Predictive Behavioral Data Insights with AI Agents
-
Bella Williams
- 10 min read
AI-Driven Behavior Prediction transforms how we understand and interpret user actions through predictive data insights. By tapping into extensive datasets, this approach allows businesses to forecast future behaviors with remarkable precision. As organizations aim to refine their strategies, the need for insights into consumer motivations and patterns has never been greater.
The integration of AI technologies enhances predictive capabilities, revealing trends and preferences that were once hidden. Through effective data collection and analysis, companies can utilize these insights to tailor their offerings, ultimately aligning with customer expectations. This document will explore the principles, challenges, and tools associated with AI-Driven Behavior Prediction, paving the way for more informed decision-making in today's data-driven landscape.
Analyze & Evaluate Calls. At Scale.
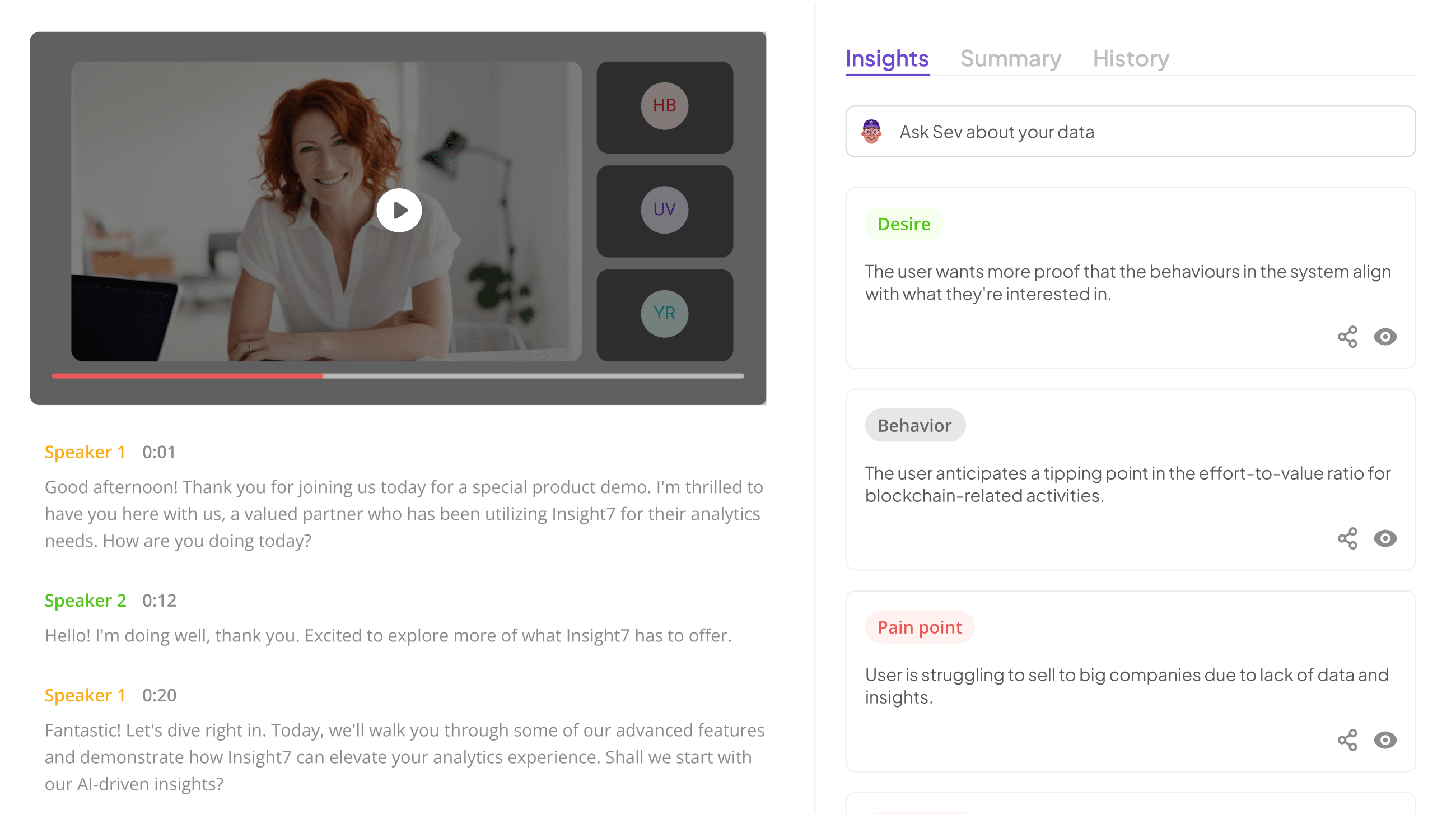
Understanding Predictive Behavioral Data Insights with AI Agents
Predictive behavioral data insights with AI agents drive a deeper understanding of consumer actions and preferences. By tapping into AI-driven behavior prediction, organizations can anticipate user needs effectively. This analytical approach allows businesses to collect and interpret vast amounts of data to uncover patterns that inform decision-making processes.
