Dynamic insight generation is transforming the landscape of research, enabling scholars to access and analyze data in real time. As researchers navigate complex datasets, the ability to respond swiftly to emerging trends creates unparalleled opportunities for innovation. This approach not only enhances the accuracy of findings but also fosters a more profound understanding of ever-evolving phenomena.
Incorporating real-time data analysis into research practices empowers professionals to refine their methodologies dynamically. Immediate feedback allows for rapid adjustments to hypotheses, making it easier to address uncertainties. As researchers embrace this capability, they unlock potential pathways to groundbreaking insights and informed decision-making. This section explores the profound impact that real-time data analysis has on the research community, illustrating its role as a game changer.
Analyze & Evaluate Calls. At Scale.
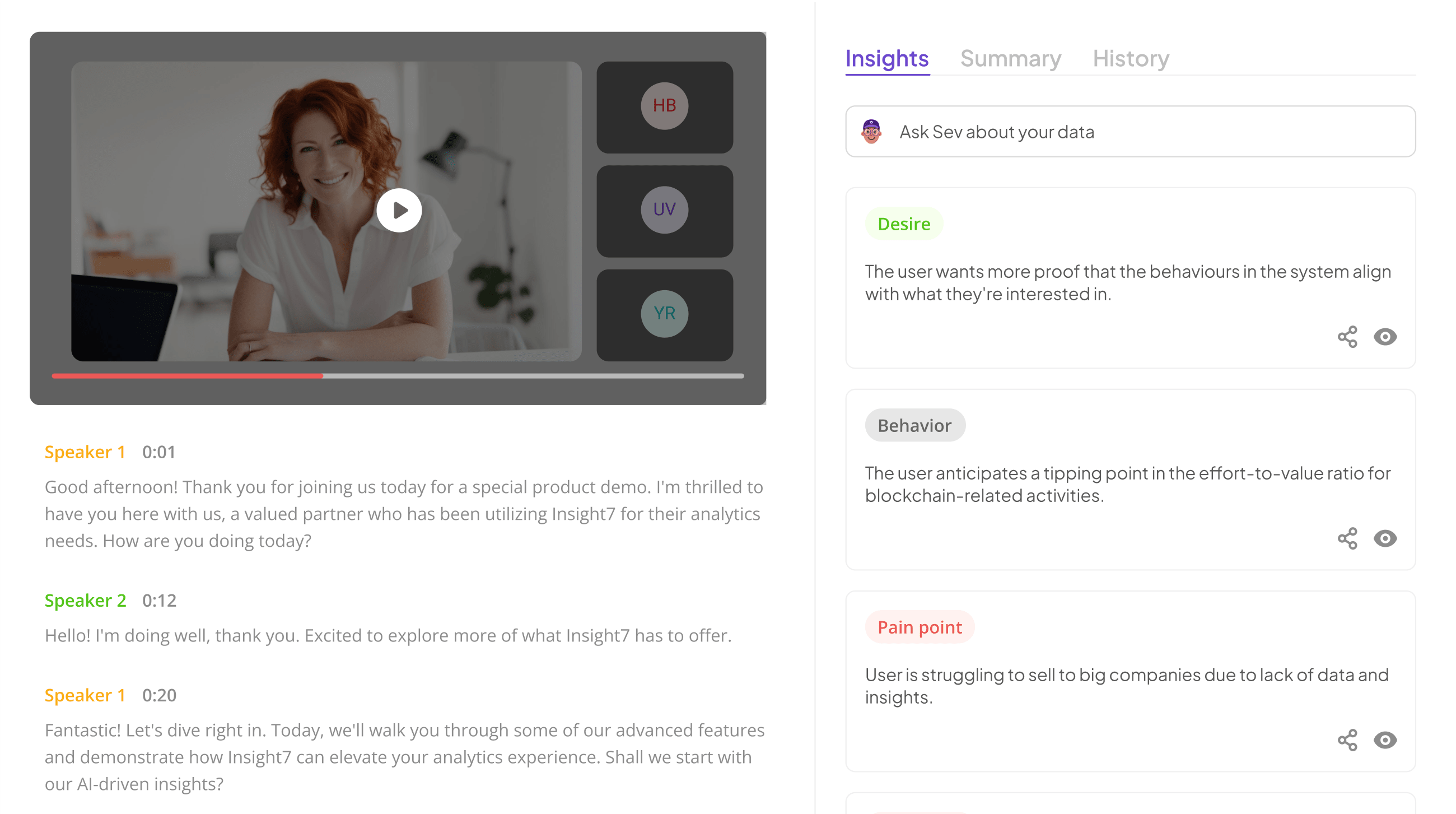
RealTime data analysis is revolutionizing the way researchers generate insights and drive innovation. By tapping into immediate data streams, scholars can obtain a more accurate understanding of dynamic phenomena and rapidly adjust their hypotheses.
