How to Use Predictive Analytics to Plan Staffing More Accurately
-
Hello Insight
- 10 min read
Predictive Staffing Solutions offer a transformative approach to workforce planning by using data-driven insights. In a rapidly changing work environment, organizations struggle to anticipate their staffing needs effectively. This is where predictive analytics can play a crucial role, helping businesses forecast future employee requirements based on historical data and trends.
By integrating these solutions, companies can optimize their hiring processes and reduce unnecessary costs. The ability to predict staffing fluctuations ensures that you maintain the right number of employees, thereby enhancing productivity and efficiency. As we explore the importance and implementation of Predictive Staffing Solutions, it’s essential to understand how they can lead to more accurate staffing decisions.
Analyze & Evaluate Calls. At Scale.
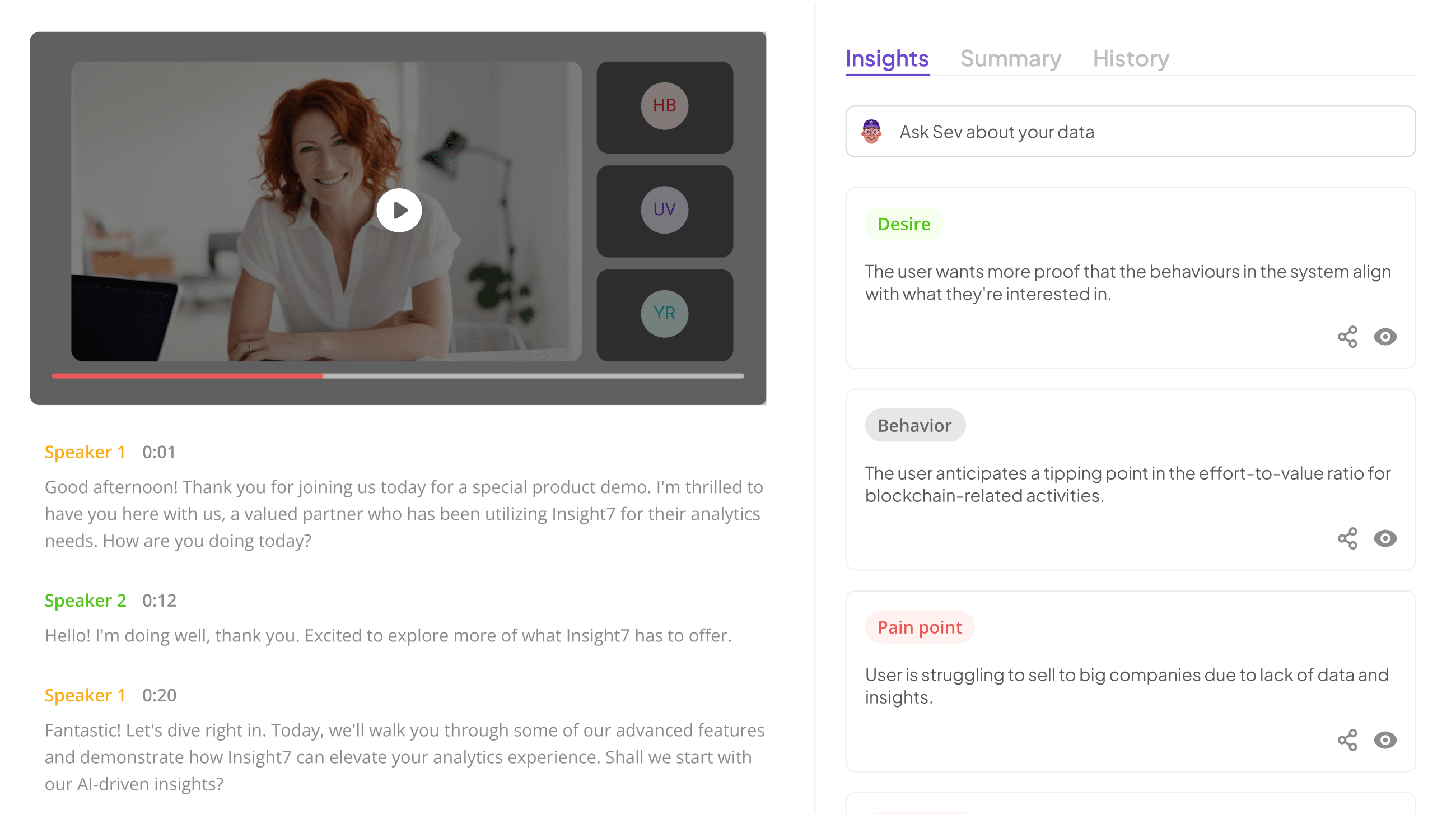
The Importance of Predictive Staffing Solutions in Workforce Planning
Predictive Staffing Solutions play a pivotal role in enhancing workforce planning. By utilizing data-driven insights, organizations can anticipate staffing needs with greater precision. This foresight allows businesses to build a dynamic workforce that adjusts to changing demands, ultimately leading to enhanced operational efficiency.
