Support Volume Forecasting is a strategic approach that helps organizations anticipate future customer inquiries and support needs. As conversations between customers and support teams grow in volume and complexity, conversation analytics becomes essential. By analyzing these interactions, businesses can reveal patterns, topics, and trends that directly influence support volume.
Utilizing conversation analytics enables organizations to transform raw customer interactions into actionable insights. This data-driven method not only enhances forecasting accuracy but also allows businesses to optimize resource allocation and improve overall customer satisfaction. In the following sections, we will explore the fundamentals of support volume forecasting, practical steps for implementation, and the tools that can aid in this effective analysis.
Analyze qualitative data. At Scale.
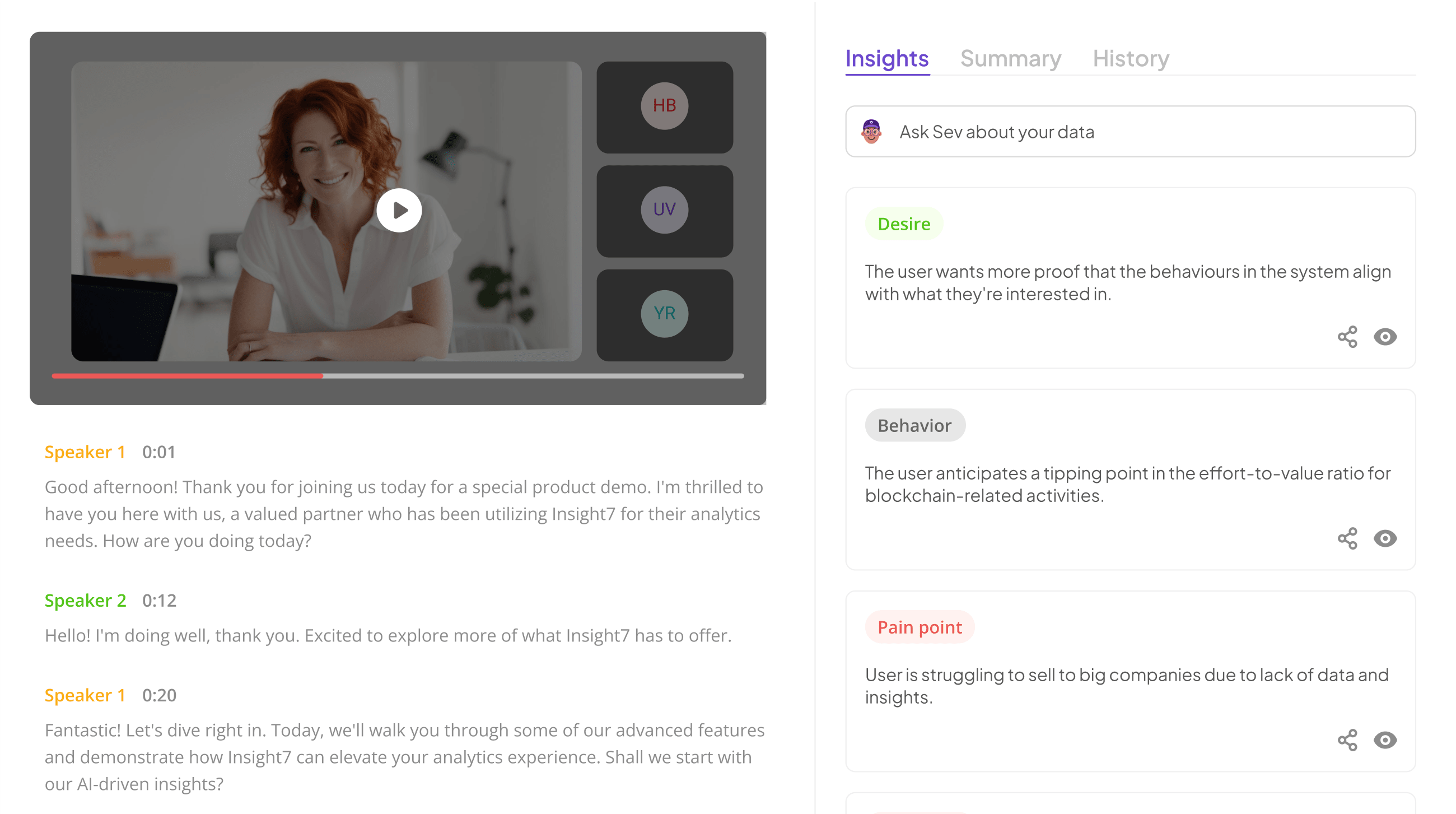
Understanding Support Volume Forecasting with Conversation Analytics
Understanding Support Volume Forecasting with Conversation Analytics provides insights into how businesses can effectively anticipate customer support needs. Leveraging conversation analytics enables organizations to analyze customer interactions systematically. By examining sentiments, keywords, and frequently discussed topics, companies can identify patterns that inform their support strategies.
