In today’s fast-paced world, the pressure for actionable insights has intensified. Businesses are challenged to adapt their concepts swiftly to meet consumer needs while ensuring their strategies are grounded in reliable data. AI-driven concept testing offers an innovative solution, enabling companies to harness qualitative data analysis for deeper understanding.
AI-driven concept testing allows organizations to efficiently analyze consumer feedback through advanced algorithms. By transforming raw qualitative data into meaningful insights, businesses can identify patterns and themes essential for effective decision-making. This approach not only saves time but also reduces bias, empowering teams to create concepts that truly resonate with their audience.
Analyze & Evaluate Calls. At Scale.
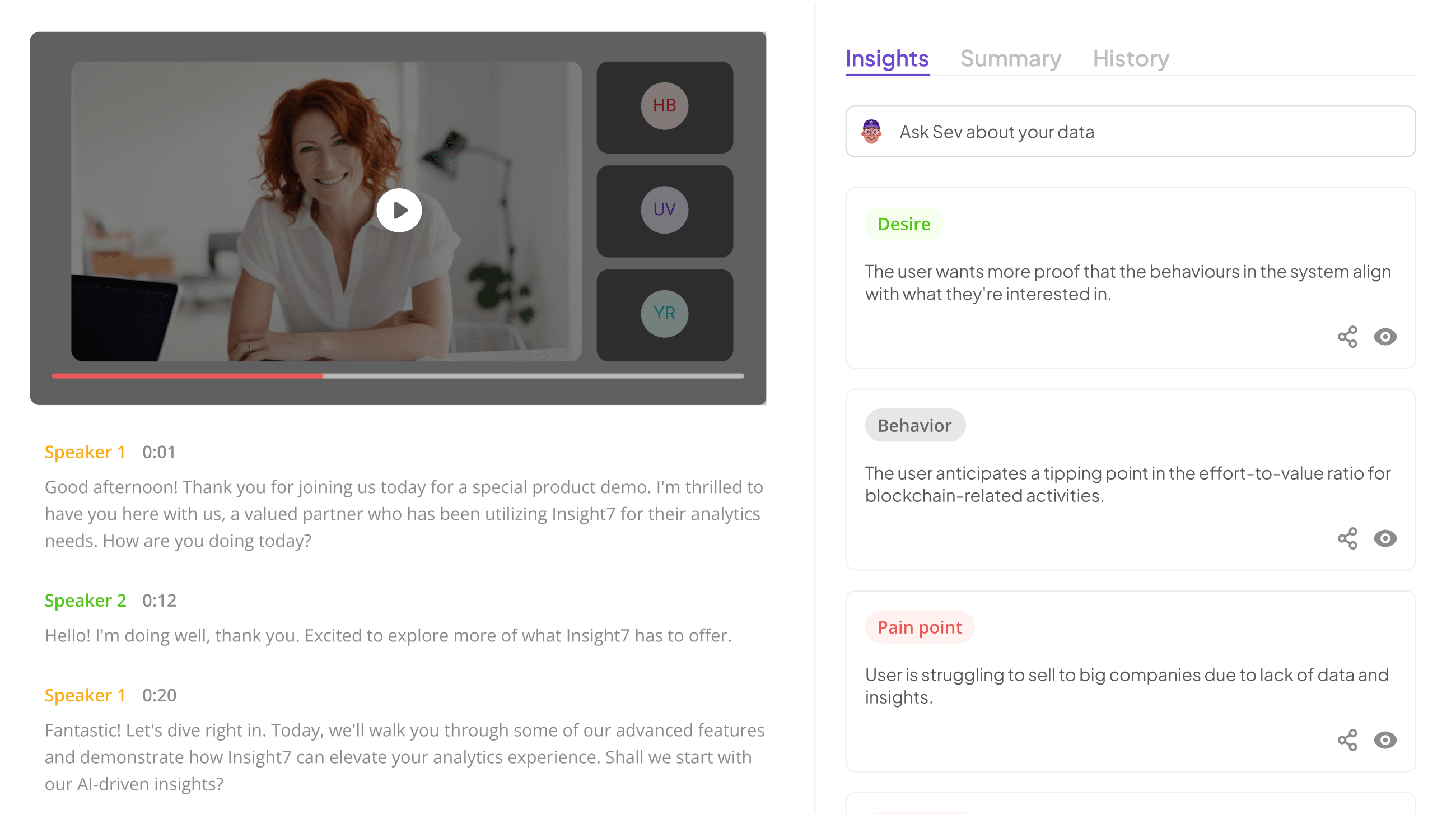
Understanding AI-Driven Concept Testing
AI-Driven Concept Testing enables organizations to streamline their understanding of potential product or service ideas through advanced qualitative data analysis. This approach eliminates traditional biases and inconsistencies often found in manual analyses. By employing AI tools, teams can efficiently analyze interviews and open-ended survey responses to uncover meaningful insights, facilitating quicker decision-making processes.
