Proactive Call Management is transforming how organizations assess and address call quality in real time. Imagine a scenario where agents receive immediate support when a conversation begins to veer off course. This proactive approach not only enhances customer satisfaction but also reduces the chances of escalation, leading to more streamlined operations.
In the realm of Predictive QA, proactive call management empowers organizations to systematically identify at-risk calls before they escalate. By leveraging AI technology, it becomes possible to analyze interactions, detect potential issues, and implement solutions dynamically. This innovative approach allows businesses to improve compliance and foster a more effective customer service environment, ultimately reshaping the call management landscape.
Analyze qualitative data. At Scale.
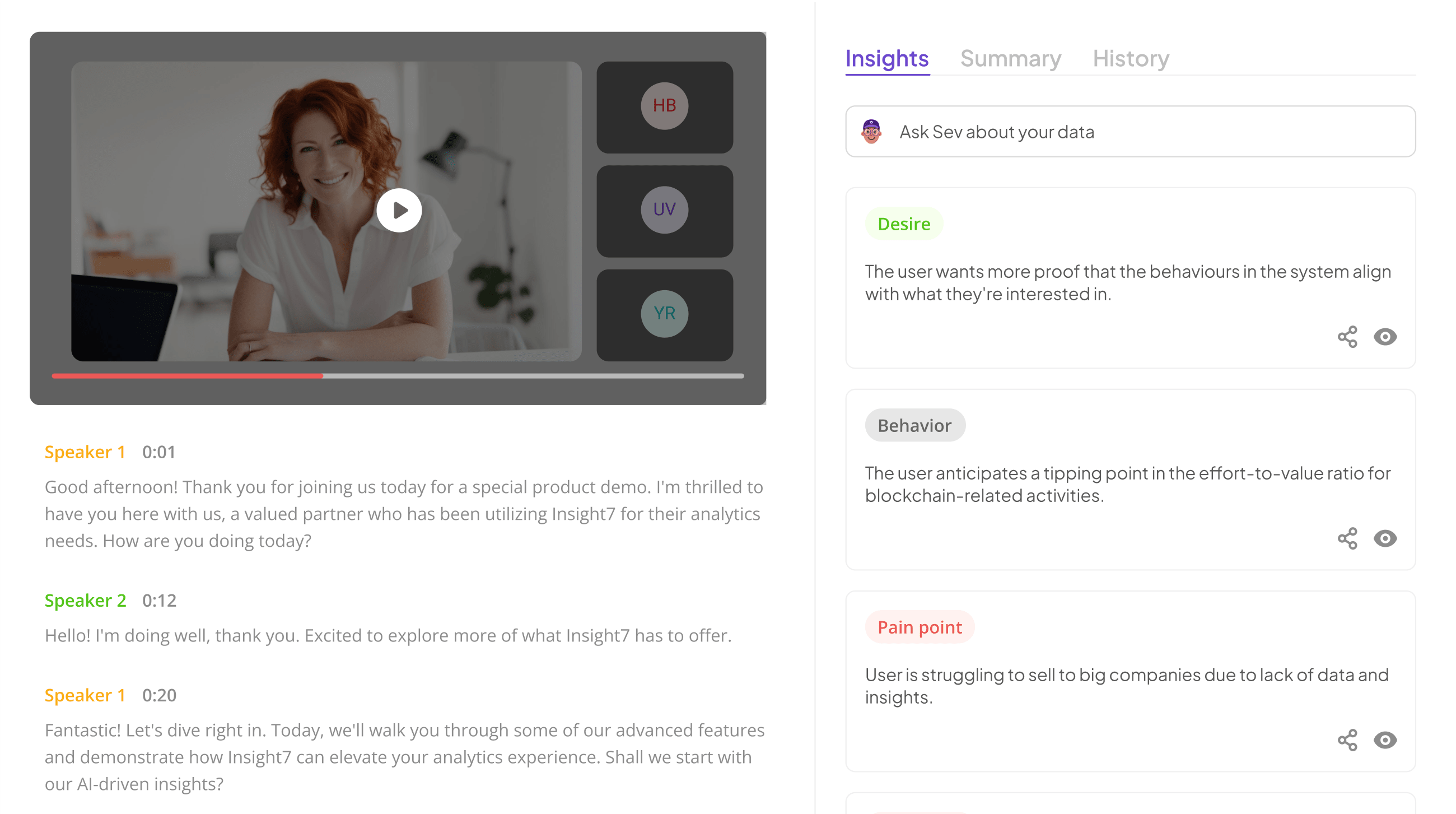
Understanding Proactive Call Management: AIs Role in Predictive QA
Proactive Call Management is essential in ensuring call quality and customer satisfaction. Understanding how AI enhances this process is key to implementing effective predictive quality assurance (QA). AI has the capability to analyze communication patterns, identifying potential issues before they escalate. By processing real-time data, AI systems can pinpoint at-risk calls, facilitating timely interventions and reducing escalation rates.
