Sentiment Analysis: Extracting Meaning from Words
-
Bella Williams
- 10 min read
Emotion Detection serves as a key component in the field of sentiment analysis, allowing researchers and businesses to go beyond simple positive or negative classifications. By identifying underlying emotions in text, such as joy, anger, or sadness, we can gain deeper insights into consumer opinions and behaviors. This understanding is crucial in today's digital age, where the volume of user-generated content continues to rise at an unprecedented rate.
As we explore the relationship between sentiment analysis and Emotion Detection, it becomes clear that effectively harnessing these techniques can empower organizations. Companies can refine their marketing strategies, enhance customer experiences, and ultimately drive loyalty by deciphering the rich emotional tapestry within consumer data. This introduction serves as the foundation for a comprehensive exploration of how emotion detection transforms the landscape of sentiment analysis, unlocking its full potential for insightful decision-making.
Analyze & Evaluate Calls. At Scale.
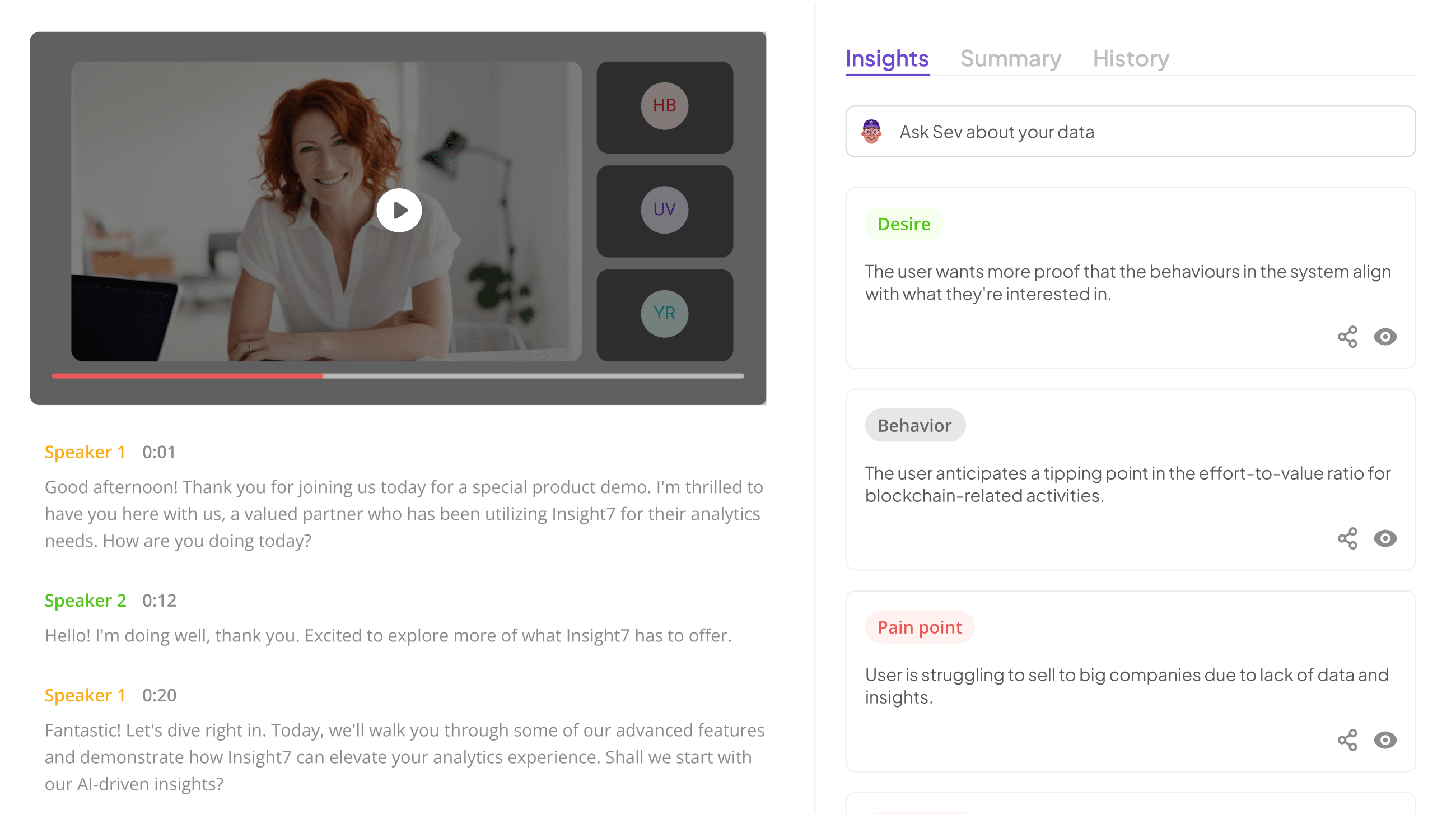
The Role of Emotion Detection in Sentiment Analysis
Emotion detection plays a pivotal role in sentiment analysis, enhancing the way we interpret text data. By accurately identifying emotions, analysts can glean deeper insights into customer feedback and opinions. This process goes beyond merely categorizing sentiments as positive or negative. Instead, it reveals the underlying emotions that drive consumer behavior, enriching our understanding of the data.
Effective emotion detection involves several steps. First, it requires robust data collection methods, pulling information from diverse sources like social media, product reviews, and forums. Next, preprocessing the collected text ensures data is cleaned and ready for analysis. Finally, advanced algorithms analyze both sentiments and emotions, allowing businesses to tailor their strategies based on nuanced emotional insights. By harnessing emotion detection, organizations can significantly enhance their sentiment analysis, ultimately leading to better customer engagement and decision-making.
Understanding Sentiment Analysis
Sentiment analysis has become essential in interpreting human emotion in the vast expanse of digital communication. By utilizing advanced algorithms, this process enables businesses and researchers to examine and understand user-generated content, from social media posts to product reviews. The key to successful sentiment analysis lies in effectively detecting emotions within the text, which allows organizations to gauge audience reactions and sentiments accurately.
