Predictive Analytics in Qualitative Research: Opportunities and Challenges
-
Bella Williams
- 10 min read
Exploring the qualitative forecasting potential of predictive analytics opens new avenues for understanding complex data. In qualitative research, traditional methods often rely on subjective interpretation, but integrating predictive analytics can enhance accuracy and foresight. By analyzing patterns and trends, researchers can uncover insights that drive informed decision-making.
The rise of predictive models presents a unique opportunity to bridge qualitative and quantitative data. These models not only improve trend analysis but also enrich market insights, benefiting organizations that aim to stay ahead in their fields. Understanding this potential enables researchers to leverage data effectively while navigating the unique challenges that arise in qualitative forecasting.
Analyze & Evaluate Calls. At Scale.
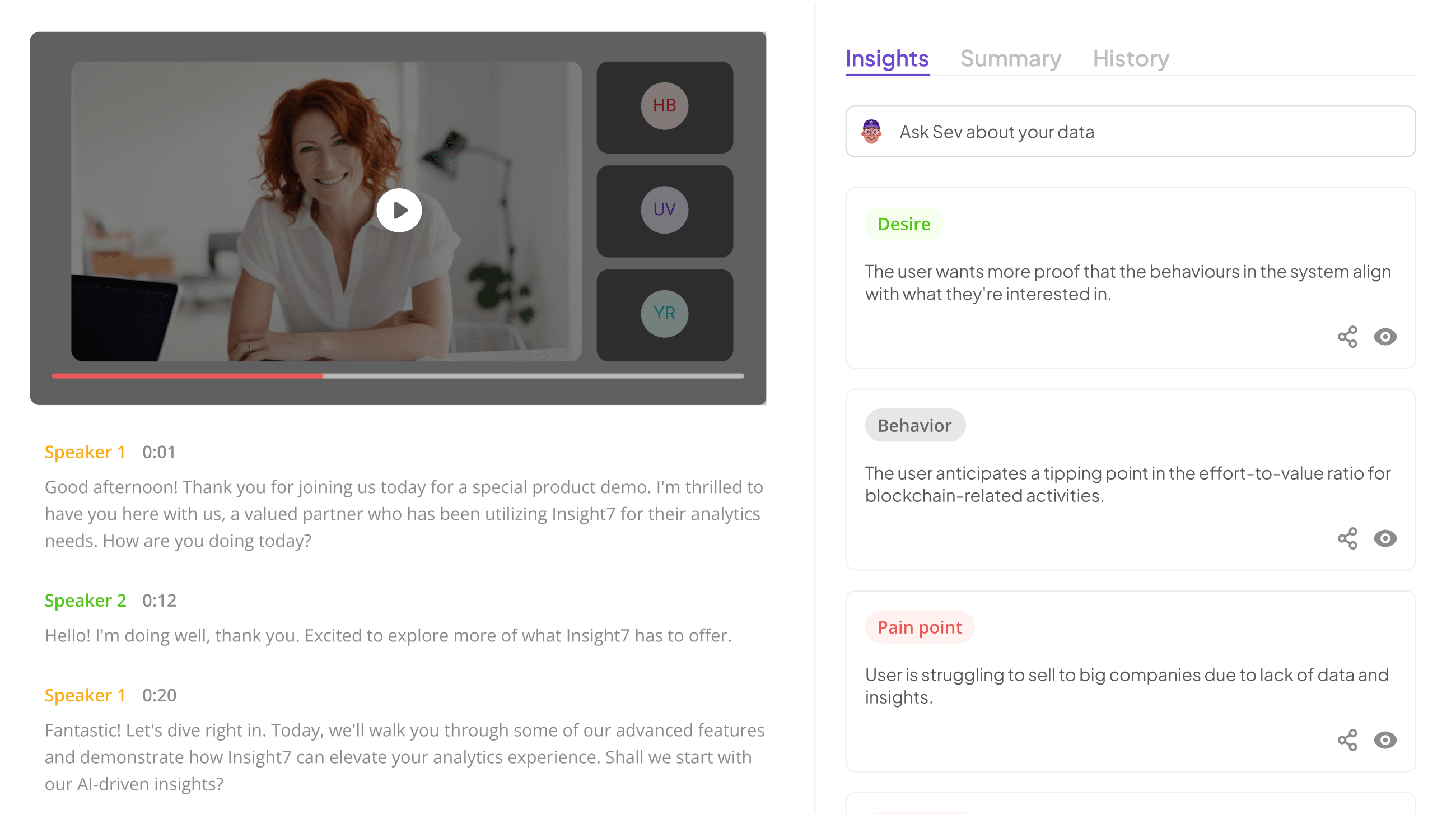
Unveiling the Qualitative Forecasting Potential: Opportunities in Predictive Analytics
The potential of qualitative forecasting in predictive analytics is gradually being recognized as a key asset in research methodologies. By integrating qualitative insights with predictive models, researchers can gain a deeper understanding of complex data patterns. This fusion not only enhances forecasting accuracy but also enriches the overall data analysis process, making it more comprehensive and reliable.
