Churn Sentiment Analysis plays a critical role in understanding customer behavior, particularly regarding retention. Imagine a scenario where a customer quietly dwindles away, leaving behind unanswered questions about their experience. By tapping into sentiment analysis, businesses can discern the emotions tied to customer interactions, identifying those at risk of churning. This proactive approach not only aids in addressing issues but also illuminates the paths to improving customer satisfaction.
As companies strive to enhance their service and nurture loyalty, understanding the sentiment behind customer feedback becomes paramount. Churn Sentiment Analysis equips organizations with valuable insights that reflect customers' feelings, allowing for tailored interventions. In a competitive market, leveraging these insights is essential for fostering long-term relationships. By prioritizing the voices of the customers, businesses can shift from reactive measures to strategies that focus on nurturing customer connections and reducing churn effectively.
Analyze qualitative data. At Scale.
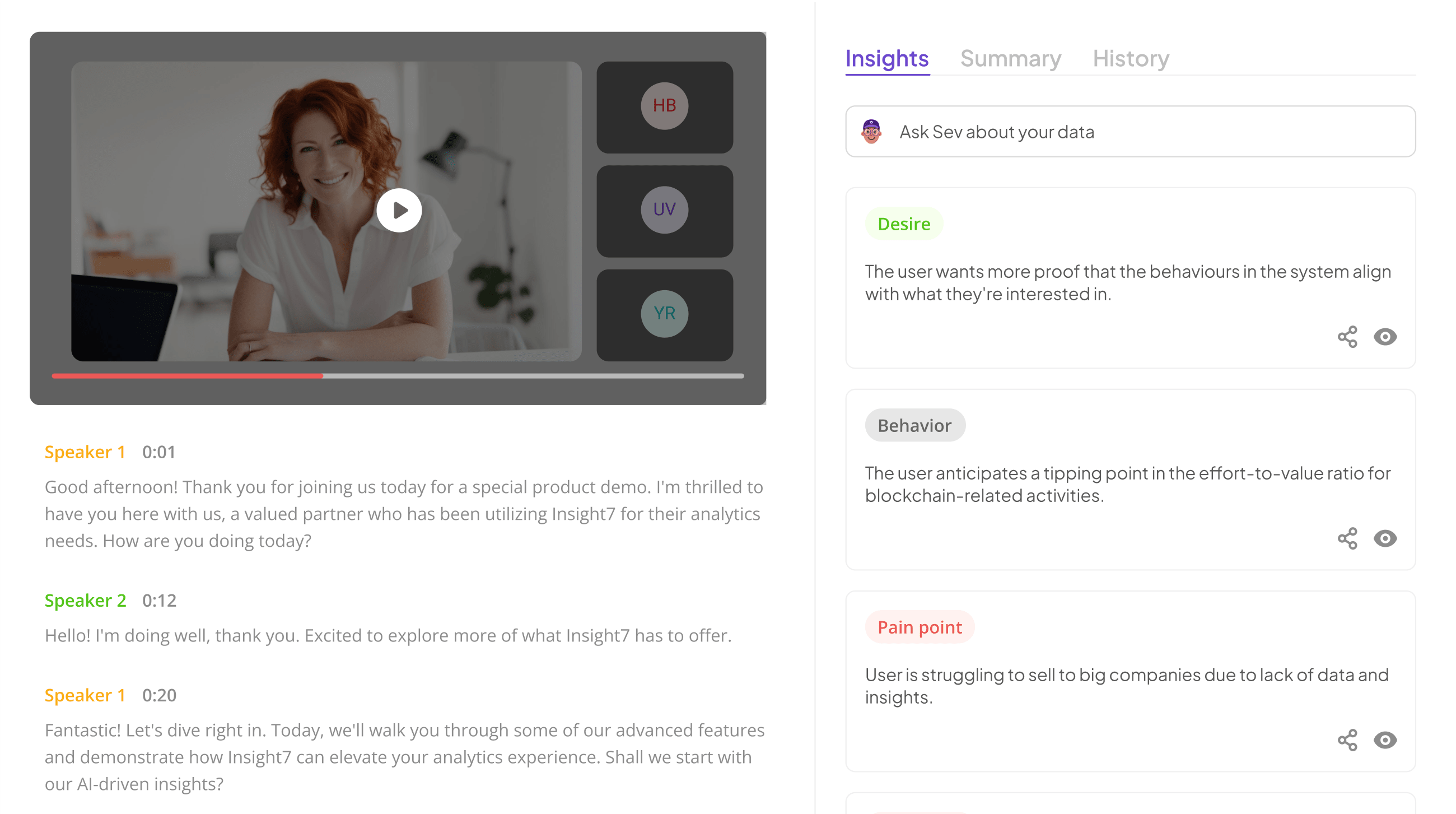
Understanding Churn Sentiment Analysis
Churn Sentiment Analysis is a crucial process for understanding the emotional landscape of customers who may be considering leaving a service or product. By analyzing customer feedback, businesses can identify patterns and sentiments that might indicate dissatisfaction or intent to churn. This analytical approach not only pinpoints specific areas needing improvement but also helps in establishing proactive strategies to enhance customer retention.
To effectively implement Churn Sentiment Analysis, businesses should focus on three key aspects. First, data gathering is vital; collecting customer interactions, feedback, and grievances forms the backbone of sentiment analysis. Next, sentiment modeling involves employing algorithms to interpret customer emotions from this data. Lastly, actionable insights derived from the analysis can inform retention strategies, directly addressing the root causes of churn. Integrating these insights into business practices ultimately fosters a more customer-centric approach, aiding in the retention of valuable clients.
What is Churn Sentiment Analysis?
Churn Sentiment Analysis is a crucial method for understanding customer behavior and predicting potential churn. This analytical approach focuses on mining sentiment data from various customer interactions, such as feedback, surveys, and social media mentions. By analyzing feelings and perceptions expressed by customers, businesses can gain insights into why customers might be considering leaving. This data-driven insight plays a significant role in formulating retention strategies.