Real-time data analysis is transforming how researchers generate insights and foster innovation. By tapping into immediate data streams, scholars can enhance their understanding of complex and ever-changing phenomena. This access allows researchers to observe fluctuations and patterns in real-time, which can lead to more accurate conclusions. The ability to quickly adjust hypotheses based on current data helps researchers remain agile in their studies.
Dynamic insight generation becomes possible when researchers actively utilize real-time analysis to refine their approaches. Immediate feedback enables swift modifications in methodologies, reducing the likelihood of errors. Additionally, researchers can create better predictive models by effectively analyzing the fluidity of complex systems. This adaptability not only promotes innovative thought but also positions researchers at the forefront of their fields, capable of addressing emerging questions and challenges with confidence.
Dynamic Insight Generation: Empowering Researchers with RealTime Analysis
Dynamic Insight Generation empowers researchers to harness real-time analysis for profound discoveries. In todayโs fast-paced research environment, timely insights can make the difference between a relevant finding and an overlooked opportunity. Through dynamic insight generation, researchers can engage with data as it unfolds, leading to swift decision-making and adapted methodologies tailored to current conditions.
Utilizing real-time data ensures that researchers maintain an edge through immediate feedback loops. This feature allows for rapid fine-tuning of experiments or studies based on the latest findings. Additionally, the ability to visualize complex data trends enhances accuracy, minimizing the likelihood of errors that might arise from outdated information. As a result, researchers are better equipped to interpret evolving phenomena and contribute meaningfully to their fields. By embracing these innovative practices, researchers not only elevate their work but also foster greater collaboration and impact in their communities.
Benefits of RealTime Data for Researchers
Real-time data analysis is transforming how researchers generate valuable insights, allowing them to respond with agility to evolving circumstances. One significant benefit is the establishment of immediate feedback loops, which enable researchers to make quick adjustments to their methodologies. This responsiveness is critical in a fast-paced research environment where conditions can shift rapidly.
Additionally, real-time analysis enhances accuracy by minimizing the risks associated with outdated data. Researchers can access current information, reducing errors and ensuring that their conclusions are based on the most relevant evidence. This dynamic insight generation empowers scholars to delve deeper, fostering more innovative and impactful research outcomes. Furthermore, understanding complex systems becomes easier when researchers can visualize the data as it changes, leading to informed decision-making and strategic planning for future studies. By embracing real-time data, researchers enhance their ability to make informed choices and refine their hypotheses effectively.
- Immediate Feedback Loops: Enabling swift adjustments in research methodologies.
Immediate feedback loops are essential for researchers navigating the complexities of dynamic insight generation through real-time data analysis. By implementing these feedback loops, researchers can swiftly adapt their methodologies based on the immediate analysis of data. This agility allows for continual improvement and refinement of research strategies, ultimately leading to richer and more relevant insights.
The ability to adjust research methodologies in real-time greatly enhances the research process. Feedback loops enable researchers to identify when a selected approach may not yield optimal results, facilitating rapid re-evaluation and course correction. This synchrony between data collection and analysis not only boosts accuracy but also fosters a research environment that is responsive to emerging trends and insights. Consequently, researchers become empowered to make informed decisions that guide their investigations toward impactful outcomes. The continuous cycle of evaluating data and refining methodologies transforms traditional research practices, allowing for more innovative and relevant breakthroughs in the field.