To harness the full potential of AI-Driven Concept Testing, teams must first gather qualitative data from diverse sources. Next, preprocessing the data using AI technologies allows for a more effective analysis, revealing patterns and themes that may not be immediately obvious. Finally, interpreting these AI-driven insights equips teams with actionable recommendations, improving the overall concept testing process and ensuring that valuable voices are heard. Emphasizing AI's role promotes a more objective, streamlined approach to understanding market needs and consumer preferences.
The Role of Qualitative Data Analysis AI
Qualitative Data Analysis AI plays a pivotal role in enhancing AI-Driven Concept Testing by providing deeper insights through efficient data processing. Traditional methods of analyzing qualitative data, such as interviews and open-ended survey responses, can be slow and inconsistent. AI tools can streamline these processes, allowing researchers to quickly identify themes and patterns from vast amounts of data without the biases that human analysis might introduce.
Moreover, the integration of AI in qualitative analysis helps teams save time and resources. With AI-Driven Concept Testing, the interpretation of emotional and nuanced feedback becomes more manageable, leading to swift decision-making. This approach enables businesses to adapt their strategies based on real-time insights from their audience. Consequently, AI serves not only as a tool for analysis but as a catalyst for more agile and informed concept development. Embracing these innovations ensures that organizations remain competitive in a fast-paced market, optimizing their approaches to meet audience expectations effectively.
Benefits of Integrating AI in Concept Testing
Integrating AI in concept testing significantly enhances the efficiency and accuracy of the analysis process. One major benefit is the ability of AI to quickly process large volumes of qualitative data, which traditionally takes considerable time and effort. This capability accelerates the feedback loop, allowing businesses to make decisions faster and adapt their concepts based on real-time insights.
Furthermore, AI-driven concept testing minimizes biases often present in human analysis. By utilizing advanced algorithms, the technology uncovers patterns and themes grounded in data rather than subjective interpretations. This leads to more reliable insights and informed decision-making. Overall, embracing AI in concept testing not only optimizes workflows but also improves the quality of insights drawn from customer feedback, ensuring better alignment with market needs. These advantages ultimately contribute to more successful product launches and enhanced customer experiences.
Implementing AI-Driven Concept Testing
Implementing AI-Driven Concept Testing requires a systematic approach to fully harness the potential of artificial intelligence in analyzing qualitative data. First, gather qualitative data through interviews, surveys, or focus groups. This step lays the foundation for effective analysis. Once collected, pre-processing the data is essential to ensure clarity and remove any irrelevant information.
Next, utilize advanced AI tools to analyze the data. These tools can identify patterns, extract themes, and generate key insights quickly and efficiently. Interpreting these AI-driven insights is the final step. It involves translating raw data into actionable recommendations that can guide product development or marketing strategies. Ultimately, effective implementation of AI-Driven Concept Testing allows businesses to make informed decisions based on solid qualitative evidence, spurring innovation and enhancing customer satisfaction. By combining human expertise with AI technology, organizations can ensure that their concept testing remains relevant and impactful.
Extract insights from interviews, calls, surveys and reviews for insights in minutes
Step-by-Step Guide to Harnessing AI for Concept Testing
To effectively harness AI for concept testing, it’s essential to follow a logical framework that boosts your chances of success. Begin by gathering qualitative data from diverse sources such as focus groups, interviews, and surveys. This initial step sets the foundation for meaningful insights by ensuring you capture varied perspectives on your concept.
Once the data is collected, utilize AI tools to preprocess and analyze it. These tools can identify themes and patterns that may not be immediately apparent, delivering a snapshot of customer sentiment and preferences. Finally, interpreting the insights garnered from AI analysis is crucial. This involves translating the findings into actionable strategies for your concept, enabling you to align your offering with consumer expectations. This structured approach not only enhances the effectiveness of AI-driven concept testing but also ensures that decisions are informed by robust qualitative data analysis.