Several opportunities arise from this integration. First, predictive analytics can significantly improve decision-making processes by offering actionable insights derived from qualitative data. Second, enhanced trend analysis is achieved by mining historical qualitative information, allowing businesses to anticipate changes in consumer behavior. Together, these advancements pave the way for enriched market insights, enabling organizations to make better-informed strategic decisions while navigating today's dynamic landscape. By embracing the qualitative forecasting potential, researchers transform raw data into meaningful narratives that drive growth and innovation.
The Role of Predictive Models in Qualitative Research
Predictive models play a pivotal role in advancing qualitative research by harnessing data-driven insights. By applying these models, researchers can deepen their understanding of qualitative data, transforming anecdotes into actionable predictions. This integration unveils patterns and themes that might otherwise remain obscured, allowing for more nuanced interpretations of human behaviors and preferences.
Moreover, bridging qualitative and quantitative approaches enhances the robustness of conclusions drawn from research. For instance, using predictive analytics facilitates trend analysis and market insights, empowering organizations to make informed decisions. Understanding these dynamics of qualitative forecasting potential fosters a richer exploration of data, driving innovative solutions in various fields. As researchers begin to embrace the power of predictive models, they are better equipped to uncover meaningful narratives that inform strategies and address challenges.
- Understanding Qualitative Data
Qualitative data offers rich, contextual insights that quantitative data often overlooks. It includes open-ended responses, interviews, or observations, capturing personal experiences and emotions. For researchers utilizing predictive analytics, understanding these data types opens avenues to enhance the qualitative forecasting potential. This potential lies in analyzing themes, sentiments, and narratives, providing a deeper understanding of trends and consumer behaviors.
To effectively harness qualitative data, researchers should focus on three essential elements. First, they must collect diverse data sources, such as customer interviews or focus groups, to capture a wide range of perspectives. Second, appropriate analytical frameworks are crucial for deriving meaningful insights from narratives, allowing the identification of patterns. Lastly, integrating qualitative findings with quantitative metrics can significantly enhance overall decision-making efficacy, offering a more holistic view of market dynamics. Through this approach, researchers can truly unlock the forecasting power of qualitative data within predictive models.
- Bridging Quantitative and Qualitative Approaches
Bridging quantitative and qualitative approaches involves creating a synthesis that enhances qualitative forecasting potential. By integrating statistical analysis with narrative insights, researchers can gain a comprehensive understanding of complex phenomena. This interconnectedness allows for richer data interpretation, enabling patterns and trends to emerge that may be overlooked when relying solely on one method.
Combining these approaches fosters a holistic view of research subjects, yielding insights that quantitatively-driven data alone might miss. For instance, qualitative narratives can provide context to statistical anomalies, helping to explain why certain trends appear in the data. Ultimately, bridging these methods not only supports accurate predictions but also enriches the decision-making process, making it more informed and nuanced. Emphasizing both quantitative data and qualitative insights can unlock new opportunities for predictive analytics, ensuring research outcomes are robust and effective.
Key Benefits and Applications
Predictive analytics offers a wealth of benefits and applications within qualitative research, especially in enhancing understanding of consumer behavior. One of the key advantages is its ability to improve decision-making processes. By utilizing data-driven insights, organizations can make more informed choices that align with customer needs and market trends. This transition from intuition-based to data-driven decisions marks a significant evolution in strategic planning.
