NextGen Predictive Analytics: From Data to HyperPersonalization
-
Bella Williams
- 10 min read
HyperPersonalized Insights mark a revolutionary shift in predictive analytics, sparking a new era where businesses can connect with their customers on an individual level. With the power of advanced data processing, companies can now harness detailed insights to predict and meet the specific desires of their users. This transition from generic outreach to tailored interactions is essential for staying competitive in today's market.
In this section, we will explore how HyperPersonalized Insights not only enhance customer experiences but also drive strategic decision-making. Understanding the dynamics of consumer behavior allows organizations to craft more relevant offerings, fostering loyalty and engagement. As we navigate through this transformative landscape, the potential of predictive analytics becomes increasingly evident, paving the way for service and innovation that responds intelligently to evolving customer needs.
Analyze & Evaluate Calls. At Scale.
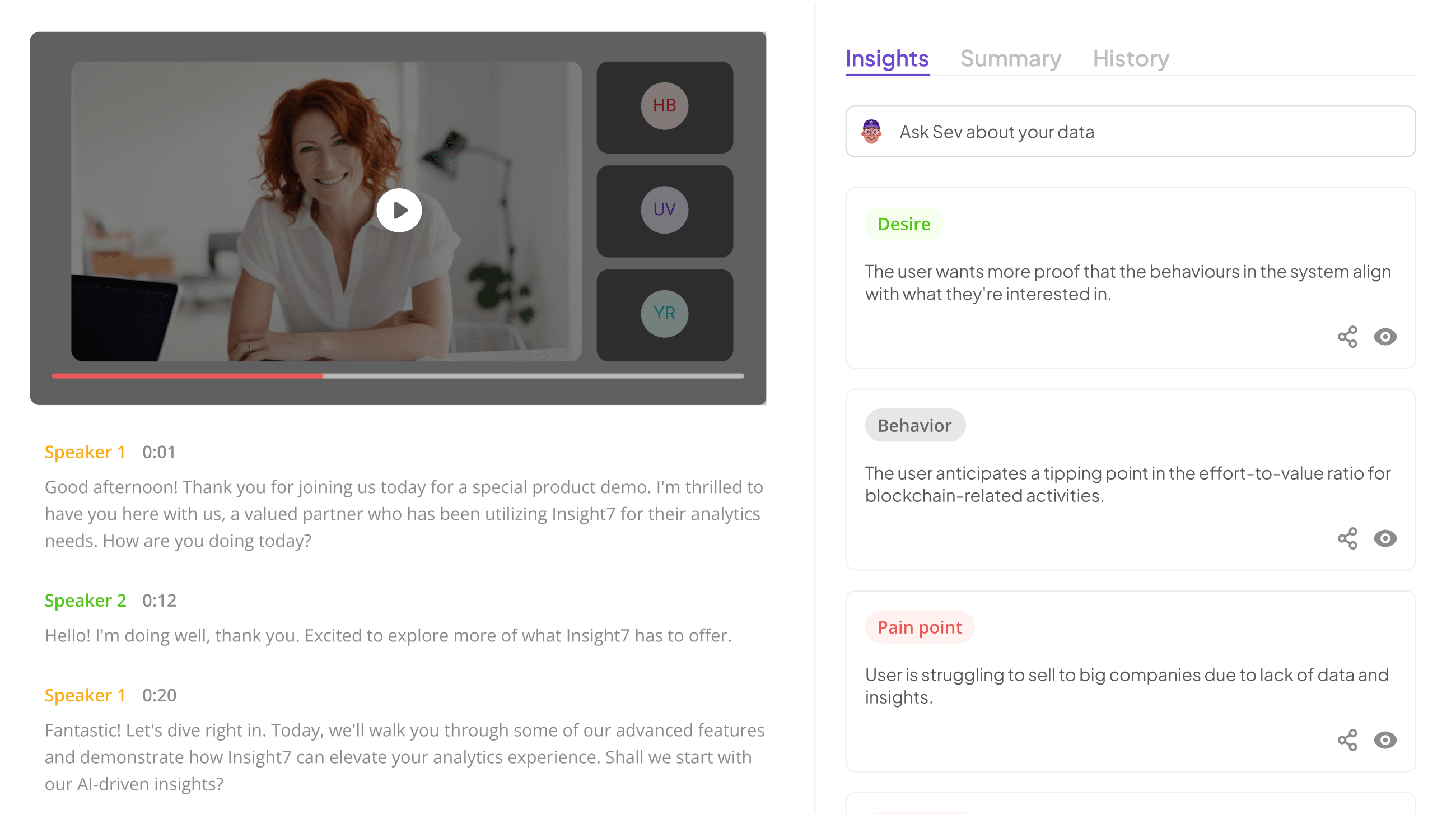
The Journey to HyperPersonalized Insights: From Data to Meaningful Interactions
The journey to HyperPersonalized Insights begins with recognizing the immense potential hidden within data. By harnessing this raw information, organizations can unlock insights that pave the way for deeper, more meaningful interactions with their audiences. Itโs essential to shift focus from merely collecting data to understanding the narratives it contains. This involves not just analyzing figures but interpreting emotions, preferences, and behaviors that drive customer decisions.