- Step 1: Gathering Qualitative Data
To embark on the journey of AI-Driven Concept Testing, the first essential step is gathering qualitative data. This process involves collecting rich, descriptive information that offers insights into consumer perceptions and experiences. Methods such as interviews, open-ended surveys, and focus groups are vital in obtaining this nuanced data. By engaging participants in meaningful conversations, researchers can capture diverse viewpoints that quantitative data might overlook.
After selecting appropriate methods, ensure the questions are open-ended to encourage detailed responses. Aim to create an environment where participants feel comfortable sharing their thoughts. Collecting this data will allow AI tools to analyze and identify patterns, sentiments, and themes, ultimately enhancing the concept development process. This qualitative foundation is critical for effective AI-driven analysis, transforming raw information into actionable insights that guide decision-making and innovation.
- Step 2: Preprocessing and Analyzing Data with AI Tools
In this phase of AI-driven concept testing, preprocessing and analyzing data is crucial. Begin by organizing data collected from interviews and surveys, ensuring it is in a format suitable for analysis. AI tools can streamline this process by automatically identifying themes and patterns, transforming raw qualitative data into actionable insights. This automation significantly reduces the time spent on manual analysis, allowing teams to focus on interpreting results rather than sifting through data.
Next, employ AI-driven analytical tools to conduct a comprehensive analysis. These tools refine qualitative responses and categorize them according to predefined criteria. This structured approach not only mitigates bias but also enhances consistency in insights across your team. Ultimately, effective preprocessing and analysis of data lead to more reliable outcomes and quicker reporting, addressing the common challenges faced during concept testing. Through these methods, stakeholders can make informed decisions based on a clear understanding of the data, enhancing the overall effectiveness of concept testing efforts.
- Step 3: Interpreting AI-Driven Insights
Interpreting AI-driven insights is a critical step in the concept testing process, allowing for a deeper understanding of qualitative data. After the data has been gathered and preprocessed, the next focus is translating AI outputs into actionable insights. This translation requires careful attention to the themes and patterns identified by AI, ensuring that the insights align with your concept objectives.
To navigate this effectively, follow these key points:
Evaluate the Context: Understand the specific context in which data was collected, including demographic factors and user feedback nuances.
💬 Ask About This Article
Have questions? Get instant answers about this article.
Powered by Insight7Analyze Themes: Review the identified themes to discern which insights are most relevant for your concept testing goals. Prioritize insights that contribute directly to decision-making.
Extract Supporting Quotes: Pull out direct quotes from participants that illustrate key insights. These quotes can lend credibility and clarity to your findings.
Summarize Key Insights: Create a concise summary of the findings that encapsulates the essential takeaways. This will facilitate easier understanding among stakeholders.
Prepare for Reporting: Organize your insights systematically into a report format, highlighting recommendations based on data analysis to guide future actions.
By synthesizing AI-driven insights through these steps, you strengthen the foundations of AI-driven concept testing, ensuring it becomes a tool for informed innovation.
Exploring Top AI Tools for Concept Testing
In the pursuit of enhancing concept testing, exploring top AI tools can significantly streamline the process. Various solutions offer advanced capabilities for qualitative data analysis, ensuring a more efficient workflow. Tools like Insight7, NVivo, and MAXQDA are leading the charge, providing robust features for data organization and interpretation. These AI-driven solutions can turn raw qualitative data into actionable insights, ultimately supporting better decision-making in concept testing.
Delving deeper, these tools each provide unique functionalities. For instance, some focus on visualizing data relationships while others prioritize user-friendly interfaces for novice analysts. The combination of these tools empowers teams to effectively harness AI in their concept testing efforts, mixing human intuition with AI-driven insights. By utilizing these resources, you can cultivate a more thorough understanding of consumer sentiment and preferences, leading to successful product iterations and enhanced market outcomes.
- Insight7
In the realm of AI-Driven Concept Testing, Insight7 emerges as a powerful ally for qualitative data analysis. This innovative tool streamlines the process of interpreting user feedback, transforming data into actionable insights. Through its advanced algorithms, Insight7 can capture nuanced responses, providing a deeper understanding of consumer preferences. This capability allows businesses to formulate concepts that resonate strongly with their target audience.
