AI Text Insights are transforming how businesses analyze textual data, enabling them to glean meaningful information from vast amounts of unstructured content. In today’s fast-paced world, understanding customer sentiments and identifying trends from conversations or feedback is crucial for success. By utilizing AI Text Insights, companies can efficiently parse and interpret complex data, turning raw text into actionable intelligence.
This approach not only simplifies the data analysis process but also democratizes insights across teams, making them accessible to non-experts. From pinpointing customer pain points to highlighting emerging themes, the integration of AI in text analytics offers invaluable resources. Ultimately, AI Text Insights empower organizations to make informed decisions driven by clear, data-backed narratives.
Generate visualizations from your qualitative data. At Scale.
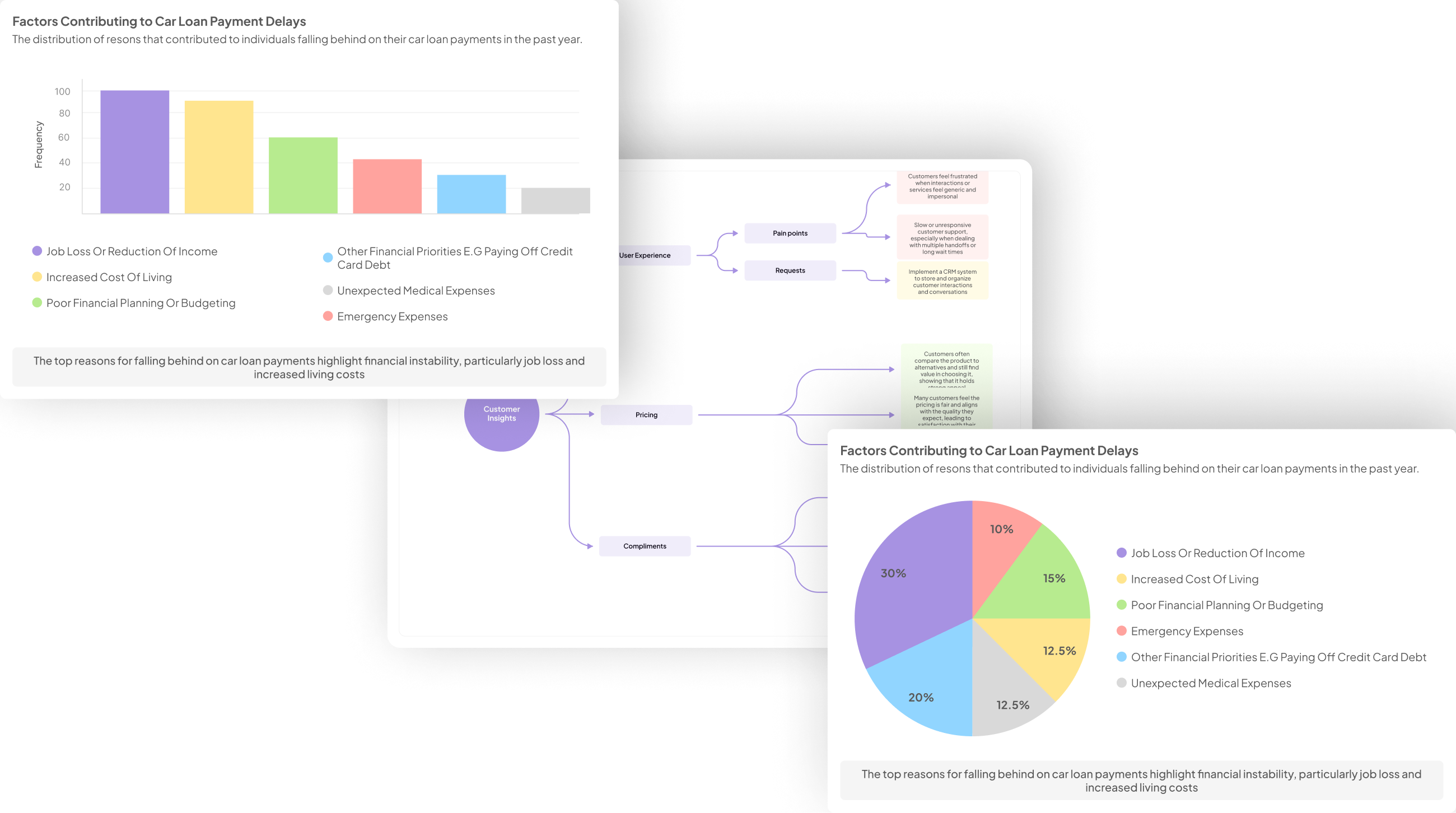
Understanding the Basics of AI Text Insights
Understanding the foundations of AI Text Insights is crucial for effective text analytics. AI Text Insights enable organizations to extract meaningful information from vast amounts of unstructured data, such as customer feedback or social media interactions. It’s essential to grasp how this technology transforms raw text into actionable insights that can inform business strategies and decisions.