The forecasting process begins with data collection from various conversation channels. This data is then analyzed to extract key insights, such as common customer pain points and peak inquiry times. Accurate support volume forecasting requires ensuring data quality and implementing templates for consistent analysis. By utilizing conversation analytics, businesses can predict future support demands, allowing them to allocate resources efficiently and improve customer satisfaction. Understanding these elements will empower organizations to enhance their support forecasting efforts, translating conversations into actionable insights.
The Basics of Conversation Analytics
Conversation analytics involves the systematic examination of dialogue data to uncover actionable insights. This process is crucial for organizations looking to improve customer support and forecasting capabilities. By analyzing conversation histories, organizations can identify trends, customer concerns, and recurring issues. This data is invaluable for support volume forecasting, as it allows teams to anticipate future inquiries based on historical patterns.
Key metrics in conversation analytics include sentiment analysis, response times, and common topics discussed. These metrics help organizations gauge customer satisfaction and determine the effectiveness of their support efforts. Additionally, tracking the frequency and type of conversations enables accurate predictions about support volume. Organizations can use these insights to optimize staff allocation and resource planning, ensuring that they are prepared to meet customers' needs. Implementing effective conversation analytics enhances not only operational efficiency but also customer experience.
- Definition and importance
Support Volume Forecasting represents a critical aspect of customer service operations. It involves predicting the volume of incoming customer inquiries, enabling organizations to allocate resources effectively. Understanding and leveraging conversation analytics is essential for building accurate forecasts. By analyzing customer interactions, companies can uncover patterns and trends that directly influence support volume.
The importance of Support Volume Forecasting cannot be overstated. Accurate forecasts lead to better staffing decisions, improved response times, and higher customer satisfaction. Additionally, this process allows businesses to proactively address issues before they escalate, ensuring that support teams are prepared to meet demand. As conversation data provides insights into customer needs, organizations can refine their processes to enhance the overall service experience. This proactive approach not only streamlines support operations but also fosters customer loyalty and trust.
- Key metrics involved in conversation analytics
Key metrics in conversation analytics play a crucial role in support volume forecasting. These metrics transform raw conversation data into actionable insights that drive effective support strategies. One vital metric is the conversation volume itself, which tracks the total number of interactions over a set period. This information helps forecast peak times, ensuring adequate staffing for high-demand periods.
Another essential metric is sentiment analysis, which gauges customer emotions during interactions. Understanding sentiment can guide teams on where to focus their training and resources. Additionally, average response time and resolution time metrics help evaluate efficiency in handling support requests. These figures illuminate areas needing improvement, ultimately promoting a more effective support environment. By honing in on these metrics, organizations can not only anticipate support demands but also tailor their services to improve overall customer satisfaction.
Implementing Support Volume Forecasting
Support Volume Forecasting begins with a meticulous analysis of conversation data to predict customer support needs effectively. This process identifies patterns and trends in customer interactions, enabling organizations to anticipate peak support demand periods. By tracking incoming queries, businesses can better allocate resources, ensuring that help is readily available during high-traffic times.
To implement support volume forecasting, several key steps should be taken. First, gather comprehensive conversation data from various sources, like calls, chats, and emails. Next, analyze this data to draw insights about customer behavior and inquiry types. Additionally, consider external factors such as seasonal trends or market fluctuations that may impact support volume. Lastly, continually refine your forecasting methods by reviewing past performance and adjusting for any emerging patterns. Investing time in these practices not only enhances customer satisfaction but also empowers teams to operate more efficiently.