Accurate workforce planning relies on the ability to predict future staffing requirements based on historical data trends. Predictive Staffing Solutions enable companies to identify patterns, such as peak periods for demand, which can inform hiring strategies. Additionally, these solutions minimize the costs associated with overstaffing or understaffing. When organizations adopt predictive analytics, they not only improve their ability to allocate resources effectively but also enhance employee satisfaction by ensuring optimal workload distribution.
In essence, embracing Predictive Staffing Solutions is essential for any organization seeking to thrive in a competitive market environment. The integration of predictive analytics fosters informed decision-making, making workforce management more strategic and agile.
How Predictive Analytics Enhances Staffing Efficiency
Predictive analytics significantly enhances staffing efficiency by providing organizations with the ability to forecast workforce needs accurately. This process allows businesses to anticipate high-demand periods and adjust staffing levels accordingly, minimizing both overstaffing and understaffing. By utilizing historical data and trends, predictive staffing solutions enable decision-makers to make informed choices about hiring, scheduling, and resource allocation, driving operational effectiveness.
Moreover, the integration of predictive analytics into staffing strategies leads to improved employee satisfaction. With optimized scheduling, workers experience reduced burnout and increased engagement, contributing to overall productivity. Organizations can swiftly adapt to changes in demand while ensuring they maintain a skilled workforce capable of meeting service expectations. By understanding both the "who" and the "how" of their staffing needs, businesses can operate with confidence, ultimately leading to a more dynamic and effective work environment.
Key Benefits of Implementing Predictive Staffing Solutions
Implementing predictive staffing solutions brings numerous advantages that enhance workforce planning accuracy. One key benefit is improved resource allocation, which allows organizations to match staffing levels with actual demand. This reduces the risk of overstaffing or understaffing, ensuring better operational efficiency and cost savings.
Another significant benefit is the ability to identify potential workforce gaps ahead of time. Predictive analytics can analyze historical data, such as employee turnover and seasonal fluctuations, enabling organizations to proactively address staffing challenges. Additionally, these solutions enhance employee engagement by allowing for strategic hires based on anticipated needs and skill requirements. As a result, companies can create a more resilient workforce equipped to adapt to changing demands. Overall, embracing predictive staffing solutions leads to informed decision-making and a more agile approach to workforce management.
Steps to Implement Predictive Staffing Solutions for Accurate Planning
To implement predictive staffing solutions effectively, organizations must follow structured steps that ensure accuracy in workforce planning. First, the collection and analysis of relevant data are critical. This involves gathering historical staffing information, employee performance metrics, and industry trends. By examining this data, organizations can uncover patterns and insights that inform staffing needs.
Next, developing predictive models tailored to specific staffing requirements is essential. These models utilize machine learning algorithms to forecast future staffing levels based on various factors, such as seasonal demand and workload fluctuations. Finally, once the models are implemented, continuous monitoring and refinement must take place. Regular updates and adjustments ensure that the predictive staffing solutions remain effective and aligned with changing business conditions. By following these steps, organizations can enhance their staffing accuracy and optimize workforce efficiency.