In the realm of emotion detection, itโs crucial to grasp various layers of meaning behind words. Emotion detection can differentiate between positive, negative, and neutral sentiments while also pinpointing specific feelings like joy, anger, or sadness. This multifaceted analysis offers invaluable insights into consumer behavior and preferences, guiding companies in making informed decisions based on authentic user sentiment. By grasping the nuances of sentiment analysis, organizations can better connect with their audiences and tailor their offerings to meet genuine customer needs.
- Define sentiment analysis and its relevance in todays digital world.
Sentiment analysis is the computational approach to determining the emotional tone behind a series of words. In todayโs digital world, where consumers express their thoughts on various platforms, sentiment analysis plays a pivotal role. Businesses harness this tool to interpret the emotional nuances of customer feedback, social media interactions, and online reviews. With the continuous growth of digital communication, the capacity to gauge public sentiment is crucial for brand strategies and decision-making.
Understanding sentiment analysis enhances our ability to discern emotions from textual data, known as emotion detection. This becomes essential for businesses dodging potential pitfalls and amplifying positive engagement. For instance, a product release may be monitored to extract valuable insights concerning user sentiment, providing essential feedback that shapes future marketing and product development strategies. Therefore, sentiment analysis not only aids in understanding current consumer emotions but also informs future actions to align with public sentiment effectively.
- Explain the relationship between sentiment analysis and emotion detection.
Sentiment analysis and emotion detection are closely related but distinct concepts in the realm of understanding human language. While sentiment analysis focuses on classifying text as positive, negative, or neutral, emotion detection delves deeper by identifying specific emotions like joy, anger, or sadness. This relationship is significant because accurate sentiment analysis often relies on understanding the nuanced emotions expressed within the text.