- Enhanced Accuracy: Minimizing errors by using up-to-date information.
In the dynamic landscape of research, enhanced accuracy hinges on access to real-time data. Utilizing up-to-date information minimizes the risk of errors, ensuring that researchers avoid outdated conclusions. This immediate access facilitates dynamic insight generation, allowing scholars to adapt their analyses based on the latest findings. With timely data, researchers can craft hypotheses that are not only relevant but also reflective of current trends, significantly improving the overall quality of their work.
To achieve enhanced accuracy through dynamic insight generation, consider three key strategies:
- Continuous Data Monitoring: By constantly tracking relevant data streams, researchers can make informed decisions based on recent developments.
- Validation of Sources: Ensuring that data comes from reputable sources helps eliminate inconsistencies and boosts reliability.
- Integrating Feedback Mechanisms: Immediate feedback loops can refine research questions and methodologies, fostering ongoing improvement in accuracy.
Implementing these strategies not only curtails errors but also amplifies the integrity of research findings, creating a resilient framework for future inquiries.
Dynamic Complex Systems Analysis
Dynamic Complex Systems Analysis delves into the intricacies of systems that evolve and adapt over time. This type of analysis aims to understand how interconnected parts react to varying conditions, allowing researchers to capture the complexities of real-world phenomena. As systems are often unpredictable, real-time data plays a critical role in revealing trends and facilitating observations that may otherwise go unnoticed.
To harness the power of Dynamic Insight Generation, researchers can employ several strategies. First, they can focus on understanding the unique dynamics of the environment being studied. This involves not just analyzing data, but interpreting the relationships and interactions within the system. Second, scenario planning and forecasting can produce predictive models that inform future research directions. By anticipating how systems evolve based on real-time factors, researchers can make informed decisions and respond to changes swiftly. Such analyses empower researchers to adapt their methodologies, enhancing both the relevance and impact of their findings.
- Understanding Dynamic Environments: Capturing the fluidity of complex systems as they evolve.
Dynamic environments are characterized by their ever-changing nature, necessitating a deep understanding of how complex systems evolve over time. Researchers must acknowledge that these systems are influenced by various factors, including social, technological, and economic trends. To effectively capture this fluidity, dynamic insight generation becomes a key asset. By utilizing real-time data analysis, researchers can observe how systems respond to changing variables, rapidly adapting their strategies and hypotheses.
This approach allows for an ongoing assessment of system dynamics, providing insights that traditional methods may overlook. Moreover, scenario planning and forecasting become feasible with current data at hand, enabling teams to anticipate future trends and challenges. By embracing the ability to analyze shifting landscapes, researchers can create informed strategies that are responsive to real-world changes. This proactive stance not only enhances decision-making but also fuels innovation across disciplines.
- Scenario Planning and Forecasting: Utilizing real-time data for predictive analytics and future-oriented strategies.
Scenario planning and forecasting can significantly impact researchers by employing real-time data for predictive analytics. When researchers harness immediate information, they can create various scenarios that anticipate possible future outcomes. This proactive approach enables them to develop robust strategies that adapt swiftly to changes. By utilizing dynamic insight generation, organizations can analyze trends and potential challenges, allowing better decision-making.
In practice, scenario planning involves evaluating multiple future possibilities and their implications. Researchers can map out different paths based on current data, creating informed strategies for each. By integrating real-time insights into forecasting methods, they gain a comprehensive understanding of evolving conditions. This resilience not only enhances research validity but also prepares teams to respond effectively to unexpected developments. Ultimately, embracing scenario planning and forecasting transforms how researchers approach their work, driving innovation and leading to more reliable outcomes.
