AI Thematic Automation transforms the way analysts engage with data, significantly reducing the time spent on manual analysis. By automating thematic grouping, it enables analysts to quickly identify patterns and insights from extensive datasets. This innovation not only streamlines workflows but also enhances decision-making processes, allowing organizations to react swiftly to emerging trends.
As the demand for real-time insights grows, AI Thematic Automation serves as a crucial tool for analysts. It allows them to focus on more strategic activities rather than getting bogged down by repetitive tasks. By harnessing advanced algorithms, organizations can ensure that valuable insights are readily available, paving the way for timely and informed business decisions.
Analyze qualitative data. At Scale.
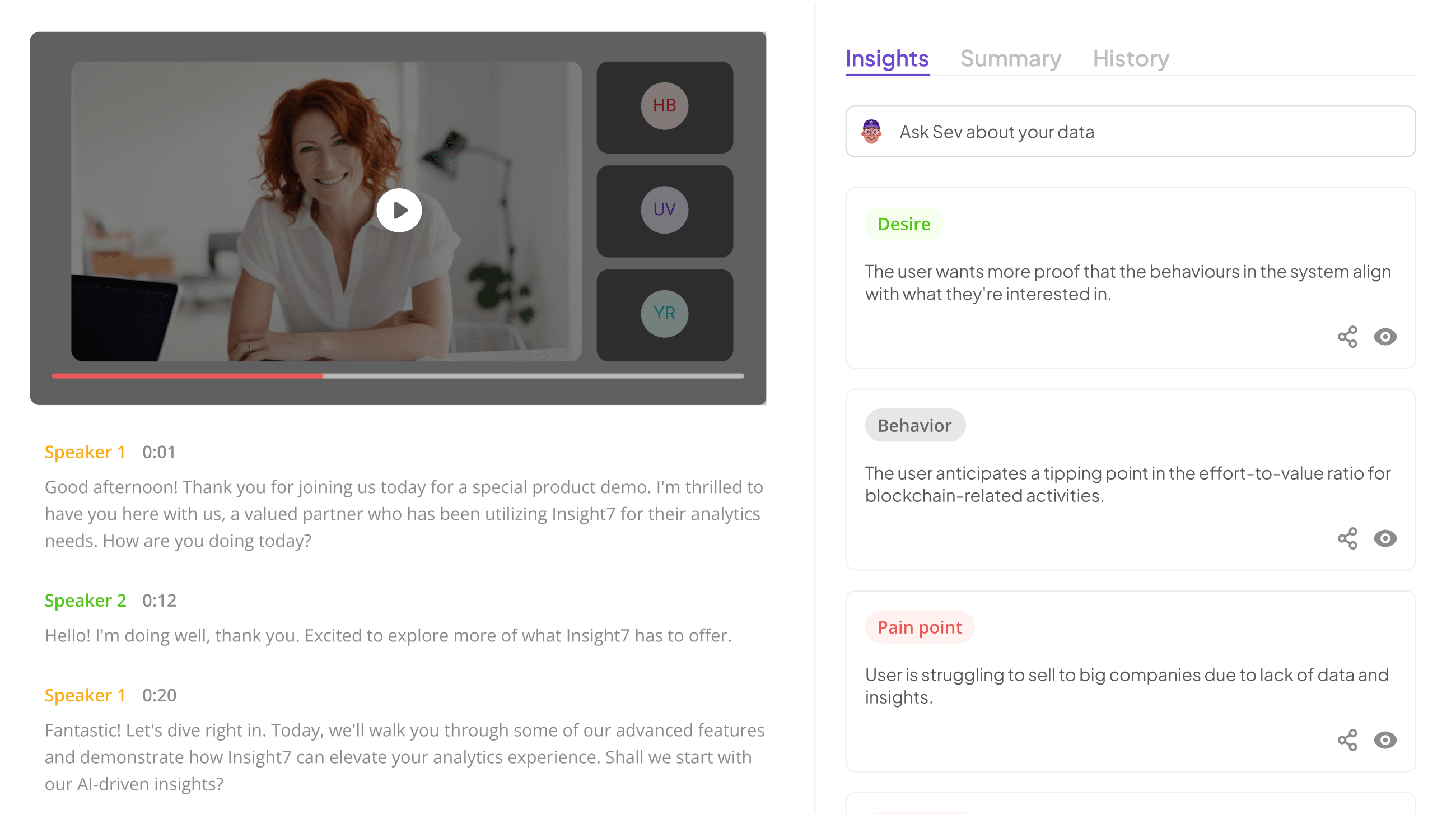
Understanding the Basics of AI Thematic Automation
AI Thematic Automation serves as a powerful tool for enhancing efficiency in thematic grouping processes. It operates by using machine learning algorithms to analyze large datasets, identifying patterns and trends that human analysts might overlook. The ability to automate this process significantly reduces the time spent on manual analysis, leading to quicker insights and decision-making.