To harness the power of AI-driven behavior prediction, several key principles emerge. First, data collection and preparation play a vital role; accurate, high-quality data ensures effective analysis. Second, selecting the right algorithms is crucial for generating actionable insights from the data. Challenges may arise, particularly concerning data privacy and ensuring prediction accuracy, but solutions exist. Techniques to enhance precision and address privacy concerns are essential to building trust and achieving reliable results.
Understanding these facets not only enhances the predictive capability of AI agents but also empowers organizations to adapt to changing consumer behaviors in real time. Embracing these insights ultimately leads to enhanced customer experiences and increased competitive advantage.
Key Principles of AI-Driven Behavior Prediction
AI-Driven Behavior Prediction hinges on several key principles that enhance its effectiveness in understanding user actions. One fundamental aspect is data collection and preparation. High-quality data is essential for accurate predictions, as it forms the foundation for any analytical process. Implementing rigorous data collection methods ensures that the information fed into the AI models is reliable and relevant, significantly influencing the outcomes of the behavior prediction.
Another crucial principle involves algorithm selection. Different algorithms can yield various insights depending on the nature of the data and the desired predictions. Selecting the right algorithm is pivotal, as it determines how well the AI can interpret patterns and trends within the data. When executed correctly, these principles guide organizations toward meaningful, actionable predictions that inform business strategies and improve user engagement.
- Data Collection and Preparation
Data collection and preparation are crucial steps in AI-Driven Behavior Prediction. High-quality data forms the foundation for accurate predictions, making it essential to gather comprehensive user behaviors and preferences systematically. Various methods such as surveys, interviews, and automated data scraping can be employed to collect relevant information effectively. Each method has its strengths, and selecting the right combination can enhance the reliability of the insights gained.
Once the data is collected, preparation becomes vital. This involves cleaning, transforming, and normalizing the data to ensure it is usable for analysis. Data consistency must be ensured, as discrepancies can lead to misleading predictions. Moreover, segmenting data based on demographic or psychographic factors can provide deeper insights into user behavior. Ultimately, thorough data collection and preparation directly impact the success of AI-Driven Behavior Prediction, setting the stage for meaningful analysis and actionable insights.
Understanding the importance of high-quality data collection methods.
High-quality data collection methods are crucial in the realm of AI-driven behavior prediction. These methods ensure that the data entered into AI systems is accurate, reliable, and relevant to the behaviors being analyzed. When data is meticulously gathered and curated, it enhances the effectiveness of predictive models, allowing them to produce more insightful and actionable results.
Moreover, strong data collection practices lay the foundation for understanding user behavior patterns. Inaccurate or biased data can lead to erroneous insights, negatively impacting decision-making. Therefore, organizations must prioritize implementing systematic strategies and tools to gather data effectively. By investing in high-quality data collection, companies can bolster their AI capabilities and achieve a deeper understanding of customer behavior, ensuring that predictions are precise, trustworthy, and valuable.
