Popular Data Analysis Tools Pros And Cons
-
Hello Insight
- 10 min read
In the rapidly evolving world of data analysis, the selection of tools has become paramount for organizations aiming to extract actionable insights. A thorough data tool comparison is essential for understanding the unique capabilities and limitations of each tool, ensuring the chosen solution aligns with specific analytical needs. With a plethora of options available, data analysts face the challenge of navigating various features to identify the best fit for their projects.
As we delve into this comparison, we will evaluate popular data analysis tools based on their strengths and drawbacks. Understanding the nuances between tools like Tableau, Power BI, and open-source coding options can significantly impact the effectiveness of data-driven decision-making. This analysis aims to empower users with the knowledge needed to make informed choices in their data analysis journey.
Analyze & Evaluate Calls. At Scale.
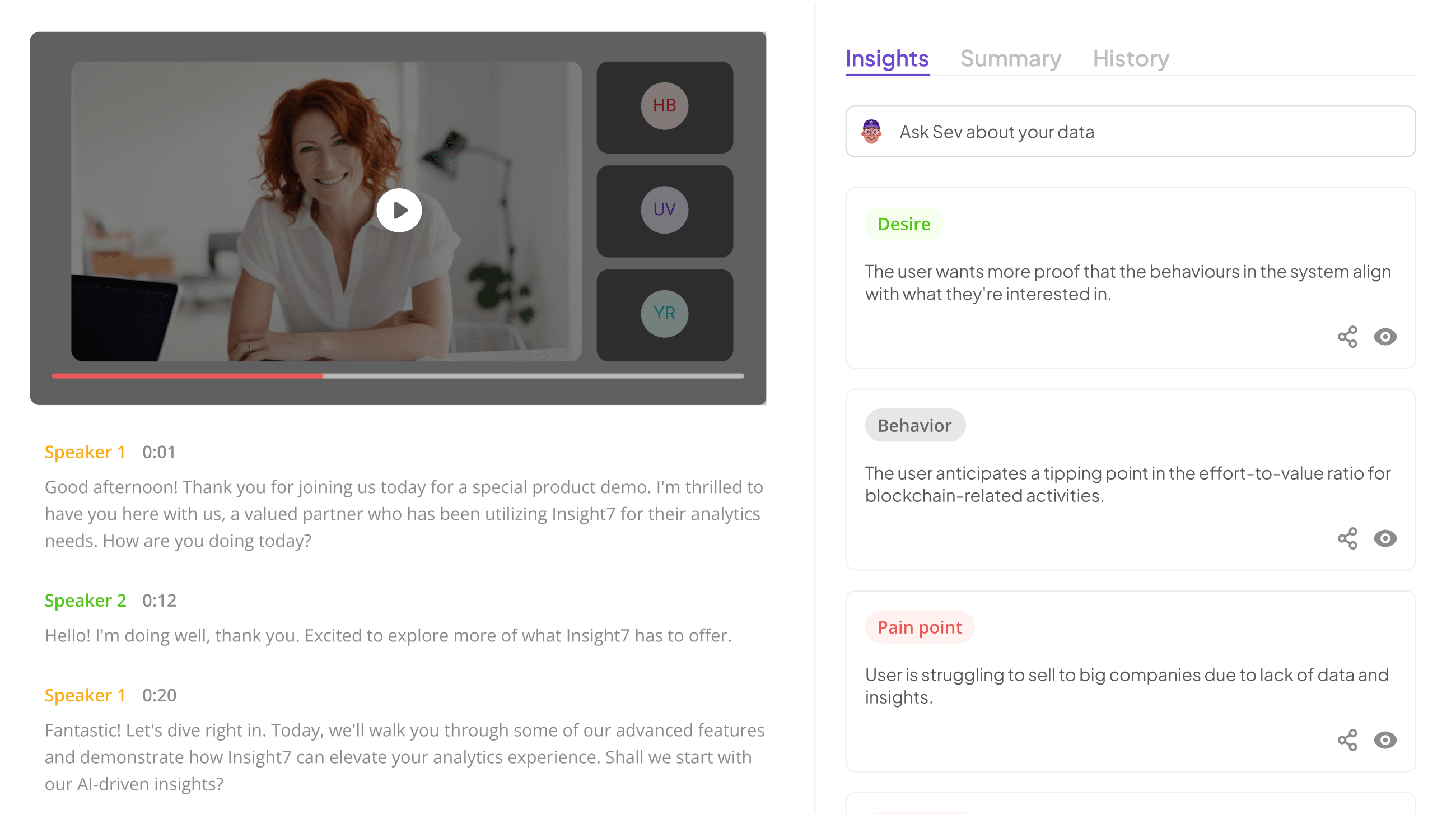
Comparing the Top Data Analysis Tools
When conducting a data tool comparison, it’s essential to understand the unique features and capabilities of each option available. Various data analysis tools have emerged, each with strengths that cater to different user needs and sectors. Selecting the right tool requires evaluating how well it aligns with your specific requirements and workflows.