For effective sentiment analysis, it is beneficial to incorporate emotion detection as part of the process. Emotion detection enriches the sentiment analysis by providing a more comprehensive view of the sentiments being expressed. For instance, a text that reads as positive may still reveal underlying emotions such as nostalgia or frustration. Thus, integrating both methods enables researchers and organizations to glean richer insights from user-generated content, enhancing decision-making and communication strategies. In todayโs data-driven environments, understanding this relationship ultimately leads to more informed interpretations and responses to public sentiment.
Steps to Implement Emotion Detection in Sentiment Analysis
To implement emotion detection in sentiment analysis effectively, one must follow certain essential steps. Begin with data collection, which involves gathering text data from various sources such as social media platforms, customer reviews, and user-generated content. This diverse data set forms the foundation for understanding the sentiments and emotions of users.
Next, move on to preprocessing the text data. This step is crucial for cleaning and preparing the data for analysis. Techniques such as removing irrelevant information, correcting typos, and standardizing language ensure that the analysis yields accurate results. Finally, in the analyzing sentiments and emotions stage, employ algorithms designed for sentiment classification and emotion detection. These algorithms analyze the context, tone, and semantics of the text, allowing you to derive insights about usersโ emotional states.
These steps not only enhance the understanding of customer opinions but also improve decision-making processes based on emotional insights.
- Step 1: Data Collection
To initiate the process of emotion detection, collecting data is of paramount importance. It enables us to analyze text and extract meaningful sentiments and emotions from it. Begin by gathering diverse data from various sources, such as social media platforms, online reviews, and customer feedback forms. This multi-source approach ensures a comprehensive understanding of the emotional landscape and provides a rich dataset for analysis.
Once data is collected, it is critical to ensure its relevance and quality. The emotions we extract from this data will significantly impact the outcomes of our sentiment analysis. By focusing on quality, we can improve the precision of emotion detection, thereby enabling organizations to make informed decisions based on genuine user sentiment. A well-rounded dataset forms the foundation for successful analysis and will help uncover insights that drive strategic improvements.
Gather text data from various sources like social media, reviews, etc.
To successfully implement emotion detection in sentiment analysis, the first crucial step is to gather text data from diverse sources. This process can include social media platforms, customer reviews, blogs, and forums. Each of these sources provides a wealth of information that captures stakeholders' emotions and sentiments regarding products or services. Social media, in particular, offers real-time data; posts, comments, and shares can reflect usersโ immediate feelings and opinions. Similarly, reviews on platforms like Amazon or Yelp can highlight both praise and criticism, which are critical for understanding customer sentiments.
Once the data is collected, it becomes essential to preprocess it for analysis. This entails cleaning the text to remove noise, such as irrelevant characters or stop words, and organizing it in a format suitable for emotion detection. By doing so, businesses can effectively analyze the sentiments expressed in the gathered data. This approach ultimately aids in discerning patterns and trends that can guide decision-making and enhance customer engagement strategies.
- Step 2: Preprocessing Text Data
To efficiently perform Emotion Detection, preprocessing text data is crucial in the sentiment analysis process. This fundamental step involves cleaning and preparing raw text to ensure accurate analysis. Initially, text data may contain noise, such as punctuation, irrelevant symbols, and varying formats. Eliminating these distractions will help enhance the quality of analysis.
The preprocessing phase typically includes several key actions. First, text normalization ensures consistency; methods like lowercasing all text or removing special characters are common practices. Next, tokenization breaks down text into meaningful units, like words or phrases. Stemming and lemmatization further refine these tokens to their base forms, which is particularly important for capturing emotion in various contexts. Finally, removing stopwords helps eliminate common but uninformative terms, allowing the model to focus on the words that truly convey sentiment. Proper preprocessing establishes a solid foundation for effective emotion detection, enabling subsequent analysis to be more robust and insightful.