- How conversation data helps predict support needs
Conversation data is a treasure trove for predicting support needs. When companies analyze interactions with customers, they uncover vital insights that inform future support volume. By identifying common queries, trends, and customer pain points, organizations can anticipate the types of assistance required and adjust their resources accordingly. This proactive approach ensures that support teams are prepared to handle increased inquiries during peak times.
Moreover, examining conversation patterns allows businesses to enhance their service strategies through continuous refinement. For instance, if certain issues appear frequently, it might signal a need for improved training or better resources for customer service representatives. By utilizing conversation data effectively, organizations can shift from reactive to proactive support paradigms. In doing so, they improve efficiency, reduce response times, and ultimately enhance customer satisfaction—key components in effective support volume forecasting.
- Factors affecting the accuracy of forecasts
Several factors can significantly impact the accuracy of support volume forecasting. First, data quality is paramount; inaccuracies or inconsistencies in the conversation data collected can lead to misleading forecasts. Ensuring that all relevant interactions are captured without bias is essential for reliable predictions. Furthermore, the context of conversations matters; seasonal trends, product changes, and customer sentiments can alter support needs unexpectedly.
Another critical element is the analytical methods employed. Sophisticated algorithms and models that account for various variables can enhance predictions, whereas overly simplistic methods may overlook key factors influencing support volume. Additionally, staff training on interpreting data from conversation analytics is vital. Skilled analysts can better understand patterns and anomalies, ultimately resulting in more accurate forecasts. By centering these elements in your strategy, you can optimize your approach to support volume forecasting and ensure it aligns with real-world needs.
Extract insights from interviews, calls, surveys and reviews for insights in minutes
Practical Steps for Using Conversation Analytics
To effectively use conversation analytics for support volume forecasting, begin by gathering relevant conversation data. Identify the tools you will use for data collection, such as Zendesk Explore or Intercom Insights. Ensure the data collected is of high quality by monitoring its relevance and accuracy throughout the process. Clean and categorize the data to eliminate noise, which can skew forecast accuracy.
Next, analyze the collected data to extract meaningful insights. Use analytics tools to identify trends in customer inquiries, pain points, and satisfaction levels. These insights will help you understand the expected support volume and how it may fluctuate. Once analysis is complete, leverage the insights to prepare your support team for upcoming needs, ensuring they are equipped to respond efficiently and effectively. Following these practical steps will empower your organization to forecast support volume accurately and enhance customer experience.
Step 1: Gathering Relevant Conversation Data
To begin effective support volume forecasting, gathering relevant conversation data is essential. Start by collecting recordings and transcripts of customer interactions. Transcription tools can convert audio files into text, enabling easier analysis. This initial step lays the foundation for understanding customer needs and patterns in support requests.
Next, organize the data to maintain quality and relevance. Ensure that the conversations are categorized based on topics like product inquiries, complaints, or feedback. Clean data is critical, as it enhances the accuracy of insights derived from the analytics process. By systematically sorting and analyzing this data, you can identify trends and predict future support volume. This approach ensures you are prepared for shifts in customer needs, ultimately helping your organization deliver timely and effective support.
- Tools for data collection
Collecting the right data is pivotal for effective Support Volume Forecasting. Utilizing various tools and techniques ensures that the data gathered is not only comprehensive but also actionable. Begin by identifying conversational data sources, such as chat logs, emails, and social media interactions. By consolidating this information, you create a robust foundation for your analysis.
Several tools can significantly enhance your data collection. First, customer support platforms like Zendesk and Freshdesk offer built-in analytics features, which streamline data extraction and reporting. Next, platforms such as Intercom and Talkdesk provide advanced tracking capabilities for real-time conversations. Additionally, AI-driven analytics tools like IBM Watson can analyze sentiment and trends, providing deeper insights. Employing these tools allows you to compare patterns, identify customer needs, and ultimately refine your forecasts with precision. Engaging with the right data collection methods strengthens your capacity to anticipate support volume effectively.