Another essential application lies in the realm of trend analysis and market insights. Predictive models enable researchers to identify emerging patterns, allowing businesses to stay ahead of competitors. Such foresight can guide product development and marketing strategies, ultimately leading to better customer engagement. Overall, recognizing the qualitative forecasting potential of predictive analytics can transform how organizations gather and utilize information, paving the way for innovative approaches in understanding market dynamics.
- Enhancing Decision-Making Processes
Effective decision-making is crucial in any research process, particularly when integrating predictive analytics into qualitative research. By understanding qualitative forecasting potential, researchers can make informed choices that lead to better outcomes. Recognizing patterns and insights within qualitative data empowers decision-makers to evaluate options accurately and act strategically.
One way to enhance decision-making is by establishing clear frameworks for evaluating qualitative data. This includes defining specific criteria for success and aligning them with organizational goals. Another critical aspect is fostering collaboration among team members to ensure diverse perspectives shape the decision-making process. Finally, leveraging feedback loops allows for continuous improvement by adapting to new insights as projects evolve. These strategies ensure that choices made are not just reactive but are rooted in comprehensive analysis, ultimately enhancing the effectiveness of qualitative forecasting potential.
- Improving Trend Analysis and Market Insights
Improving trend analysis and market insights requires a multifaceted approach. By integrating predictive analytics with qualitative research, organizations can gain a deeper understanding of market dynamics. This blend enhances decision-making by identifying patterns within consumer behavior, allowing businesses to anticipate shifts in demand. Ultimately, this leads to more informed strategies.
To optimize qualitative forecasting potential, there are effective methods to consider. First, employing advanced data visualization tools can help interpret qualitative feedback clearly. These tools convert complex data into easily digestible insights. Second, conducting regular sentiment analysis provides an overview of customer opinions, which can be segmented based on specific demographics. Lastly, fostering ongoing engagement with customers can help refine insights over time, ensuring that strategies remain relevant in a rapidly changing market landscape. Through these practices, organizations can significantly enhance their predictive capabilities.
💬 Questions about Predictive Analytics in Qualitative Research: Opportunities and Challenges?
Our team typically responds within minutes
Extract insights from interviews, calls, surveys and reviews for insights in minutes
Navigating the Challenges of Qualitative Forecasting Potential in Predictive Analytics
Qualitative forecasting potential offers immense possibilities but also presents unique challenges in predictive analytics. One primary challenge stems from the complexity of data involved in qualitative research. Analysts often need to manage diverse data sources, which can include interviews, surveys, and social media interactions. By ensuring these varied data types are integrated cohesively, organizations can draw more accurate conclusions.
Moreover, ensuring data quality and consistency is imperative. Without reliable data, predictions may lead to misguided strategies. Another significant hurdle involves ethical considerations. Predictive models can inadvertently introduce bias, which may skew results and impact decision-making. Developing strategies to identify and mitigate these biases is crucial for fostering trust and reliability in outcomes. Ultimately, navigating these challenges is essential for unlocking the transformative power of qualitative forecasting potential in predictive analytics.
Addressing Data Complexity and Integration
The complexity of data significantly influences the effectiveness of qualitative forecasting potential in predictive analytics. Managing diverse data sources is paramount to ensure a holistic view of insights gathered from various contexts. As organizations compile information from surveys, interviews, and transactional data, integrating these varied data sets becomes crucial for generating meaningful conclusions.
Moreover, ensuring data quality and consistency can mitigate issues inherent in predictive modeling. Inconsistent data can yield misleading insights, hindering decision-making processes. By employing robust data management strategies, including data cleaning and validation techniques, researchers can enhance the integrative capacity of their predictive models. This continuous refinement process not only elevates the accuracy of forecasts but also builds a more reliable foundation for informed strategic planning. By effectively addressing data complexity and integration, organizations can unlock the full potential of predictive analytics in qualitative research.
- Managing Diverse Data Sources
Managing diverse data sources is essential in qualitative research using predictive analytics. Different sources, such as interviews, surveys, and social media, each offer unique insights. However, consolidating these varied data types into a coherent framework can be challenging. It requires careful planning to ensure that each source is considered for its strengths and weaknesses.
Integrating these diverse data inputs can reveal patterns that enhance qualitative forecasting potential. One effective strategy is to categorize data into thematic groups. This allows researchers to identify key trends across distinct sources. Furthermore, employing tools that facilitate data comparison, such as AI-driven analytics, streamlines this process. Ultimately, managing diverse data sources effectively enhances the robustness of findings, leading to improved predictions and informed decision-making. By understanding and utilizing these varied inputs, researchers can significantly enhance the qualitative forecasting potential within their studies.