Understanding Churn Sentiment Analysis enables companies to proactively address underlying issues leading to customer dissatisfaction. It allows businesses to identify patterns in sentiment that correlate with increased churn risk. Companies can then take targeted actions to enhance the customer experience and improve retention rates. By utilizing Churn Sentiment Analysis, businesses not only mitigate the risk of losing valuable customers but also foster long-term loyalty and engagement.
- Definition and importance in customer retention.
Understanding churn sentiment analysis begins with recognizing its definition and significance in customer retention. Churn sentiment analysis involves evaluating customer emotions and perceptions to gauge their likelihood of disengagement. By interpreting this sentiment data, businesses can proactively address issues, enhance customer experiences, and implement strategies that foster loyalty.
The importance of churn sentiment analysis cannot be overstated. First, it provides valuable insights that guide decision-making processes aimed at reducing customer churn. Second, it creates opportunities for personalized engagement, which strengthens customer relationships. Lastly, leveraging sentiment analysis helps organizations anticipate and respond to customer needs in real-time, ultimately retaining a loyal customer base. Engaging in multiple retention strategies, such as personalized communication and loyalty programs, maximizes the chances of keeping customers satisfied and committed.
- How it leverages sentiment data to predict churn.
Understanding customer sentiment is crucial for predicting churn effectively. Churn sentiment analysis focuses on evaluating emotional responses from customers to identify potential risks of disengagement. By carefully monitoring feedback, organizations can uncover underlying issues driving dissatisfaction, ultimately leading to improved customer retention.
To utilize sentiment data in predicting churn, businesses typically follow three primary steps. First, they gather qualitative and quantitative feedback from various sources, such as surveys and social media. Next, sentiment analysis techniques are employed to quantify emotions expressed in this feedback. Finally, insights derived from sentiment data are integrated into traditional churn prediction models, enhancing their accuracy. This integration allows companies to proactively address issues and refine strategies, ensuring a better customer experience and reducing turnover. By prioritizing emotional insights, organizations can foster stronger relationships and diminish churn rates effectively.
