AI-Driven Capacity Metrics have emerged as a transformative force in forecasting, reshaping how organizations approach capacity planning. In a world increasingly driven by data, these metrics provide a framework for integrating AI forecasting outputs with key performance indicators. This alignment not only enhances decision-making but also fosters greater accuracy in predicting future needs based on past performance.
Understanding AI-Driven Capacity Metrics is essential for organizations looking to optimize resources and respond to market demands effectively. By connecting the insights gained from AI with specific capacity planning metrics, businesses can mitigate risks and improve operational efficiency. The journey involves establishing clear relationships between forecasting data and capacity-related KPIs, ultimately enabling informed strategies that drive growth in a competitive landscape.
Analyze qualitative data. At Scale.
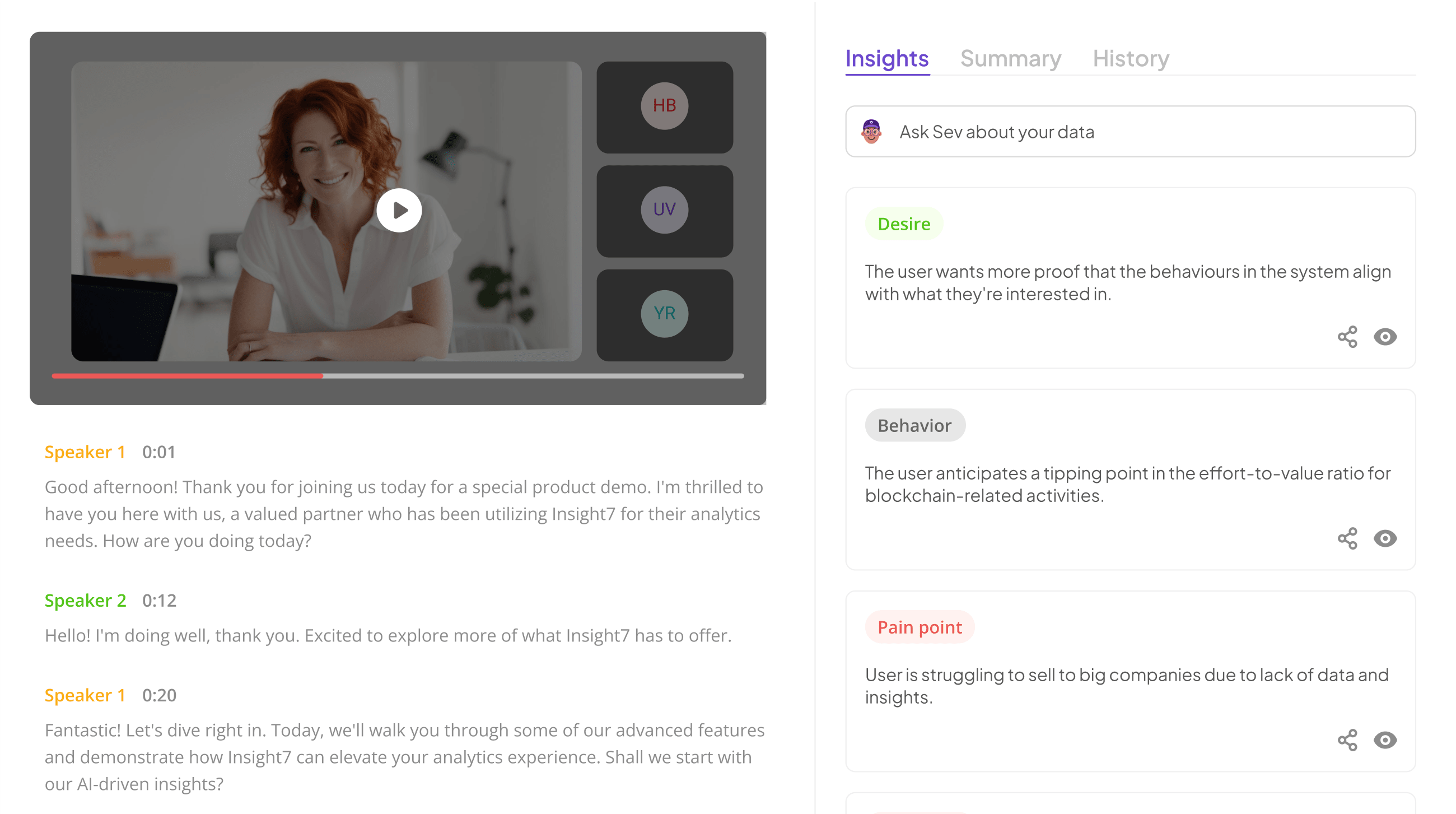
Understanding AI-Driven Capacity Metrics in Planning
AI-Driven Capacity Metrics play a crucial role in integrating forecasting outputs into capacity planning. These metrics allow organizations to make informed decisions by analyzing data patterns and trends. Understanding these metrics is essential for aligning resources effectively with anticipated demand, thereby optimizing operational efficiency. They provide insights that reflect not only current capacities but also forecast future needs, enabling proactive planning.