- Algorithm Selection
In the realm of AI-Driven Behavior Prediction, selecting the right algorithm is a pivotal step. The choice of algorithm influences the effectiveness and accuracy of predictions made by AI agents. Algorithms can vary in complexityโfrom simple linear regression to advanced neural networksโeach having its strengths and limitations, depending on the specific behavioral patterns being analyzed.
Understand Data Characteristics: Before selecting an algorithm, it is crucial to analyze the nature of the data. Consider factors such as volume, variety, and velocity, which significantly influence performance.
Evaluate Algorithm Requirements: Each algorithm comes with specific requirements, including data preprocessing and parameter tuning. Make sure to comprehend these needs to achieve optimal results.
Consider Scalability and Efficiency: When implementing predictive models, assess the scalability and computing resources required for each algorithm. Choosing a scalable solution ensures that your predictive capabilities grow with your data.
By thoroughly evaluating these factors, you can make a well-informed choice in your algorithm selection, leading to improved predictability and actionable insights in behavioral analytics.
Choosing the most suitable algorithms for effective behavior prediction.
Selecting the most suitable algorithms for effective behavior prediction is crucial for businesses aiming to harness AI-Driven Behavior Prediction. Organizations must begin by identifying their specific objectives and the type of behavior they wish to predict, whether itโs customer purchasing habits or user engagement patterns. The effectiveness of the prediction hinges on aligning the chosen algorithms with the data characteristics and the goals set by the organization.
To facilitate this process, consider the following important factors. First, data quality plays a pivotal role, as cleaner, well-structured data significantly enhances algorithm performance. Second, algorithm complexity should match the use case; simpler models may suffice for basic predictions, while complex scenarios could require advanced methods like neural networks. Lastly, computation efficiency is essential, especially for real-time applications; algorithms must provide timely insights without overwhelming technical resources. By carefully evaluating these aspects, organizations can make informed selections that optimize their AI-Driven Behavior Prediction initiatives.
Challenges and Solutions
In the pursuit of AI-driven behavior prediction, several challenges arise that need careful consideration. Data privacy concerns are paramount, as organizations must navigate regulations while ensuring that user data is collected and utilized ethically. Balancing the need for comprehensive data with the protection of individual privacy requires transparent practices and robust security measures. Furthermore, obtaining informed consent from users enhances trust and fosters positive relationships.
Another significant challenge is ensuring accuracy in predictions. AI models can only be as good as the data they are trained on, making high-quality data collection and algorithm selection critical. Techniques like continuous model evaluation and refinement can enhance precision and reliability. Regularly revisiting algorithms and data sources ensures that insights remain relevant and actionable. By addressing these challenges, organizations can leverage AI-driven behavior prediction to gain a competitive edge while maintaining ethical standards.
- Data Privacy Concerns
The growing reliance on AI-Driven Behavior Prediction raises significant data privacy concerns. As organizations leverage advanced algorithms to gather and analyze user behavior, the potential misuse of sensitive data becomes a pressing issue. Individuals worry about how their personal information is being collected, stored, and implemented. With AI technologies, especially in predictive analytics, itโs essential to prioritize transparency and ethical data practices.
In tackling these privacy dilemmas, key strategies come into play. First, organizations must adhere to robust data protection standards. Second, implementing clear user consent protocols is vital for fostering trust. Third, regular audits of data practices can ensure compliance with regulations such as GDPR. Users deserve clarity on what data is collected and how itโs utilized, reinforcing confidence in the predictive models in use. Addressing these concerns effectively not only strengthens user loyalty but also enhances the credibility of AI-driven initiatives.
Addressing issues related to user data privacy and security in predictive analysis.