Incorporating AI into Proactive Call Management involves several critical aspects. First, accurate identification of speaking agents is crucial for analysis. AI can recognize agents through voice patterns or by matching names provided in training data. Furthermore, this technology generates comprehensive performance reports, allowing organizations to evaluate individual agent effectiveness. Such insights empower teams to address specific training needs and enhance overall communication strategies. By leveraging AI, businesses can create a more efficient and responsive call management system that prioritizes customer satisfaction.
The Basics of Predictive QA
Predictive Quality Assurance (QA) focuses on preemptively identifying potential issues in customer interactions, optimizing call management effectively. By assessing calls before they escalate, organizations can enhance customer experiences and streamline their operations. This proactive approach helps in recognizing at-risk calls, significantly mitigating the risk of customer dissatisfaction and subsequent escalations.
To effectively implement predictive QA, it is essential to understand several key components. First, call analysis relies on advanced algorithms that evaluate various interaction metrics, allowing teams to pinpoint areas needing improvement. Second, real-time data processing ensures immediate insights, enabling managers to take rapid action on flagged calls. Together, these elements form a foundation for Proactive Call Management, enhancing both operational efficiency and customer satisfaction. Embracing this methodology can lead to a more responsive and adaptable service environment.
- Explanation of Predictive Quality Assurance.
Predictive Quality Assurance (QA) is a pivotal aspect of proactive call management, designed to enhance customer interactions. This approach employs AI to analyze calls and assess the quality of conversations in real-time. By monitoring various criteria, businesses can identify calls that are at risk of escalation, allowing for timely intervention. This not only improves customer satisfaction but also reduces operational costs by preventing issues before they intensify.
Understanding the mechanics of Predictive QA involves several key elements. First, AI algorithms scrutinize call transcripts for compliance and engagement standards. Next, they assess factors such as problem identification, context collection, and customer education. This comprehensive evaluation enables organizations to benchmark performance against predefined templates. Ultimately, Predictive QA provides a reliable framework for ensuring quality interactions, empowering teams to make data-driven decisions that align with customer needs.
- Importance of Identifying At-Risk Calls.
Identifying at-risk calls is crucial for effective customer service management. It allows organizations to pinpoint potential issues before they escalate, ensuring a smoother customer experience. By recognizing patterns and evaluating conversations, proactive call management helps identify areas where representatives struggle, allowing for timely intervention and additional coaching. This process not only enhances individual performance but also improves overall team dynamics, fostering a culture of continuous improvement.
Furthermore, timely identification of at-risk calls can significantly reduce customer churn. When potential problems are addressed swiftly, customers feel valued and understood. As a result, enhancing customer satisfaction leads to increased loyalty and long-term relationships. Harnessing AI for this purpose equips organizations with the tools needed to analyze calls more effectively, ensuring that every conversation aligns with predetermined standards. Ultimately, prioritizing the identification of at-risk calls creates a foundation for success in delivering exceptional service.
How AI Powers Proactive Call Management
AI drives proactive call management by automatically analyzing conversations and identifying patterns that signal potential issues before they escalate. Through advanced algorithms, AI can sift through data in real time, providing organizations with immediate insights into call quality. This proactive approach allows teams to address concerns swiftly, ensuring a smooth customer experience.
Additionally, AI enhances the ability to predict which calls are at risk by evaluating multiple factors, such as sentiment and engagement levels. By flagging these calls early, teams can intervene effectively and provide additional training or resources to agents. This not only improves customer satisfaction but also fosters a culture of continuous improvement within the organization. As a result, proactive call management becomes a proactive strategy, transforming potential negative experiences into opportunities for growth and better service delivery.
- AI Algorithms in Call Analysis.
AI algorithms play a crucial role in enhancing call analysis by facilitating proactive call management strategies. These algorithms analyze vast volumes of data in real-time, identifying patterns that indicate potential issues within calls. The ability to detect these at-risk calls before escalation is vital for maintaining service quality and customer satisfaction.