Why Businesses Need Churn Sentiment Analysis
Customer churn is a critical concern for businesses seeking to maintain a strong market position. Churn Sentiment Analysis helps companies understand the emotions and attitudes driving customer dissatisfaction. By analyzing customer sentiment, businesses can identify early warning signs of churn and respond proactively to mitigate risks.
One of the essential benefits of Churn Sentiment Analysis is its ability to improve customer retention. Active monitoring of customer sentiments provides insights into specific pain points, enabling businesses to enhance services or support. Moreover, industries such as telecommunications, retail, and software-as-a-service (SaaS) heavily benefit from these insights as they directly correlate customer perceptions with churn rates. Understanding these sentiments can lead to tailored strategies that resonate with customers, ultimately boosting loyalty and reducing churn. Through effective sentiment analysis, businesses not only react to issues but also create a more engaging and satisfying customer experience.
- The impact of customer churn on business performance.
Customer churn significantly influences business performance, often dictating the overall health of an organization. When customers leave, the immediate consequences are evident: lost revenue, decreased market share, and diminished brand reputation. Additionally, acquiring new customers typically costs more than retaining existing ones, making churn a critical metric for sustainability.
To address churn effectively, businesses must understand the drivers behind it. Churn Sentiment Analysis provides insight into customer emotions and opinions, allowing organizations to pinpoint where improvements are needed. For instance, a rise in negative sentiment could indicate frustration with customer service or product quality issues. By analyzing these sentiment trends, businesses can proactively address concerns, engage their customer base, and ultimately enhance retention strategies, fostering a cycle of growth and loyalty.
In summary, understanding the impact of customer churn through sentiment analysis is invaluable. It enables companies to take informed actions that not only mitigate losses but also strengthen customer relationships.
- Examples of industries benefiting from sentiment analysis.
Many industries are increasingly recognizing the importance of sentiment analysis, particularly its role in predicting customer churn risk. First, the telecommunications sector utilizes churn sentiment analysis to monitor customer feedback and gauge satisfaction levels. By analyzing sentiment from social media, reviews, and surveys, companies can identify issues before they lead to customer departures.
Next, the retail industry has also adapted sentiment analysis to enhance customer experiences. By observing sentiments expressed in customer interactions, retailers can adjust their offerings and improve service quality. Additionally, the banking sector leverages this analysis to understand client sentiment surrounding their services, providing insights into potential dissatisfaction, ultimately aiding in retention strategies. In summary, various industries harness churn sentiment analysis to better understand and respond to customer emotions, thus actively reducing the risk of churn and enhancing overall customer loyalty.
Extract insights from interviews, calls, surveys and reviews for insights in minutes
Implementing Churn Sentiment Analysis: A Step-by-Step Guide
Implementing Churn Sentiment Analysis begins with systematic data collection. First, identify relevant customer feedback sources, such as surveys, social media, and reviews. These channels provide essential insights into customer experiences and emotions. Itโs vital to use tools like Insight7, SurveyMonkey, or Google Forms for efficient data gathering. After collection, the data needs preprocessing to eliminate noise and ensure reliable sentiment analysis.
Next, employ various sentiment analysis techniques to gauge customer opinions. Techniques may include natural language processing and machine learning algorithms that analyze the sentiment behind customer comments. Interpreting sentiment scores is crucial; high negativity often indicates increased churn risk. Finally, integrate these insights with traditional predictive models to enhance accuracy. Continuous refining of this process enables businesses to respond quicker to customer sentiment, significantly improving retention strategies.
Step 1: Gathering and Preprocessing Data
To effectively begin your journey with Churn Sentiment Analysis, the first critical step involves gathering and preprocessing data. Quality data is the backbone of any successful sentiment analysis project. Start by collecting diverse datasets, which may include customer feedback, reviews, surveys, and social media posts. Each data source can provide unique insights into customer feelings and behaviors.