At its core, AI Text Insights automates the process of recognizing patterns, sentiments, and themes within text. By employing natural language processing (NLP) algorithms, it identifies pain points, desires, and behaviors of customers, empowering businesses to respond effectively. This information is invaluable for improving user experience and refining product offerings. As a result, companies can drive customer satisfaction and enhance engagement by leveraging these insights to foster a more responsive and data-informed culture. Understanding these basics lays the groundwork for engaging with more complex applications of AI in text analysis.
What is Machine Learning in Text Analytics?
Machine learning in text analytics refers to the application of algorithms that can interpret and analyze textual data. This powerful technology enables systems to learn from data patterns, thereby providing valuable insights. By leveraging AI text insights, businesses can automate the process of understanding customer feedback, social media interactions, and other forms of unstructured text. This process helps organizations make informed decisions based on real-time data analysis.
To grasp the significance of machine learning in text analytics, it's essential to consider three key components:
- Natural Language Processing (NLP): This technology helps machines understand and interpret human language more effectively.
- Sentiment Analysis: This involves assessing emotions behind text, offering a nuanced view of customer attitudes.
- Topic Modeling: This allows for the identification of different themes within large datasets, streamlining content categorization.
Through these components, AI text insights pave the way for improved decision-making and more personalized customer experiences.
How AI Text Insights are Revolutionizing Data Analysis
AI Text Insights are reshaping the landscape of data analysis by uncovering valuable patterns within unstructured text data. By automating the extraction of key themes, sentiments, and customer feedback, businesses can turn raw text into actionable insights. This revolution enables companies to respond more effectively to customer needs and market trends, all while saving significant time and resources.
One of the most profound impacts of AI Text Insights is their ability to analyze vast datasets quickly. Traditional data analysis methods often struggle with the volume and complexity of text data. However, AI-powered tools can process hundreds of documents simultaneously, highlighting pain points and desires directly from customer conversations. This not only enhances operational efficiency but also democratizes access to valuable insights across teams, empowering even those without technical expertise to derive meaningful conclusions from their data.
Generate Journey maps, Mind maps, Bar charts and more from your data in Minutes
Implementing AI Text Insights for Practical Applications
AI Text Insights transform the way organizations understand and utilize data. By implementing these insights, businesses can streamline their decision-making processes and enhance customer experiences. The practical application of AI Text Insights begins with collecting relevant text data from various sources. This can include customer interviews, survey responses, or social media interactions.
The next essential step involves cleaning the data to remove any noise or irrelevant information, ensuring a solid foundation for analysis. After cleaning, selecting the right models for training becomes crucial. This phase helps identify patterns and trends within the text data effectively. Finally, analyzing the results can yield valuable insights that inform strategic decisions and improve operational efficiency. Real-world applications span numerous industries, from healthcare to finance, underscoring the versatile nature of AI Text Insights in driving meaningful outcomes.
Step-by-Step Process of Analyzing Text Data
Analyzing text data begins with systematic steps that ensure meaningful insights are drawn from large volumes of information. The first phase is data collection, where relevant textual information is gathered from various sources. This includes emails, social media posts, customer feedback, and other documents. Once data is collected, it may contain noise and errors, making the next step crucial: data cleaning. This involves removing irrelevant information, correcting typos, and standardizing formats to enhance the quality of the analysis.
The subsequent step is model selection and training, where the right algorithms are chosen based on the type of analysis required. Here, the AI models learn to recognize patterns and extract pertinent insights from the text. Finally, analyzing results is essential to derive actionable conclusions. This entire process culminates in AI Text Insights, which not only improves data interpretation but also facilitates better decision-making across various industries. Each step is integral to transforming raw text data into valuable insights for businesses.
- Step 1: Data Collection
Data collection is the cornerstone of any effective machine learning project, particularly in the realm of text analytics. Initially, it is paramount to define the types of data required, considering factors such as context, relevance, and purpose. For AI text insights, the data can come from diverse sources, including social media, customer feedback, and surveys. This extensive array of data contributes to a more nuanced understanding of user sentiment and behavior.