Key AI techniques utilized in call analysis include natural language processing and sentiment analysis. Natural language processing helps in understanding the nuances of conversations, while sentiment analysis gauges the emotional tone of the dialogue. Both techniques contribute to a clearer insight into how agents interact with customers. Additionally, machine learning models refine these processes over time, improving accuracy as more data is collected. Thus, effective use of AI algorithms not only streamlines operations but also proactively addresses challenges, ensuring that organizations remain ahead in their quality assurance efforts.
- Real-time Data Processing for Immediate Insights.
Real-time data processing has revolutionized the ability to glean immediate insights from call interactions. In the realm of proactive call management, AI technologies analyze call data as it happens, allowing for swift identification of at-risk conversations. This immediacy empowers teams to intervene before issues escalate, enhancing customer satisfaction and operational efficiency.
Consider how this process works: as calls progress, AI systems actively monitor sentiment, keywords, and interactions, compiling data in real time. This capability means decision-makers can quickly respond to emerging patterns or potential problems. Immediate insights generated from this analysis provide a roadmap for identifying training needs or improving processes, creating a proactive approach to call management that addresses challenges early on. By harnessing real-time data, organizations position themselves to enhance overall service quality and reduce escalation rates.
Extract insights from interviews, calls, surveys and reviews for insights in minutes
Tools for Proactive Call Management in Predictive QA
Effective tools for proactive call management play a critical role in predictive quality assurance. These tools empower organizations to identify potential issues before they escalate into significant problems. By integrating intelligent software solutions, companies can process call data in real-time, allowing for immediate insights into agent performance and customer needs.
One key element is the use of AI-driven analytics. These systems analyze historical call trends and flag anomalies, helping supervisors quickly address performance issues. Another essential tool involves dashboards that summarize call metrics, providing easy access to vital information. Furthermore, sentiment analysis tools enhance customer interactions by gauging emotions through vocal cues, enabling agents to adjust their approach accordingly. By combining these powerful tools, businesses can fine-tune their customer service strategies, ensuring a higher level of satisfaction and efficiency.
Top Tools for At-Risk Call Identification
To effectively identify at-risk calls, several essential tools play a critical role in proactive call management. These tools utilize cutting-edge AI algorithms to analyze conversation patterns, flagging potential issues before they escalate. Features like sentiment analysis, keyword recognition, and real-time feedback provide agents with crucial insights that can enhance customer service interactions.
A key component of these tools is their ability to aggregate and analyze call data. This data-driven approach enables organizations to pinpoint common concerns and frequently asked questions. Subsequently, this helps in refining training programs, aligning them more closely with customer needs. By integrating these tools into existing systems, teams can foster a culture of continuous improvement, leading to better outcomes for both customers and staff. The proactive identification of at-risk calls not only aids in resolution but also enhances overall customer satisfaction.
- Insight7
AI transforms the landscape of customer support by facilitating proactive call management. In this context, Insight7 stands out as an advanced platform that enables teams to identify at-risk calls before they escalate. This proactive approach is essential, as it creates opportunities for timely interventions, allowing service representatives to address potential issues before they become significant problems.
By utilizing AI, Insight7 analyzes customer interactions, providing insights that help identify patterns indicating dissatisfaction. With this critical information, organizations can cultivate a supportive environment for customers. They can personalize conversations, enhancing customer experiences and building trust. Consolidating these insights enables teams to transition from reactive responses to a more engaging, proactive strategy. Thus, the shift toward proactive call management marks a significant evolution in how businesses can retain customers and improve overall service quality.
- Tool 1: Features and Benefits.
Proactive Call Management enhances the quality of customer interactions through insightful analysis and timely feedback. This tool offers several important features that make it indispensable for identifying at-risk calls before escalation. First, it uses advanced AI algorithms to transcribe and analyze calls, ensuring that real-time insights are available to support goal-oriented coaching and training. Second, users can generate comprehensive reports that break down customer sentiments and specific challenges associated with calls, allowing teams to adapt their strategies promptly.
Another significant benefit is the ability to create dashboards that deliver a visual representation of important metrics. This helps organizations quickly gauge performance and identify areas needing improvement. By actively managing calls with predictive analytics, teams can shift from a reactive to a proactive approach, ultimately improving customer satisfaction and reducing escalation rates. In summary, these features collectively empower organizations to foster stronger customer relationships and enhance their overall service quality.
- Tool 2: Features and Benefits.
Proactive Call Management is designed to enhance the quality of customer interactions, ensuring that potential issues are swiftly identified and addressed. One significant feature of this tool is its ability to analyze incoming calls in real time, allowing for immediate feedback. This ensures that customer service agents are not just reacting to problems but proactively managing them to prevent escalation. The platform also provides a consultative approach, encouraging agents to engage meaningfully with customers, rather than just processing their requests.
