Sentiment Text Analytics: Comprehensive Overview
-
Hello Insight
- 10 min read
Understanding Sentiment Insights Analysis is crucial for businesses striving to enhance customer engagement. Sentiment analysis goes beyond mere data interpretation; it unveils emotional tones and attitudes embedded in text. By systematically assessing customer feedback, organizations can gain valuable insights into public perception and sentiment trends.
Effective sentiment insights can drive impactful changes in marketing strategies, product development, and customer service. Emphasizing user-friendly approaches, the analysis democratizes access to critical insights, enabling every team member to glean valuable information. As we delve deeper into the techniques and applications, the transformative power of sentiment insights will become increasingly evident.
Generate visualizations from your qualitative data. At Scale.
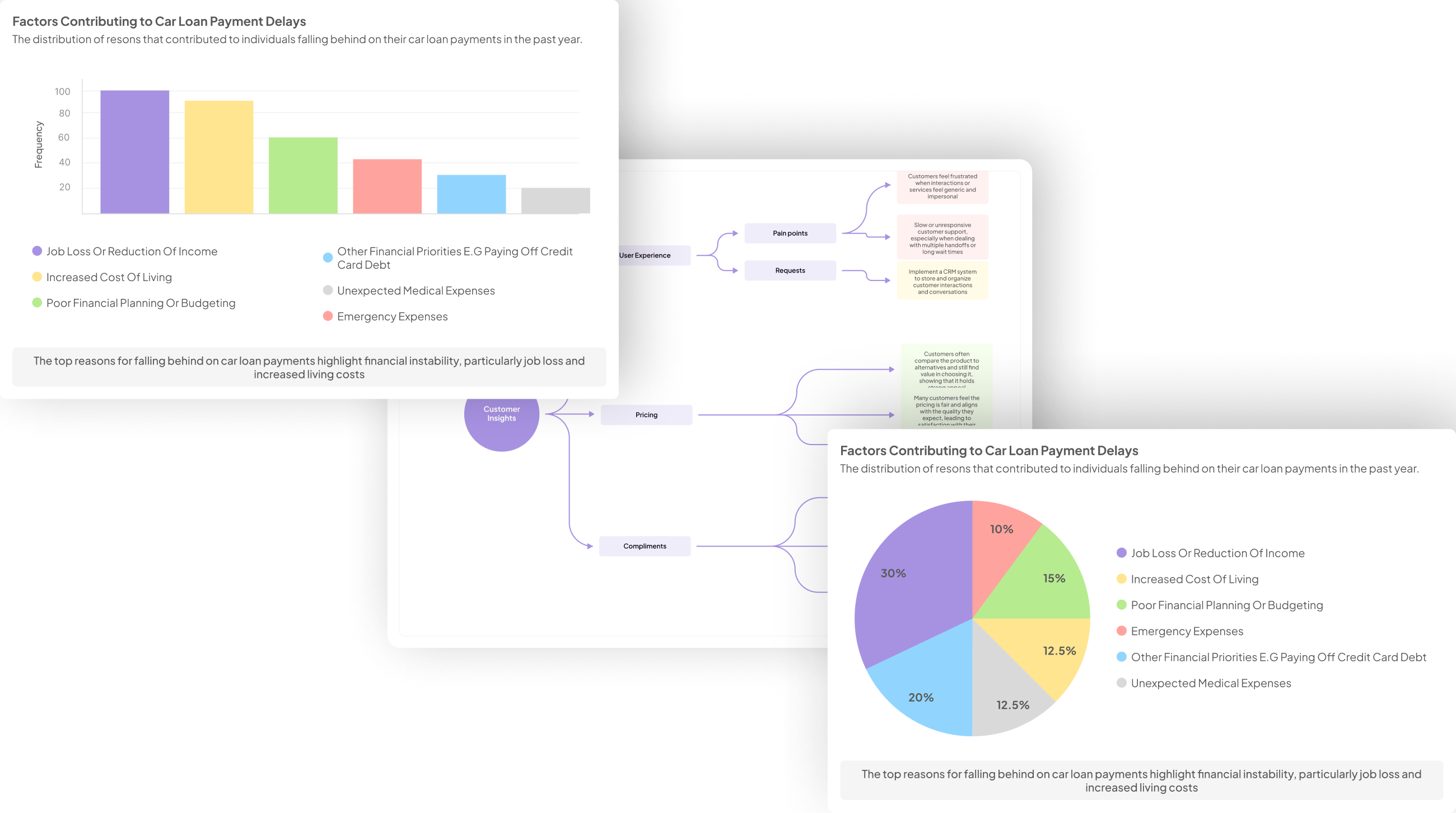
Exploring Sentiment Insights Analysis Techniques
Sentiment Insights Analysis techniques are essential for understanding the emotional tone behind consumer feedback. This analysis encompasses various approaches, each designed to extract valuable insights from large volumes of text. By identifying patterns in customer sentiment, businesses can make informed decisions that align with their audience's feelings and preferences.