Each popular data analysis tool offers distinct advantages and limitations. For instance, Tableau is renowned for its exceptional visualization capabilities, making it perfect for presenting data intuitively. Power BI, on the other hand, excels in integrating seamlessly within the Microsoft ecosystem, favoring those already utilizing Microsoft products. Meanwhile, R Programming and Python libraries provide robust statistical and analytical capabilities, appealing to users needing deeper data insights. Analyzing these factors is crucial to making informed decisions when choosing data analysis tools for your projects.
insight7: Revolutionizing Data Analysis
Data analysis has undergone a remarkable transformation due to the rise of advanced tools tailored for various needs. Insight7 exemplifies this revolution, providing a user-friendly, self-service platform that streamlines the analysis of customer data at scale. With increased volumes of customer interactions, companies are finding it essential to analyze these signals effectively. Traditional methods struggle to keep pace, leading to inefficiencies in insight extraction and application.
The shift toward modern data tools emphasizes not only the need for better analysis but also for timely insights that can drive competitive advantage. These platforms promote collaboration and simplify the process of understanding complex data. A key takeaway is that organizations must adopt solutions that allow for agile decision-making. This evolution in data tool comparison not only enhances efficiency but also cultivates a proactive culture focused on customer engagement and tailored solutions.
Advanced Features in Other Notable Tools
In exploring the advanced features of various popular data analysis tools, a few stand out for their unique capabilities. Tableau is highly regarded for its exceptional visualization options. It allows users to create dynamic and interactive dashboards, facilitating a deeper understanding of data trends and patterns. This makes it an ideal choice for those who prioritize visual storytelling in their data presentations.
Power BI, on the other hand, offers seamless integration with the Microsoft ecosystem. This feature is particularly beneficial for organizations already using Microsoft products, as it allows for easy data manipulation and reporting. Similarly, R programming shines as a statistical powerhouse, ideal for those who require advanced analytical techniques. Last but not least, Python libraries add versatility and flexibility, catering to both data analysis and machine learning needs. These distinctions form the backbone of a comprehensive data tool comparison, guiding users in selecting the best tool for their unique requirements.
- Tableau: Visualization Excellence
Tableau excels in transforming complex datasets into clear, interactive visualizations. This tool allows users to create stunning graphics and dashboards, making data interpretation accessible to both technical and non-technical stakeholders. With its drag-and-drop interface, users can analyze data trends and patterns effortlessly. The quality of visual representation plays a crucial role in effective data storytelling, which is where Tableau shines.
However, when evaluating a Data Tool Comparison, it’s essential to consider both strengths and challenges. While Tableau's capabilities are impressive, the software may face a learning curve for beginners. Additionally, costs can be a factor, especially for small businesses or individual users. Despite these considerations, Tableau remains a favorite among data professionals aiming for visualization excellence, helping organizations derive actionable insights from their data. In summary, Tableau offers powerful visualization options, but potential users should weigh its ease of use against cost and complexity.