Once you have gathered your data, preprocessing is essential for accurate analysis. This includes cleaning up text data by removing any irrelevant information, correcting misspellings, and standardizing formats. Utilize tools like Insight7 for quantitative analysis to help streamline the process. By organizing your data effectively, you ensure that your sentiment analysis will yield actionable insights. Proper gathering and preprocessing allow you to build a strong foundation for predicting customer churn risk effectively.
- Types of data needed for sentiment analysis.
To effectively conduct Churn Sentiment Analysis, several types of data are essential. First and foremost, customer feedback is crucial. This includes reviews, comments, and ratings collected through surveys, social media, or direct customer interactions. Customers express their feelings and experiences through these channels, offering invaluable insights into their satisfaction levels.
Next, behavioral data plays a significant role in understanding customer sentiment. This involves tracking metrics such as purchase history, usage frequency, and engagement levels with products or services. By analyzing this data, businesses can identify patterns that may indicate dissatisfaction or potential churn. Finally, demographic data, such as age, location, and preferences, can provide context for sentiment analysis. It helps tailor strategies specifically to different customer segments, ultimately enhancing retention efforts and reducing churn risk. Together, these datasets provide a comprehensive picture necessary for effective churn prediction.
- Preprocessing techniques for effective analysis.
Understanding the preprocessing techniques for effective analysis is critical to enhancing churn sentiment analysis outcomes. Prior to conducting sentiment analysis, the collected data must undergo preprocessing to ensure accuracy and relevance. This stage involves cleaning the dataset by removing noise, filler words, and irrelevant information that might skew the analysis. Techniques like tokenization and stemming transform raw textual data into a format suitable for analysis, allowing for more effective sentiment classification.
Additionally, it's essential to consider the normalization of data. Standardizing formats and categories ensures consistency across different datasets. By implementing these preprocessing steps, businesses can extract valuable insights while minimizing the risk of misinterpretation. Overall, effective preprocessing lays the groundwork for accurate predictions of customer churn, enabling better decision-making and strategic interventions for customer retention.
Step 2: Analyzing Customer Sentiment
Analyzing customer sentiment plays a crucial role in understanding the likelihood of churn. Companies can effectively use tools to extract insights from various data sources. These sentiments can be captured through surveys, social media feedback, and customer interactions, allowing organizations to gauge emotional responses towards their products or services. By interpreting these sentiments, businesses can address underlying issues and enhance customer experience, thus reducing churn risk.
Through churn sentiment analysis, organizations can identify patterns in customer feedback. For instance, consistently negative sentiment scores might indicate dissatisfaction with a service or product. On the other hand, positive sentiment can reveal strengths that should be amplified. Understanding customer sentiment not only helps in mitigating risk but also informs strategic decisions for customer retention efforts. By focusing on behaviors and feedback, businesses can adapt their strategies to maintain a thriving customer base.
- Overview of sentiment analysis techniques.
Sentiment analysis techniques form the backbone of Churn Sentiment Analysis, enabling businesses to grasp customer emotions effectively. Various methods exist for analyzing sentiment, including rule-based approaches, machine learning, and deep learning techniques. Rule-based systems rely on predefined lists of terms, while machine learning leverages large datasets to identify sentiment patterns. Deep learning uses neural networks to enhance accuracy and understand complex emotional nuances.
Each of these techniques offers unique advantages. Rule-based methods are transparent and easily interpretable, suitable for straightforward tasks. In comparison, machine learning and deep learning techniques can handle a greater variety of data, capturing more subtle sentiments. Businesses benefit immensely from these analyses as they can predict customer churn and take proactive measures. By understanding customer sentiment better, companies can implement targeted strategies that address concerns, improve satisfaction, and ultimately reduce churn risk.
- How to interpret sentiment scores related to churn.