- How to ensure data quality and relevance
To ensure data quality and relevance, begin by standardizing the methods of collecting conversation data. Consistency in your data gathering techniques promotes reliability in support volume forecasting. It is crucial to utilize intuitive tools that not only capture conversations but also maintain a structured approach to data entry. This will help eliminate noise and ensure the insights gleaned from the data are applicable and meaningful.
Next, regularly review and update your data sources to address changing market dynamics. Unbiased and accurate data collection methods enhance the relevance of the forecasts you generate. Conduct analysis on a periodic basis to identify patterns and adjust your approach based on findings. Incorporating user feedback directly from conversation data can further refine your analytics process, solidifying the foundation upon which your support volume forecasts rest.
Step 2: Analyzing the Data
In analyzing the data, the first step is to dissect the conversation metrics gathered from various interactions. This insight collection focuses specifically on understanding customer sentiments, pinpointing frequent issues, and identifying emerging trends. By diving deep into these metrics, one can ascertain patterns that signal potential increases or decreases in support volume. You might look for common phrases that correlate with either customer satisfaction or frustration, which can significantly enhance your forecasts.
Next, visualizing this data can be immensely beneficial. Utilize graphs and charts to spot trends over time, making it easier to communicate your findings to stakeholders. Additionally, integrating data from multiple sources, such as different support channels, allows you to paint a clearer picture of the overall support landscape. This thorough analysis not only supports accurate volume forecasting but also aids in strategic decision-making, ultimately leading to improved customer satisfaction.
- Insight7: Leading the way in conversation analytics
Conversation analytics is revolutionizing how businesses approach customer interactions. With the ability to analyze vast amounts of conversational data, companies gain invaluable insights into customer behavior and preferences. This leads to more accurate support volume forecasting, a crucial element for optimizing resources and enhancing customer satisfaction. By understanding what customers are saying, organizations can anticipate trends in support inquiries and adjust their strategies accordingly.
This innovation allows businesses to move beyond reactive customer service. Instead of merely responding to questions as they arise, teams can proactively seek out valuable insights and engage more meaningfully with customers. As organizations harness conversation analytics, they open doors to efficiency and improved collaboration, translating insights into actionable strategies. Ultimately, this proactive approach ensures that businesses are better equipped to meet customer needs and stay ahead in a competitive landscape.
- Other tools to consider:
Exploring additional tools can significantly enhance your approach to support volume forecasting. While you may already be utilizing conversation analytics, considering alternative platforms can provide unique insights and methodologies. Each tool brings specific strengths, allowing for a diverse array of data analysis that can refine your predictions regarding support needs.
Zendesk Explore: This platform offers robust reporting features that allow users to track key metrics effectively. Simple dashboards can be customized, and the integration of various data sources means you gain a comprehensive view of customer interactions.
Intercom Insights: Known for its unique engagement approach, Intercom not only provides conversation metrics but also enables user segmentation. This gives you the opportunity to analyze support volume trends based on user behavior.
Talkdesk: This customer service software focuses on intelligent data routing, which can provide insights into peak support times and patterns. You can fine-tune resource allocation based on these analytics.
Freshdesk Analytics: With user-friendly metrics dashboards, Freshdesk simplifies the tracking of support tickets and customer queries. This aids in turnover predictions, essential for effective resource management.
IBM Watson Analytics: Leveraging artificial intelligence, Watson helps uncover deeper insights from your data. Predictive analysis functions can help forecast future trends, ensuring preparedness for volume spikes.
By considering these tools, you'll enhance the accuracy and efficiency of support volume forecasting, empowering your team to make data-driven decisions.
- Zendesk Explore
Zendesk Explore offers powerful capabilities that can significantly enhance support volume forecasting through conversation analytics. By analyzing data from customer interactions, it provides valuable insights into patterns and trends. This allows support teams to predict future inquiries and adjust staffing levels accordingly.
Utilizing Zendesk Explore means tapping into a range of metrics, from ticket volumes to response times. These metrics help identify peak periods in support requests, enabling teams to prepare effectively. Moreover, the ability to visualize data through dashboards makes it easier to communicate findings and trends across the organization.