Clean and prepare the text data for analysis.
Preparing your text data is crucial for effective analysis in emotion detection. The first step involves gathering text from various sources like social media, customer reviews, and forums. However, raw text can be unstructured and noisy, requiring a thorough cleansing process. This includes removing irrelevant information, such as URLs and special characters, while also standardizing formats, such as converting all text to lowercase.
Next, itโs essential to tokenize the text, breaking it into smaller components like words and phrases to enable more nuanced sentiment analysis. Stop wordsโcommon words that add little meaningโshould be removed to enhance the relevance of the remaining data. Additionally, stemming and lemmatization might be applied to reduce words to their base forms, ensuring that variations of a word are classified consistently. By effectively cleaning and preparing the text data, you set the stage for accurately detecting emotions and sentiments, ultimately enhancing the quality of your analysis.
- Step 3: Analyzing Sentiments and Emotions
In this step, we delve into the pivotal aspect of analyzing sentiments and emotions, which is essential for understanding the underlying feelings in textual data. By employing advanced algorithms, we can effectively interpret emotions conveyed in various types of written content, such as reviews and social media interactions. This process helps identify whether the sentiment is positive, negative, or neutral, opening pathways to meaningful insights.
To facilitate a thorough analysis, it is crucial to implement strategies that include sentiment scoring and emotional categorization. First, classify sentiments based on pre-defined parameters, categorizing them into distinct emotional states like joy, anger, or sadness. Next, enhance the analysis by visualizing these emotions across datasets to uncover patterns and trends. By mastering these techniques in emotion detection, analysts can transform simple textual data into valuable insights, ultimately aiding in decision-making processes and improving customer engagement.
Utilize algorithms to determine the sentiment and detect emotions within the text.
Utilizing algorithms to assess sentiment and detect emotions in text is a critical component of modern sentiment analysis. Algorithms harness natural language processing to scrutinize and interpret human emotions within written communication. These algorithms categorize text into various sentiment classes such as positive, negative, or neutral, enabling us to gauge public sentiment accurately.
To effectively implement emotion detection in sentiment analysis, there are several steps to consider. First, data collection is essential; gathering text from sources like social media and online reviews ensures a diverse dataset. Next, preprocessing the text data prepares it for analysis by cleaning and organizing the information. Finally, the core analysis phase employs sophisticated algorithms to derive insights not just about sentiment but also specific emotions expressed in the text, such as joy, anger, or sadness. Embracing these technologies allows organizations to respond more effectively to their audience's feelings and expectations, enhancing engagement and strategy.
Extract insights from interviews, calls, surveys and reviews for insights in minutes
Tools for Emotion Detection and Sentiment Analysis
Emotion Detection plays a pivotal role in understanding sentiment analysis and interpreting how individuals feel about specific topics or brands. Various tools have emerged to enhance this practice, offering innovative ways to decipher emotions from textual data. The right tools can analyze sentiments accurately, providing insights into customer opinions and preferences.
Several noteworthy tools excel in emotion detection. Insight7 is designed for comprehensive analysis, capable of extracting nuanced emotional data from user-generated content. MonkeyLearn utilizes machine learning to perform real-time text analysis, while Lexalytics offers robust sentiment analysis with powerful emotion detection features. Aylien specializes in tracking sentiments from news and social media, further broadening the scope of analysis. Lastly, IBM Watson Natural Language Understanding employs advanced AI techniques to assess emotions embedded in web content. Selecting the appropriate tool involves considering the scale and complexity of your data source, ensuring effective sentiment analysis tailored to your needs.
Top Tools for Emotion Detection
Emotion Detection tools play a crucial role in enhancing sentiment analysis by providing insights into feelings expressed in textual data. Understanding the emotional tone helps businesses refine their strategies based on customer feedback. Some of the top tools in the market are designed to deliver precise and nuanced emotion detection, allowing for an enriched comprehension of user sentiments.