Extract insights from interviews, calls, surveys and reviews for insights in minutes
Step 1: Collect and Analyze Relevant Data
To initiate the process of implementing predictive staffing solutions, begin by collecting relevant data. This foundational step involves gathering information from various sources such as employee performance metrics, attendance records, and historical staffing trends. By compiling this data, organizations can start to form a clearer picture of their current staffing situation and potential needs. The accuracy of your predictive models heavily relies on this data, making its quality and comprehensiveness crucial.
Next, engage in a thorough analysis of the collected data. This analysis should identify patterns and trends that could inform future staffing decisions. Consider segmenting data by departments, shifts, or project demands to uncover insights specific to your organization. Understanding these nuances allows for more informed staffing adjustments, ultimately aiding in workforce efficiency. Combining robust data collection with detailed analysis sets the stage for using predictive analytics in a meaningful way, aligning staffing levels with actual business needs.
Step 2: Develop Predictive Models for Staffing
Developing predictive models for staffing is a crucial step in creating effective predictive staffing solutions. This involves analyzing historical data and identifying patterns that influence workforce needs. By using advanced statistical techniques and algorithms, organizations can anticipate staffing requirements and make informed decisions about hiring, training, and resource allocation.
The first stage involves gathering data on various factors, such as peak business periods, employee turnover rates, and seasonal trends. Next, it is essential to select the appropriate modeling techniques, like regression analysis or machine learning, to predict future needs. Finally, organizations should continuously validate and refine these models to ensure they remain accurate and relevant. By embracing predictive analytics in staffing, companies can improve efficiency and significantly reduce costs while meeting operational demands effectively.
Step 3: Implement and Monitor the Models
After developing your predictive models in staffing, the next critical step is to implement and monitor these models effectively. Initially, you must ensure that all stakeholders understand the model's purpose and how it integrates into existing staffing processes. This includes training employees on how to utilize insights generated by predictive staffing solutions for informed decision-making.
Monitoring is vital to evaluate the accuracy and performance of these models. Regularly review the model’s outcomes against actual staffing requirements and adjust parameters as necessary. In this phase, gather feedback from staff who utilize these models in their daily operations. Foster an environment where continuous learning takes place, enabling staff to adapt to using data-driven insights efficiently.
By focusing on the implementation and ongoing assessment of predictive staffing solutions, organizations can enhance their staffing accuracy and responsiveness to changing needs.
Tools for Achieving Predictive Staffing Solutions
To achieve effective predictive staffing solutions, organizations can utilize a range of analytics tools that streamline data analysis and enhance decision-making. These tools help identify staffing needs by analyzing historical data, market trends, and future projections, ensuring resources are allocated efficiently. For instance, using advanced platforms like Tableau allows teams to visualize data easily, enabling quicker insights into staffing requirements.
Equally, platforms such as IBM Watson and Microsoft Azure AI apply machine learning algorithms to predict future staffing demands accurately. Additionally, SAS Analytics provides robust statistical capabilities, which can fine-tune forecasts based on demographic changes and business cycles. Leveraging these tools not only aids in meeting workforce needs accurately but also improves overall operational efficiency, allowing organizations to remain competitive in dynamic environments. Proper integration and usage of these advanced solutions are essential for successful implementation of predictive staffing strategies.
insight7
In the realm of staffing, understanding customer signals and data analytics is critical. Predictive staffing solutions elevate this understanding by providing transformative insights that shape workforce planning. By harnessing data-driven methodologies, organizations can anticipate staffing needs, optimize schedules, and ensure resources align seamlessly with demand fluctuations. This proactive approach addresses traditional workforce challenges, significantly enhancing efficiency.
To implement predictive staffing solutions effectively, organizations should focus on three primary areas. First, collecting and analyzing relevant data is essential for identifying key workforce trends. Second, developing predictive models empowers teams to forecast staffing requirements, tailoring strategies to meet projected demands. Lastly, continuous monitoring of these models allows businesses to adapt and reassess strategies, ensuring that staff planning remains accurate in an ever-changing environment. Embracing this approach positions organizations to thrive amidst competitive pressures.