In todayโs landscape of AI-Driven Behavior Prediction, attentively addressing user data privacy and security remains paramount. Organizations harnessing predictive analysis must adopt robust strategies to safeguard sensitive information. These measures not only protect users but also build trust, ensuring compliance with regulations like GDPR. Transparent data usage and proactive communication regarding data handling practices can significantly mitigate user concerns.
To effectively address privacy and security issues, consider the following aspects:
Data Anonymization: By removing personally identifiable information from datasets, organizations can protect individual identities while still gaining valuable insights from aggregated data.
User Consent: Gaining explicit consent for data collection fosters a sense of agency among users. Transparent consent processes enhance trust, allowing users to feel secure about how their data is utilized.
Regular Audits: Conducting frequent assessments of data management practices helps to identify vulnerabilities. This step can not only enhance security but also align operations with current compliance requirements.
Encryption Protocols: Implementing advanced encryption safeguards data at rest and in transit, ensuring unauthorized parties cannot easily access sensitive information.
By integrating these practices, companies can effectively navigate the complexities of user data privacy while leveraging AI-Driven Behavior Prediction for actionable insights.
- Ensuring Accuracy
Ensuring accuracy in AI-driven behavior prediction is crucial for developing reliable insights. The first key aspect involves data validation, ensuring that the collected data is complete and representative of the target user base. Without accurate data, the predictions generated can lead to misleading insights, affecting decision-making processes.
Another critical element is the refinement of algorithms. Continuous testing and adjusting of the machine learning models ensure they adapt to new data patterns and trends. By employing techniques such as cross-validation, developers can enhance the predictive accuracy over time. Lastly, implementing feedback loops allows AI systems to learn from past predictions, thereby improving future outcomes. Building trust in AI-driven behavior prediction hinges on these robust methods, aligning technical accuracy with user expectations. By prioritizing these steps, organizations can significantly elevate the reliability of the insights they generate.
Techniques to improve the precision and reliability of AI-driven predictions.
To enhance the precision and reliability of AI-driven predictions, it is essential to focus on several key techniques. First, ensuring high-quality data is paramount. This involves implementing rigorous data collection practices that eliminate inconsistencies and biases, thus providing a solid groundwork for analysis. Additionally, data preparation should include cleaning and structuring to facilitate effective model training.
Next, selecting the most appropriate algorithms is crucial for the success of AI-driven behavior prediction. Each machine learning algorithm has its strengths and is suited for specific types of data and prediction tasks. Experimenting with different algorithms can lead to improved performance, allowing for a more nuanced understanding of user behavior patterns. Fine-tuning these algorithms through hyperparameter optimization can further enhance predictive accuracy.
Another vital aspect is incorporating a feedback loop, which enables continuous learning and adaptation of models to new data. This iterative process helps in addressing drift in user behavior, ensuring predictions remain relevant and accurate over time. By focusing on these techniques, organizations can significantly elevate the quality and reliability of their AI-driven predictions.
๐ฌ Questions about Predictive Behavioral Data Insights with AI Agents?
Our team typically responds within minutes
Extract insights from interviews, calls, surveys and reviews for insights in minutes
Tools and Technologies Powering AI-Driven Behavior Prediction
Tools and technologies play a crucial role in the realm of AI-driven behavior prediction. These solutions empower businesses to transform vast amounts of data into actionable insights. Advanced AI tools streamline the analysis process, making it easier to recognize patterns and trends that might otherwise go unnoticed. By utilizing tools such as insight7, Google Cloud AI, IBM Watson, and Microsoft Azure AI, organizations can harness the power of machine learning algorithms to make accurate predictions based on user behavior.
Moreover, implementing predictive models involves several key steps that enhance the overall effectiveness of behavior prediction efforts. First, clearly defining objectives ensures that the analysis aligns with business goals. Next, selecting and training models is essential for fitting the data precisely. This comprehensive approach not only improves accuracy but also drives more informed decision-making, ultimately leading to a competitive edge in today's data-driven market.