Moreover, cultivating HyperPersonalized Insights requires an iterative process. First, organizations need to gather relevant data across various touchpoints. Next, they must clean and integrate this data to ensure accuracy. Finally, employing advanced analytics techniques enables businesses to transform that data into actionable insights. By leveraging these insights wisely, companies can foster connections that resonate on a personal level, ultimately enhancing customer satisfaction and loyalty. Through this meticulous journey, brands not only meet customer expectations but anticipate them, fostering long-lasting relationships.
Collecting and Preparing Data for HyperPersonalized Insights
To achieve HyperPersonalized Insights, the initial step is collecting and preparing quality data. This process begins with identifying key data sources, such as customer interactions, transaction histories, and demographic information. These diverse datasets provide the foundation for gaining deep insights into user behavior and preferences. Collecting data from multiple sources helps build a richer understanding of customer needs.
Once the data is gathered, it must undergo rigorous cleaning and integration. This involves removing inaccuracies and ensuring consistency across datasets. Effective data integration allows seamless analysis, facilitating the discovery of meaningful patterns. By harmonizing data from various channels, businesses can generate actionable HyperPersonalized Insights that drive strategic decisions and enhance customer experiences. Remember, the quality of insights is determined by the quality of the underlying data, making this preparation phase essential in the journey toward effective predictive analytics.
- Key Data Sources in Predictive Analytics
Data-driven decision-making is essential in predictive analytics, particularly when aiming to achieve HyperPersonalized Insights. Understanding where to source this data is crucial for businesses seeking to enhance customer experiences. Key data sources can be broadly categorized into several types: transactional data, behavioral data, demographic data, and external data.
1. Transactional Data: This includes sales records and transaction histories, which offer insights into buying patterns and customer preferences.
2. Behavioral Data: Information such as website interactions, app usage, and social media engagement helps identify user behaviors, leading to tailored experiences.
3. Demographic Data: Data on age, gender, location, and income levels provides context for personalization efforts, ensuring messages resonate with target audiences.
4. External Data: Incorporating third-party insights, such as market trends and consumer reports, can augment internal data and provide a comprehensive view for predictive analytics.
Utilizing these diverse sources enables organizations to build robust predictive models that drive HyperPersonalized Insights, fostering deeper connections with customers. As analytics evolve, integrating various data streams allows for more accurate predictions and enhanced customer engagement.
- Data Cleaning and Integration
To achieve HyperPersonalized Insights, data cleaning and integration stands as an essential step in the predictive analytics journey. This phase involves meticulously preparing raw data for analysis, ensuring its accuracy and consistency. It helps to identify and rectify errors, handle missing values, and eliminate duplicates that could skew insights. Clean data sets lay the foundation for meaningful patterns that drive personalization strategies.
Once data is cleaned, the next step is integration, where disparate data sources are harmonized into a unified view. This consolidation facilitates a comprehensive understanding of user behavior. By integrating various datasets, organizations can unveil nuanced insights that contribute to the hyperpersonalization of interactions. Ultimately, effective data cleaning and integration are vital for transforming raw data into actionable insights, enhancing customer experiences, and fostering meaningful relationships.
Building Predictive Models for HyperPersonalization
Building predictive models for hyperpersonalization is a pivotal step in the journey toward delivering hyperpersonalized insights. To achieve this, organizations must utilize machine learning techniques that enable them to tailor experiences based on individual preferences and behaviors. By analyzing vast amounts of customer data, businesses can create models that predict future actions, thereby enhancing engagement and satisfaction.
Several key components play a significant role in this process. Firstly, selecting appropriate machine learning algorithms is crucial for accurate predictions. Next, fine-tuning these models through extensive training ensures they perform optimally. Furthermore, evaluating model performance is essential to validate the effectiveness of predictions and refine approaches over time.