Interpreting sentiment scores related to churn involves understanding emotional responses and their implications on customer retention. Sentiment analysis translates customer feedback into quantifiable scores, reflecting their perceptions. A positive score usually indicates satisfaction, while a negative score suggests a likelihood of disengagement. When examining these scores, itโs crucial to consider the context behind them. For instance, a sudden drop in sentiment may highlight unresolved issues, prompting preemptive action.
Additionally, recognizing patterns over time can reveal the overall sentiment trend. Repeated negative scores might signal deeper problems in service or product offerings that need addressing. It's also important to correlate these scores with churn rates to gauge real impact. Engaging with customers who express dissatisfaction can provide critical insights into their experience. Such proactive outreach not only helps mitigate churn but also fosters a stronger relationship, ultimately enhancing retention strategies. By continually analyzing sentiment scores, businesses can make informed decisions that contribute to customer loyalty.
Step 3: Integrating Sentiment Analysis with Churn Prediction Models
Integrating sentiment analysis with churn prediction models involves merging customer feedback with data-driven insights. By utilizing sentiment scores derived from customer interactions, businesses can enhance their understanding of customer behavior. This integration allows organizations to identify at-risk customers proactively and tailor their retention strategies accordingly.
To effectively combine these two approaches, businesses should consider several key steps. First, adopt sentiment analysis tools that extract valuable insights from customer feedback. Following this, integrate these insights with existing churn prediction models, which often rely on historical data and statistical methods. This combination facilitates real-time analysis, enabling decision-makers to act quickly. As a result, companies can develop targeted interventions based on both quantitative data and qualitative sentiments, ultimately reducing churn rates. Adopting a holistic approach to Churn Sentiment Analysis significantly improves the potential for retaining valuable customers.
- Combining sentiment data with traditional churn prediction models.
Integrating sentiment data with traditional churn prediction models can significantly enhance the understanding of customer behavior. By merging qualitative insights from sentiment analysis with quantitative data, businesses can predict churn with greater accuracy. Traditional models often overlook emotional signals, which can provide crucial context about customer satisfaction or dissatisfaction. These emotional indicators can highlight potential reasons for churn that numerical data might miss.
To effectively combine these approaches, consider these key steps:
Data Integration: Merge sentiment scores with existing churn data collected from customer interactions.
Feature Engineering: Develop features that capture the emotional sentiment in relation to churn triggers, such as frustration or confusion with pricing.
Model Training: Utilize machine learning algorithms that can analyze both numerical and sentiment data to improve prediction outcomes.
By implementing Churn Sentiment Analysis, businesses can achieve a holistic view of their customers, helping to identify at-risk users before they leave. This proactive approach can foster retention strategies aimed at addressing specific pain points, ultimately reducing churn rates.
- Real-time analysis and automated decision-making.
Real-time analysis plays a critical role in predicting customer churn through sentiment analysis. By continuously monitoring customer sentiment, businesses can quickly identify potential red flags that indicate disengagement. Automated decision-making algorithms can then act on these insights promptly, triggering immediate interventions to retain at-risk customers.
To harness this capability, organizations should integrate sentiment data with predictive churn models. This process typically involves several key steps. First, data collection tools like surveys and feedback forms must be utilized to capture customer sentiment effectively. Next, advanced sentiment analysis techniques can process this data in real time, providing ongoing updates about customer attitudes. Finally, decision-making systems can evaluate sentiment scores and automatically suggest personalized retention strategies, ensuring timely responses. This proactive approach to churn sentiment analysis not only enhances customer satisfaction but also strengthens retention efforts.
Tools for Effective Churn Sentiment Analysis
To effectively conduct churn sentiment analysis, leveraging the right tools is crucial for success. Various platforms enable businesses to gather, analyze, and interpret customer feedback, allowing for proactive measures against potential churn. One standout tool is Insight7, which excels at quantitative analysis and offers valuable insights from customer data. Its features are designed to efficiently process feedback and highlight significant sentiment trends.