Top AI Tools for Behavioral Analysis
A variety of powerful AI tools can play a critical role in behavioral analysis. Notably, these tools enable businesses to gain insights into customer interactions, leading to informed decision-making. The effectiveness of AI-driven behavior prediction relies on employing the right technology to sift through vast amounts of data.
First on the list, Insight7 offers advanced algorithms designed for comprehensive behavioral insights. Its robust nature allows users to analyze customer conversations and extract valuable signals efficiently. Next, Google Cloud AI stands out for its machine learning capabilities, providing powerful prediction models tailored for diverse datasets. IBM Watson follows closely, utilizing its sophisticated AI to both predict and analyze user behavior, allowing for strategic planning. Lastly, Microsoft Azure AI offers a comprehensive platform for behavior prediction, integrating various AI tools to enhance accuracy and efficiency. Each tool is pivotal for businesses aiming to optimize their predictive analytics through AI-driven methods.
- insight7
AI-Driven Behavior Prediction plays a pivotal role in enhancing our understanding of customer interactions. Through innovative algorithms, we can analyze vast amounts of data to predict behavior more accurately. This predictive capacity allows businesses to engage customers proactively, refining strategies based on emerging patterns and insights.
To successfully implement AI-Driven Behavior Prediction, several key components must be addressed. First, high-quality data collection ensure the accuracy of predictions, while the right algorithms facilitate effective behavior analysis. Businesses also need to remain mindful of data privacy concerns, reassuring customers that their information is handled securely. Moreover, it is crucial to continuously improve the models to maintain the precision and reliability of the insights generated. By aligning these elements, organizations can position themselves strongly against competitors and respond to customer needs with agility and effectiveness.
A tool providing comprehensive behavioral insights through advanced AI algorithms.
A tool offering comprehensive behavioral insights operates through advanced AI algorithms. These algorithms analyze vast amounts of data to identify patterns and trends in user behaviors. By synthesizing this information, businesses can gain invaluable insights into customer preferences and pain points, enabling informed decision-making and strategic planning.
The core functionalities of this AI-driven behavior prediction tool include seamless data collection, nuanced behavioral analysis, and intuitive reporting. Users can easily access a library of recorded interactions, where the tool transcribes calls and highlights significant insights. This process not only uncovers pain points but also reveals customer desires and sentiments. By democratizing data access, organizations empower all team members to utilize these insights, paving the way for improved customer experiences and ultimately driving business success. The advanced algorithms form a critical backbone, ensuring the precision and relevance of the insights generated.
- Google Cloud AI
Google Cloud AI is a powerful resource for enhancing predictive behavioral data insights through advanced machine learning techniques. By integrating AI-driven behavior prediction, organizations can unveil vital patterns in user interactions and trends. This insight equips businesses to tailor their services more effectively and meet customer needs with precision.
Central to Google Cloud AIโs offerings is its scalable infrastructure, which allows for extensive data analysis. The machine learning capabilities facilitate the identification of significant behavioral trends, thus enabling data-driven decision-making. Additionally, the platform supports various algorithms that can be fine-tuned to improve prediction accuracy. As businesses strive to adapt in a rapidly changing environment, utilizing Google Cloud AI can provide a competitive advantage. With these tools, organizations are better positioned to understand behavioral shifts and optimize their engagement strategies accordingly.
Leveraging machine learning models for data-driven predictions.
Machine learning models are essential for making accurate, data-driven predictions in today's landscape of AI-driven behavior prediction. These models work by analyzing vast datasets to identify patterns and trends that could influence user behavior. For example, organizations can use historical data to train models that anticipate future actions, enabling more informed decision-making.
The implementation of these predictive models involves several crucial steps. First, defining clear objectives helps in tailoring the model to specific behavioral predictions. Next, selecting and training the most appropriate algorithms ensures the model effectively captures relevant behaviors from the datasets. Finally, continuously testing and refining these models improves their accuracy, delivering more reliable insights over time. By focusing on these aspects, organizations can significantly enhance their understanding of consumer behavior and optimize their strategies accordingly.