In its essence, AI Thematic Automation works by categorizing data into coherent themes, allowing analysts to focus on strategic interpretation rather than time-consuming sorting. It enables precise grouping based on specified parameters, ensuring clarity in the data collection and analysis. By streamlining thematic analysis, organizations can not only enhance productivity but also improve the quality of insights derived from complex datasets. Embracing this technology equips analysts with the ability to uncover actionable insights more efficiently, ultimately driving better business outcomes.
The Need for Efficient Thematic Grouping
Efficient thematic grouping is crucial for reducing the time analysts spend on data interpretation. As information volumes grow, the complexity of categorizing themes increases significantly. Analysts often find themselves overwhelmed, sifting through vast amounts of data to identify patterns, trends, and actionable insights. This bottleneck can lead to missed opportunities and hinder decision-making processes.
AI Thematic Automation addresses these challenges by employing advanced algorithms to streamline the grouping of data. By automating the identification and categorization of themes, analysts can focus on higher-level strategic thinking rather than repetitive tasks. Effective thematic grouping not only accelerates analysis but also enhances the accuracy of insights derived from data. This becomes particularly valuable as organizations strive to be data-driven, ensuring they remain competitive in their respective fields.
How AI Thematic Automation Streamlines Analysis
AI Thematic Automation optimizes the analysis process by systematically grouping data based on underlying themes. This automation enables analysts to focus on high-value insights rather than getting bogged down by manual tasks. The automated approach allows for quick identification of patterns and trends, facilitating efficient decision-making.
Moreover, AI Thematic Automation enhances report generation by summarizing large datasets into digestible insights. Analysts can visualize key themes and pain points easily, allowing stakeholders to grasp essential information swiftly. By transforming qualitative data into quantifiable outputs, the automation streamlines workflows, reducing the time required for analysis. Consequently, organizations can respond more rapidly to market changes, empowering teams to make informed decisions based on clear, actionable insights.
Extract insights from interviews, calls, surveys and reviews for insights in minutes
Tools for Implementing AI Thematic Automation
To effectively implement AI thematic automation, it's essential to select the right tools that can enhance thematic grouping processes. Various AI tools are available, each designed to streamline data analysis and improve insights. These tools utilize advanced algorithms to efficiently categorize information based on predefined themes, significantly reducing the time analysts spend sifting through data.
Utilizing tools like insight7, MonkeyLearn, Lexalytics, IBM Watson Natural Language Understanding, and Google Cloud Natural Language can help organizations develop a streamlined workflow for thematic grouping. Each tool offers unique features that can be tailored to specific needs, ensuring a comprehensive approach to data management. Furthermore, incorporating AI thematic automation allows teams to focus on strategic decision-making rather than manual data analysis, ultimately fostering more productive outcomes. Making the right choice will cause a shift in operational efficiency and greatly minimize the time analysts require for thorough data analysis.
Top AI Tools for Thematic Grouping
AI Thematic Automation plays a crucial role in expediting the process of thematic grouping, helping reduce the time analysts spend on data analysis. To effectively implement this automation, various AI tools are available that can streamline tasks and enhance accuracy. Below are the top AI tools for thematic grouping:
insight7: This tool integrates intuitive interfaces and advanced analytics. It organizes data, identifies themes, and generates insightful reports, simplifying complex analyses.
MonkeyLearn: Ideal for text analysis, MonkeyLearn allows users to categorize data flexibly. Its user-friendly platform enables real-time insights and customizable features.