Firstly, Insight7 stands out as an advanced tool that offers comprehensive emotion detection capabilities, making it suitable for various industries. Secondly, MonkeyLearn employs machine learning algorithms to analyze text in real-time, allowing immediate emotional insights. Another noteworthy tool is Lexalytics, which excels in delivering deep sentiment analysis alongside advanced emotion detection functions. Aylien focuses on providing sentiment tracking through news and social media platforms, emphasizing recognition of complex emotional cues. Lastly, IBM Watson Natural Language Understanding utilizes AI to parse web content emotions effectively, helping businesses make informed decisions. Selecting the right tool involves evaluating your specific needs regarding the data's scale and complexity, ensuring optimal results.
- Insight7
Emotion detection is a critical aspect that enhances the capability of sentiment analysis. By understanding emotional cues within text, businesses can gain valuable insights that drive decision-making processes. In a world where consumer feedback is abundant, accurately identifying emotions helps organizations respond more effectively to their audienceโs needs.
To successfully implement emotion detection, itโs essential to follow a structured approach. First, collect diverse text data from sources like social media, reviews, and interviews. This rich dataset allows for a more comprehensive understanding of public sentiment. Next, preprocessing the data is vital to ensure itโs clean and ready for detailed analysis. This step includes removing noise and irrelevant information. Finally, applying appropriate algorithms for analyzing sentiments and emotions allows businesses to extract meaningful insights, shaping their strategies and improving customer engagement.
By focusing on emotion detection, organizations can create deeper connections with their customers and remain competitive in todayโs dynamic market.
๐ฌ Questions about Sentiment Analysis: Extracting Meaning from Words?
Our team typically responds within minutes
An advanced tool for comprehensive emotion detection and sentiment analysis.
An advanced tool for comprehensive emotion detection and sentiment analysis is essential for businesses looking to understand customer feedback effectively. This tool harnesses cutting-edge algorithms to analyze text data from various sources, such as social media posts, customer reviews, and interview transcripts. By doing so, it identifies nuanced emotional responses, allowing organizations to grasp not just what customers think but also how they feel.
The process begins with data collection, where diverse text inputs are sourced. This wealth of information is then preprocessed to ensure clarity and readiness for analysis. The powerful algorithms come into play in analyzing sentiments and detecting emotions, producing actionable insights. By employing such advanced technology, companies can uncover pain points and customer desires, leading to improved experiences and business strategies. Ultimately, effective emotion detection is a cornerstone of modern sentiment analysis, fostering a deeper connection between businesses and their customers.
- MonkeyLearn
In the realm of sentiment analysis, the significance of advanced tools becomes evident. One such tool excels in providing robust emotion detection capabilities, transforming the way businesses interact with their customers. Through its machine learning features, it can analyze text data in real-time, allowing organizations to gather insights from customer feedback, social media, and more.
As companies increasingly seek to understand customer sentiments, this tool stands out by automating the emotion detection process. It accurately identifies various emotions such as happiness, anger, and sadness by examining word choices and context. By integrating these analyses into organizational workflows, businesses can measure emotional responses, driving informed decision-making and enhancing customer experiences. Using this powerful tool allows for a deeper comprehension of customer sentiments and emotions, ultimately fostering stronger connections and better outcomes in competitive marketplaces.
Features machine learning for real-time text analysis and emotion detection.
Machine learning plays a pivotal role in real-time text analysis and emotion detection, changing how we interpret language. By utilizing advanced algorithms, these systems can instantly process large volumes of text, extracting crucial emotional indicators and insights. Emotion detection allows organizations to understand customer sentiment deeply, which is essential for improving products and services.
One significant feature of machine learning is its ability to adapt and learn from new data, continually enhancing the quality of emotion detection. For example, as more conversational data is fed into the system, it improves its accuracy in identifying subtle emotional cues. Additionally, the capability to analyze text in real-time means businesses can respond swiftly to customer feedback and adjust strategies accordingly. Overall, employing machine learning in text analysis not only streamlines data processing but also enriches user experience through better understanding and engagement.