In essence, building robust predictive models transforms raw data into actionable insights, revolutionizing how businesses interact with their customers. By embracing these advanced techniques, organizations can foster deeper connections with their audience, ultimately leading to sustained growth and competitive advantage.
- Machine Learning Techniques for Personalization
Machine Learning Techniques for Personalization play a crucial role in developing HyperPersonalized Insights. These techniques allow businesses to tailor experiences based on individual user behaviors and preferences. They analyze vast datasets, uncovering patterns that inform personalized recommendations, communications, and offers that resonate deeply with users.
To achieve effective personalization, businesses implement several machine learning techniques. First, collaborative filtering helps in recommending products based on similar user behaviors. Second, content-based filtering focuses on the characteristics of items in relation to user preferences. Third, clustering algorithms group users with similar traits, enabling targeted marketing strategies. Finally, natural language processing analyzes customer feedback to extract sentiments and trends, enhancing customer-centric decision-making. By applying these techniques systematically, organizations can transform raw data into actionable HyperPersonalized Insights that foster deeper customer relationships and drive engagement.
๐ฌ Questions about NextGen Predictive Analytics: From Data to HyperPersonalization?
Our team typically responds within minutes
- Evaluating Model Performance
Evaluating model performance is a critical step in the journey to achieving HyperPersonalized Insights. The effectiveness of predictive models hinges on their ability to generate actionable recommendations. To ensure that these models meet their intended goals, various metrics and evaluation techniques are employed. Understanding these evaluation criteria is essential for delivering impactful personalization.
When assessing model performance, consider the following keys: accuracy, precision, recall, and F1 score. Accuracy measures the overall correctness of the model's predictions, while precision and recall provide insights into how well the model identifies relevant data points. The F1 score harmonizes precision and recall, offering a comprehensive view of model performance. By analyzing these metrics, organizations can refine their models, ensuring they yield the HyperPersonalized Insights necessary for success. This ongoing evaluation process fosters continual improvement, empowering businesses to adapt to changing consumer behaviors effectively.
Extract insights from interviews, calls, surveys and reviews for insights in minutes
Tools for Creating HyperPersonalized Insights
Creating HyperPersonalized Insights relies heavily on innovative tools designed to analyze and interpret large datasets. These tools enable organizations to automate processes that previously required extensive time and expertise. For instance, platforms like IBM Watson Studio and Google Cloud AI Platform offer user-friendly interfaces for data visualization and modeling. They allow users to access insights without needing advanced technical skills.
Another important aspect is the ability to integrate various data sources seamlessly. Tools like Microsoft Azure Machine Learning and Amazon SageMaker facilitate the merging of data from different origins, crucial for generating comprehensive insights. By harnessing these technologies, organizations can delve deeper into customer preferences, behaviors, and sentiments, ultimately enhancing their offerings and engagement strategies. The result is the creation of tailored experiences that resonate with individual customersโmaking HyperPersonalized Insights not just achievable but essential for thriving in today's competitive landscape.
insight7: Leading the Charge
The advent of HyperPersonalized Insights marks a significant shift in how businesses connect with their customers. In this era, organizations must move beyond one-size-fits-all strategies and embrace a more nuanced approach to data analysis. Leading the charge involves adopting advanced predictive analytics tools that transform raw data into tailored customer experiences. This transformation not only enhances customer engagement but also drives customer loyalty, providing a critical competitive advantage.
To successfully harness HyperPersonalized Insights, organizations should focus on three core aspects: understanding customer signals, streamlining data analysis, and fostering collaboration. First, identifying and interpreting customer signals allows businesses to create more meaningful interactions. Next, using automated tools to analyze vast amounts of data efficiently will help in crafting responsive strategies. Lastly, ensuring that insights are shared across teams can lead to a unified approach in delivering personalized experiences. Embracing these principles is essential for any organization aiming to thrive in todayโs information-rich environment.
Other Essential Tools
In the quest for HyperPersonalized Insights, several essential tools can enhance predictive analytics capabilities. These tools not only streamline the analytics process but also empower teams to draw meaningful insights from vast datasets. Platforms like IBM Watson Studio and Google Cloud AI serve as key players, providing robust frameworks for machine learning and data management.