- IBM Watson
IBM Watson stands at the forefront of AI-driven behavior prediction, offering powerful capabilities to transform user data into actionable insights. By utilizing advanced algorithms, it analyzes behavioral patterns, helping businesses understand customer intentions and preferences better. The AI system enhances predictive accuracy, allowing organizations to forecast trends based on historical data, all while ensuring compliance with data privacy regulations.
One of the notable features of Watson is its ability to process vast amounts of unstructured data. This includes text from customer interactions, feedback, and social media. By identifying sentiment and extracting valuable information, it provides a holistic view of customer behavior. Organizations can leverage these insights to refine their strategies and improve decision-making processes. Ultimately, IBM Watson exemplifies how AI-driven behavior prediction can empower companies to engage their audiences more effectively.
Utilizing Watsons AI capabilities to predict and analyze user behavior.
Harnessing Watson's AI capabilities allows businesses to gain valuable insights into user behavior. By employing advanced algorithms, organizations can analyze vast amounts of data, uncovering patterns and trends that traditional methods may overlook. These insights help predict future actions and preferences, enabling targeted engagement strategies. Companies can refine their offerings based on predictive behavioral data, enhancing customer satisfaction and loyalty.
To maximize the effectiveness of AI-driven behavior prediction, several key practices should be followed. First, ensure data collection is thorough and clean, as high-quality data is crucial for accurate predictions. Next, invest time in selecting the right algorithms tailored to specific user behavior scenarios. Lastly, prioritize user privacy by implementing robust security measures to build trust with your user base. This structured approach ultimately transforms data into actionable insights, supporting informed decision-making and strategic planning.
- Microsoft Azure AI
Microsoft Azure AI provides a comprehensive suite of tools designed for AI-Driven Behavior Prediction. By utilizing advanced machine learning algorithms, it equips organizations with the capabilities to analyze vast datasets, glean insights, and forecast user behaviors effectively. This platform can streamline your processes, enabling quicker and more accurate decision-making based on predictive analytics.
The ecosystem integrates seamlessly with existing workflows, allowing businesses to foster innovation while addressing user needs. One of its key features is the ability to handle diverse datasets, ensuring that the AI tools powered by Microsoft Azure can adapt to various scenarios. Furthermore, by applying rigorous data security measures, the platform enhances user confidence while performing predictive analysis. As organizations increasingly rely on data-driven strategies, tools like Microsoft Azure AI become essential, enabling insights that drive meaningful change and elevate user experiences.
Offering robust solutions for behavior prediction through its AI platform.
AI-Driven Behavior Prediction offers organizations a powerful framework for understanding customer actions and preferences. By utilizing advanced algorithms, businesses can analyze vast amounts of data to uncover trends and patterns. This data-driven approach allows for accurate predictions of customer behavior, enabling companies to make informed decisions and enhance their marketing strategies.
One core aspect of this AI platform is its ability to streamline the analysis process. Traditional methods often struggle to keep pace with the increasing volume of data, resulting in slow delivery times and fragmented insights. However, with robust AI tools, organizations can quickly transform raw data into actionable insights. This not only improves efficiency but also ensures that teams can collaborate effectively, ultimately driving better business outcomes. Adopting AI-driven behavior prediction solutions positions companies to stay ahead in a competitive market, empowering them with timely and relevant insights.
Implementing Predictive Models
To effectively implement predictive models, it's crucial first to define clear objectives. Begin by identifying the specific behaviors or trends you want to predict using AI-driven behavior prediction. This focused approach ensures that your analysis effectively addresses relevant questions and aligns with your business goals.
Next, select and train models tailored to your data. This process involves choosing the right algorithms based on the type of insights you seek and ensuring they are trained on high-quality datasets. A consistent evaluation of the models is necessary, allowing adjustments to improve accuracy over time. Regularly refining these models will enhance their predictive capabilities, ensuring they remain relevant in a dynamic environment. By following these steps, you position your organization to reap the benefits of AI-driven behavior prediction, unlocking valuable insights that can inform strategic decisions.