Lexalytics: Focusing on natural language understanding, Lexalytics excels at extracting sentiments and themes from vast text datasets, facilitating deeper insights into customer opinions.
IBM Watson Natural Language Understanding: This powerful tool uses AI to mine unstructured data for valuable themes, crucial for businesses looking to analyze conversational data efficiently.
Google Cloud Natural Language: Known for its scalability and robust capabilities, Google’s offering enables automated theme extraction from documents and other text sources, enhancing the analytic process.
These tools help analysts focus on strategic insights rather than data processing, ultimately leading to improved decision-making and efficiency.
- insight7
In the realm of data analysis, insight7 exemplifies how AI thematic automation can dramatically reduce analyst workload. Traditionally, analysts faced challenges sifting through vast amounts of customer conversation data, which was often time-consuming and inefficient. The introduction of AI-driven tools facilitates the identification and grouping of themes within this data, enabling analysts to focus on drawing actionable insights rather than getting bogged down in the minutiae.
By streamlining the thematic grouping process, AI thematic automation not only quickens analysis but also enhances the quality of insights generated. This method helps organizations leverage real-time customer signals, ensuring that they stay ahead of competitors who still rely on outdated analytical methods. With AI handling the heavy lifting in data categorization, analysts can now deliver insights more quickly and effectively, ultimately driving better decision-making across the business landscape.
- MonkeyLearn
AI Thematic Automation has transformed how businesses analyze and interpret vast amounts of data. One key player in this space utilizes advanced algorithms to automate thematic grouping, allowing analysts to focus on strategic decision-making rather than getting bogged down by data overload. This platform harnesses natural language processing (NLP) techniques, making it easier for organizations to uncover insights from customer feedback, market research, and social media.
The innovative features provided support seamless integration, enabling users to upload data and customize analysis parameters effortlessly. Additionally, its intuitive interface simplifies the process, ensuring that even teams with limited technical expertise can utilize its capabilities effectively. By minimizing the time spent on manual categorization, teams can allocate more resources towards understanding market trends and customer needs, ultimately enhancing overall productivity. Embracing this AI technology is vital for organizations looking to streamline their analytic processes and extract valuable insights efficiently.
- Lexalytics
The AI Thematic Automation revolutionizes data analysis by simplifying the thematic grouping process. By automating data handling, businesses can gain insights without requiring extensive training or expertise. This ease of use democratizes access to key information, allowing employees at all levels to interact with data effectively. Here, analysts can quickly turn multiple conversations into meaningful reports, revealing trends and pain points that inform decision-making.
A powerful feature of this automation is its ability to process vast amounts of data simultaneously. Analysts can gather insights from hundreds of calls at once, efficiently analyzing themes and extracting crucial evidence with minimal manual effort. By streamlining this process, organizations drastically reduce the time spent on traditional analysis methods, leaving more room for strategic activities. Ultimately, the focus on AI Thematic Automation enhances productivity and fosters a data-driven culture within any organization.
- IBM Watson Natural Language Understanding
AI Thematic Automation significantly transforms how organizations approach data analysis, and one of the most effective tools available is Natural Language Understanding (NLU). NLU technology enables systems to comprehend and analyze human language effectively, making it easier to extract themes and insights from large datasets. This capability can drastically reduce the time analysts spend on manual thematic grouping, allowing them to focus on more strategic tasks.
By employing advanced algorithms, NLU can quickly identify recurring themes in unstructured data. This means that analysts can receive insights within minutes, rather than hours or days. The automation of thematic grouping not only improves efficiency but also enhances the accuracy of reports and findings. As organizations increasingly rely on data-driven decision-making, AI Thematic Automation becomes essential in streamlining processes and optimizing resource allocation. It represents a vital shift in how data intelligence is harnessed, ultimately promising higher productivity and better outcomes for businesses.
- Google Cloud Natural Language
Google Cloud Natural Language offers robust capabilities that can significantly enhance AI Thematic Automation for thematic grouping tasks. By harnessing advanced natural language processing (NLP) techniques, it enables organizations to analyze extensive text data efficiently. With the ability to extract insights, identify sentiment, and categorize documentation, this tool empowers analysts to focus on strategic decision-making rather than tedious data sorting.