In addition to Insight7, other tools can enhance churn sentiment analysis. IBM Watson Tone Analyzer provides extensive emotion analytics, while RapidMiner offers robust data mining capabilities. For those looking for user-friendly interfaces, MonkeyLearn and Lexalytics can easily handle feedback categorization and sentiment scoring. Selecting the right combination will help businesses gain deeper insights into customer sentiments, ultimately assisting in predicting and reducing churn rates. With the right tools in place, organizations can transform sentiment data into actionable strategies, leading to enhanced customer satisfaction and retention.
Insight7
Gathering and leveraging customer feedback is essential for improving business strategies and reducing churn risk. Effective sentiment analysis begins with obtaining valuable insights from your customers. Consider using tools like Insight7, alongside others like SurveyMonkey or Typeform, to collect feedback and understand customer sentiments. These tools can transform complex data into actionable insights, enabling businesses to adapt to customer needs more effectively.
Once customer sentiments are analyzed, patterns may emerge, highlighting areas requiring attention. This could include product enhancements or customer service improvements. Monitoring sentiment over time will also help you identify potential churn, giving you the chance to take proactive steps. Regularly assessing customer feedback and sentiment allows businesses to stay aligned with their customers, significantly improving retention efforts and reducing churn risk. By understanding and acting on customer sentiment, companies can build trust and long-lasting relationships with their clientele.
- Overview of Insight7 as a leading tool for sentiment analysis.
As businesses increasingly recognize the value of understanding customer sentiment, Insight7 stands out as an essential tool for effective analysis. This platform specializes in evaluating sentiment data, which is crucial for predicting factors like customer churn. Its advanced algorithms enable businesses to derive actionable insights from varied feedback, allowing them to anticipate customer issues before they escalate.
Key features of Insight7 include its intuitive user interface and robust data processing capabilities. The tool effectively aggregates customer feedback from multiple channels, which ensures a comprehensive understanding of customer sentiments. Additionally, Insight7โs ability to seamlessly integrate with existing customer relationship management systems enhances its value. By utilizing this platform, businesses can proactively address concerns, thereby minimizing churn risk and fostering customer loyalty.
Incorporating Churn Sentiment Analysis through Insight7 enables companies to make informed strategy decisions while enhancing their overall customer experience.
- Key features that help predict customer churn.
Key features that help predict customer churn involve understanding the nuances of customer sentiment and behavior. Churn Sentiment Analysis plays a critical role in identifying at-risk customers before they decide to leave. A few essential features to focus on include customer feedback patterns, engagement scores, and historical purchase behavior. By analyzing negative feedback and declining engagement over time, businesses can pinpoint potential churn risks and take proactive measures to retain their customers.
Additionally, demographic information can significantly enhance predictive accuracy. Recognizing trends across different customer segments may reveal specific vulnerabilities. Tools that facilitate this analysis, such as Insight7, can help distill large datasets into actionable insights. By integrating sentiment data with traditional churn models, businesses can create a more holistic view of their customer landscape. This integrated approach not only allows for targeted interventions but also fosters a culture of customer-centric growth. Understanding these features is crucial for devising effective strategies to combat churn.
Additional Tools for Churn Prediction
In the quest for understanding customer churn, additional tools can greatly enhance the precision of Churn Sentiment Analysis. While sentiment analysis is vital, integrating other tools can provide deeper insights into customer behavior. One valuable resource is the IBM Watson Tone Analyzer, which assesses emotional tones in customer interactions. Similarly, platforms like RapidMiner and MonkeyLearn offer robust capabilities for data analysis, allowing businesses to visualize churn trends effectively.
Additionally, Lexalytics provides advanced text analytics capabilities, helping to decode customer feedback and correlate it with churn risk. By employing these tools in conjunction with sentiment analysis, businesses can not only identify potential churn risk but also understand the underlying factors contributing to it. Leveraging these technologies leads to more informed decision-making and ultimately improves customer retention strategies.