Tableau
Tableau is a powerful analytics platform that transforms data into actionable insights, facilitating better decision-making for workforce planning. With its user-friendly interface, anyone in an organization can access and utilize Tableau without needing advanced training, democratizing data and making predictive staffing solutions attainable for all employees. This ease of use encourages a culture of data-driven decision-making, significantly enhancing staffing accuracy.
One key feature of Tableau is its ability to visualize complex datasets effectively. By integrating historical staffing data and trends, users can create reports and dashboards that reveal patterns and forecast future staffing needs. As teams collaborate on these insights, they can address potential challenges proactively, ensuring a more efficient allocation of resources. Ultimately, utilizing Tableau enables organizations to implement predictive staffing solutions that not only streamline their operations but also enhance overall effectiveness in workforce management.
SAS Analytics
SAS Analytics serves as a powerful tool in optimizing workforce planning through predictive staffing solutions. By analyzing historical data and trends, organizations can uncover patterns that inform staffing decisions. This analysis emphasizes crucial factors, such as employee turnover rates, seasonal demand fluctuations, and skill gaps, enabling businesses to forecast staffing needs accurately.
To leverage SAS Analytics effectively, organizations should focus on its data integration capabilities and advanced analytics. First, they can gather relevant data from various sources, ensuring a comprehensive overview of workforce dynamics. Next, predictive models can be generated based on this data, allowing teams to simulate different scenarios and their potential staffing impacts. Finally, continuous monitoring ensures adjustments are made as new data emerges. By adopting SAS Analytics, organizations can significantly enhance their staffing strategies, making them more precise and responsive to changing business needs.
IBM Watson
In the realm of predictive staffing solutions, advanced technology plays a critical role. Through sophisticated algorithms, AI systems analyze vast amounts of data, extracting actionable insights for workforce planning. By utilizing predictive analytics, organizations can anticipate staffing needs with greater precision, ensuring the right number of employees are available at any given time. This proactive approach reduces staffing gaps and overloads.
When it comes to optimizing your staffing strategy, it is essential to focus on three key areas. First, gathering historical data about workforce patterns provides the foundation for analysis. Second, developing tailor-made models that reflect specific organizational needs further enhances accuracy. Finally, ongoing monitoring of these models helps maintain effectiveness, adjusting forecasts based on real-time shifts in staffing demands. By embracing these techniques, organizations can significantly enhance planning and operational efficiency.
Microsoft Azure AI
Microsoft Azure AI offers powerful tools to revolutionize workforce planning through Predictive Staffing Solutions. At its core, the platform harnesses advanced machine learning capabilities to analyze historical data and extract valuable insights. By leveraging these insights, organizations can predict staffing needs more accurately, ensuring they have the right number of employees at the right time.
To begin using Microsoft Azure AI, teams can start by gathering diverse datasets, including employee performance metrics, attendance records, and industry trends. Once this data is collected, the platform can develop predictive models that identify potential staffing gaps or surpluses. Regular monitoring of these models ensures that organizations remain agile and able to adjust staffing levels as needed. Ultimately, Microsoft Azure AI empowers businesses to enhance their decision-making process and optimize workforce efficiency, leading to improved operational success.
Conclusion on Predictive Staffing Solutions
Predictive Staffing Solutions offer a robust framework for organizations to plan their workforce with greater accuracy. By incorporating data-driven insights, businesses can anticipate staffing needs based on various factors such as historical trends and market demands. This proactive approach not only optimizes resource allocation but also reduces costs associated with overstaffing or understaffing.
In conclusion, the integration of Predictive Staffing Solutions enables companies to create a responsive workforce planning strategy. Ultimately, organizations that embrace these advanced analytics will find themselves better equipped to meet fluctuating demands while enhancing overall operational efficiency. Competitiveness in today's market hinges on the ability to adapt, making predictive analytics an invaluable asset.