In practice, establishing a connection between conversation analytics and support volume forecasting involves understanding key variables, such as customer behavior and seasonal trends. Thus, by integrating analytics tools like Zendesk Explore, organizations can empower their support teams to make informed decisions, ultimately leading to improved customer satisfaction and operational efficiency.
- Intercom Insights
Intercom Insights offers a powerful suite of tools designed to enhance conversation analytics, which is pivotal for effective support volume forecasting. With user-friendly features, any team member can access insights without needing extensive technical training. This democratization of data enables businesses to quickly identify patterns in customer interactions, paving the way for informed decision-making regarding support volume.
Moreover, Intercom’s platform allows you to analyze conversations at both individual and project levels. This means you can scrutinize hundreds of calls simultaneously, extracting vital insights such as customer pain points and desires. By summarizing these interactions, businesses can derive actionable strategies tailored to meet future support demands. Understanding these insights not only optimizes service delivery but also aligns resources to manage anticipated support volume effectively. By leveraging these tools, companies can transform raw conversation data into a roadmap for improved customer experiences and operational efficiency.
- Talkdesk
Conversation analytics plays a crucial role in effectively predicting support volume. By analyzing customer interactions, organizations can uncover trends and patterns that directly influence support needs. This insight allows businesses to proactively manage resources and improve customer satisfaction.
Utilizing conversation analytics enables teams to identify key metrics, such as call volume trends, peak interaction times, and emerging customer concerns. As these conversations generate substantial data, understanding and interpreting this information becomes essential in ensuring timely responses to customer inquiries. With this knowledge, support teams can better prepare for fluctuations in demand and allocate resources efficiently, ultimately enhancing overall service delivery and optimizing support volume forecasting.
Recognizing the potential of conversation analytics paves the way for forward-thinking strategies, allowing organizations to stay one step ahead in meeting customer needs. Such insight not only aids in resource management but also fosters a culture of continuous improvement across customer service operations.
- Freshdesk Analytics
Freshdesk Analytics serves as a powerful tool for organizations aiming to understand their support volume forecasting better. By centralizing conversation data, it aids in identifying trends and patterns that indicate shifts in customer inquiries. This functionality is vital for businesses looking to streamline their support operations effectively.
One of the key features of Freshdesk Analytics is its ability to break down conversation metrics into digestible insights. Users can easily explore factors such as response times, resolution rates, and customer satisfaction feedback. Such analysis not only enhances visibility into current support performance but also empowers teams to make informed predictions about future workload and staffing needs.
The platform facilitates a user-friendly environment, making it accessible for personnel without technical expertise. By interpreting conversation analytics, organizations can strategically prepare for fluctuating support demands, ultimately improving customer service delivery and satisfaction.
- IBM Watson Analytics
IBM Watson Analytics serves as a powerful tool for enhancing support volume forecasting. It provides advanced capabilities to analyze conversational data, helping teams extract insights from customer interactions. By leveraging its effective data visualization and query features, organizations can identify trends in customer feedback and engagement that directly inform support requirements.
This platform empowers users to generate meaningful forecasts about support volume by analyzing both positive and negative customer sentiment. Through capabilities like comparative analysis across different datasets, organizations can pinpoint specific areas for improvement and adapt their support strategies accordingly. Utilizing IBM Watson Analytics not only streamlines the forecasting process but also fosters proactive decision-making, enabling teams to better align resources with anticipated support demands.
Conclusion on Support Volume Forecasting with Conversation Analytics
Utilizing conversation analytics for support volume forecasting offers organizations a proactive approach to managing customer service needs. By analyzing conversations at scale, businesses can extract valuable insights that point to emerging trends and future demands. This method enables teams to anticipate customer inquiries and adjust resources accordingly, ensuring efficient support delivery.
The integration of conversation analytics into forecasting processes ultimately enhances decision-making. By understanding the nuances of customer interactions, organizations can identify key topics and issues affecting support volume. As a result, companies become more agile in responding to customer needs while optimizing support operations for improved outcomes.