- Lexalytics
In the realm of sentiment analysis, the choice of tools plays a pivotal role, and one such tool is particularly noteworthy for its focus on emotion detection. This platform streamlines the process of identifying key emotional indicators from text data, allowing users to gain deeper insights into customer sentiments. By harnessing advanced algorithms, it can analyze vast amounts of text data to reveal powerful emotional nuances that traditional analysis might overlook.
The platform excels at transforming raw data into actionable insights. With features designed for ease of use, it empowers anyone in an organization to access critical information without specialized knowledge. Users can gather feedback, identify pain points, and gauge overall customer experience, all while discerning underlying emotions. This capability is essential for organizations aiming to enhance customer satisfaction and brand loyalty through informed decision-making. By integrating emotion detection within their analysis processes, businesses can unlock a new dimension of understanding that drives meaningful engagement.
Offers in-depth sentiment analysis with powerful emotion detection capabilities.
Emotion detection stands as a pivotal element in the realm of sentiment analysis, transforming raw text into meaningful insights. By utilizing sophisticated algorithms, this process encompasses the identification and categorization of emotions conveyed in various textual forms, from social media posts to customer reviews. Emotion detection enables organizations to grasp not just the sentiment behind the words but also the underlying feelings that drive customer behavior. This capability is essential for accurate decision-making and effective communication strategies.
In-depth sentiment analysis, combined with powerful emotion detection, enriches the understanding of customer feedback and interactions. Businesses can identify pain points, desires, and compliments by analyzing conversations. This, in turn, allows for tailored responses and enhanced customer experiences. Embracing such technology is crucial in todayโs data-driven landscape, where being attuned to customer emotions can significantly impact brand loyalty and overall success. Through diligent implementation, organizations can unlock the true power of their data.
- Aylien
Aylien serves as a powerful platform for analyzing sentiments within text, particularly from sources like news and social media. By using advanced algorithms, this tool enables users to not only track the sentiment but also recognize emotions embedded within the text. This capability is crucial in today's fast-paced digital world where understanding customer sentiment is key to making informed business decisions.
Leveraging Aylien for emotion detection involves several steps. First, users can input extensive text datasets to gather insights. Next, the platform preprocesses this data, cleaning it for effective analysis. Finally, Aylien applies its state-of-the-art algorithms to analyze the sentiments and unveil the emotions, delivering valuable insights. By employing such a tool, you position yourself to better comprehend audience reactions, helping drive successful strategies tailored to meet customer needs.
Provides news and social media sentiment tracking with emotion recognition.
Analyzing news and social media sentiment is crucial for understanding public perception. By tracking emotional responses, businesses can gauge how their brand is perceived in real-time. Employing robust tools for sentiment analysis allows organizations to monitor a multitude of data sources simultaneously. This process transforms raw data into valuable insights, guiding strategic decision-making.
To effectively implement news and social media sentiment tracking with emotion recognition, consider the following points:
Diverse Data Sources: Collect data from various platforms such as Twitter, Facebook, and news websites. This diversity ensures a comprehensive understanding of sentiment across different audiences.
Emotion Detection Algorithms: Utilize advanced algorithms that can not only determine sentiment polarity but also recognize subtle emotional nuances like joy, anger, or sadness. This enhances the depth of analysis.
Real-Time Monitoring: Establish a system for live sentiment tracking. This enables businesses to respond promptly to potential crises or capitalize on positive sentiment trends.
By integrating these elements, organizations can gain a nuanced understanding of their audience's feelings, paving the way for informed marketing strategies and improved customer relationships.