Extract insights from interviews, calls, surveys and reviews for insights in minutes
Key Tools for Dynamic Insight Generation in RealTime Data Analysis
Key Tools for Dynamic Insight Generation in RealTime Data Analysis include essential technologies that empower researchers to transform raw data into actionable insights. Utilizing these tools allows researchers to harness immediate data streams effectively, facilitating a deeper understanding of complex phenomena. One influential tool in this space is Insight7, which provides robust analytical features tailored for real-time data processing.
In addition to Insight7, platforms like Apache Kafka and Amazon Kinesis enhance the capacity for dynamic insight generation. Apache Kafka supports distributed event streaming, making it ideal for processing vast amounts of real-time data. Similarly, Kinesis offers powerful data streaming analytics that enables researchers to visualize trends instantly. Flume further complements this ecosystem by efficiently collecting and aggregating data streams. Finally, Tableau serves as a key visualization tool, allowing scholars to interpret trends in real-time data effectively. By integrating these tools into their workflows, researchers can optimize their analyses and swiftly adapt their methodologies to emerging insights.
Insight7 and Other Leading Tools
Modern research demands tools that can keep pace with the rapid influx of data. Insight7 and other leading tools empower researchers to generate dynamic insights seamlessly. By facilitating immediate analysis, these platforms help researchers grasp complex trends and patterns from real-time data, ultimately driving informed decision-making.
Among these tools, Insight7 stands out due to its user-friendly interface and powerful feature set that allows for efficient data analysis at scale. Researchers can effortlessly collect and analyze customer signals, bypassing the cumbersome traditional methods that often slow down the process. In addition to Insight7, platforms like Apache Kafka and Kinesis provide robust options for real-time data streaming. Flume enables the aggregation of vast data streams, while Tableau specializes in visualization, making it easier to interpret results. Each tool plays a critical role in enhancing dynamic insight generation, ensuring researchers remain competitive in their fields.
- Insight7: Premier tool for facilitating instant insight generation with robust analytical features.
Insight7 stands out as a premier tool for facilitating instant insight generation, designed to cater to the needs of researchers seeking dynamic analysis. Its robust analytical features enable researchers to delve deep into real-time data streams, thereby facilitating a more comprehensive understanding of their subjects. In an age where timely data interpretation is crucial, Insight7 empowers users to extract meaningful insights without the need for extensive technical expertise.
The platform's intuitive interface allows researchers to easily navigate through various functionalities. Users can upload multiple data sources, allowing for a consolidated view of insights across separate calls or interviews. By harnessing dynamic insight generation, researchers can quickly identify trends, pain points, and significant themes that may surface within their datasets. This instant access to actionable insights equips scholars with the ability to make informed decisions, refine their hypotheses, and respond effectively to evolving research landscapes.
- Apache Kafka: A distributed event streaming platform optimized for real-time data processing.
Apache Kafka serves as a pivotal technology in the realm of real-time data processing. As a distributed event streaming platform, it enables organizations to process large streams of data seamlessly and efficiently. This capability allows researchers to collect, analyze, and act upon continuous data flows, which is essential for dynamic insight generation.
Utilizing Kafka, researchers can build robust data pipelines that facilitate the immediate integration of insights into their projects. This agility in processing data can significantly enhance decision-making, allowing scholars to respond proactively to evolving trends. Moreover, Kafka's scalability ensures that as research data expands, the platform can accommodate increased loads without sacrificing performance. By employing such advanced tools, researchers can transform their inquiries into actionable insights, thus driving innovation in their respective fields. This unprecedented access to real-time data presents a game-changing opportunity for research in todayโs fast-paced landscape.
- Flume: Specifically designed for collecting, aggregating, and moving large streams of data in real time.
Flume serves as a vital component in the landscape of real-time data analysis, specifically engineered for collecting, aggregating, and transferring vast amounts of data. Its architecture ensures that high-velocity data flows can be managed effortlessly, enabling researchers to access real-time information and respond efficiently. The capabilities of Flume empower users to harness significant data streams, transforming raw information into actionable insights.