Utilizing Insight7 involves several key steps. First, gather qualitative data through surveys, interviews, or open-ended feedback forms. Next, preprocess this data within the platform to identify patterns or recurring themes. Finally, interpret the AI-generated insights to inform your concept testing strategy. By following this structured approach, companies can enhance their concept development process and make informed decisions with confidence. Embracing AI in qualitative analysis not only improves accuracy but also accelerates the time to market for new ideas and products.
- NVivo
NVivo is a powerful qualitative data analysis tool that proves vital for AI-Driven Concept Testing. With its user-friendly interface, NVivo enables researchers to efficiently analyze complex text, audio, and visual data. Importantly, it streamlines the process of identifying patterns and themes, essential for informed decision-making. As you embark on your concept testing journey, NVivo's capabilities allow you to transform raw data into insightful narratives, further enriching the analysis process.
Utilizing NVivo entails a few key components. First, data organization is crucial; users can categorize sources into nodes for easy reference. Second, the software offers advanced query functions that facilitate deep analysis of responses, ensuring no significant trend goes unnoticed. Third, you can create visualizations to communicate findings effectively and engage stakeholders. By integrating NVivo into your qualitative analysis framework, you enhance the overall effectiveness of your AI-Driven Concept Testing efforts.
- MAXQDA
MAXQDA serves as a robust tool for qualitative data analysis, especially in the context of AI-driven concept testing. This software allows researchers to systematically analyze data derived from interviews, focus groups, and other qualitative sources. By employing MAXQDA, users can enhance their workflow, effectively managing and interpreting large volumes of textual data, which is essential for making data-driven decisions.
One key feature of MAXQDA is its ability to automate certain aspects of data analysis. This is particularly beneficial for professionals who juggle multiple projects and need efficient ways to extract insights from their qualitative data. The platform supports various data formats, making it versatile for different research contexts. Additionally, its integration with AI tools simplifies the task of identifying patterns and themes within the data, ultimately leading to more meaningful insights during concept testing. Utilizing MAXQDA in your processes can significantly streamline analyses and bolster the development of insights for your projects.
- Dedoose
Dedoose serves as a powerful tool for qualitative data analysis and streamlining concept testing. This platform allows researchers to effectively upload various data forms, making it easier to glean significant insights from qualitative sources. By harnessing the capabilities of AI, users can analyze interviews and discussions collectively or individually. Data can be effortlessly organized into projects, where themes and quotes are extracted for easier interpretation.
The unique features of Dedoose make it especially useful for AI-driven concept testing. It provides a user-friendly interface that enables the generation of reports and visualizations from qualitative data. These visual insights can facilitate better decision-making, turning complex data into actionable strategies. With a focus on data privacy, users can feel secure knowing their information is handled responsibly while tapping into the full potential of AI to enhance their concept testing processes.
- ATLAS.ti
In the realm of AI-Driven Concept Testing, ATLAS.ti stands out as a robust tool for qualitative data analysis. This software excels at processing complex data sets and generating insights to drive informed decisions. By using ATLAS.ti, researchers can efficiently organize and code data, allowing them to identify key themes that emerge from participant feedback. The software's ability to conduct sentiment analysis further enhances its utility by providing a clear understanding of user attitudes and preferences.
Using ATLAS.ti also streamlines the task of analyzing qualitative data. Its intuitive interface allows users to create detailed analysis kits tailored to specific research objectives. The software can easily pull relevant themes and codes from data, ultimately saving time and effort. By defining goals—such as improving user experience or identifying potential challenges—users can quickly extract vital insights that inform their concept testing strategies. Overall, ATLAS.ti is an invaluable asset for those looking to optimize their approach to AI-Driven Concept Testing.
Conclusion: Maximizing the Impact of AI-Driven Concept Testing
To maximize the impact of AI-Driven Concept Testing, it is essential to embrace the evolving capabilities of qualitative data analysis. By integrating AI tools into concept testing, businesses can unlock deeper insights more efficiently. The speed and accuracy of AI allow for a swift transformation of raw data into actionable recommendations, fostering a more dynamic decision-making process.
Moreover, leveraging AI reduces potential biases that could skew results. By employing structured methodologies and advanced algorithms, organizations can arrive at reliable insights while maintaining high ethical standards around data privacy. Investing in AI-Driven Concept Testing ensures that companies remain competitive, responsive, and attuned to consumer needs in an ever-changing market.
💬 Ask About This Article
Have questions? Get instant answers about this article.