- IBM Watson Natural Language Understanding
IBM Watson Natural Language Understanding provides a powerful platform for emotion detection within sentiment analysis. This tool uses advanced AI algorithms to analyze textual content, enabling users to extract nuanced emotions from written communication. By understanding the emotional tone of text, businesses can gain valuable insights into customer opinions and behaviors.
To effectively implement emotion detection, it is crucial to grasp the underlying principles of sentiment analysis. The platform allows for granular sentiment scoring, highlighting not just positive or negative sentiments but also a range of emotions such as joy, anger, or sadness. Users can leverage these insights to refine marketing strategies or enhance customer engagement. The ability to decode emotional context enables organizations to respond thoughtfully to customer feedback, ultimately fostering stronger relationships and improved satisfaction.
Leveraging emotion detection capabilities can significantly enhance business strategies by offering a deeper understanding of audience sentiments.
Utilizes AI to analyze web content emotions and sentiments.
AI plays a pivotal role in understanding and analyzing web content emotions and sentiments. By employing advanced algorithms, AI systems can sift through vast amounts of text, whether from social media, reviews, or articles, to extract meaningful emotional insights. Emotion detection allows organizations to gauge public sentiments effectively, pinpointing not just whether expressions are positive or negative, but also uncovering underlying emotions such as joy, anger, or sadness.
One of the critical steps in this process includes preprocessing the gathered data, which involves cleaning and formatting text for analysis. Once this is complete, AI algorithms analyze the sentiments expressed, classifying them based on emotional frameworks. This comprehensive analysis provides organizations with actionable insights that can steer decision-making and enhance customer experiences. By effectively utilizing AI for emotion detection, businesses can stay in tune with consumer feelings, adapting their strategies accordingly.
Choosing the Right Tool for Your Needs
When considering emotion detection for sentiment analysis, choosing the right tool is crucial. Start by assessing your specific needs. Think about the scale of your project and the complexity of the text data you are working with. For example, if your focus is on social media monitoring, you may require a tool that handles real-time analysis effectively.
Next, evaluate the features offered by each tool. Some tools come equipped with advanced emotion detection algorithms, while others may provide basic sentiment scoring. Itโs essential to match these capabilities with your goals. Additionally, consider factors such as user interface, customer support, and integration options. Ultimately, making the right choice will empower you to extract meaningful insights, leading to better decision-making and enhanced understanding of customer sentiments. With the right tool in hand, you elevating your sentiment analysis efforts effectively.
- Consider the scale, complexity, and type of text data when selecting sentiment analysis tools.
When choosing sentiment analysis tools, it is crucial to consider the scale, complexity, and type of text data involved. Different tools excel in various contexts, so understanding your specific needs will help you find the right solution. For instance, if you are managing large volumes of data from social media, you may require tools that specialize in real-time analytics. Conversely, simpler datasets may allow for tools that focus on straightforward sentiment analyses without extensive features.
The complexity of your specific text data also plays a significant role. Analyzing informal language from social media requires different capabilities compared to formal reviews or legal documents. Additionally, various types of text data, such as verbal transcripts or written feedback, may necessitate tools with advanced emotion detection features. Therefore, assess each toolโs ability alongside your text dataโs unique traits for effective sentiment analysis.
Conclusion: The Future of Sentiment Analysis and Emotion Detection
The future of sentiment analysis and emotion detection holds tremendous potential as technology continues to evolve. As machine learning algorithms become more sophisticated, they will enhance our ability to analyze varying emotional states in text. This advancement will facilitate improved understanding of customer sentiments, allowing businesses to tailor their approaches more effectively.
As we move forward, the integration of emotion detection into sentiment analysis will play a pivotal role across numerous sectors. From marketing strategies to mental health applications, the insights gleaned from analyzing sentiments can lead to more informed decisions and deeper connections with users. Embracing these innovations will undoubtedly shape the way organizations interpret and respond to emotional cues in communication.
๐ฌ Questions about Sentiment Analysis: Extracting Meaning from Words?
Our team typically responds within minutes