To effectively utilize AI-Driven Capacity Metrics, itโs important to focus on their key components. First, identifying critical performance indicators helps in measuring success. Next, continuous monitoring of AI outputs is vital to ensure metrics remain aligned with changing conditions. Lastly, feedback loops between data analysis and capacity adjustments enhance decision-making processes. These steps foster a data-driven culture, encouraging organizations to be agile and responsive to market dynamics, ultimately driving better outcomes in capacity planning.
The Role of AI Forecasting Outputs in Capacity Planning
AI forecasting outputs play a crucial role in capacity planning by providing data-driven insights that enhance decision-making processes. These outputs serve as vital indicators of future demand, enabling planners to align resources effectively. By integrating AI-driven capacity metrics, organizations can transform their forecasting accuracy, resulting in improved efficiency and lower operational costs.
Utilizing AI technologies, businesses can analyze historical data trends to predict future requirements. This analysis helps in identifying potential bottlenecks and optimizing resource allocation. Key benefits include the ability to quickly adapt to market changes, forecast customer demand accurately, and streamline operations. When AI forecasting outputs are linked with capacity planning, organizations can establish clear, actionable KPIs that drive performance and encourage continuous improvement. Ultimately, harnessing AI-driven capacity metrics empowers businesses to make informed decisions and maintain competitiveness in today's dynamic market.
Key Benefits of AI-Driven Capacity Metrics in Enhancing KPIs
AI-Driven Capacity Metrics significantly enhance Key Performance Indicators (KPIs) by providing precise, data-backed insights. This rigorous analysis enables organizations to optimize resource allocation effectively. By integrating AI technologies, businesses can anticipate demand fluctuations, leading to more informed decision-making processes.
The primary benefits of these metrics include increased efficiency and reduced costs. AI analyzes vast datasets to highlight potential bottlenecks, allowing managers to address issues proactively. Moreover, continuous monitoring of key indicators ensures responsiveness to changing market dynamics. As a result, companies can achieve optimal capacity utilization without overcommitting resources. Implementing AI-Driven Capacity Metrics not only advances operational effectiveness but also positions organizations to enhance overall performance by aligning strategies with actionable insights.
Extract insights from interviews, calls, surveys
and reviews for insights in minutes
Extract insights from interviews, calls, surveys and reviews for insights in minutes
Extract insights from interviews, calls, surveys and reviews for insights in minutes
Bridging AI Forecasting Outputs with AI-Driven Capacity Metrics Tools
Integrating AI forecasting outputs with AI-driven capacity metrics tools is essential for effective capacity planning. Understanding the precise data generated from forecasting can lead to actionable insights that directly influence your operational capabilities. By blending these technologies, organizations can streamline processes, resulting in more accurate predictions and improved resource allocation.
To successfully bridge these outputs, consider the following approaches:
Identify Key Metrics: Clearly define the KPIs that are critical to your capacity planning strategy. Exact metrics will guide your analysis and ensure relevance.
Utilize Advanced Tools: Implementing AI-driven capacity metrics tools allows for automation and enhances data accuracy. Tools such as IBM Watson and Azure Machine Learning can optimize your forecasting models.
Continuous Monitoring: Regularly evaluate the performance of your AI metrics. Adjusting based on real-time data insights ensures that your capacity planning aligns effectively with evolving demands.
This proactive approach facilitates a cohesive framework where forecasting outputs seamlessly interact with capacity metrics, driving efficiency and strategic decision-making.
Top Tools for Implementing AI-Driven Capacity Metrics
Implementing AI-Driven Capacity Metrics is crucial for enhancing your organizationโs capacity planning. Various tools offer innovative solutions to integrate these metrics into your existing systems. The right tools streamline data analysis, making it easier to generate actionable insights and improve decision-making.