Another key benefit is the seamless integration of data analytics. By transcribing and analyzing conversations, the tool offers insights that inform training and coaching practices. As trends emerge, teams can adjust their strategies accordingly to align better with customer needs. This not only improves the service experience but also empowers agents with valuable knowledge, leading to more effective problem resolution. Overall, the features and benefits of proactive call management create a foundation for sustained customer satisfaction.
- Tool 3: Features and Benefits.
Effective Proactive Call Management is crucial in today's customer service environment. Through advanced analytics and data processing, organizations can identify at-risk calls before they escalate. With Tool 3, teams can access robust features that facilitate real-time insights into call interactions. This empowers agents to act quickly, reducing the chances of customer dissatisfaction and potential escalations.
Key features of Tool 3 include call transcription and analysis, which transform spoken interactions into searchable text. This allows for a comprehensive evaluation of call dynamics, enabling the identification of common issues or trends that may impact customer experience. Additionally, instant feedback mechanisms inform agents how they can improve their approach during calls, promoting a consultative sales strategy rather than an merely transactional one. Overall, the benefits of implementing Tool 3 extend beyond immediate problem-solving; they foster a culture of continuous improvement and enhanced customer connection.
Integrating Predictive QA Tools into Existing Systems
Integrating predictive QA tools into existing systems is an essential step towards enhancing proactive call management. Organizations can achieve this by taking a few key steps. First, audit current call management systems to identify areas where AI can be integrated effectively. It's crucial to assess the data inputs and the relevant metrics that the predictive tools will analyze. Second, ensure seamless functionality by configuring the tool to work alongside existing software. This integration allows for a unified approach to call handling, enabling agents to receive alerts for at-risk calls in real time.
Moreover, training staff on the new systems can alleviate common challenges faced during integration. By emphasizing collaborative learning, teams can better adapt to AI-driven insights that assist in identifying potential issues before escalation. Ultimately, the goal is to create a cohesive environment where predictive QA tools empower agents with actionable insights, enhancing overall customer satisfaction and operational efficiency.
- Steps for Seamless Integration.
To achieve seamless integration of predictive QA tools into your existing call management systems, the first step is to assess your current technology. Evaluate which tools are already in place, and identify gaps that the new predictive QA system can fill. This groundwork is crucial for ensuring that the new system will complement your operations rather than disrupt them.
Next, establish clear objectives for integration. Define what you aim to achieve with proactive call management, such as reducing escalations or improving customer satisfaction. Communicate these goals across all levels of your organization, ensuring that employees understand how the AI-driven approach benefits them and enhances the customer experience.
Finally, conduct thorough testing of the tools in a controlled environment. Monitor for compatibility issues and gather feedback from team members who will be using the system. This will help to refine the integration process, leading to a smoother transition and optimal usage of advanced analytics in preventing at-risk calls from slipping through the cracks.
- Overcoming Common Challenges.
Navigating the landscape of proactive call management can pose several challenges. First and foremost is the complexity of integrating AI into existing systems. Organizations often grapple with the technical aspects of implementation, which can lead to disruptions in workflow. To address this challenge, a clear strategy is essential. Prioritize training employees to ensure they feel comfortable with the new tools, enhancing overall adoption rates.
Another prevalent challenge lies in analyzing the data generated by AI. It’s vital to distinguish between noise and actionable insights. Implementing user-friendly dashboards can alleviate this concern by providing accessible data visualization. Regular team reviews of the insights gathered can also foster a culture of continuous improvement. By actively engaging with the identified patterns and trends, companies can better refine their approach to managing at-risk calls. Overcoming these obstacles not only streamlines processes but significantly enhances customer satisfaction through improved predictive QA initiatives.
Conclusion: The Future of Proactive Call Management in Predictive QA
In conclusion, the future of proactive call management in predictive QA is bright, marked by continuous advancements in AI technology. As organizations increasingly adopt these systems, they will enhance their ability to identify at-risk calls before escalation. This proactive approach not only improves overall customer satisfaction but also empowers teams with actionable insights for training and development.
Looking ahead, the integration of advanced algorithms and real-time data processing will revolutionize how call centers operate. By enabling continuous monitoring and evaluation, proactive call management will foster a culture of compliance and excellence. As businesses embrace this shift, they will be better positioned to address customer needs promptly, ensuring a positive experience at every interaction.