- Step 1: Define Objectives
Defining clear objectives is the cornerstone of successful AI-driven behavior prediction. Start by identifying what you want to achieve with your analysis, whether that means increasing customer satisfaction or improving product recommendations. A well-defined objective sets the direction for your entire project and helps ensure your predictive analysis aligns with your business goals.
Next, consider the key metrics that will gauge your success. For instance, if your objective focuses on enhancing user engagement, relevant metrics might include click-through rates and user retention rates. Well-defined objectives streamline your efforts, making it easier to select the appropriate algorithms and tools. By focusing on the purpose of your analysis, you can cultivate actionable insights that lead to better decision-making and ultimately drive growth in your organization.
Establish the goals of your AI-driven predictive analysis.
Establishing the goals of your AI-driven predictive analysis is crucial for effective implementation. Clear objectives guide the entire process, allowing teams to focus on specific outcomes. To start, identify what insights you aim to gain from AI-driven behavior prediction. These may include understanding customer needs, enhancing user experience, or predicting market trends.
Next, prioritize your goals. Consider combining immediate objectives, such as improving customer service efficiency, with long-term aspirations like personalized marketing strategies. Utilizing a structured approach ensures that every stage of your predictive analysis aligns with these goals. This alignment fosters an environment where data is not only collected but analyzed meaningfully. The ultimate benefit lies in harnessing predictive insights that drive strategic decisions, leading to improved business performance and customer satisfaction. Clarity in goal setting sets the foundation for creating actionable, meaningful insights from your AI-driven predictive analysis.
- Step 2: Select and Train Models
Selecting and training models is a crucial step in AI-driven behavior prediction. The objective is to identify models that effectively interpret the collected data and yield accurate insights about user behaviors. Begin by exploring different algorithms based on the nature of your data and desired outcomes. Common choices include decision trees, neural networks, and support vector machines, each offering unique strengths.
Once you've selected models, training comes next. Itโs essential to divide your dataset into training and validation subsets. The training dataset enables the model to learn patterns, while the validation set tests its performance. Continuous evaluation during this phase helps in fine-tuning parameters and improving accuracy. Ultimately, the right selection and training of models will empower your AI agents to provide nuanced behavioral insights, enhancing decision-making and strategic planning.
Process of model training to fit the data for effective predictions.
The model training process is essential for delivering accurate predictions in AI-driven behavior prediction. Initially, data quality is paramount; clean, organized, and relevant datasets lay the foundation for any successful model. Once the data is gathered, the next step is to define clear objectives and criteria tailored to the specific prediction goals. This ensures that any predictive model aligns closely with real-world scenarios and insights.
Subsequently, algorithm selection takes center stage, as the choice of algorithm significantly impacts the prediction's effectiveness. Training the model involves feeding it these curated datasets, allowing it to identify patterns and relationships. Continuous evaluation and adjustments based on performance metrics refine the model, enhancing its ability to make predictions accurately. Over time, this iterative training process produces models that not only fit the data but also provide valuable insights for behaviors, driving informed decision-making.
Conclusion: Advancing Predictive Insights with AI-Driven Behavior Prediction
AI-Driven Behavior Prediction represents a fundamental shift in how organizations derive insights from data. This approach not only enhances decision-making but also empowers businesses to understand customer preferences on a deeper level. By utilizing advanced algorithms, companies can transform raw data into actionable intelligence that drives strategic initiatives.
Moreover, the integration of AI in predictive analytics addresses many challenges, such as data accuracy and privacy concerns. As organizations continue to navigate this landscape, the focus will remain on optimizing AI-driven insights to ensure relevance and reliability in predictions. Ultimately, embracing this technology will enable businesses to stay ahead in competitive markets.
๐ฌ Questions about Predictive Behavioral Data Insights with AI Agents?
Our team typically responds within minutes