Several prominent solutions can facilitate the implementation of AI-Driven Capacity Metrics. First, IBM Watson Forecasting provides robust predictive analytics that helps businesses forecast demand accurately. Second, SAP Integrated Business Planning allows for enhanced visibility across supply chain operations, ensuring that resources align effectively with demand. Third, the Oracle Autonomous Database automates data management processes, streamlining the preparation of capacity metrics. Fourth, Azure Machine Learning offers a comprehensive platform for building, training, and deploying machine learning models tailored to your capacity needs. Finally, insight7 stands out for its focused analytics on customer feedback, allowing companies to derive meaningful insights from user data. By evaluating these tools, organizations can successfully link their forecasting outputs to key capacity planning performance indicators.
- insight7
Integrating AI-Driven Capacity Metrics into capacity planning is essential for modern organizations striving for operational efficiency. These metrics facilitate real-time analysis of data, thus enhancing decision-making processes. By linking AI forecasting outputs to capacity planning, businesses can proactively address changes in demand and optimize resource allocation.
To effectively implement AI-Driven Capacity Metrics, focus on three key strategies. First, define the specific forecasting and capacity KPIs that align with your business objectives. Establishing clear metrics ensures a focused approach to capacity management. Next, utilize AI tools to automate the data analysis process. This step minimizes manual effort and allows teams to concentrate on interpreting insights rather than collecting data. Finally, continuously monitor and adjust these metrics to stay responsive to changing conditions. This dynamic approach not only leads to improved efficiency but also positions organizations to thrive in an evolving market.
- IBM Watson Forecasting
IBM Watson Forecasting provides robust capabilities that significantly enhance the accuracy and reliability of demand predictions. This AI-based system leverages historical data alongside current trends to inform decisions effectively. By integrating these forecasting tools, organizations can improve their AI-Driven Capacity Metrics, leading to better resource allocation and planning accuracy.
The forecasting outputs facilitate clearer visibility into future demands, making it easier to align capacity planning Key Performance Indicators (KPIs). Through iterative learning, the platform helps refine predictions continuously, allowing for adjustments in strategies over time. The insights gleaned from AI-driven forecasting empower organizations to respond proactively to market fluctuations, ultimately enhancing efficiency in capacity management.
Incorporating these forecasting techniques leads to a more strategic planning process that addresses both current and anticipated capacity needs, ensuring that resources are optimally utilized. This journey toward smarter capacity planning is fueled by the actionable insights generated by AI forecasting technologies.
- SAP Integrated Business Planning
SAP Integrated Business Planning is a comprehensive solution designed to streamline supply chain processes, enabling organizations to make informed decisions based on accurate data. This tool integrates various functions, including sales and operations planning, demand planning, and supply planning, creating a cohesive view that aids in optimizing resources. By utilizing AI-driven capabilities, businesses can enhance their accuracy in forecasting and better align their capacity planning efforts with market demands.
The integration of AI-driven capacity metrics within SAP Integrated Business Planning allows organizations to monitor performance indicators effectively. It provides real-time insights that help in identifying discrepancies between forecasted demand and actual capacity. Furthermore, automated data analysis from AI tools enhances the agility of the planning process, ensuring that businesses can pivot quickly to adapt to changing market conditions. This seamless connection between forecasting outputs and capacity planning ultimately leads to improved efficiency and responsiveness in meeting customer needs.
- Oracle Autonomous Database
Oracle Autonomous Database plays a pivotal role in harnessing AI-driven capacity metrics. This advanced cloud-based database service enables organizations to efficiently manage vast amounts of data. By automating routine tasks, it allows teams to focus on strategic decision-making rather than on database maintenance. The self-managing capabilities reduce the likelihood of human error and ensure optimal performance, enhancing data integrity.
Integrating AI-driven capacity metrics within the Oracle Autonomous Database empowers companies to access real-time insights seamlessly. These insights enable informed forecasting, helping organizations scale their resources effectively. By linking AI forecasting outputs to key performance indicators, teams can proactively address capacity planning needs. This dynamic approach helps streamline resource allocation and supports data-driven decisions, ultimately enhancing organizational efficiency and performance. In a rapidly evolving business environment, leveraging automated data analytics through Oracle Autonomous Database can significantly influence success in capacity planning.
- Azure Machine Learning
Azure Machine Learning serves as a pivotal tool in the realm of AI-driven capacity metrics. By enabling organizations to build, deploy, and manage machine learning models, Azure Machine Learning allows for more data-driven insights into capacity planning. It leverages advanced algorithms to interpret complex datasets, making it easier to forecast demand accurately and align it with organizational capabilities.