- Power BI: Seamless Integration with Microsoft Ecosystem
Power BI excels in providing seamless integration with the Microsoft ecosystem, making it a preferred choice for businesses already using Microsoft tools. This integration allows users to easily connect Power BI with various data sources like Excel, Azure, and SharePoint, ensuring a smooth workflow. Users can import data directly from these platforms without the need for complicated setup processes. The convenience of sharing dashboards and reports across these applications enhances collaboration and data accessibility.
Additionally, this integration supports real-time data updates, crucial for dynamic business environments. Teams can analyze their data visually and interactively, promoting data-driven decision-making. However, while Power BI shines in integration, it may fall short in advanced analytical capabilities compared to specialized tools. Thus, for those already invested in the Microsoft ecosystem, Power BI stands out as an efficient option, yet it's essential to evaluate its features in the broader context of a comprehensive data tool comparison.
- R Programming: Statistical Powerhouse
R has gained a strong reputation as a statistical powerhouse in the realm of data analysis. Its extensive collection of packages enables users to perform complex statistical tests and advanced analyses with relative ease. This versatility makes R particularly appealing for statisticians, researchers, and data scientists aiming to derive meaningful insights from their data.
In a data tool comparison, R stands out for its powerful visualization capabilities, allowing for clear presentation of results. The vibrant community around R continuously contributes packages and support, making it a robust choice for varied analysis needs. However, its learning curve can present challenges for beginners, which could lead to frustration when compared to more user-friendly tools. Ultimately, R’s efficiency and depth make it an invaluable asset for those willing to invest time in mastering its capabilities.
- Python Libraries: Versatility and Flexibility
Python libraries stand out in data analysis due to their remarkable versatility and flexibility. This adaptability allows users from various backgrounds to manipulate data effortlessly and derive meaningful insights. Whether you're working with small datasets or dealing with complex algorithms, Python's extensive libraries cater to different analytical needs, making it a go-to choice for both beginners and seasoned professionals.
Moreover, the active community and plethora of resources enhance the usability of these libraries. Each library—such as pandas for data manipulation or Matplotlib for visualization—provides unique features tailored to specific tasks. This variety fosters a culture of exploration, encouraging users to experiment with their data. In a data tool comparison context, Python libraries demonstrate how blending usability with functionality can lead to effective data analysis. Ultimately, the versatility and flexibility of Python ensure that it remains a top choice among diverse analytical tools.
[ hfe_template id=22694]In-Depth Data Tool Comparison: Pros and Cons
Data Tool Comparison reveals the nuances between various popular data analysis tools. Each tool presents distinct strengths and weaknesses that can significantly influence the user experience. For instance, ease of use is often highlighted as a crucial factor, making some tools more accessible to beginners, while others may cater to advanced users with specialized features.
💬 Questions about Popular Data Analysis Tools Pros And Cons?
Our team typically responds within minutes
On the flip side, the complexity of certain platforms can pose a steep learning curve for new users. Cost implications are another vital consideration, as some tools may offer robust functionality at a premium price, potentially deterring smaller organizations. In evaluating these aspects, users can make informed choices that align with their specific needs, enhancing their overall data analysis experience. Ultimately, understanding the pros and cons of each tool ensures that users identify the best fit for their objectives.
Strengths of Leading Data Tools
Leading data tools possess several strengths that make them essential for effective data analysis. First and foremost, ease of use and accessibility stand out. These tools often come with intuitive interfaces that facilitate quick learning, allowing users with varying skill levels to navigate efficiently. As a result, organizations can quickly harness insights from data without requiring extensive training.
Integration capabilities define another significant advantage. Various data tools seamlessly connect with other software applications, enabling users to consolidate data sources and streamline workflows. This is vital in today's data-driven environment, where timely access to combined information can lead to better decision-making. When conducting a data tool comparison, these strengths play a critical role in determining which tool aligns best with organizational needs and goals.
- Ease of Use and Accessibility
When evaluating popular data analysis tools, ease of use and accessibility play a critical role in their effectiveness. Data Tool Comparison emphasizes that a user-friendly interface is essential for both novice and experienced users. Many tools are designed without requiring specialized training. This democratization of insight allows anyone in a business to access and utilize data easily, making analysis more efficient.