The ability to analyze data streams as they are generated facilitates dynamic insight generation, allowing researchers to make informed decisions quickly. Through efficient aggregation techniques, Flume supports seamless integration with various data sources, enhancing the speed and reliability of research initiatives. Ultimately, its real-time functionalities not only optimize the data collection process but also streamline collaboration among research teams, making research efforts more effective and impactful.
- Kinesis: AWS tool for real-time data streaming and analytics.
Kinesis serves as a robust solution tailored for handling real-time data streaming and analytics, making it invaluable for researchers aiming to adapt dynamically to their findings. With its capacity to process vast amounts of data seamlessly, Kinesis empowers users to generate insights swiftly. This is particularly crucial when circumstances are fluid, allowing researchers to respond to changes in data patterns instantaneously.
Moreover, Kinesis enhances the ability to perform real-time analytics, enabling immediate reactions to emerging trends. As researchers dive deeper into their fields, the utilization of Kinesis fosters a culture of experimentation and agility. This tool's integration into research workflows ensures that scholars can continually refine their hypotheses and methodologies, driving towards more accurate outcomes. Ultimately, the synergy of Kinesis with real-time data analysis represents a significant leap forward in dynamic insight generation.
Features of Kinesis That Enable Dynamic Insight Generation
- Scalability: Kinesis can handle increasing data loads without compromising performance.
- Real-Time Processing: It captures and analyzes data as it flows, ensuring timely insights.
- Integration Capability: Kinesis easily connects with other AWS services, enhancing its functionality.
- User-Friendly Interfaces: Simplifies data visualization, making analytical results more accessible for all stakeholders.
These features collectively position Kinesis as a pivotal component in the ecosystem of dynamic insight generation, revolutionizing how researchers engage with data.
- Tableau: Offers powerful visualization capabilities to intuitively understand real-time data trends.
Data visualization plays a crucial role in real-time data analysis, as it transforms complex data sets into intuitive visual formats. Applications like Tableau empower researchers by providing them with powerful visualization capabilities, allowing for dynamic insight generation. Users can effortlessly create interactive dashboards that showcase trends and patterns, enabling them to identify key insights at a glance.
The ability to visualize real-time data is especially beneficial for researchers engaged in fast-paced environments. With dashboards that update instantaneously, scholars can make informed decisions based on current data rather than relying on outdated information. This agility not only enhances accuracy in analysis but also fosters an environment where hypotheses can be tested and refined efficiently. By fully leveraging such visualization tools, researchers can unlock new dimensions of understanding, revolutionizing their approach to data analysis.
Steps to Implement Realtime Data Analysis
To implement real-time data analysis, begin by identifying appropriate data sources that directly align with your research objectives. Focus on gathering data streams that are timely and relevant, allowing for immediate reflection on your methodologies. This foundational step ensures that the insights generated from your analysis are both accurate and actionable.
Next, choose the right tools that suit your analytical needs. Tools vary significantly in features, so evaluate options like Insight7, which offers robust analytical capabilities, or Tableau for enhanced data visualization. Selecting the right tools enables researchers to streamline the data processing and visualization stages, ultimately enhancing the dynamics of insight generation. Together, these steps create a solid framework for effectively harnessing real-time data, making it a powerful ally in your research endeavors.
By following these steps, you can unlock the potential of dynamic insight generation, transforming how you approach research and decision-making.
Step 1: Identify Appropriate Data Sources
Identifying appropriate data sources is a critical first step in the journey of dynamic insight generation. Start by understanding your research objectives clearly, as this will guide your source selection process. Engage with various data streams, such as social media platforms, surveys, and existing databases, which can offer immediate insights relevant to your focus area. These data sources should align seamlessly with your research questions to ensure that the information gathered is both actionable and pertinent.
Next, assess the quality and reliability of your selected data sources. Utilize platforms that provide real-time data access, which enhances the accuracy of your analyses. Integrating diverse sources also helps in capturing a holistic view of the research topic, allowing for a more nuanced understanding. By meticulously identifying and curating the right data sources, you lay a strong foundation for effective real-time data analysis, ultimately driving valuable insights important for your research objectives.