Two primary categories of techniques exist: machine learning approaches and rule-based methods. Machine learning utilizes algorithms to classify sentiments based on training data, allowing for nuanced interpretations. Rule-based methods, on the other hand, rely on predefined linguistic rules to determine sentiment. Both techniques offer strengths and can be used in tandem for more comprehensive analysis. This exploration into sentiment techniques equips organizations with the tools needed to derive critical insights from their data, ultimately enhancing customer experiences and driving product improvements.
Machine Learning Approaches in Sentiment Insights Analysis
Machine learning approaches significantly enhance the accuracy and efficiency of sentiment insights analysis. By employing algorithms that can learn from data patterns, these techniques enable researchers to automatically classify sentiments from vast textual data. Common methods include supervised learning, where models are trained on pre-labeled datasets to identify sentiments as positive, negative, or neutral.
Furthermore, unsupervised learning plays a crucial role in discovering hidden patterns in unlabelled datasets, providing deeper insights into customer opinions. Natural language processing (NLP) techniques, such as tokenization and sentiment scoring, are integral to this analysis, allowing for a nuanced understanding of context and emotional tone. These machine learning methodologies not only streamline the data-processing pipeline but also offer valuable insights that can inform business decisions and product development strategies. As a result, adopting machine learning in sentiment insights analysis is essential for organizations aiming to stay ahead in understanding customer perspectives.
Rule-Based Methods for Sentiment Insights Analysis
Rule-based methods for sentiment insights analysis involve using predefined rules and lexicons to determine the sentiment of textual data accurately. This approach relies on a systematic set of guidelines that define which words, phrases, or patterns indicate positive, negative, or neutral emotions. Such methods are particularly useful for analyzing customer reviews or social media posts, where sentiments can influence decision-making processes.
The primary advantage of rule-based methods is their transparency and interpretability. Stakeholders can easily understand how different sentiments are categorized based on explicit rules. However, despite their strengths, these methods may struggle with nuances and context in language, leading to potential misinterpretations. As a result, while rule-based methods serve as a robust foundation for sentiment insights analysis, they are often complemented by more sophisticated machine learning techniques to enhance accuracy and capture complex sentiments.
Evaluate Performance on Customer Calls for Quality Assurance.
Applications of Sentiment Insights Analysis
Sentiment Insights Analysis offers a broad spectrum of applications that can significantly enhance various business functions. One of the critical areas is customer feedback. Companies can utilize sentiment data to analyze customer emotions and opinions, allowing them to refine products and services. By understanding positive and negative sentiments, organizations can make informed decisions that align with customer needs and expectations.
Social media also presents a valuable platform for sentiment insights. Through careful analysis of online conversations, businesses can gauge public perception and react to trends in real-time. This helps in brand management and enables companies to engage effectively with their audience. Social media sentiment analysis thus not only provides insights into customer loyalty but also uncovers potential opportunities for growth and market expansion.
Enhancing Customer Feedback through Sentiment Insights Analysis
Customer feedback is a vital component for businesses seeking to improve their offerings and enhance customer satisfaction. By harnessing sentiment insights analysis, organizations can extract valuable information from vast amounts of qualitative data such as reviews and surveys. This process enables companies to identify various sentiments—positive, negative, and neutral—associated with their products or services. Recognizing these patterns helps in understanding customer experiences more effectively and shaping future business strategies accordingly.
Furthermore, sentiment insights analysis offers targeted feedback interpretation. For instance, businesses can categorize comments to distinguish between specific aspects of their service that appeal to customers versus those that require improvement. This systematic approach allows for actionable insights that can drive enhancements in product development and customer service. Consequently, the implementation of sentiment insights analysis not only boosts customer loyalty but also fosters a culture of continuous improvement by aligning business offerings with customer expectations.
Leveraging Social Media Sentiment Insights Analysis
Analyzing sentiment insights from social media can yield valuable information regarding public opinion and consumer behavior. Social media platforms serve as vast repositories of user-generated content, where emotions are expressed openly. By mining this data, businesses can uncover trends related to customer sentiments, identify potential areas for improvement, and track the effectiveness of their marketing strategies. Understanding these sentiments helps organizations tailor their offerings to meet audience expectations effectively.
To effectively analyze social media sentiment, it’s essential to consider several key aspects. First, employ robust data collection methods to gather relevant comments from various platforms, ensuring a comprehensive dataset. Next, utilize advanced text analytics tools to interpret emotional nuances within the comments, enabling more precise insights. Finally, visualizing this data can help stakeholders easily comprehend the overarching trends and behaviors that emerge from user interactions. By systematically harnessing social media sentiment insights, organizations can make informed decisions and enhance their market strategies.
Conclusion: The Future of Sentiment Insights Analysis
As we look to the future of sentiment insights analysis, it's clear that advancements in technology will drive innovation in this field. Enhanced algorithms and machine learning techniques will allow for more accurate sentiment detection and greater context understanding, enabling businesses to interpret consumer emotions more precisely. This evolution will empower organizations to shape their strategies based on nuanced customer feedback.
Moreover, integrating sentiment insights analysis with other analytical tools will create a comprehensive understanding of market trends. The role of real-time data will become vital, providing timely insights to inform decisions. Companies that embrace these innovations will be better positioned to meet changing consumer needs and gain a competitive edge in their industries.