Incorporating Azure Machine Learning into capacity planning offers numerous benefits. It helps in identifying trends and potential bottlenecks in operations, ensuring that decision-makers are equipped with timely information for effective planning. Additionally, it enhances the alignment between AI forecasting outputs and capacity planning KPIs, driving operational efficiency and ensuring resources are adequately allocated. Ultimately, Azure Machine Learning innovates how businesses approach forecasting, empowering them to make informed decisions grounded in robust data analysis.
Steps to Align AI Forecasting Outputs with Capacity KPIs
To align AI forecasting outputs with capacity KPIs, organizations must first define the relevant metrics. Establishing clear forecasting and capacity KPIs allows teams to understand what success looks like. These standards guide the integration of AI-driven insights, ensuring that forecasting outputs effectively measure performance and resource allocation.
Next, implementing AI tools for automated data analysis is crucial. These tools sift through vast datasets, identifying patterns and trends that inform capacity planning. Regular monitoring and adjustments of the AI-driven capacity metrics are essential for optimal performance. By staying proactive in analyzing data and refining metrics, organizations can enhance their decision-making processes and overall capacity planning efforts. This systematic approach ensures that AI forecasting outputs remain aligned with the strategic objectives of capacity management, ultimately driving efficiency and growth.
Step 1: Define Relevant Forecasting and Capacity KPIs
To effectively link AI forecasting outputs to capacity planning, it is crucial to first define relevant forecasting and capacity KPIs. Knowing what to measure lays the groundwork for effective decision-making. Start by identifying key performance indicators that directly relate to your capacity objectives. These metrics can include forecast accuracy, capacity utilization, and lead times, all of which inform how well resources align with demand.
Next, consider the specific AI-driven capacity metrics you wish to implement. These metrics will help assess the performance of your forecasting models and their impact on operations. By clearly defining these indicators, you can establish a robust framework for measuring success over time. This clarity enables teams to make informed adjustments as needed, ensuring that forecasts remain versatile and responsive to changing market conditions. Ultimately, understanding and defining the appropriate KPIs is the first step towards a successful integration of AI into capacity planning.
Step 2: Implement AI Tools for Automated Data Analysis
To effectively implement AI tools for automated data analysis, it is essential to first understand the context in which these tools operate. Utilizing AI-Driven Capacity Metrics allows organizations to harness vast amounts of data to enhance their capacity planning processes. By automating data analysis, businesses can eliminate manual errors and significantly improve efficiency. This leads to more accurate forecasting outputs, crucial for meeting key performance indicators (KPIs) in capacity planning.
Begin by identifying the specific needs of your organization. Choose AI tools that align well with your current systems and have capabilities to generate meaningful insights. Regularly evaluate users' requirements, as their requests will shape the reporting process. Continuous refinement and adjustment of AI models and data inputs will further enhance the reliability of the AI-Driven Capacity Metrics, ultimately guiding decision-making and optimizing resource allocation.
Step 3: Monitor and Adjust AI-Driven Capacity Metrics
Monitoring and adjusting AI-Driven Capacity Metrics is essential for effective capacity planning. By continuously evaluating these metrics, organizations can quickly identify discrepancies between forecasts and actual performance. This step ensures that capacity aligns with demand, thereby minimizing underutilization or resource shortages. Regular reviews of the metrics enable better insights into evolving business needs and customer behaviors.
To successfully monitor and adjust, organizations should consider the following actions:
- Establish Baseline Metrics: Define key performance indicators (KPIs) that provide benchmarks for operational performance.
- Review Forecast Accuracy: Regularly assess how accurately the AI forecasts align with actual capacities. Adjust AI models to improve precision.
- Implement Feedback Loops: Encourage team feedback to understand practical challenges faced on the ground and refine the metrics accordingly.
- Adapt to Changing Variables: Stay alert to any changes in market trends or business objectives that may require a reassessment of capacity metrics.
By adopting these practices, organizations can enhance the reliability of AI-Driven Capacity Metrics and ensure more strategic capacity planning.
Conclusion: Enhancing Capacity Planning Through AI-Driven Metrics
AI-driven capacity metrics can significantly enhance capacity planning by providing precise, actionable insights. By integrating AI forecasting outputs with established capacity planning key performance indicators (KPIs), organizations can better anticipate demand fluctuations and optimize resource allocation. This synergy not only aids in identifying inefficiencies but also lays the groundwork for strategic decision-making.
Furthermore, the implementation of AI-driven metrics fosters a proactive approach to capacity planning. As organizations continuously refine their processes, the ability to adapt in real time becomes invaluable. Embracing these metrics ensures that data-driven decisions are guiding capacity planning efforts towards improved operational efficiency and customer satisfaction. Achieving this alignment can ultimately lead to a responsive, resilient capacity planning framework.