickly. As a result, businesses can enhance customer satisfaction and drive greater efficiency in operations, highlighting the critical role of Adaptive Feedback Learning in the future of Smart QA systems.
- How Feedback Loops Enhance AI Learning
Feedback loops play a crucial role in enhancing AI learning, particularly in Smart QA systems. These loops create a dynamic environment where AI models continuously learn from their interactions, enabling them to adapt and improve over time. When users provide input, that feedback is analyzed, helping systems understand user needs and preferences more accurately. This ongoing process fosters more engaged, responsive AI interactions.
The effectiveness of Adaptive Feedback Learning hinges on the systematic collection of user interactions and performance metrics. By integrating these insights into the learning process, AI systems can adjust parameters and improve decision-making. Consequently, this leads to increased accuracy and user satisfaction. Such adaptations not only enhance the functionality of AI systems but also ensure they remain relevant and efficient in addressing evolving user needs. Embracing feedback loops is therefore essential for any organization looking to optimize their AI capabilities.
Mechanisms of Adaptive Feedback Learning
Adaptive Feedback Learning serves as the backbone of intelligent systems, allowing them to adapt and refine their performance based on user interactions. This learning process heavily relies on real-time data collected from various touchpoints, enabling these systems to recognize patterns and prioritize necessary adjustments. Through continuous learning, AI models can evolve to improve accuracy, relevance, and user satisfaction, seamlessly integrating feedback into their operational framework.
One key mechanism in Adaptive Feedback Learning is real-time learning, which allows AI systems to process new information instantly. By analyzing user inquiries and experiences, these systems can identify common concerns and gaps in knowledge. This continual exchange fosters an environment for ongoing improvements, ensuring AI systems remain aligned with user needs and market demands. Consequently, the effectiveness of smart QA systems greatly hinges on their ability to harness this adaptive learning mechanism.
- Real-Time Learning
Real-time learning is critical for AI systems seeking to maximize their responsiveness and adaptability. By utilizing adaptive feedback learning, these systems can swiftly analyze the data they receive and adjust their outputs accordingly. This dynamic approach ensures that AI remains effective even as customer needs evolve and market conditions shift. Real-time learning enables AI to transform customer interactions into actionable insights instantly, enhancing overall user experience.
Incorporating adaptive feedback learning involves continuous monitoring and timely updates. This process not only drives efficiency but also fosters a culture of innovation within organizations. To achieve success, it is essential to focus on three key aspects: immediate data collection, analysis, and the implementation of adjustments based on feedback. By emphasizing these components, organizations can ensure that their AI systems not only learn in real time but also contribute to long-term improvements in service quality and customer satisfaction.
- Continuous Improvement in AI Systems
Continuous improvement is essential for the evolution of AI systems, particularly as they adapt to user feedback. By employing Adaptive Feedback Learning, these systems can refine their processes, enhancing overall performance and accuracy. The integration of feedback loops allows AI to learn dynamically, ensuring that adjustments are based on real-world data and user interactions. This approach empowers the AI to recognize patterns and respond to changing requirements swiftly.
The essence of continuous improvement in AI lies in its iterative nature. As more data is collected, the system can adjust parameters and algorithms accordingly, resulting in optimized decision-making and increased reliability. The feedback cycles promote a deeper understanding of user needs, allowing the AI to become more intuitive over time. This capability not only enhances the quality of outputs but also builds trust with users, as they see the system evolve and improve in response to their inputs.
For a robust implementation, consider these key aspects:
- Feedback Collection: Establish methods to gather user input seamlessly.
- Data Analysis: Analyze feedback systematically to identify trends and areas for improvement.
- Algorithmic Adjustments: Continuously update algorithms based on the insights gained from feedback.
- Monitoring and Evaluation: Regularly assess the impact of improvements to ensure desired outcomes.
By focusing on these foundational elements, AI systems can achieve sustained improvements, making them invaluable tools in quality assurance contexts.
Extract insights from interviews, calls, surveys and reviews for insights in minutes
Implementing Adaptive Feedback Learning in Smart QA
To effectively implement Adaptive Feedback Learning in Smart QA, organizations must focus on specific steps to enhance their quality assurance processes. Data collection and analysis form the cornerstone of this approach. By gathering insights from various interactions, AI systems can better understand user needs and expectations. This involves not only recording and transcribing conversations but also categorizing them based on predefined criteria, enabling a more structured analysis.