- Select data streams that align with your research objectives.
Selecting data streams that align with your research objectives is crucial for effective analysis. Start by clearly defining your research aims. This step helps identify the specific data required to answer your research questions. Whether you need customer sentiment, market trends, or operational metrics, choosing the right streams directly impacts the insights you will gather.
Next, assess the relevancy and reliability of potential data sources. High-quality, real-time data is essential for dynamic insight generation. This means considering sources like social media, customer feedback, or industry reports, which can provide timely information vital for understanding active trends. By strategically selecting data streams, you not only enhance the accuracy of your findings but also create a foundation for actionable insights, allowing your research to remain impactful and aligned with your goals.
Step 2: Choose the Right Tools
Selecting the right tools is crucial for achieving effective Dynamic Insight Generation. Your choice of tools will directly impact the speed and quality of the insights you derive from your data streams. To begin, assess your specific research needs, such as the size and complexity of data, as well as the types of analyses you intend to perform. A simple project may be served with basic tools, while more complex analyses will require advanced software solutions designed for real-time data processing.
Consider tools like Insight7, which offers seamless integration and powerful analytics features. Additionally, Apache Kafka can efficiently handle moving data in real time, ensuring you stay on top of changing conditions. Other options like Tableau provide robust visualization capabilities, allowing for intuitive understanding of trends and patterns. Evaluating these tools in relation to your dynamic research needs will foster a more effective analytical environment, ultimately enhancing your ability to generate valuable insights.
- Evaluate and select tools based on your analytical needs and data complexity.
Selecting the right tools for real-time data analysis is crucial for effective dynamic insight generation. Researchers must first assess their unique analytical needs, considering factors like the type of data being analyzed and the complexity of their research questions. If your project involves intricate data sets, look for tools that handle multidimensional data and integrate seamlessly with existing systems.
Once you pinpoint your requirements, evaluate the functionality and user-friendliness of potential tools. Some platforms excel in visualizations, while others are designed for processing large volumes of data. Prioritize user-friendly interfaces and robust support to facilitate the tool's adoption into your workflow. By strategically aligning the right tools with your research objectives, you can streamline data analysis processes and enhance the quality of insights derived, ultimately transforming your research outcomes.
Conclusion: Transforming Research Through Dynamic Insight Generation
Real-time data analysis represents a transformative shift in how researchers approach their work. By focusing on dynamic insight generation, researchers unlock immediate access to evolving data, allowing them to adapt their strategies quickly and intelligently. This agility not only enhances the accuracy of results but also fosters a culture of continuous improvement and innovation in academic settings.
Embracing dynamic insight generation empowers researchers to navigate complex environments with confidence. As the landscape of information becomes increasingly intricate, the ability to synthesize real-time data into actionable insights will be essential. With tools designed to facilitate this process, researchers can enhance collaboration and streamline their workflows, paving the way for groundbreaking discoveries and applications across disciplines.
RealTime data analysis stands as a pivotal innovation for researchers, offering unprecedented possibilities for generating dynamic insights. By leveraging this approach, scholars can not only achieve more accurate outcomes but also pioneer new paths in their respective fields.
RealTime data analysis represents a transformative leap for researchers, enabling them to generate dynamic insights that were previously unattainable. This approach allows scholars to engage with data as it unfolds, providing a foundation for more responsive and adaptable research methodologies. By staying attuned to real-time information, researchers can identify trends and anomalies more swiftly, thus refining their hypotheses and improving the accuracy of their findings.
Furthermore, RealTime data analysis fosters innovative exploration within various academic fields. Scholars can utilize immediate feedback loops to adjust experiments or surveys instantaneously, enhancing their understanding of complex phenomena. With the ability to capture and analyze data dynamically, researchers not only benefit from timely insights but also contribute to the ongoing evolution of knowledge in their respective domains. This capability not only empowers researchers but also elevates the entire research process, offering avenues for groundbreaking discoveries.