Additionally, Google Cloud Natural Language supports seamless integration with various data sources, making it easy to ingest and process information. This integration allows for real-time analytics, enabling teams to quickly generate reports tailored to current market trends. The automation of thematic grouping not only reduces the time spent by analysts but also enhances the overall quality of insights gleaned from data. In an environment where timely information is critical, tools like Google Cloud Natural Language position organizations to respond adeptly to customer needs and market dynamics.
Steps to Implement Automation with insight7
Implementing AI Thematic Automation effectively requires a structured approach to streamline the process. Begin with data integration and pre-processing. Collect relevant datasets, ensuring they are clean and organized, as this foundational step is crucial for producing valid insights. Properly formatted data sets enhance the software's ability to identify patterns and themes, ultimately reducing the analytical workload.
Next, focus on configuration and customization. Tailor the system settings to fit your specific workflows and preferences. This customization allows the tool to better understand and respond to your unique requirements, leading to more accurate thematic grouping. Finally, consistently monitor and optimize the system’s performance. Regularly assess the efficacy of the automated outputs, making adjustments as needed to improve the overall quality and relevance of insights generated. This iterative process ensures that AI Thematic Automation continuously evolves to meet your analytical needs effectively.
Step 1: Data Integration and Pre-processing
In the journey of AI thematic automation, the first step involves data integration and pre-processing. This phase is critical as it lays the foundation for effective analysis and thematic grouping. Initially, analysts must gather diverse data sources, which may include customer interviews, survey results, or market reports. With the right tools, this information can be consolidated into a single, manageable dataset. This integration enables a holistic view of the data, enhancing the capability to identify patterns and trends.
Once the data is integrated, pre-processing comes into play. This stage focuses on cleaning and organizing the data, ensuring it is free from errors and inconsistencies. Analysts might employ techniques such as text normalization, removing duplicates, and categorizing the data for improved clarity. The outcomes of this process directly influence the quality of insights derived from AI thematic automation. Properly prepared data not only saves time but also bolsters the reliability of the subsequent thematic analysis, paving the way for informed decision-making.
Step 2: Configuration and Customization
Configuration and Customization are critical steps in applying AI Thematic Automation effectively. This phase allows users to tailor the system based on their specific needs and objectives. After integrating and preprocessing data, users can configure parameters that influence how themes are identified and grouped. Customization options enable analysts to select keywords, phrases, or specific segments of data that matter most to their projects.
Moreover, the customization process involves adjusting settings to refine results continuously. Analysts can choose to prioritize certain themes over others, enhancing relevance and accuracy. Ultimately, thorough configuration and customization not only improve the effectiveness of AI Thematic Automation, but they also significantly reduce the time analysts spend on manual data interpretation. By automating these processes, you empower yourself to focus on more strategic tasks within your organization.
Step 3: Monitoring and Optimization
Monitoring and optimization are essential components in ensuring effective AI thematic automation. Once your AI system is in place, continuous assessment becomes vital. This process involves regularly reviewing the results produced by the AI to ensure that the thematic grouping remains relevant and accurate. Regular insights into how the AI operates can help identify areas for improvement.
To effectively monitor and optimize AI thematic automation, key steps should be taken. First, establish clear metrics for success that reflect your specific analytical goals. Second, gather feedback from users regarding the usefulness of the thematic groups. Regularly updating the AI’s training data is crucial for maintaining its accuracy over time. Finally, iterate on the configuration settings to fine-tune the performance. By continuously monitoring these factors, businesses can maximize the benefits of AI thematic automation, streamline their analytical processes, and ultimately reduce the time analysts spend on repetitive tasks.
Conclusion: Leveraging AI Thematic Automation for Time Efficiency
AI Thematic Automation offers a robust solution for enhancing time efficiency in data analysis. By automating thematic grouping, analysts can quickly identify trends and insights from vast volumes of data. This not only reduces the time spent on manual analysis but also improves accuracy by minimizing human errors often associated with data interpretation.
Furthermore, the implementation of AI tools streamlines workflows, allowing analysts to focus on strategic decision-making rather than tedious data management. This shift not only boosts productivity but also empowers teams to deliver actionable insights faster, fostering a more responsive and effective analysis process overall.