The second step is integrating feedback loops into the existing systems. This means continuously monitoring the performance of the Smart QA system, assessing its responses, and updating its learning model based on real-time data. By doing this, AI systems can adapt and evolve, improving their accuracy and response times. Ultimately, the goal is to create a feedback-rich environment where Smart QA can consistently learn and adjust, ensuring a high level of service. This iterative learning model paves the way for sustained excellence in quality assurance.
Steps to Incorporate Feedback Loops
Incorporating feedback loops into Smart QA systems is essential for leveraging Adaptive Feedback Learning effectively. To begin, data collection and analysis should prioritize the most pertinent customer interactions. By aggregating insights from various data sources, such as customer feedback and user behavior, teams can better understand areas for improvement. Detailed analysis of this data sets the stage for meaningful adjustments in the AI system.
๐ฌ Questions about Smart QA: AI Systems That Learn From Feedback Loops?
Our team typically responds within minutes
Next, loop integration and monitoring become crucial. Integrating feedback loops allows the AI to adapt based on real-time data, refining its algorithms for better accuracy. Regular monitoring of these loops ensures the system remains responsive to evolving user needs. This ongoing evaluation fosters continuous improvement, enabling the AI to not only learn but also enhance its performance consistently. Through these steps, organizations can create systems that are more aligned with user expectations.
- Step 1: Data Collection and Analysis
Data collection and analysis serve as the cornerstone of Adaptive Feedback Learning in Smart QA systems. Initially, organizations must gather relevant data to evaluate performance and customer interactions. This stage involves compiling both qualitative and quantitative data, such as user feedback, call transcripts, and survey responses. By systematically categorizing these inputs, insights emerge that reveal underlying patterns and challenges.
Once the data is collected, analysis takes center stage. Employing various analytical tools enables the identification of trends, common customer pain points, and evolving needs. This insight allows for meaningful interpretations, aiding organizations in making informed decisions. In essence, comprehensive data analysis drives improvements by fostering a better understanding of user experiences and expectations, setting the foundation for adaptive learning mechanisms that continually refine QA operations.
- Step 2: Loop Integration and Monitoring
Effective integration and monitoring of feedback loops are critical to the success of any Adaptive Feedback Learning system. This process involves establishing a robust framework where data and insights are not only collected but are also continuously evaluated and refined. By setting up appropriate channels for feedback collection, organizations can ensure that their AI systems evolve in response to user interactions and changing requirements.
Monitoring is equally vital, as it provides real-time visibility into system performance. Regular assessment helps identify areas for improvement, allowing developers to adjust algorithms and methods as necessary. This proactive approach to loop integration and monitoring cultivates a responsive AI environment. Ultimately, implementing these strategies leads to systems that not only learn from past experiences but also adapt swiftly to new challenges, enhancing overall user satisfaction and value.
Tools for Enhancing QA with Adaptive Feedback Learning
Adaptive Feedback Learning enhances quality assurance (QA) processes by integrating systematic feedback into AI systems. This methodology encourages continuous learning and improvement, allowing AI to refine its understanding based on real-world interactions. Various tools can facilitate this adaptive approach and streamline QA tasks effectively.
First, consider tools like TensorFlow and Keras, which allow for the development of robust machine learning models. These frameworks enable teams to train models on past feedback data, thus improving accuracy in future assessments. Additionally, H2O.ai offers a platform for rapid experimentation, helping organizations test and iterate models in real-time. Microsoft Azure Machine Learning provides a cloud-based environment that simplifies deployment and monitoring, ensuring that feedback loops are effectively integrated for ongoing optimization.
By leveraging these tools, organizations can create a QA ecosystem where AI continuously evolves, leading to enhanced accuracy and efficiency. Adaptive Feedback Learning transforms traditional QA into a dynamic process that benefits from ongoing insights and adjustments.
- insight7
In the realm of Smart QA, understanding Adaptive Feedback Learning is crucial for enhancing AI systems' efficiency. By establishing feedback loops, these systems can learn and adapt in real-time, continuously improving their response accuracy. This iterative process allows AI to not only analyze previous interactions but also to refine its algorithms based on user feedback, creating a dynamic learning environment. Consequently, businesses that leverage these advancements position themselves ahead of the competition.