- IBM Watson Tone Analyzer
The IBM Watson Tone Analyzer is a powerful tool for understanding customer sentiment, providing businesses with deeper insights into their clientele's emotional responses. This tool can analyze text and determine the tone behind customer feedback, which is essential for churn sentiment analysis. With its ability to identify emotions such as joy, anger, and frustration, businesses can tailor their responses and improve customer experiences.
Utilizing the IBM Watson Tone Analyzer enhances the effectiveness of customer feedback collection. By interpreting the emotional context of responses, companies can pinpoint potential churn risks and develop strategies to mitigate them. Engaging with customers who express negative sentiments can encourage loyalty and improve retention rates. Overall, the integration of this technology into sentiment analysis allows for better prediction of churn, driving targeted intervention efforts and fostering long-term relationships with customers.
- RapidMiner
RapidMiner is a powerful tool that facilitates the application of Churn Sentiment Analysis. It combines advanced data processing capabilities with machine learning, making it an excellent choice for businesses aiming to predict customer churn risk through sentiment data. Users can easily import customer feedback data, including reviews and survey results, enabling them to extract valuable insights into customer emotions and behaviors.
To effectively utilize RapidMiner for Churn Sentiment Analysis, follow these pivotal steps. First, prepare your dataset by cleaning and organizing the data to ensure accurate sentiment extraction. Next, employ RapidMinerโs suite of sentiment analysis operators to assess customer sentiments, categorizing them as positive, negative, or neutral. Finally, integrate these sentiment scores with your existing churn prediction models to forecast potential churners accurately. By strategically harnessing RapidMiner, businesses can not only identify at-risk customers but also develop targeted retention strategies to foster loyalty and reduce churn.
- MonkeyLearn
MonkeyLearn stands out as a powerful tool for conducting Churn Sentiment Analysis, helping businesses understand customer sentiments better. It utilizes machine learning to analyze textual data from various sources such as customer feedback, reviews, and social media. By doing so, it identifies patterns in customer emotions and opinions, enabling companies to predict potential churn risks effectively.
To maximize the benefits of Churn Sentiment Analysis using this tool, businesses can follow these steps:
- Data Integration: Import data from diverse sources to ensure comprehensive sentiment analysis.
- Sentiment Classification: Use the platform's pre-built models to classify customer sentiments as positive, negative, or neutral.
- Insights Generation: Extract actionable insights from the analyzed data to inform customer retention strategies.
By incorporating these features into their strategies, organizations can proactively address customer concerns, ultimately reducing churn rates and enhancing customer satisfaction.
- Lexalytics
In the realm of Churn Sentiment Analysis, several tools can significantly enhance the accuracy of customer insights, with one notable tool standing out for its effective capabilities. This tool offers advanced features that help businesses analyze customer feedback and sentiments, ultimately aiding in churn prediction efforts. By processing vast amounts of unstructured text data, this tool can unveil hidden patterns and sentiments that might indicate customer dissatisfaction or potential churn.
Using this tool, businesses can evaluate customer interactions across channels, allowing them to understand how sentiment fluctuates over time. Through these insights, companies can proactively address concerns before they escalate. Implementing Churn Sentiment Analysis effectively requires integrating such tools into existing systems, ensuring that the analysis translates into actionable strategies. Ultimately, leveraging these advanced tools empowers organizations to make data-driven decisions that enhance customer retention and loyalty.
Conclusion: Leveraging Churn Sentiment Analysis for Business Success
Understanding the impact of customer sentiments on churn is crucial for business success. By thoroughly analyzing churn sentiment analysis, organizations can proactively identify the underlying reasons for customer dissatisfaction. This approach enables businesses to implement strategic improvements, which can significantly enhance retention rates and overall performance.
Incorporating insights from customer feedback allows companies to prioritize actionable changes. By focusing on user experience aspects, like onboarding and support documentation, businesses can cultivate a loyal customer base. Ultimately, using churn sentiment analysis not only helps mitigate churn risks but also drives sustained growth through strengthened customer relationships.