Furthermore, the accessibility of these tools extends to various functionalities. For instance, some platforms automatically transcribe calls, extract key insights, and visualize data without complex navigation. This reduces friction for users, allowing them to focus on decision-making rather than grappling with challenging software. The ability to quickly analyze multiple datasets at once enhances overall productivity and encourages collaboration across teams, showcasing the importance of selecting tools that prioritize usability.
- Integration Capabilities
Integration capabilities are crucial in the evaluation of popular data analysis tools. They define how well a tool can connect and work cohesively with other systems, databases, and technologies. For organizations seeking efficient workflows, robust integration ensures that various data sources can communicate seamlessly, allowing for more comprehensive analysis and reporting.
When performing a data tool comparison, it’s essential to consider the following aspects of integration capabilities. First, assess how easily the tool integrates with pre-existing software and databases, as this can significantly reduce implementation time. Next, evaluate the nature of integration; some tools offer direct integrations, while others may require third-party solutions. Lastly, consider the scalability of these integrations, ensuring that they can adapt as your data needs evolve. Ultimately, a tool's ability to integrate fluidly with other platforms can enhance its overall value and effectiveness in data analysis.
Limitations to Consider
When engaging in a Data Tool Comparison, it’s essential to recognize the limitations tied to each tool's capabilities. The complexity of the learning curve can vary significantly. Many tools, while powerful, require extensive training and expertise. This can hinder swift adoption, particularly among teams that are not data-savvy.
Additionally, cost implications must be carefully considered. Many data analysis platforms come with substantial licensing fees and add-on costs, which can strain budgets. It is vital for organizations to evaluate their financial resources against the tool's benefits in terms of return on investment.
Ultimately, while tools serve as facilitators in data analysis, understanding their limitations is crucial. Prioritize these factors during your selection process to ensure alignment with your team's expertise and budget. This approach enhances your overall data strategy, leading to more informed decisions and better insights.
- Complexity in Learning Curve
When considering the complexity in the learning curve for various data analysis tools, it's essential to understand how this aspect impacts overall usability. Each tool presents its own challenges, which can vary greatly in terms of user-friendliness and required expertise. For instance, while tools like Tableau may offer intuitive interfaces, others, such as R or Python, necessitate a steeper learning curve.
Users must weigh the time and effort required to master these tools against the benefits they provide. A significant challenge with some software is the need for prior programming knowledge or statistical understanding, which can be a barrier for many. Moreover, comprehensive training resources are sometimes lacking, making self-learning difficult. Therefore, in any data tool comparison, both ease of entry and depth of capability become critical factors for decision-making.
- Cost Implications
When considering the Cost Implications of various data analysis tools, it’s crucial to evaluate not just initial expenses but also ongoing costs. A robust Data Tool Comparison should analyze software licenses, subscription fees, and hardware requirements. For instance, open-source tools like R or Python may come with lower costs but require significant time investment for setup and maintenance.
Additionally, businesses need to factor in the associated costs of training and support. While tools like Tableau and Power BI offer intuitive interfaces, their licensing models can become expensive as user numbers increase. Furthermore, consider the potential return on investment (ROI) and how a tool's capabilities can help drive efficiency and reduce operational costs over time. Balancing upfront costs with long-term benefits can lead to a more informed and strategic choice in the crowded data analysis market.
Conclusion: Choosing the Right Data Tool Based on Comparison
To make an informed decision regarding data tools, it's essential to conduct a thorough data tool comparison. Each tool presents unique strengths and weaknesses that cater to different analytical needs. Consider the specific objectives you aim to achieve with data analysis, whether through ease of use, integration capabilities, or statistical power.
As you evaluate your options, weigh the pros and cons carefully. The right choice can significantly enhance your analytical efficiency and insights. Thus, prioritizing your organization's requirements will help identify the most suitable tool, ensuring it aligns with both your current goals and future growth.
💬 Questions about Popular Data Analysis Tools Pros And Cons?
Our team typically responds within minutes