Using these advanced tools, organizations can efficiently analyze customer data and recognize patterns that inform strategic decisions. Microsoft Azure Machine Learning and Amazon SageMaker further bolster these efforts by offering scalable environments that support the creation, training, and deployment of predictive models. Each tool contributes uniquely to the analytics ecosystem, providing diverse functionalities tailored towards achieving HyperPersonalized Insights. The combination of these technologies fosters a culture of data-driven decision-making, ultimately leading to more customized and satisfying customer interactions.
- IBM Watson Studio
IBM Watson Studio empowers organizations to derive HyperPersonalized Insights from vast datasets, driving targeted engagement and enhancing overall customer experiences. This platform democratizes data access, enabling users of all skill levels to create predictive models without needing deep technical expertise. By facilitating intuitive data exploration and analysis, it streamlines the process from raw data to actionable insights.
The platform's innovative features include a centralized library for managing multiple datasets and advanced analytical engines that extract critical trends and themes. Users can analyze responses from various interactions, revealing customer pain points and desires. This ability to visualize and interpret data fosters informed decision-making. Ultimately, by utilizing tools like this, businesses can deliver tailored experiences that resonate deeply with individual preferences, ushering in a new era of HyperPersonalized Insights in predictive analytics.
- Google Cloud AI Platform
The Google Cloud AI Platform offers a robust infrastructure for businesses aiming to derive HyperPersonalized Insights from their data. By utilizing its advanced machine learning tools, organizations can seamlessly process vast amounts of information, transforming raw data into actionable intelligence. This platform allows users to create sophisticated predictive models tailored to individual customer behaviors, preferences, and needs.
Key features include automated machine learning capabilities that simplify the model-building process, as well as powerful analytics that facilitate real-time decision-making. Additionally, its ability to integrate with various cloud services ensures security and scalability, adapting to evolving business requirements. As businesses increasingly prioritize the customer experience, the Google Cloud AI Platform stands out as an essential tool for conducting deep analyses that inform personalized strategies and drive customer engagement.
- Microsoft Azure Machine Learning
Microsoft Azure Machine Learning serves as a powerful tool in the journey toward achieving HyperPersonalized Insights. By harnessing machine learning algorithms, organizations can create tailor-made predictive models that cater specifically to customer behaviors and preferences. This adaptability is crucial in today's data-driven landscape, where businesses must respond rapidly to changing market dynamics.
Azure provides an intuitive interface for building, training, and deploying machine learning models. Users can easily integrate various data sources, ensuring that insights generated are based on comprehensive and accurate datasets. Furthermore, the platform allows for advanced analytics, enabling organizations to examine patterns, detect anomalies, and uncover hidden insights. Through continuous learning and model optimization, companies can refine their predictive capabilities, leading to more meaningful interactions with customers. Ultimately, leveraging Azure's features fosters a deeper understanding of the target audience, paving the way for successful HyperPersonalized Insights.
- Amazon SageMaker
In the realm of hyperpersonalization, Amazon SageMaker plays a pivotal role by providing a comprehensive, fully-managed service for building, training, and deploying machine learning models. This platform allows organizations to unleash the power of artificial intelligence, resulting in hyperpersonalized insights that can transform customer interactions. By streamlining the development process, SageMaker makes machine learning accessible, facilitating real-time decision-making based on customer data.
With features such as automated model training and seamless integration with data sources, SageMaker empowers businesses to harness predictive analytics effectively. Users can easily visualize and analyze data, ensuring that the insights derived are actionable and relevant. The platform's user-friendly interface encourages collaboration across teams, enabling organizations to translate insights into meaningful strategies. By prioritizing hyperpersonalized insights, businesses can enhance their growth trajectory and ultimately foster stronger customer relationships.
Conclusion: Embracing HyperPersonalization with NextGen Predictive Analytics
HyperPersonalized Insights signify a transformative approach to customer engagement, driven by NextGen Predictive Analytics. To fully embrace this paradigm, organizations must understand that it is not merely about data collection but about meaningful interaction. By analyzing comprehensive datasets, businesses can unlock deep insights into customer preferences, enabling tailored experiences that resonate deeply with individual needs.
The integration of advanced predictive models fosters a new level of connection and relevance. As companies transition from standard analytics to HyperPersonalized Insights, they will revolutionize their engagement strategies. This evolution enhances customer satisfaction, fostering loyalty and driving success. Embracing these innovative analytics offers a path toward a future where every interaction is personalized and impactful.
๐ฌ Questions about NextGen Predictive Analytics: From Data to HyperPersonalization?
Our team typically responds within minutes