Once the sources are determined, the next challenge is ensuring data quality and comprehensiveness. It's crucial to gather a sufficiently large dataset to enable meaningful analysis and draw reliable conclusions. This may involve employing various web scraping techniques or utilizing APIs to collect structured data. The depth of your analysis hinges on the richness of your collected data, which will eventually lead to powerful AI text insights and informed decision-making.
- Step 2: Data Cleaning
Data cleaning is an essential step in the machine learning and text analytics process. This phase focuses on refining raw data, ensuring it is accurate, consistent, and usable for analysis. Without proper data cleaning, any insights derived from analysis can be misleading or completely invalid, making it crucial to invest time in this stage.
A few key activities play a central role in data cleaning. First, the identification of irrelevant or duplicate data points must be done to maintain dataset integrity. Next, it's important to address missing values by either removing them or imputing them with suitable alternatives. Furthermore, standardizing values for consistency allows for smoother comparisons during analysis. Finally, performing text normalization—such as converting to lower case or stemming—ensures that variations of words are treated uniformly. By conducting meticulous data cleaning, practitioners set the foundation for generating accurate AI text insights that can significantly impact decision-making processes.
- Step 3: Model Selection and Training
Model selection and training represent crucial stages in achieving effective AI text insights. This phase involves choosing the right algorithms and tools tailored to the nature of your text data and your specific analytical goals. Depending on the complexity and volume of data, options range from traditional machine learning techniques to advanced deep learning frameworks.
Initially, you must evaluate various models based on their performance against distinct metrics. It is essential to consider factors such as accuracy, precision, and recall. Once the models are selected, effective training of the chosen model is paramount. This process typically includes feeding the model with preprocessed data, allowing it to learn patterns and relationships inherent in the text.
Finally, iterative training and fine-tuning are necessary to enhance the accuracy of AI text insights. This continuous improvement cycle ensures that models not only adapt to new data but also remain effective over time, leading to actionable outcomes in your projects. By prioritizing these steps, organizations can significantly improve their capability to glean insights from textual data.
- Step 4: Analyzing Results
Analyzing results is a crucial part of extracting valuable insights from text data using AI tools. In this step, it's essential to evaluate the findings generated from your models critically. Begin by examining both the qualitative and quantitative aspects of the results, focusing on patterns and trends that emerge from the data. This analysis can reveal hidden correlations and help inform decisions. Furthermore, visualizing the data can enhance your understanding, allowing you to communicate insights more effectively to stakeholders.
To perform a thorough analysis, consider these key points:
- Review Data Outputs: Carefully analyze the AI model's outputs to identify relevant insights.
- Segment Insights: Break down results by categories to notice patterns within different data sets.
- Evaluate Performance: Assess the accuracy and efficiency of the machine learning model used for analysis.
- Compare Metrics: Look for similarities or discrepancies in insights across various data segments or timeframes.
- Iterate for Improvement: Use findings to refine your models or approaches for future analyses.
By following these steps, you'll gain comprehensive AI Text Insights, ensuring that your machine learning efforts deliver actionable and valuable outcomes.
Real-world Use Cases of AI Text Insights in Different Industries
AI Text Insights have shown remarkable potential across various industries, transforming how organizations analyze and utilize text data. In the healthcare sector, for instance, AI can process patient notes to identify common symptoms and treatment outcomes. This capability enhances clinical decision-making and improves patient care by offering timely insights.
In the retail industry, AI Text Insights can analyze customer feedback and reviews, extracting sentiments and preferences that inform product development and marketing strategies. These insights can reveal emerging trends, helping brands adjust their offerings to better meet consumer needs. Furthermore, sectors like finance and education utilize AI to digest vast amounts of unstructured data, identifying key themes and compliance risks along the way. Overall, the application of AI Text Insights across diverse fields underscores its importance in deriving actionable information and fostering innovation.
Conclusion: Harnessing AI Text Insights for Future Innovations
As we conclude our exploration into AI Text Insights, it becomes clear that these insights hold transformative potential for various industries. By effectively harnessing AI capabilities, organizations can uncover patterns in data that inform critical decisions, driving innovation and enhancing customer experiences. Integrating AI Text Insights into workflows not only boosts efficiency but also democratizes access to valuable information, allowing teams at all levels to leverage data for strategic objectives.
Looking forward, the continued evolution of machine learning tools will further empower businesses to tap into real-time insights. Future innovations will likely focus on refining user experience and integrating more sophisticated analysis techniques. Embracing AI Text Insights today sets the stage for a more agile and informed decision-making process, ensuring your organization stays ahead in a rapidly changing landscape.