- Ensuring Data Quality and Consistency
Maintaining data quality and consistency is paramount when leveraging predictive analytics in qualitative research. Reliable data serves as the foundation for accurate insights and informed decision-making. The process begins with rigorous data collection, where diverse sources must be harmonized to form a cohesive dataset. Each entry should undergo a validation process to ensure it accurately represents the information being sought.
Additionally, regular audits and assessments of the data are essential. This helps identify inaccuracies or inconsistencies that may undermine the qualitative forecasting potential. By implementing standardized protocols for data entry and updating, researchers can minimize errors and discrepancies that could skew insights. Ultimately, consistent attention to data quality fosters trust in findings and supports the overarching goal of extracting meaningful conclusions from qualitative research efforts.
Ethical Considerations and Bias Management
Ethical implications play a crucial role in the application of predictive models within qualitative research. Researchers must ensure that these models do not perpetuate existing biases or create new ethical dilemmas. The use of predictive analytics can lead to unintended consequences if ethical considerations are overlooked. Therefore, it's vital to actively assess the potential impacts on diverse populations. By prioritizing ethical decision-making, researchers can harness the qualitative forecasting potential while maintaining integrity and social responsibility.
Bias management is equally important in predictive analytics. Data sources may contain inherent biases that can skew results. Thus, researchers should employ transparent methodologies to identify and mitigate these biases. Strategies may include using diverse data sets, conducting rigorous validation of models, and continuously revisiting ethical guidelines. Ensuring ethical integrity and minimizing bias not only enhances research outcomes but also fosters trust among stakeholders, resulting in more reliable insights in qualitative research.
- Ethical Implications of Predictive Models
Predictive models in qualitative research present both unique opportunities and profound ethical challenges. As we delve into the qualitative forecasting potential of these models, it becomes crucial to consider their implications. Ethical concerns primarily revolve around data privacy, consent, and the potential for misuse of collected information. Researchers must ensure that individuals' data is handled responsibly, maintaining transparency about how it is used.
Moreover, the risk of bias in model predictions can lead to misinterpretations and outright discrimination if not managed carefully. This necessitates ongoing scrutiny of the algorithms behind predictive models to ensure fairness. Adopting comprehensive strategies to mitigate these biases, such as continuous feedback loops and inclusive datasets, is essential for cultivating trust in predictive analytics. By acknowledging and addressing these ethical considerations, researchers can harness the full qualitative forecasting potential while safeguarding participant rights and promoting equity.
- Strategies for Mitigating Bias
Mitigating bias in qualitative research is essential for maximizing the qualitative forecasting potential of predictive analytics. The first step involves establishing a clear framework for data collection. Researchers should consciously design questions that avoid leading responses, ensuring participants express genuine views without influence. This practice enhances the integrity of the findings.
Furthermore, employing multiple data sources can significantly reduce biases. By triangulating insights from interviews, focus groups, and surveys, researchers can build a more holistic understanding. Engaging diverse participant demographics also ensures that varied perspectives are represented, adding depth to the findings. Lastly, continuous reflection on personal biases is crucial; researchers must actively question their assumptions and be open to feedback regarding their methodologies. This self-awareness fosters an environment where insights are recognized for their validity, enhancing the overall quality and reliability of qualitative analytics.
Conclusion: Harnessing the Qualitative Forecasting Potential in Predictive Analytics
In harnessing the qualitative forecasting potential, organizations can unlock deeper insights from predictive analytics. By integrating qualitative data with traditional predictive models, analysts can capture nuanced consumer behaviors that numbers alone may overlook. This holistic approach not only enriches understanding but also enhances decision-making, allowing businesses to tailor strategies more effectively.
As we move forward, embracing this potential is essential for navigating complexities in data and addressing bias that may arise in qualitative research. By prioritizing data quality and ethical considerations, organizations can create predictive frameworks that genuinely reflect human experiences and preferences. Ultimately, this commitment paves the way for more accurate forecasts and impactful outcomes in the ever-evolving landscape of predictive analytics.
💬 Questions about Predictive Analytics in Qualitative Research: Opportunities and Challenges?
Our team typically responds within minutes