The application of Adaptive Feedback Learning involves several key steps. Initially, data collection and analysis are paramount; it sets the foundation for understanding user needs. Following that, integrating feedback loops and establishing monitoring mechanisms ensure ongoing learning and improvement. These strategies result in AI systems that don't just respond reactively but also engage proactively with users, enhancing overall customer satisfaction and operational effectiveness. This approach fosters a deeper connection between consumers and AI, ultimately transforming the Smart QA experience.
- TensorFlow
TensorFlow serves as a powerful framework for developing AI systems, particularly those that thrive on Adaptive Feedback Learning. This versatile platform enables developers to create robust machine learning models, facilitating the continuous improvement of smart QA systems. Its flexibility allows for the integration of real-time feedback, making it possible to adjust the models dynamically based on user interactions.
One of TensorFlow's core strengths lies in its ability to process vast amounts of data efficiently. Developers can harness TensorFlow to create neural networks that learn from previous interactions, refining their outputs based on feedback loops. By utilizing such capabilities, smart QA systems can evolve, enhancing their accuracy and relevance to user needs. This not only optimizes performance but also fosters a more engaging user experience, ultimately laying the groundwork for intelligent, responsive AI solutions.
- Keras
Keras is a powerful and user-friendly deep learning framework designed to simplify the process of building neural networks. This makes it particularly valuable when developing AI systems aimed at Adaptive Feedback Learning. Developers can create and train models that can continuously learn from user inputs and enhance their performance over time. Such capabilities are vital for Smart QA systems that rely on constant feedback loops to improve accuracy and efficiency.
When using Keras, developers can leverage its modular architecture and intuitive API for straightforward model building. With features like automatic differentiation and optimized training routines, Keras allows rapid experimentation. This is crucial in refining models based on feedback, driving improvements in AI systems. By integrating Keras with workflows focused on adaptive learning, teams can harness data-driven insights, leading to smarter, more responsive quality assurance processes. Overall, Keras plays an essential role in nurturing robust AI systems that evolve through user interactions.
- H2O.ai
H2O.ai plays a pivotal role in the realm of Adaptive Feedback Learning within AI systems. This platform enables organizations to harness the power of feedback loops to refine their quality assurance processes. By utilizing diverse data sources and sophisticated algorithms, it empowers systems to learn continuously from user interactions. This adaptability allows AI to not only improve its performance but also tailor its analysis based on real-world usage.
At the heart of this technology is the integration of user feedback, which drives robust training models. As AI assesses ongoing performance and incorporates user insights, it evolves to meet the dynamic needs of businesses. Consequently, AI becomes more accurate, responsive, and aligned with organizational objectives. This journey of continuous improvement through adaptive learning showcases how insights transform AI capabilities, ultimately enhancing operational efficiency. By embracing such technology, organizations can foster a culture of innovation and responsiveness, ensuring that they remain competitive in an ever-changing landscape.
- Microsoft Azure Machine Learning
Microsoft Azure Machine Learning offers an advanced platform for developing intelligent systems powered by Adaptive Feedback Learning. By leveraging this robust toolset, developers can create models that refine their outputs based on feedback loops. Specifically, the platform facilitates continuous data analysis and model retraining. This iterative process enhances the ability of AI systems to learn from past interactions, improving accuracy and performance over time.
Moreover, Azure's machine learning capabilities allow for seamless integration with other tools, enabling experts to craft tailored solutions for their specific needs. Utilizing Azure can help streamline the feedback integration process, ensuring insights are captured and applied effectively. This approach not only raises the quality of smart QA systems but also fosters a culture of ongoing learning and adaptation, which is vital in today's fast-paced technological environment.
Conclusion: The Future of Smart QA with Adaptive Feedback Learning
The future of Smart QA lies in the transformative potential of Adaptive Feedback Learning. This innovative approach allows AI systems to adjust and evolve based on real-time feedback from users and data interactions. By embracing this learning paradigm, organizations can significantly enhance the quality and accuracy of their evaluations, moving towards a more intuitive and responsive quality assurance process.
As we continue to improve these systems, the integration of Adaptive Feedback Learning is set to redefine how AI interacts with human insights. This synergy will not only enhance performance but also foster a deeper understanding of user requirements, ultimately contributing to a more effective and reliable quality assurance ecosystem. Organizations that invest in this future will gain a competitive edge through continuous improvement and adaptability.
๐ฌ Questions about Smart QA: AI Systems That Learn From Feedback Loops?
Our team typically responds within minutes